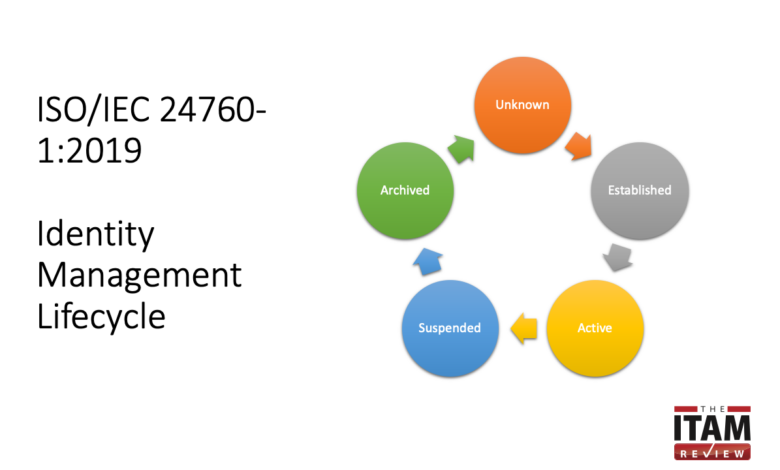
AI for Identity Security 5 Ways AI Boosts SecOps & IAM
Ai for identity security 5 ways ai augments secops and iam teams today – AI for Identity Security: 5 Ways AI augments SecOps and IAM teams today – that’s a mouthful, but it perfectly captures the exciting revolution happening in cybersecurity. We’re no longer relying solely on outdated rule-based systems; AI is transforming how we protect our digital identities and critical data. This post dives into five key ways AI is making our systems stronger, faster, and smarter, ultimately helping security and IAM teams stay ahead of the ever-evolving threat landscape.
Get ready to explore the future of identity security!
From detecting subtle anomalies in login patterns to automating user provisioning and enhancing multi-factor authentication, AI is proving to be a game-changer. We’ll look at the practical applications, benefits, and challenges of implementing AI-driven security measures, providing a clear picture of how these technologies are already impacting organizations of all sizes. We’ll even touch on the ethical considerations and potential biases, ensuring a well-rounded perspective.
AI-Driven Anomaly Detection in Identity and Access Management (IAM)
AI is revolutionizing identity security, offering powerful new tools to detect and respond to sophisticated threats. One of the most impactful applications is AI-driven anomaly detection within Identity and Access Management (IAM) systems. This technology goes beyond traditional rule-based approaches, leveraging machine learning to identify subtle deviations from established user behavior patterns, ultimately strengthening overall security posture.AI algorithms analyze vast quantities of IAM data, including login attempts, access requests, and user activity, to build a baseline of “normal” behavior for each user and system.
Any significant departure from this baseline triggers an alert, indicating a potential security breach. This proactive approach significantly improves threat detection and response capabilities.
Anomaly Detection Methods and Response Actions
The effectiveness of AI-driven anomaly detection hinges on the ability to accurately identify unusual activity while minimizing false positives. The following table illustrates several common anomaly types, the AI methods used for detection, the appropriate response actions, and strategies to reduce false positives.
Anomaly Type | Detection Method | Response Action | False Positive Mitigation |
---|---|---|---|
Unusual Login Location | Geographic location analysis compared to user’s typical login locations; machine learning model trained on historical location data. | Account lockout; multi-factor authentication (MFA) challenge; security alert notification. | Establish a flexible geolocation policy allowing for occasional deviations; incorporate user-defined trusted locations. |
Suspicious Access Pattern | Analysis of access frequency, time of day, and accessed resources compared to historical patterns; unsupervised learning techniques to identify outliers. | Investigation of user activity; temporary access restriction; security audit initiation. | Fine-tune anomaly detection thresholds; incorporate user roles and permissions into the analysis. |
Abnormal Data Access Volume | Monitoring data access volume and speed; identification of unusually high or low activity compared to historical baselines. | Account review; data loss prevention (DLP) system activation; investigation for data exfiltration attempts. | Establish realistic data access thresholds based on user roles and typical workloads; account for seasonal variations in activity. |
Unauthorized Privilege Escalation | Monitoring changes in user privileges and permissions; detection of unusual privilege assignments or elevation attempts. | Immediate privilege revocation; security incident response; system log analysis for root cause determination. | Implement strict change management processes; regularly audit user permissions; use least privilege access principle. |
Benefits of AI-Powered Anomaly Detection
AI-powered anomaly detection offers significant advantages over traditional rule-based systems. Rule-based systems rely on pre-defined rules, making them inflexible and vulnerable to sophisticated attacks that evade established patterns. In contrast, AI systems adapt to evolving threats, learning from new data and adjusting detection thresholds accordingly. This adaptive capability enables them to identify novel attack techniques that would otherwise go undetected.
For example, AI can effectively mitigate insider threats by detecting subtle changes in employee behavior indicative of malicious intent, such as unusual access patterns to sensitive data or communication with external entities. Furthermore, AI can help detect and prevent credential stuffing attacks, where attackers use lists of stolen credentials to attempt to gain unauthorized access to accounts.
Implementation Challenges of AI-Driven Anomaly Detection
While AI-driven anomaly detection offers substantial benefits, its implementation presents several challenges. High-quality data is crucial for training accurate AI models. Incomplete, inconsistent, or noisy data can lead to inaccurate predictions and high rates of false positives. Integrating AI solutions with existing IAM infrastructure can also be complex, requiring careful planning and coordination. Furthermore, ensuring data privacy and compliance with relevant regulations (such as GDPR) is paramount.
For example, anonymization techniques may be necessary to protect sensitive user information while still enabling effective anomaly detection. Finally, ongoing model maintenance and retraining are essential to ensure the system’s continued effectiveness as threats evolve.
AI-Powered User and Entity Behavior Analytics (UEBA) for Security Operations (SecOps): Ai For Identity Security 5 Ways Ai Augments Secops And Iam Teams Today
UEBA systems are transforming how SecOps teams detect and respond to sophisticated threats. By leveraging the power of artificial intelligence, these systems analyze user and entity behavior across an organization’s IT infrastructure, identifying anomalies that often go unnoticed by traditional security tools. This allows for proactive threat hunting and faster incident response, significantly improving overall security posture.UEBA uses AI algorithms to correlate vast amounts of data from various sources, including network logs, security information and event management (SIEM) systems, endpoint detection and response (EDR) tools, and cloud access security brokers (CASB).
AI is revolutionizing identity security, boosting SecOps and IAM teams with automation and threat detection. Think about how much easier managing access would be with streamlined workflows, and that’s where the power of low-code/no-code development comes in. Check out this article on domino app dev the low code and pro code future to see how it can help.
Ultimately, integrating these faster development cycles with AI-powered security enhances our ability to build and protect robust identity systems.
This correlation allows for the creation of baselines of “normal” behavior for users and entities. Any deviation from these baselines triggers alerts, allowing security analysts to investigate potential threats in a timely manner. This proactive approach is crucial in today’s threat landscape, where attackers often employ advanced techniques to evade traditional detection methods.
UEBA Process Flow
The UEBA process can be visualized as a flowchart. Imagine a diagram starting with a large box labeled “Data Ingestion,” representing the collection of data from various sources mentioned above. Arrows then flow to a box labeled “Data Normalization and Enrichment,” where data is cleaned, standardized, and contextualized. Next, an arrow leads to “Behavioral Baselining,” where AI algorithms analyze the normalized data to establish baselines of normal activity for each user and entity.
From there, an arrow points to “Anomaly Detection,” where the AI continuously monitors activity against these baselines, flagging any significant deviations. Finally, an arrow leads to “Threat Response,” where alerts are generated, investigated, and appropriate actions are taken, such as blocking malicious activity or initiating an investigation. The entire process is iterative, with feedback loops allowing the system to continuously learn and improve its accuracy.
Comparison of AI-Based UEBA and Traditional SIEM
Traditional SIEM systems primarily focus on log aggregation and correlation, often relying on predefined rules and signatures to detect threats. AI-based UEBA systems, however, offer significant advantages:
- Proactive Threat Detection: UEBA proactively identifies anomalies based on behavioral deviations, while SIEM primarily reacts to known threats.
- Advanced Analytics: UEBA leverages machine learning to detect complex and evolving threats that might be missed by rule-based SIEM systems.
- Contextual Awareness: UEBA provides richer context around security events, offering better understanding of the threat landscape.
- Reduced Alert Fatigue: By focusing on significant anomalies, UEBA reduces the number of false positives, improving the efficiency of security analysts.
- Scalability and Automation: UEBA systems are designed to handle large volumes of data from diverse sources, automating much of the threat detection process.
Best Practices for Deploying and Managing AI-Powered UEBA, Ai for identity security 5 ways ai augments secops and iam teams today
Successful deployment and management of an AI-powered UEBA system requires careful planning and consideration of several factors. Data privacy and compliance are paramount.
- Data Privacy and Compliance: Implement robust data governance policies and procedures to ensure compliance with relevant regulations (e.g., GDPR, CCPA). Anonymize or pseudonymize sensitive data where possible.
- Data Quality: Ensure high-quality data is ingested into the system. Poor data quality can lead to inaccurate baselines and false positives.
- Integration with Existing Security Tools: Integrate the UEBA system with existing SIEM, EDR, and other security tools to maximize its effectiveness.
- Continuous Monitoring and Tuning: Continuously monitor the system’s performance and adjust its parameters as needed to optimize its accuracy and efficiency.
- Security Analyst Training: Provide adequate training to security analysts on how to effectively use and interpret the insights provided by the UEBA system.
AI for Automated Identity Provisioning and De-provisioning
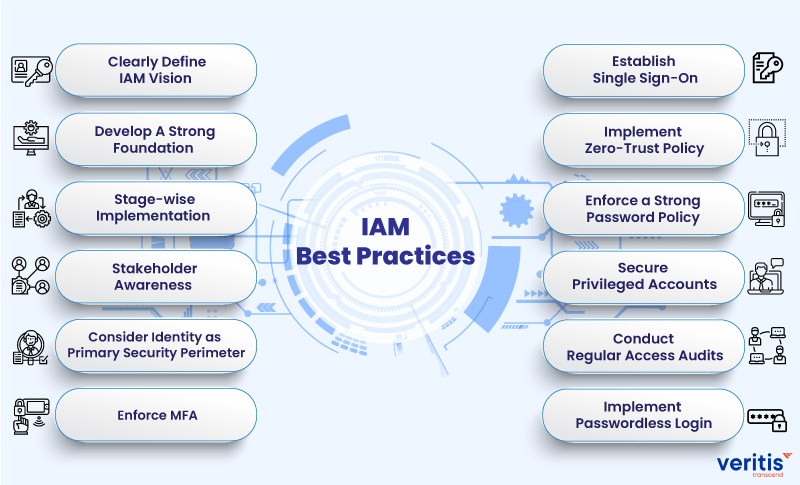
Automating the creation and removal of user accounts is crucial for maintaining a secure and efficient IT infrastructure. Manual processes are slow, error-prone, and struggle to keep pace with the demands of modern, dynamic organizations. AI offers a powerful solution, streamlining identity management and significantly enhancing security. This automation extends beyond simple account creation; AI can intelligently manage access rights, ensuring users only have the permissions necessary for their roles, minimizing the attack surface.AI significantly improves identity provisioning and de-provisioning by automating tasks previously handled manually.
This automation reduces the time and resources required for account management, minimizes human error, and strengthens overall security. The integration of AI-powered systems allows for real-time adjustments to access rights based on changes in roles or responsibilities, ensuring continuous compliance with security policies. This proactive approach minimizes security risks associated with outdated or improperly assigned permissions.
Comparison of Manual vs. AI-Driven Provisioning Processes
The table below highlights the key differences between manual and AI-driven identity provisioning processes. Manual processes are inherently slower and more susceptible to errors, while AI-driven systems offer greater efficiency and improved security.
Feature | Manual Provisioning | AI-Driven Provisioning |
---|---|---|
Speed | Slow, often taking days or weeks | Fast, often near real-time |
Accuracy | High risk of human error leading to misconfigurations | High accuracy due to automated checks and validation |
Efficiency | Labor-intensive, requiring significant manual effort | Highly efficient, automating repetitive tasks |
Scalability | Difficult to scale to handle large numbers of users | Easily scalable to accommodate growing user bases |
Security | Increased risk of misconfigurations and vulnerabilities | Enhanced security through automated access control and auditing |
Potential Risks and Mitigation Strategies for Automated Identity Provisioning
Implementing automated identity provisioning requires careful consideration of potential risks. However, with appropriate mitigation strategies, these risks can be effectively managed.The automation of identity provisioning, while offering significant advantages, introduces potential risks if not implemented and managed correctly. These risks can range from unintended access grants to complete system breaches. Therefore, a robust risk mitigation strategy is crucial.
- Risk: Incorrect access rights assigned. Mitigation: Implement rigorous access control policies and automated checks to validate access requests against predefined roles and permissions. Regular audits of access rights should be conducted to identify and correct any anomalies.
- Risk: Unauthorized access due to system vulnerabilities. Mitigation: Employ robust security measures, including multi-factor authentication, regular security assessments, and penetration testing, to identify and address vulnerabilities in the automated provisioning system.
- Risk: Data breaches due to compromised credentials. Mitigation: Implement strong password policies, utilize password managers, and regularly rotate credentials. Employ robust monitoring and alerting systems to detect suspicious activity and potential breaches.
- Risk: Lack of audit trails hindering accountability. Mitigation: Maintain detailed audit logs of all provisioning and de-provisioning activities, including timestamps, user actions, and system changes. These logs should be regularly reviewed and analyzed to ensure compliance and identify potential security incidents.
AI’s Role in Improving Accuracy and Speed of Identity Provisioning
AI algorithms can analyze vast amounts of data to identify patterns and anomalies, improving the accuracy and speed of identity provisioning. For instance, AI can automatically verify user identities, validate access requests against organizational policies, and detect potential security risks in real-time. This proactive approach reduces the likelihood of human error and strengthens the overall security posture. A large financial institution, for example, might use AI to automatically provision access for new employees based on their job description and department, ensuring compliance with regulatory requirements and internal policies while drastically reducing the time spent on manual processes.
This speed and accuracy are critical for maintaining a secure and efficient operational environment.
AI-Enhanced Identity Verification and Authentication
AI is revolutionizing identity verification and authentication, moving beyond traditional methods to offer more secure and user-friendly experiences. This enhanced security relies on the power of AI algorithms to analyze vast amounts of data and detect subtle anomalies indicative of fraudulent activity, ultimately strengthening our digital defenses.AI algorithms significantly enhance multi-factor authentication (MFA) by incorporating behavioral biometrics. Instead of relying solely on static factors like passwords or one-time codes, behavioral biometrics analyze user behavior patterns during login attempts.
This includes typing rhythm, mouse movements, and even the pressure applied to the screen. Deviations from established baselines trigger alerts, flagging potentially fraudulent logins even if the user possesses the correct credentials. This offers a significant advantage over traditional MFA, as it adds a layer of continuous authentication that adapts to individual user behavior.
Behavioral Biometrics in MFA
Behavioral biometrics work by creating a profile of a user’s typical interaction patterns. This profile is built over time, learning the nuances of how a legitimate user interacts with a system. When a user attempts to log in, the system compares their current behavior against this established profile. Significant discrepancies, such as unusual typing speed or mouse movements, raise a red flag, suggesting a potential compromise.
For example, if a user typically logs in from their home network at a consistent time, a login attempt from a different location at an unusual hour would trigger an alert. The advantage of behavioral biometrics lies in its passive nature; it continuously monitors user behavior without requiring explicit actions from the user, making it a seamless and unobtrusive security measure.
AI-Powered Authentication Methods
Various AI-powered authentication methods offer varying levels of security and user experience. The choice depends on the specific security requirements and the context of the application.
Authentication Method | Accuracy | Security Level | User Experience |
---|---|---|---|
Facial Recognition | High (95%+ in controlled environments, accuracy varies with lighting, angle, etc.) | High (difficult to spoof with high-quality systems) | Generally good, but can be intrusive and dependent on camera quality and lighting. |
Voice Recognition | Moderate to High (accuracy depends on background noise, voice clarity, and potential for impersonation) | Moderate to High (vulnerable to voice cloning but improving with advanced techniques) | Good, but susceptible to environmental noise and voice changes due to illness. |
Risk-Based Authentication | Variable (depends on the factors considered and the accuracy of risk scoring) | Moderate to High (adapts to changing risk profiles) | Generally good, but can lead to increased friction if risk thresholds are set too aggressively. |
Ethical Considerations and Potential Biases
AI-powered identity verification and authentication systems raise significant ethical concerns, primarily revolving around potential biases and privacy implications. Facial recognition systems, for instance, have been shown to exhibit bias against certain demographics, leading to inaccurate or discriminatory outcomes. Similarly, voice recognition systems may struggle with accents or speech impediments, potentially excluding certain user groups. Furthermore, the collection and storage of biometric data raise serious privacy concerns, necessitating robust data protection measures and transparent data handling practices.
The potential for misuse of biometric data, for example, in mass surveillance or discriminatory profiling, needs careful consideration and regulation. Striking a balance between security and ethical considerations is paramount in deploying AI-powered authentication systems responsibly.
AI for Threat Intelligence and Predictive Security in Identity Management
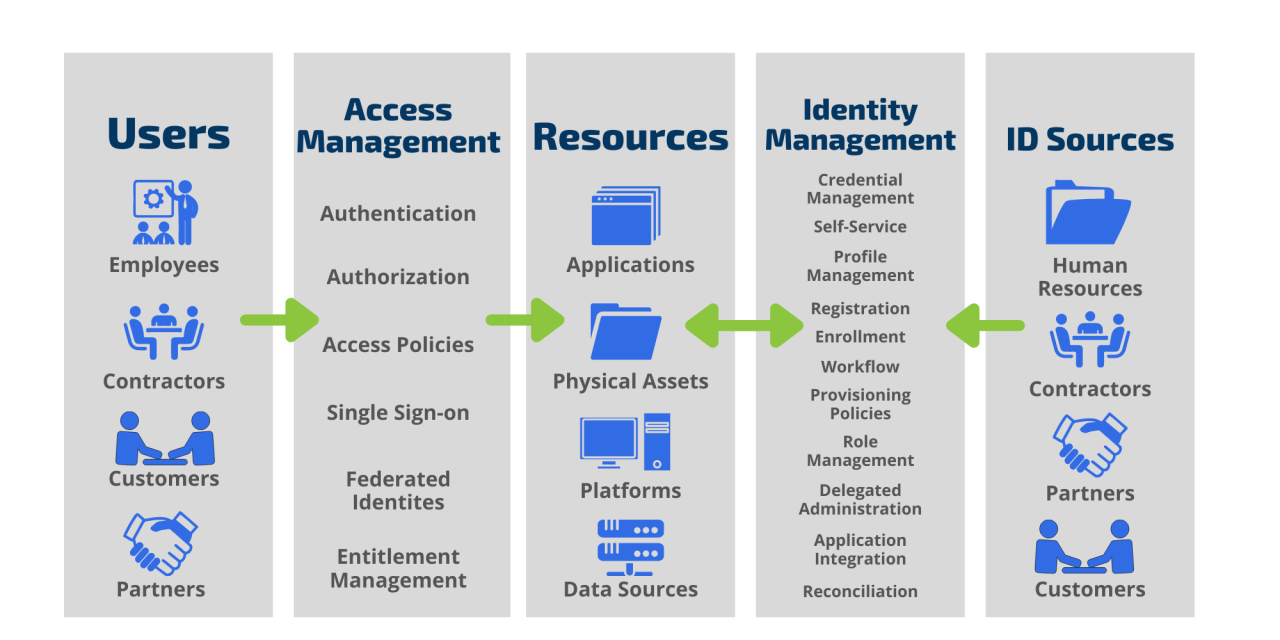
AI is revolutionizing identity security, moving beyond reactive measures to proactive threat mitigation. By integrating advanced threat intelligence feeds and leveraging machine learning, organizations can significantly enhance their IAM systems’ resilience against sophisticated attacks. This allows for a more predictive approach, identifying potential vulnerabilities before they’re exploited.AI’s role in threat intelligence for IAM focuses on analyzing vast datasets from diverse sources, identifying patterns indicative of malicious activity, and ultimately predicting potential breaches.
This proactive approach reduces response times and minimizes the impact of successful attacks.
Threat Intelligence Integration into IAM Security Processes
Imagine a visual representation: a central hub (IAM system) receiving input from various streams. These streams represent different threat intelligence feeds (e.g., vulnerability databases, security information and event management (SIEM) systems, open-source intelligence, and internal security logs). AI algorithms analyze this data, identifying correlations and anomalies. These insights are then fed back into the IAM system, triggering automated responses like account lockouts, access restrictions, or security alerts.
The system learns from each event, continuously refining its threat detection capabilities. This continuous feedback loop enhances the system’s ability to proactively identify and respond to emerging threats.
AI-Driven Predictions of Potential Security Breaches
AI can analyze historical data, including past successful and unsuccessful attacks, combined with real-time threat intelligence to predict future breaches. This predictive capability is crucial for proactive security measures.
- Scenario: A surge in credential stuffing attacks targeting a specific industry. AI Prediction: The AI system identifies this trend and proactively strengthens password policies, implements multi-factor authentication (MFA) for vulnerable accounts, and increases monitoring of login attempts from known malicious IP addresses.
- Scenario: An employee’s access patterns significantly deviate from their usual behavior. AI Prediction: The AI system flags this anomaly as a potential indicator of compromised credentials or insider threat, triggering an investigation and potential account suspension.
- Scenario: A newly discovered vulnerability in a widely used authentication protocol is reported. AI Prediction: The AI system identifies the vulnerability’s potential impact on the organization’s IAM infrastructure, prioritizing patching and mitigation efforts.
Strategies for Leveraging AI-Driven Threat Intelligence
Effectively leveraging AI-driven threat intelligence requires a strategic approach. Organizations should focus on data integration, algorithm selection, and continuous monitoring and improvement.Implementing AI-driven threat intelligence requires a robust data infrastructure capable of handling diverse data sources and volumes. Careful selection of AI algorithms is also critical, ensuring they are suited to the specific threat landscape and data characteristics. Finally, continuous monitoring and improvement of the AI system are essential to adapt to evolving threats and improve accuracy.
Regular updates to threat intelligence feeds and retraining of AI models ensure the system remains effective in identifying and mitigating emerging risks.
Closing Notes
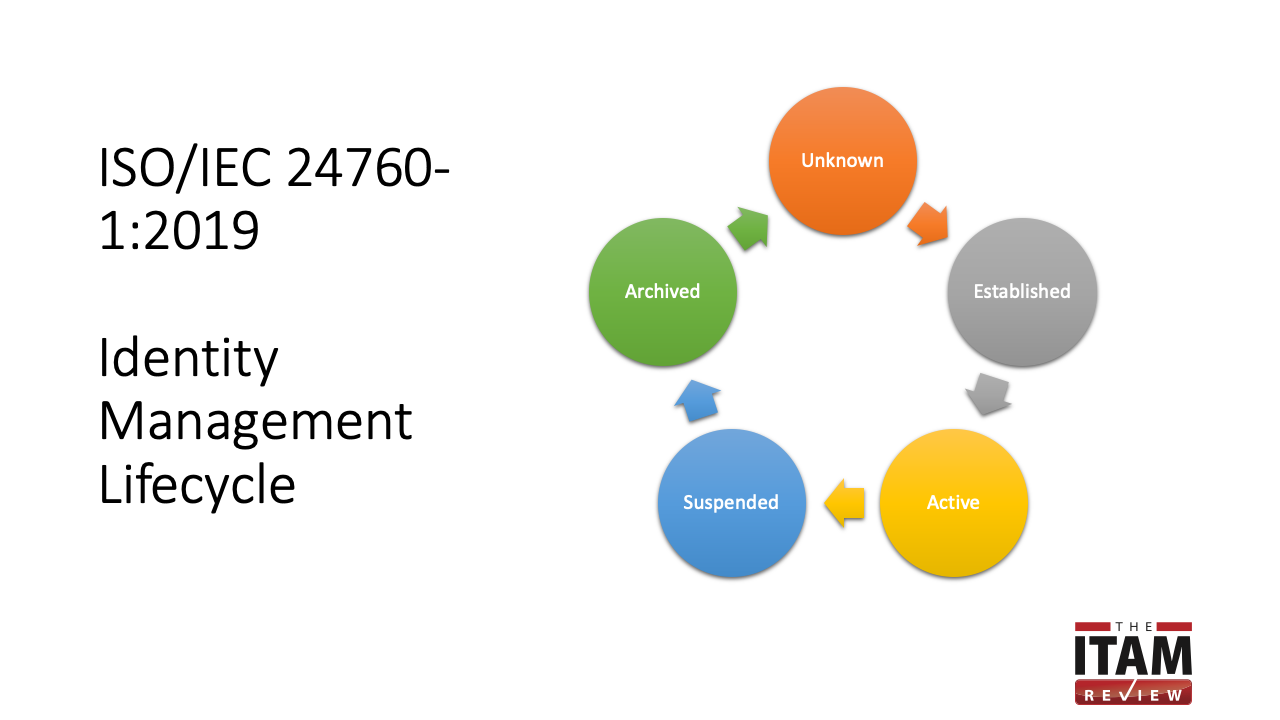
The integration of AI into identity security isn’t just a trend; it’s a necessity. As cyber threats become increasingly sophisticated, relying on human intervention alone is simply not enough. The five key areas explored—anomaly detection, UEBA, automated provisioning, enhanced authentication, and threat intelligence—demonstrate the transformative power of AI in bolstering our defenses. By embracing these technologies and understanding their implications, we can build a more resilient and secure digital future.
So, let’s continue to explore the exciting possibilities of AI in safeguarding our identities and data!
General Inquiries
What are the biggest risks associated with implementing AI in identity security?
The biggest risks include data privacy concerns, potential biases in algorithms leading to unfair decisions, and the dependency on robust data quality for accurate AI functioning. Over-reliance on AI without human oversight is also a key risk.
How can I determine if my organization is ready for AI-powered identity security?
Consider your current security posture, data volume and quality, existing infrastructure, and budget. Start with a pilot project focusing on a specific area like anomaly detection to assess feasibility and ROI before large-scale implementation.
What are the costs associated with AI-driven identity security solutions?
Costs vary widely depending on the specific solution, vendor, and implementation complexity. Consider factors like software licenses, integration services, training, and ongoing maintenance.