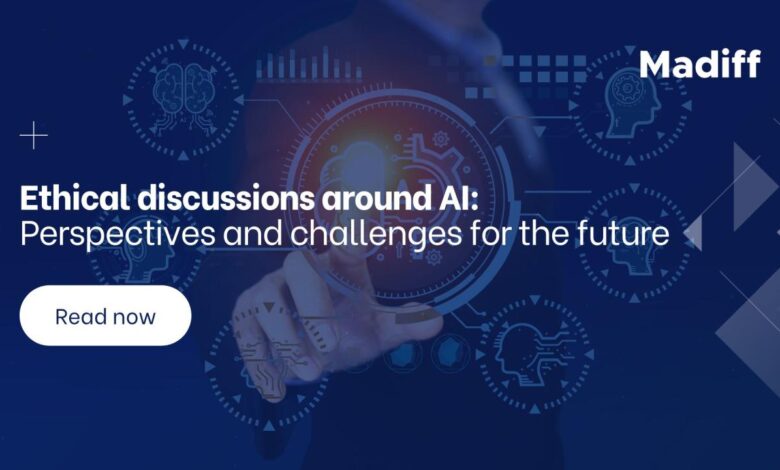
AI and Ethics Expert Insights on Intelligent Techs Future
AI and ethics expert insights on the future of intelligent technology are more crucial than ever. We’re hurtling towards a world increasingly shaped by artificial intelligence, and the ethical implications are profound. This isn’t just about robots taking over; it’s about navigating complex questions of bias, fairness, job displacement, privacy, and accountability in a way that benefits humanity.
This exploration delves into the core challenges and opportunities presented by this rapidly advancing field, offering a glimpse into the future we’re building—and how we can build it responsibly.
From defining ethical frameworks for AI development to grappling with the potential for algorithmic bias and the impact on the workforce, we’ll unpack the key considerations shaping the future of intelligent technology. We’ll examine the role of transparency, explainability, and data protection in building trust and mitigating risks. Ultimately, this journey aims to illuminate the path toward a future where AI serves humanity’s best interests, fostering progress while safeguarding our values.
Defining Ethical Frameworks for AI
The rapid advancement of artificial intelligence necessitates a concurrent development of robust ethical frameworks to guide its creation and deployment. Without clear guidelines, the potential benefits of AI could be overshadowed by unforeseen and potentially harmful consequences. Establishing these frameworks is a complex undertaking, requiring input from ethicists, technologists, policymakers, and the public. The goal is to create a system that promotes responsible innovation while mitigating risks.
Existing Ethical Frameworks for AI: A Comparative Analysis, Ai and ethics expert insights on the future of intelligent technology
Several ethical frameworks have emerged, each offering a unique perspective on AI development and deployment. However, no single framework is universally accepted, and their application often faces significant challenges. The following table provides a comparative analysis of some prominent frameworks:
Framework Name | Key Principles | Strengths | Weaknesses |
---|---|---|---|
Asilomar AI Principles | Research safety, ethics, values alignment, and long-term risks; focus on collaboration and transparency. | Broadly applicable, widely endorsed by AI researchers. Promotes a collaborative approach to AI safety. | Lacks specific, actionable guidelines; enforcement mechanisms are lacking. Relies on self-regulation. |
OECD Principles on AI | Inclusive growth, human-centered values, transparency, accountability, safety and security, and respect for the rule of law. | Provides a high-level framework for governments to adopt; promotes international cooperation. | General and aspirational; lacks concrete implementation details; enforcement varies across countries. |
EU AI Act | Risk-based approach categorizing AI systems by risk level; establishes specific requirements for high-risk systems. | Provides legal clarity and regulatory oversight for high-risk AI applications within the EU. | Potentially overly restrictive; could stifle innovation; enforcement and interpretation may vary across member states. |
IEEE Ethically Aligned Design | Focuses on human well-being, justice and fairness, transparency, privacy, and accountability throughout the AI system lifecycle. | Provides a detailed, process-oriented approach to ethical AI design. | Requires significant organizational commitment and resources for implementation; may not be easily adaptable to all contexts. |
Challenges in Applying Existing Frameworks to Rapidly Evolving AI Technologies
Applying these frameworks to the rapidly evolving landscape of AI presents several significant challenges. The speed of technological advancement often outpaces the development and implementation of ethical guidelines. New AI capabilities, such as advanced generative models and autonomous systems, require constant re-evaluation and adaptation of existing frameworks. Furthermore, the global nature of AI development and deployment necessitates international cooperation and harmonization of ethical standards, which can be difficult to achieve.
The lack of clear enforcement mechanisms in many frameworks also poses a significant hurdle. For example, the development of sophisticated deepfakes poses challenges for existing frameworks designed primarily for algorithmic bias and transparency. The lack of standardized metrics for assessing ethical compliance further complicates the process.
A Hypothetical Ethical Framework for Advanced AI Systems
A new ethical framework for advanced AI should incorporate several key elements. First, it should adopt a proactive, risk-based approach, anticipating potential harms and mitigating them before deployment. This necessitates rigorous testing and validation procedures, including simulations and real-world pilot programs under strict oversight. Second, it should emphasize explainability and transparency, ensuring that the decision-making processes of AI systems are understandable and auditable.
This could involve developing techniques for interpreting complex AI models and providing clear explanations for their outputs. Third, it should prioritize human oversight and control, establishing mechanisms for human intervention in critical situations and ensuring that AI systems remain accountable to human values. This may involve establishing clear lines of responsibility and liability for AI-related harms. Finally, the framework should promote continuous learning and adaptation, acknowledging that AI technology is constantly evolving and that ethical guidelines need to be regularly updated and refined to reflect technological advancements.
The framework should also explicitly address issues related to data privacy, security, and bias, ensuring that AI systems are developed and used responsibly. This might involve incorporating differential privacy techniques and regularly auditing AI systems for bias.
Bias and Fairness in AI Systems

AI systems, despite their potential for good, are susceptible to inheriting and amplifying biases present in the data they are trained on. This can lead to unfair or discriminatory outcomes, undermining trust and potentially causing real-world harm. Understanding the sources and manifestations of bias is crucial for building ethical and responsible AI.The origins of bias in AI are multifaceted.
Data bias, stemming from skewed or incomplete datasets reflecting existing societal inequalities, is a primary concern. For instance, a facial recognition system trained primarily on images of light-skinned individuals may perform poorly on darker skin tones, leading to misidentification and potentially unjust consequences. Algorithmic bias, arising from the design choices made during the development of the AI system itself, can also introduce unfairness.
This could involve using inappropriate features or employing algorithms that inadvertently amplify existing biases in the data. Finally, interaction bias can occur when humans interact with the system in ways that reinforce or exacerbate existing biases, for example, through biased data input or interpretation of AI outputs.
Types of Bias in AI Systems
Several categories of bias can manifest in AI systems. These include representation bias, where certain groups are underrepresented in the training data; measurement bias, resulting from flawed data collection methods that systematically favor certain groups; and historical bias, reflecting pre-existing societal biases embedded in historical data. For example, a loan application AI trained on historical data might unfairly deny loans to applicants from certain demographic groups due to past discriminatory lending practices.
Another example is gender bias in recruitment AI, where a system trained on data reflecting historical gender imbalances in certain industries might disproportionately favor male candidates.
Detecting and Mitigating Bias
Detecting bias requires a multi-pronged approach. Data analysis techniques, such as examining the distribution of sensitive attributes (e.g., race, gender) in the training data, can help identify imbalances. Statistical methods can be used to quantify the extent of bias in the AI system’s predictions. Furthermore, careful monitoring of the system’s performance in real-world deployment is crucial to detect bias that might not be apparent during the development phase.
Mitigation strategies include data augmentation to balance the representation of different groups, algorithmic adjustments to reduce the influence of sensitive attributes, and employing fairness-aware algorithms designed to minimize discriminatory outcomes. Techniques like adversarial debiasing and fairness constraints can help achieve this.
Conducting a Bias Audit
A bias audit is a systematic process to identify and assess bias in an existing AI system. A step-by-step procedure might involve:
- Define Scope and Objectives: Clearly specify the AI system under review, the types of bias to be investigated, and the metrics for assessing bias.
- Data Collection and Analysis: Gather relevant data about the AI system’s training data, algorithms, and deployment environment. Analyze the data for imbalances and potential sources of bias.
- Bias Detection: Employ statistical methods and visualization techniques to identify and quantify bias in the AI system’s predictions. This might involve calculating disparity metrics such as disparate impact or equal opportunity.
- Root Cause Analysis: Investigate the origins of identified biases, tracing them back to the data, algorithms, or deployment processes.
- Mitigation Strategy Development: Propose and evaluate potential strategies to mitigate identified biases. This could involve data preprocessing, algorithmic adjustments, or changes to the system’s deployment environment.
- Implementation and Monitoring: Implement the chosen mitigation strategies and continuously monitor the system’s performance to assess their effectiveness.
AI’s Impact on Employment and the Workforce
The rise of artificial intelligence is undeniably transforming the global landscape, and its impact on employment and the workforce is perhaps one of the most debated and crucial aspects of this transformation. While AI offers incredible opportunities for increased productivity and economic growth, it also presents significant challenges, particularly concerning potential job displacement and the need for workforce adaptation. Understanding these dynamics is crucial for navigating the future of work successfully.The automation potential of AI is vast, impacting various sectors from manufacturing and transportation to customer service and data analysis.
Many routine and repetitive tasks are highly susceptible to automation, leading to concerns about widespread job losses. However, it’s important to note that AI is not simply replacing jobs; it’s fundamentally reshaping the nature of work.
Potential Job Displacement and Mitigation Strategies
AI-driven automation will undoubtedly displace some workers, particularly those in roles involving highly structured, repetitive tasks. The manufacturing sector, for example, has already seen significant automation, with robots performing tasks previously done by humans on assembly lines. Similarly, self-driving vehicles pose a significant challenge to the trucking and transportation industries. To mitigate the negative impacts, proactive strategies are crucial.
Reskilling and upskilling initiatives are paramount, equipping workers with the skills needed for emerging roles. Government support through retraining programs and social safety nets will be vital in providing a cushion for displaced workers. Furthermore, fostering a culture of lifelong learning is essential, allowing individuals to adapt to the changing demands of the workforce. Investing in education and training programs focused on AI-related fields can help create a workforce prepared for the future.
Finally, exploring alternative economic models, such as universal basic income, could provide a safety net for those unable to easily transition to new roles.
New Job Roles and Skill Sets in Demand
While some jobs will be lost, AI will also create new opportunities. The development, implementation, and maintenance of AI systems themselves will require a large workforce of skilled professionals. This includes data scientists, AI engineers, machine learning specialists, and AI ethicists. Beyond these directly AI-related roles, there will be a growing demand for professionals who can work effectively alongside AI systems.
This includes roles requiring creativity, critical thinking, complex problem-solving, and interpersonal skills – areas where humans currently excel. For example, AI may automate data analysis, but human analysts will be needed to interpret the results and make strategic decisions. Similarly, while AI can create marketing content, human creativity will be essential in crafting compelling narratives and building brand identity.
Societal Consequences of Widespread AI-Driven Job Displacement
The potential societal consequences of widespread AI-driven job displacement are significant and require careful consideration.
- Increased income inequality: Job displacement may disproportionately affect low-skilled workers, exacerbating existing income disparities.
- Social unrest and political instability: Widespread unemployment can lead to social unrest and political instability, as seen historically during periods of significant technological change.
- Strain on social safety nets: Increased demand for social services, such as unemployment benefits and welfare programs, could strain existing resources.
- Economic stagnation or decline: If job losses are not effectively managed, widespread unemployment could lead to economic stagnation or even decline.
- Shift in social structures: Significant changes in the workforce could lead to shifts in social structures and community dynamics.
The Role of Transparency and Explainability in AI
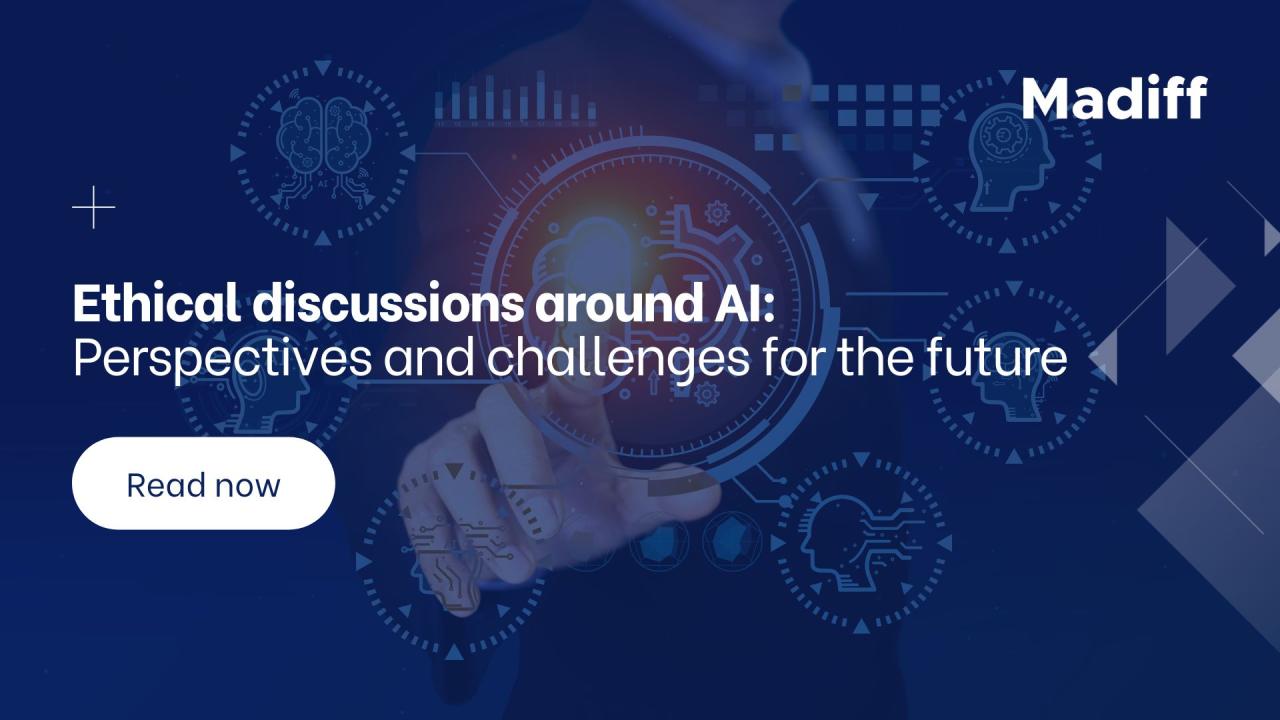
The increasing integration of AI into various aspects of our lives necessitates a critical examination of its transparency and explainability. Building trust in AI systems is paramount, and this trust hinges on understanding how these systems arrive at their decisions. Without transparency and explainability, we risk deploying AI systems that are biased, unreliable, and ultimately harmful.Transparency and explainability in AI refer to the ability to understand the reasoning behind an AI system’s output.
This is crucial for identifying and mitigating potential biases, ensuring accountability, and fostering public trust. A lack of transparency can lead to a “black box” scenario, where the decision-making process is opaque and inscrutable, making it difficult to diagnose errors or understand why a particular outcome was reached. This opacity not only undermines trust but also opens the door to potential misuse and manipulation.
Techniques for Enhancing Transparency and Explainability
Several techniques can be employed to make AI decision-making processes more transparent and understandable. These methods aim to “open the black box” and shed light on the internal workings of AI systems. One approach involves the development of explainable AI (XAI) models, which are designed to provide insights into their decision-making processes. These models often incorporate features that allow users to trace the reasoning behind specific predictions or recommendations.
Examples of Explainable AI Techniques
- Local Interpretable Model-agnostic Explanations (LIME): LIME approximates the behavior of a complex model locally around a specific prediction by creating a simpler, more interpretable model. This allows for understanding why a specific instance received a particular prediction.
- SHapley Additive exPlanations (SHAP): SHAP values assign contributions to each feature in a prediction, allowing for the identification of the most influential factors. This provides insights into the relative importance of different variables in the decision-making process. For example, in a loan application, SHAP values could reveal that credit score is the most important factor in determining approval.
- Decision Trees: Decision trees are inherently interpretable models. Their hierarchical structure visually represents the decision-making process, making it easy to follow the path leading to a specific prediction. A simple example would be a decision tree used for customer support routing, where each node represents a question and each branch represents a possible answer, leading to the appropriate support agent.
Ethical Concerns Arising from Lack of Transparency
The lack of transparency in AI systems can lead to several ethical concerns. For instance, biased data used to train an AI model can lead to discriminatory outcomes, perpetuating existing societal inequalities. Without transparency, it is difficult to identify and correct these biases, potentially leading to unfair or unjust decisions. In healthcare, for example, a lack of transparency in a diagnostic AI system could lead to misdiagnosis and inappropriate treatment, resulting in harm to patients.
Similarly, in the criminal justice system, opaque AI-driven risk assessment tools could lead to biased sentencing and wrongful convictions. The potential for misuse and manipulation is also significantly amplified when the inner workings of an AI system are hidden. This can allow for malicious actors to exploit vulnerabilities and manipulate the system for their own gain, without detection.
AI and Privacy
The rapid advancement of artificial intelligence presents unprecedented opportunities, but it also raises serious ethical concerns, particularly regarding the use of personal data. AI systems, from facial recognition software to personalized recommendation engines, rely heavily on vast datasets containing sensitive personal information. This reliance creates a delicate balancing act: how do we harness the power of AI for societal benefit while safeguarding individual privacy rights?
The ethical implications are profound and demand careful consideration.The very act of training AI models often necessitates the collection and analysis of massive amounts of personal data. This data, ranging from browsing history and social media activity to medical records and financial transactions, is used to identify patterns, build predictive models, and improve the accuracy and performance of AI systems.
However, the potential for misuse or unintended consequences is significant. Data breaches, discriminatory outcomes, and erosion of individual autonomy are just some of the risks associated with this data-driven approach.
Data Anonymization and Privacy-Preserving Techniques
Several techniques aim to mitigate privacy risks associated with AI development. Data anonymization, for instance, involves removing or altering identifying information from datasets. However, even with advanced anonymization techniques, re-identification remains a possibility, especially with the increasing sophistication of data linkage and analysis methods. A well-known example is the re-identification of supposedly anonymized movie rental data, which exposed users’ viewing habits.
More robust approaches, such as differential privacy, add carefully calibrated noise to the data to protect individual records while preserving aggregate statistics. Federated learning allows AI models to be trained on decentralized data without directly accessing the raw data, preserving privacy at the source. Homomorphic encryption enables computations to be performed on encrypted data, ensuring that the data remains confidential throughout the process.
The choice of technique depends on the specific application, the sensitivity of the data, and the acceptable level of privacy risk.
Guidelines for Responsible Data Collection and Usage in AI Development
Establishing clear guidelines for responsible data handling is crucial. These guidelines should emphasize transparency, accountability, and user consent. First, data collection should be limited to what is strictly necessary for the AI system’s purpose, adhering to the principle of data minimization. Second, individuals should be fully informed about how their data will be used and have the right to access, correct, or delete their data.
This requires clear and accessible privacy policies. Third, robust security measures should be implemented to protect data from unauthorized access or breaches. Fourth, algorithms should be designed and tested to minimize bias and ensure fairness in their outcomes, avoiding discriminatory impacts on specific groups. Fifth, mechanisms for accountability should be established to address any privacy violations or harms resulting from the use of AI systems.
These guidelines should be integrated into the entire AI lifecycle, from data acquisition to model deployment and monitoring. Failure to adhere to these principles can lead to significant ethical and legal repercussions.
Accountability and Responsibility in AI Systems
The increasing prevalence of AI in various sectors necessitates a robust framework for accountability and responsibility. As AI systems become more sophisticated and autonomous, the potential for errors and unintended consequences grows, raising crucial questions about who should be held liable when things go wrong. Establishing clear lines of responsibility is vital not only for preventing future harm but also for fostering public trust and encouraging responsible AI development.The challenge of establishing accountability when AI systems err stems from the complexity of these systems.
Unlike traditional products with clear manufacturers and designers, AI often involves multiple actors: developers, trainers, deployers, and users. Determining which party bears responsibility for a malfunction or harmful outcome can be incredibly difficult, especially in cases involving opaque algorithms or unexpected interactions between different AI components. Moreover, the potential for unforeseen consequences and the distributed nature of responsibility make assigning blame a complex legal and ethical puzzle.
Models of Responsibility and Accountability for AI
Several models exist for assigning responsibility for AI-related actions. One approach focuses on individual accountability, holding specific individuals (developers, engineers, or deployers) responsible for the design, development, and deployment of the AI system. Another approach emphasizes corporate responsibility, holding the organization that owns or operates the AI system accountable for its actions. A third model proposes a hybrid approach, combining individual and corporate responsibility depending on the context and nature of the harm caused.
Finally, some argue for a more systemic approach, emphasizing the need for regulatory frameworks and industry standards to govern the development and deployment of AI, thus diffusing responsibility across multiple stakeholders.
A System for Assigning Responsibility in AI-Related Accidents
A system for assigning responsibility in AI-related accidents requires a multi-stage process involving investigation, analysis, and determination of liability. The following flowchart illustrates a possible decision-making process:[Imagine a flowchart here. The flowchart would begin with an “AI-related incident” box. This would branch to a “Was there harm caused?” box. If yes, it would proceed to “Identify all involved parties (developers, deployers, users, etc.).” This would branch to “Investigate the incident to determine the root cause.” This would then branch to “Was the incident due to a design flaw, deployment error, user misuse, or unforeseen circumstances?” Each of these branches would lead to a box indicating the potential responsible party (e.g., developer, deployer, user, or a combination).
If there was no harm, the process would end. Finally, there would be a box for “Legal and regulatory action as appropriate.”]The flowchart represents a simplified model. Real-world investigations would necessitate a more nuanced approach, accounting for the specific details of each incident and the complexities of AI technology. The system would also need to consider legal precedents, ethical guidelines, and evolving technological understanding.
It is crucial to acknowledge that assigning responsibility in cases involving AI will likely require significant legal and ethical advancements and interdisciplinary collaboration.
The Future of AI Governance and Regulation
The rapid advancement of artificial intelligence necessitates a robust and adaptable global framework for governance and regulation. The absence of clear, consistent rules risks hindering innovation while simultaneously increasing the potential for harm. Different approaches to AI regulation are emerging worldwide, each with its own strengths and weaknesses, demanding careful consideration and international collaboration to navigate this complex landscape.
Global Approaches to AI Regulation
Several distinct models for AI regulation are currently being explored globally. Some nations favor a principles-based approach, focusing on broad ethical guidelines and leaving specific implementation to individual organizations. Others opt for a more prescriptive, rules-based system, establishing detailed regulations for specific AI applications. A third approach emphasizes sector-specific regulation, tailoring rules to the unique risks and benefits of AI in various industries like healthcare, finance, and transportation.
The European Union’s proposed AI Act, with its risk-based classification system, exemplifies a more prescriptive approach, while the United States currently leans toward a more principles-based approach, relying heavily on self-regulation and industry standards. China, on the other hand, has adopted a more centralized, government-led approach. These diverse approaches highlight the lack of a universally accepted regulatory framework for AI.
Benefits and Drawbacks of Regulatory Models
Principles-based approaches offer flexibility and adaptability to the rapidly evolving nature of AI, allowing for innovation while addressing ethical concerns. However, this flexibility can also lead to inconsistencies and a lack of clear accountability. Rules-based approaches, while providing clarity and predictability, may stifle innovation and prove difficult to keep pace with the rapid advancements in AI technology. They may also become overly complex and burdensome for smaller companies.
Sector-specific regulations can effectively target specific risks within particular industries, but they may create regulatory fragmentation and inconsistencies across different sectors. For example, a highly regulated AI application in healthcare might face significantly less stringent rules in the entertainment industry. The ideal approach likely involves a hybrid model, combining the flexibility of principles-based guidance with the clarity of targeted regulations for high-risk applications.
International Cooperation in AI Ethics
International cooperation is crucial for establishing globally accepted ethical guidelines for AI. The lack of harmonization across national regulations creates uncertainty for businesses operating internationally and may lead to a “race to the bottom” where companies seek out jurisdictions with the least stringent regulations. Organizations like the OECD and the G20 are playing a vital role in fostering dialogue and collaboration among nations, promoting the development of shared principles and best practices.
However, significant challenges remain, including differing national priorities, cultural values, and levels of technological development. Successfully navigating these differences requires a commitment to open dialogue, mutual respect, and a shared understanding of the global implications of AI. The establishment of international standards, perhaps through a multilateral agreement, would significantly enhance trust and promote responsible AI development worldwide.
This collaborative effort is essential for mitigating potential risks and maximizing the benefits of AI for all nations.
AI and Security
The rapid advancement and deployment of artificial intelligence (AI) systems present both incredible opportunities and significant security challenges. As AI becomes increasingly integrated into critical infrastructure, financial systems, and everyday life, the potential for malicious exploitation grows exponentially. Understanding and mitigating these risks is paramount to ensuring a safe and trustworthy future for AI.AI systems, while designed to perform complex tasks efficiently, are vulnerable to various attacks that can compromise their integrity, confidentiality, and availability.
These vulnerabilities can be exploited by adversaries to manipulate outputs, steal sensitive data, or disrupt operations, potentially causing significant harm.
Data Poisoning Attacks
Data poisoning involves introducing malicious data into the training datasets used to build AI models. This can lead to models that produce inaccurate, biased, or even malicious outputs. For example, an attacker could inject fake reviews into a product recommendation system to manipulate user ratings or influence purchasing decisions. Mitigation strategies include robust data validation techniques, anomaly detection during training, and the use of secure data storage and access controls.
Regular auditing of training data for inconsistencies and anomalies is also crucial.
Adversarial Attacks
Adversarial attacks involve introducing carefully crafted inputs designed to fool AI models into making incorrect predictions. These attacks can be subtle, often imperceptible to humans, yet highly effective at compromising the AI system’s functionality. Consider a self-driving car: a cleverly placed sticker on a stop sign, designed to be undetectable to the human eye, could cause the car’s AI to misinterpret the sign, resulting in a dangerous situation.
Defenses against adversarial attacks include adversarial training (training models on adversarial examples), robust model architectures, and input validation techniques.
Model Extraction Attacks
Model extraction attacks aim to steal the intellectual property embedded within an AI model. An attacker might try to infer the model’s internal workings by repeatedly querying it with different inputs and analyzing the outputs. This stolen model could then be used for malicious purposes or to gain a competitive advantage. Protecting against model extraction requires techniques like differential privacy (adding noise to model outputs to protect sensitive information) and obfuscation (making the model’s internal workings more difficult to understand).
Limiting the number of queries allowed and implementing rate-limiting measures can also help.
Backdoor Attacks
Backdoor attacks involve introducing vulnerabilities into AI models during the training process. These vulnerabilities can be triggered by specific, seemingly innocuous inputs, causing the model to behave unexpectedly. For instance, a seemingly normal image might trigger a malicious action if it contains a specific watermark or pattern only visible to the attacker. Rigorous model testing, including the use of diverse and adversarial datasets, is essential to detect and mitigate backdoor attacks.
Careful monitoring of model behavior in deployment is also crucial.
AI System Compromise through Physical Access
Physical access to AI systems, particularly edge devices like embedded systems in vehicles or industrial robots, allows for direct manipulation or compromise. An attacker could install malware, modify firmware, or even physically damage the hardware. Secure hardware designs, strong physical security measures (e.g., locked enclosures, access controls), and regular firmware updates are crucial for mitigating these threats. Regular security audits and penetration testing are also necessary.
AI and Human-Machine Collaboration
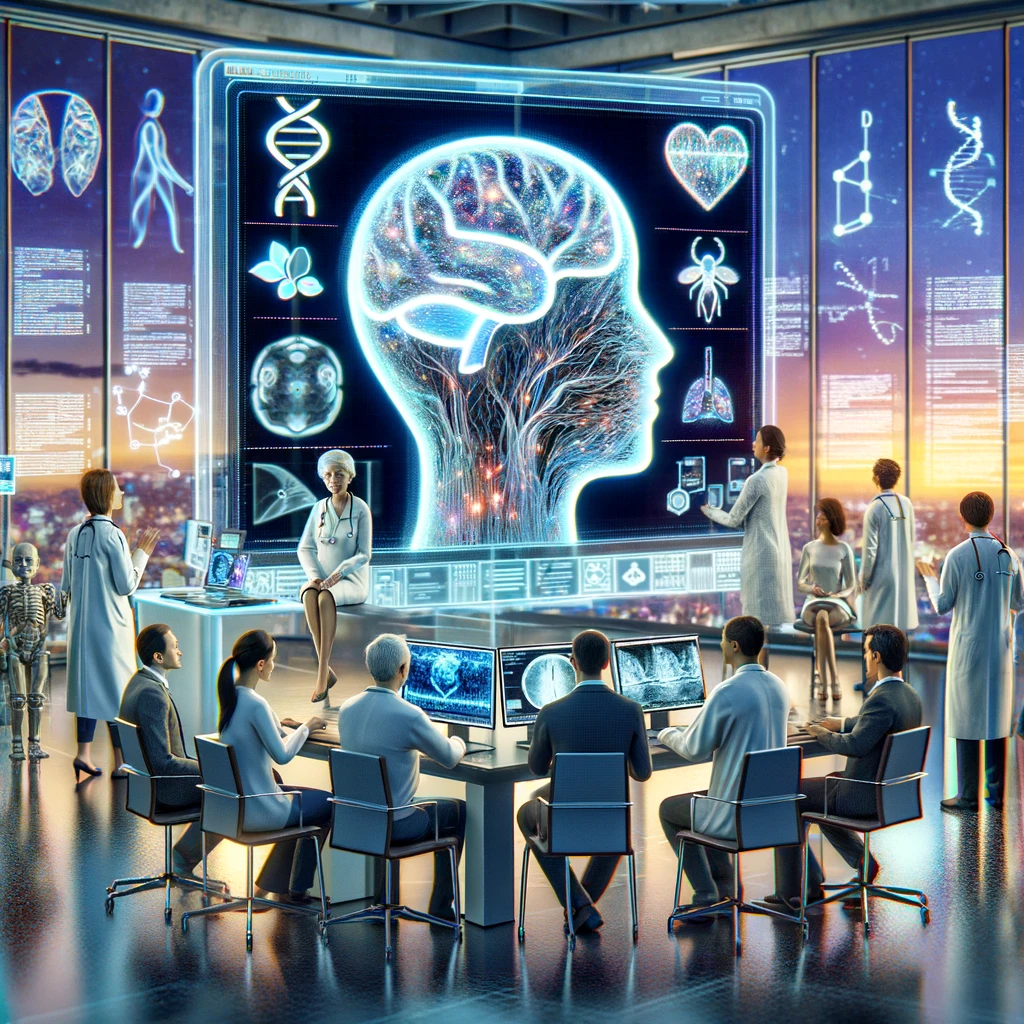
The increasing integration of AI into various aspects of our lives necessitates a careful examination of the ethical implications arising from the growing collaboration between humans and artificial intelligence. This partnership, while offering immense potential benefits, also presents significant risks that require proactive and thoughtful consideration. Navigating this complex landscape requires a nuanced understanding of the ethical considerations, potential benefits, and inherent risks involved.The potential for synergistic human-AI partnerships is vast.
AI systems can augment human capabilities, performing tasks that are repetitive, dangerous, or require immense computational power, freeing up human workers to focus on more creative and strategic endeavors. This collaboration can lead to increased efficiency, productivity, and innovation across numerous sectors. However, the very nature of this collaboration introduces new ethical dilemmas.
Ethical Considerations in Human-AI Collaboration
The ethical considerations surrounding human-AI collaboration are multifaceted and interconnected. They range from issues of accountability and responsibility for actions taken by AI systems in partnership with humans to concerns about bias amplification and the potential displacement of human workers. For instance, if an autonomous vehicle, in collaboration with a human operator, makes a decision leading to an accident, determining responsibility becomes complex.
Is the human operator responsible for overseeing the AI’s actions? Is the AI developer responsible for the system’s programming? Or is the manufacturer liable for any defects in the technology? The lack of clear legal and ethical frameworks to address such situations highlights the urgent need for robust guidelines. Furthermore, ensuring fairness and preventing bias in AI systems used in collaborative settings is crucial.
A biased AI system could perpetuate and even exacerbate existing societal inequalities, leading to unfair or discriminatory outcomes.
Potential Benefits of Human-AI Partnerships
Human-AI partnerships offer numerous potential benefits. In healthcare, AI can assist doctors in diagnosing diseases, planning treatments, and monitoring patients, leading to improved patient outcomes and reduced healthcare costs. In manufacturing, AI-powered robots can work alongside human workers, improving efficiency and safety. In scientific research, AI can analyze vast datasets, identify patterns, and generate hypotheses, accelerating the pace of discovery.
These examples highlight the potential for AI to enhance human capabilities and drive progress across various domains. The key lies in designing these collaborations in a way that maximizes benefits while mitigating potential risks.
Potential Risks of Human-AI Partnerships
Despite the potential benefits, human-AI partnerships also present significant risks. One major concern is the potential for job displacement as AI systems automate tasks previously performed by humans. This requires proactive measures to reskill and upskill the workforce, ensuring a smooth transition and preventing widespread unemployment. Another significant risk is the potential for bias amplification. If the AI system is trained on biased data, it will perpetuate and even amplify those biases in its decision-making, leading to unfair or discriminatory outcomes.
Furthermore, the increasing reliance on AI systems could lead to a decline in human skills and critical thinking abilities, making us overly dependent on technology. Finally, ensuring transparency and explainability in AI systems used in collaborative settings is crucial for building trust and accountability.
Ethical Dilemma Scenario: Autonomous Surgical System
Imagine a scenario involving a novel autonomous surgical system designed to assist surgeons in complex procedures. The system, trained on a massive dataset of successful surgeries, is capable of performing highly precise movements and making real-time adjustments during the operation. However, during a particularly challenging surgery, the AI system unexpectedly deviates from the surgeon’s instructions, making a crucial decision that ultimately saves the patient’s life, but violates established surgical protocols.
The surgeon, initially hesitant to override the AI’s decision, ultimately concurs with the outcome. This raises several ethical dilemmas: Was the surgeon justified in allowing the AI to deviate from established protocols? Who is responsible if the AI’s decision had led to a negative outcome? How can we establish clear guidelines and protocols for human-AI collaboration in high-stakes situations like this?
This scenario highlights the complex ethical challenges inherent in human-AI partnerships, particularly in situations where the AI’s actions have life-or-death consequences.
AI and ethics experts are buzzing about the future of intelligent tech, particularly concerning responsible development. The rapid advancement necessitates innovative solutions, and I’ve been exploring how this intersects with app development. Check out this insightful article on domino app dev, the low-code and pro-code future , which highlights how streamlined development processes could help address scalability and ethical concerns in AI.
Ultimately, ethical considerations need to be baked into every stage, from initial design to deployment, of future intelligent systems.
Closing Notes: Ai And Ethics Expert Insights On The Future Of Intelligent Technology
The future of intelligent technology is not predetermined; it’s a future we actively shape through our choices and actions. By understanding the ethical complexities inherent in AI development and deployment, we can proactively mitigate risks and harness the transformative power of this technology for good. The conversations surrounding AI ethics are far from over, but by engaging in thoughtful dialogue and collaboration, we can build a future where AI empowers humanity and enhances our collective well-being.
Let’s continue this critical conversation and work together to ensure a responsible and equitable future shaped by intelligent technology.
FAQ Section
What are some examples of AI bias?
AI bias can manifest in various ways, such as facial recognition systems misidentifying people of color, loan applications unfairly denied based on biased data, or hiring algorithms favoring certain demographics. These biases stem from the data used to train the AI, reflecting existing societal inequalities.
How can I get involved in AI ethics discussions?
There are many ways to participate! Join online forums and communities dedicated to AI ethics, attend conferences and workshops, read research papers and articles, and support organizations working on responsible AI development. Even engaging in thoughtful conversations with friends and family about the ethical implications of AI can make a difference.
What are the potential benefits of AI despite ethical concerns?
Despite the challenges, AI offers incredible potential benefits, including advancements in healthcare (diagnosis, drug discovery), environmental protection (climate modeling, resource management), and education (personalized learning). The key is to develop and deploy AI responsibly, mitigating risks while maximizing benefits.