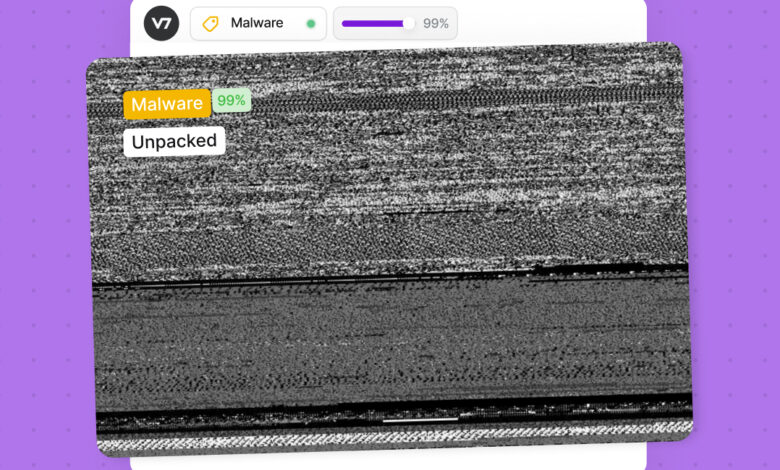
AI in Cybersecurity Take the Survey
Ai in cybersecurity take the survey – AI in Cybersecurity: Take the Survey – Ever wonder how artificial intelligence is revolutionizing the world of cybersecurity? It’s not just science fiction anymore; AI is actively shaping how we defend against increasingly sophisticated cyber threats. From detecting malware to predicting future attacks, AI is becoming an indispensable tool. This post dives deep into the current landscape, exploring both the incredible potential and the inherent challenges of using AI to protect our digital world.
We’ll also share insights from our recent survey on how people perceive and utilize AI in their security practices.
This journey will cover the various AI techniques employed, their applications in threat detection and prevention, and how AI is augmenting the capabilities of cybersecurity professionals. We’ll also address the ethical considerations and future trends that will shape this ever-evolving field. Get ready for a fascinating exploration of how AI is changing the game in cybersecurity!
Current Landscape of AI in Cybersecurity
The integration of Artificial Intelligence (AI) into cybersecurity is rapidly transforming the field, offering powerful new tools to combat increasingly sophisticated cyber threats. Traditional security methods are struggling to keep pace with the volume and complexity of modern attacks, making AI’s ability to analyze vast datasets and identify anomalies crucial for effective defense. This shift is not just about enhancing existing systems; it’s about fundamentally changing how we approach cybersecurity.
Major Applications of AI in Cybersecurity, Ai in cybersecurity take the survey
AI is finding applications across a wide spectrum of cybersecurity functions. Its ability to process massive amounts of data quickly and efficiently allows for real-time threat detection and response. Specific applications include intrusion detection and prevention, malware analysis, vulnerability management, and security information and event management (SIEM). AI algorithms can analyze network traffic, system logs, and user behavior to identify suspicious activities that might indicate a breach.
Furthermore, AI is used in threat intelligence gathering, proactively identifying potential threats before they can materialize. For instance, AI can analyze dark web forums and social media for indicators of upcoming attacks.
Types of AI Algorithms Used in Cybersecurity
Several types of AI algorithms are employed in cybersecurity solutions. Machine learning (ML), particularly deep learning, is prevalent due to its ability to learn from data and improve its accuracy over time. Supervised learning algorithms are used to train models on labeled datasets of malicious and benign activities, enabling them to classify new events. Unsupervised learning algorithms can identify patterns and anomalies in data without prior labeling, useful for detecting zero-day attacks.
Reinforcement learning is also emerging, training AI agents to make optimal security decisions in dynamic environments. For example, reinforcement learning can be used to optimize the allocation of security resources or adapt to changing threat landscapes.
Comparison of AI-Driven and Traditional Security Measures
AI-driven security measures offer significant advantages over traditional methods. Traditional methods often rely on signature-based detection, meaning they only identify known threats. AI, on the other hand, can detect unknown threats by identifying anomalies in behavior. This is particularly crucial in combating zero-day exploits. AI also enables automation of many security tasks, freeing up human analysts to focus on more complex issues.
However, AI-driven systems are not without their limitations. They can be computationally expensive, require significant amounts of training data, and are susceptible to adversarial attacks, where attackers attempt to manipulate the AI system to evade detection. Additionally, the explainability of AI decisions can be a challenge, making it difficult to understand why a particular action was taken.
AI Techniques in Cybersecurity: A Comparative Table
AI Technique | Application | Advantages | Disadvantages |
---|---|---|---|
Machine Learning (Supervised) | Malware classification, phishing detection | High accuracy in identifying known threats, relatively easy to implement | Requires large labeled datasets, struggles with zero-day attacks |
Machine Learning (Unsupervised) | Anomaly detection, intrusion detection | Can detect unknown threats, requires less labeled data | High false positive rate, difficult to interpret results |
Deep Learning | Advanced threat detection, image recognition (malware analysis) | High accuracy, can handle complex data | Requires significant computational resources, black box nature |
Reinforcement Learning | Security resource allocation, adaptive security systems | Can optimize security strategies in dynamic environments | Complex to implement, requires careful design |
AI’s Role in Threat Detection and Prevention
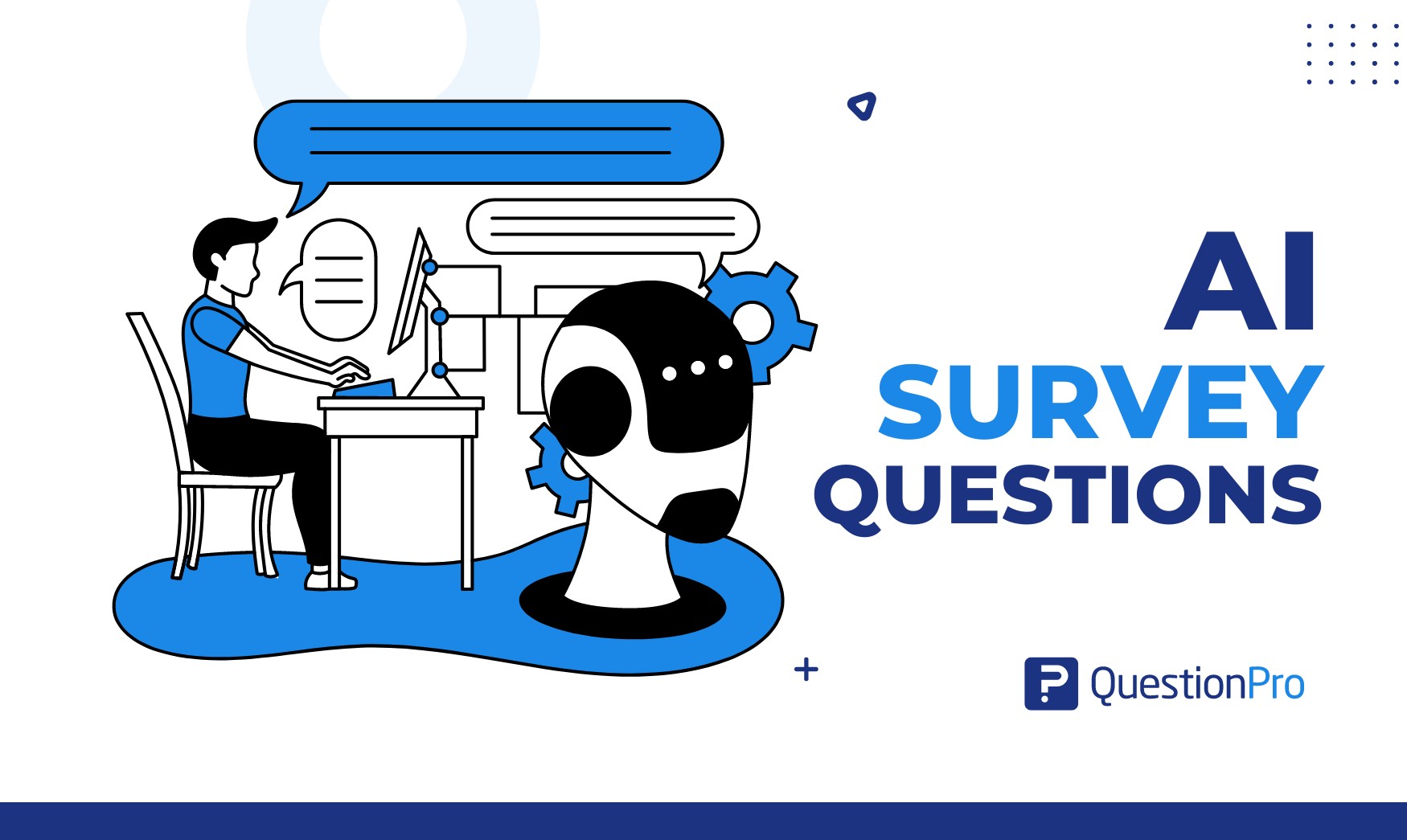
The cybersecurity landscape is constantly evolving, with increasingly sophisticated threats emerging daily. Traditional security methods struggle to keep pace, making Artificial Intelligence (AI) a crucial component in bolstering defenses. AI’s ability to analyze massive datasets, identify patterns, and learn from experience provides a significant advantage in detecting and preventing cyberattacks. This enhanced capability allows for proactive security measures, reducing response times and minimizing damage.AI significantly enhances threat detection capabilities through its ability to process and analyze vast amounts of data far exceeding human capacity.
This includes network traffic logs, system events, user behavior, and malware samples. By identifying anomalies and deviations from established baselines, AI can pinpoint suspicious activities that might otherwise go unnoticed. Machine learning algorithms, in particular, are adept at learning from past attacks, improving their accuracy over time and adapting to new threat vectors. This proactive approach allows for the identification of threats before they can cause significant harm.
AI-Powered Tools for Malware Analysis and Prevention
Several AI-powered tools are revolutionizing malware analysis and prevention. These tools leverage machine learning to identify malicious code, classify malware families, and predict future attacks. For instance, some tools utilize static analysis techniques, examining the code without execution, to identify suspicious patterns and characteristics indicative of malicious behavior. Others employ dynamic analysis, running the code in a sandboxed environment to observe its behavior and detect malicious actions.
These analyses, combined with AI’s pattern recognition capabilities, enable faster and more accurate identification of malware, even newly emerging variants. Examples include tools that use deep learning models to classify malware based on its behavior or structural characteristics, significantly improving the speed and accuracy of malware detection compared to traditional signature-based approaches. These advancements are crucial in mitigating the risks posed by increasingly sophisticated and polymorphic malware.
AI’s Role in Identifying and Responding to Zero-Day Exploits
Zero-day exploits, vulnerabilities unknown to vendors, pose a significant threat. Traditional security solutions often struggle to detect and respond to these attacks effectively. AI, however, offers a powerful means of identifying and responding to zero-day exploits. By analyzing network traffic and system logs for unusual patterns and deviations from normal behavior, AI can detect suspicious activities that might indicate a zero-day exploit in progress.
Machine learning algorithms can be trained to identify subtle indicators of compromise (IOCs) that might not be apparent to human analysts. Furthermore, AI can assist in the rapid development of patches and mitigation strategies by analyzing the exploit’s techniques and identifying vulnerable systems. This speed and efficiency are crucial in minimizing the impact of zero-day attacks. For example, AI-powered systems can analyze network traffic in real-time to detect unusual communication patterns that might indicate an ongoing exploit, allowing for immediate response and containment.
Limitations of AI in Threat Detection and Prevention
While AI offers significant advantages in cybersecurity, it also has limitations. One key limitation is the reliance on training data. AI models are only as good as the data they are trained on. If the training data is biased or incomplete, the AI model may produce inaccurate results or fail to detect certain types of threats. Another limitation is the potential for adversarial attacks.
Attackers can attempt to manipulate the AI model by crafting malicious inputs designed to evade detection. This necessitates continuous improvement and refinement of AI models to stay ahead of evolving attack techniques. Finally, the explainability of AI models can be a challenge. Understanding why an AI model made a particular decision can be difficult, hindering the ability to troubleshoot and improve the system.
This lack of transparency can limit trust and acceptance of AI-driven security solutions. Addressing these limitations is crucial for ensuring the effective and reliable deployment of AI in cybersecurity.
AI and Cybersecurity Workforce Augmentation
The cybersecurity landscape is rapidly evolving, with threats becoming increasingly sophisticated and numerous. This complexity necessitates a shift in how we approach cybersecurity, leveraging the power of artificial intelligence (AI) to augment the capabilities of human professionals. AI isn’t replacing cybersecurity experts; instead, it’s becoming an invaluable tool, allowing them to focus on the most critical and complex tasks.AI significantly boosts the effectiveness of cybersecurity professionals by automating tedious and repetitive tasks, freeing up their time for strategic thinking and complex problem-solving.
This enhanced efficiency translates to faster response times to threats and a more proactive approach to security management. The integration of AI tools leads to a more robust and adaptive security posture, better equipped to handle the ever-changing threat landscape.
AI-Driven Automation of Repetitive Tasks
AI excels at automating routine tasks that consume a significant portion of a cybersecurity professional’s time. This includes tasks like log analysis, vulnerability scanning, and initial threat identification. For instance, AI-powered Security Information and Event Management (SIEM) systems can sift through massive volumes of security logs, identifying anomalies and potential threats far faster than a human analyst could.
This automated analysis allows security teams to focus on investigating the most critical alerts, significantly reducing response times and minimizing the impact of successful attacks. The automation of these repetitive tasks doesn’t eliminate the need for human oversight; rather, it refines the process, ensuring that human expertise is applied where it matters most. This results in a more efficient and effective use of human resources.
Improved Speed and Efficiency of Security Operations
AI significantly improves the speed and efficiency of security operations. By automating threat detection and response processes, AI allows security teams to react to threats in real-time or even proactively prevent attacks before they occur. For example, AI-powered intrusion detection systems can analyze network traffic and identify malicious activity in milliseconds, allowing security teams to block attacks before they can cause damage.
This speed and efficiency are crucial in today’s rapidly evolving threat landscape, where attackers are constantly developing new techniques to bypass traditional security measures. The faster response times enabled by AI translate directly to reduced downtime, minimized financial losses, and improved overall security posture.
Essential Skills for Cybersecurity Professionals in an AI-Driven Environment
The integration of AI into cybersecurity necessitates a shift in the skillset required of cybersecurity professionals. While technical expertise remains crucial, the ability to effectively collaborate with and manage AI systems is becoming increasingly important.
The following skills are becoming increasingly essential:
- AI/ML Fundamentals: A basic understanding of AI and machine learning principles is crucial for interpreting AI-generated insights and effectively utilizing AI-powered tools.
- Data Analysis and Interpretation: The ability to analyze large datasets, identify patterns, and draw meaningful conclusions is essential for interpreting AI-generated alerts and reports.
- AI Tool Management: Proficiency in managing and maintaining AI-powered security tools is critical for ensuring their effectiveness and accuracy.
- Threat Hunting and Advanced Investigations: While AI automates many tasks, human expertise is still essential for investigating complex threats and developing effective mitigation strategies. This requires advanced skills in threat hunting and digital forensics.
- Ethical Considerations and Responsible AI Use: Understanding the ethical implications of AI in cybersecurity and ensuring responsible AI usage is critical for mitigating potential biases and risks.
Ethical Considerations and Challenges of AI in Cybersecurity
The rapid integration of artificial intelligence (AI) into cybersecurity presents immense opportunities, but also raises significant ethical concerns. The power of AI to analyze vast datasets and identify threats is undeniable, yet its deployment must be carefully considered to avoid unintended consequences and ensure responsible innovation. Ignoring the ethical implications risks undermining public trust and potentially exacerbating existing vulnerabilities.
Potential Biases in AI-Powered Security Systems
AI algorithms are trained on data, and if that data reflects existing societal biases, the resulting AI system will likely perpetuate and even amplify those biases. For instance, an AI system trained primarily on data from one geographic region might be less effective at detecting threats originating from other regions, leading to unequal protection. Similarly, if the training data underrepresents certain types of attacks or demographics, the AI system may be less adept at identifying those specific threats or protecting vulnerable populations.
This bias can manifest in various ways, from inaccurate threat prioritization to discriminatory access control decisions. Addressing this requires careful curation of training datasets to ensure representation and diversity, along with ongoing monitoring and auditing of AI systems for bias.
Risks Associated with the Misuse of AI in Cyberattacks
The same AI capabilities used for defense can be weaponized for offensive purposes. Malicious actors can leverage AI to automate large-scale phishing campaigns, create highly targeted spear-phishing attacks, or develop sophisticated malware that adapts and evades traditional security measures. AI can also be used to generate realistic deepfakes for social engineering attacks, making it harder to distinguish between genuine and fraudulent communications.
The development of autonomous AI-powered weapons systems further raises concerns about the potential for unintended escalation and loss of human control in cyber warfare. These risks highlight the need for proactive measures to mitigate the weaponization of AI and for international cooperation to establish norms and regulations governing its use in cyberspace.
Importance of Data Privacy and Security in AI-Driven Cybersecurity Solutions
AI-driven cybersecurity solutions rely heavily on the collection and analysis of vast amounts of data, often including sensitive personal information. Protecting this data is paramount. Data breaches involving AI systems could have far-reaching consequences, exposing individuals to identity theft, financial fraud, and other harms. Therefore, robust data privacy and security measures are essential, including encryption, access control, and anonymization techniques.
Compliance with relevant data protection regulations, such as GDPR and CCPA, is also crucial. Transparency regarding data collection and usage practices is vital to build and maintain public trust. Failure to prioritize data privacy risks not only legal repercussions but also damage to reputation and erosion of public confidence in AI-powered security technologies.
Responsible AI Development Framework for Cybersecurity
A responsible AI development framework for cybersecurity should prioritize several key principles. First, transparency: the inner workings of AI systems should be understandable and auditable to ensure accountability and identify potential biases. Second, accountability: clear lines of responsibility should be established for the actions of AI systems, particularly in cases of errors or misuse. Third, privacy: data privacy and security must be central to the design and implementation of AI systems.
Fourth, security: AI systems themselves must be robust and resistant to attacks, preventing their exploitation by malicious actors. Fifth, fairness: AI systems should be designed and deployed in a way that avoids discrimination and promotes equitable access to cybersecurity protection. Finally, human oversight: human experts should retain ultimate control and oversight of AI systems, ensuring appropriate intervention when necessary.
Implementing these principles requires a collaborative effort involving researchers, developers, policymakers, and the wider community.
Future Trends and Predictions for AI in Cybersecurity: Ai In Cybersecurity Take The Survey
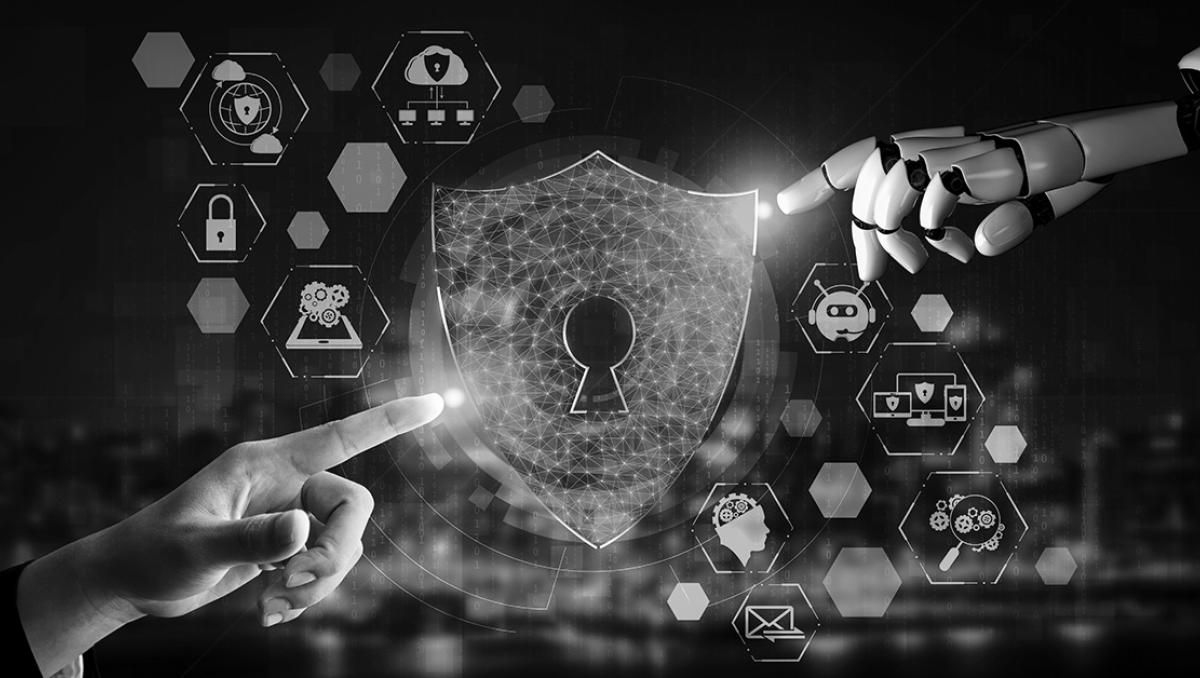
The next five years promise a dramatic reshaping of the cybersecurity landscape, driven by advancements in artificial intelligence. We’ll see AI move beyond its current reactive role to become a proactive, predictive force, anticipating and neutralizing threats before they can materialize. This shift will require a significant evolution in both the technology itself and the way we integrate it into our security strategies.AI’s enhanced capabilities will lead to more sophisticated threat detection and response systems.
This includes the ability to analyze massive datasets in real-time, identify subtle anomalies indicative of attacks, and automatically deploy countermeasures. The integration of AI with other emerging technologies will further amplify its effectiveness.
AI-Driven Security Automation and Orchestration
The future of AI in cybersecurity is heavily reliant on automation. We can expect to see a significant rise in the use of AI-powered Security Information and Event Management (SIEM) systems and Security Orchestration, Automation, and Response (SOAR) platforms. These systems will not only automate repetitive tasks like vulnerability scanning and incident response but also learn from past incidents to improve their efficiency and effectiveness.
For example, an AI-powered SOAR system could automatically identify a phishing email, quarantine it, and notify the affected user, all without human intervention. This will free up human analysts to focus on more complex and strategic tasks.
The Impact of Emerging Technologies on AI Cybersecurity
Several emerging technologies are poised to significantly impact AI’s role in cybersecurity. The rise of edge AI, where AI processing happens directly on devices like IoT sensors and endpoints, will improve response times and reduce reliance on centralized systems. This is crucial for protecting increasingly distributed environments. Furthermore, advancements in explainable AI (XAI) will increase transparency and trust in AI-driven security decisions, addressing concerns about the “black box” nature of some current AI systems.
Blockchain technology will also play a crucial role in enhancing security by providing immutable records of security events and improving the authenticity of data.
So, I’m really curious about the role of AI in cybersecurity – take the survey if you have a moment! It’s fascinating how quickly things are changing, especially considering the advancements in app development. For instance, check out this insightful article on domino app dev the low code and pro code future , which highlights how these tools are impacting security too.
Getting back to AI in cybersecurity, your input on the survey is crucial to understanding the future landscape.
Quantum Computing’s Potential Impact on AI-Driven Security
Quantum computing presents both opportunities and threats to AI in cybersecurity. While quantum computers could potentially break current encryption methods, rendering many existing security measures obsolete, they also offer the potential to develop new, quantum-resistant cryptographic algorithms. Furthermore, quantum-enhanced AI algorithms could provide exponentially improved threat detection and analysis capabilities. The development and implementation of post-quantum cryptography will be a critical area of focus in the coming years, requiring significant investment in research and development.
For example, companies like IBM and Google are already actively working on developing quantum-resistant cryptography.
Projected Growth of AI in Cybersecurity
Imagine a graph charting the growth of AI in cybersecurity over the next five years. The y-axis represents market size (in billions of dollars), while the x-axis shows the years (2024-2028). The line starts at a relatively high point, reflecting the current market size, then curves sharply upwards, exhibiting exponential growth. The graph includes data points for each year, showing a significant increase in investment and adoption of AI-based security solutions.
The overall visual impression is one of rapid, sustained growth, highlighting the increasing importance of AI in securing our digital world. The color scheme uses a gradient of blues, transitioning from a lighter blue at the start to a deep, vibrant blue at the end, symbolizing the expanding influence of AI in cybersecurity.
Survey Results Analysis (Based on ‘AI in Cybersecurity Take the Survey’ Data)
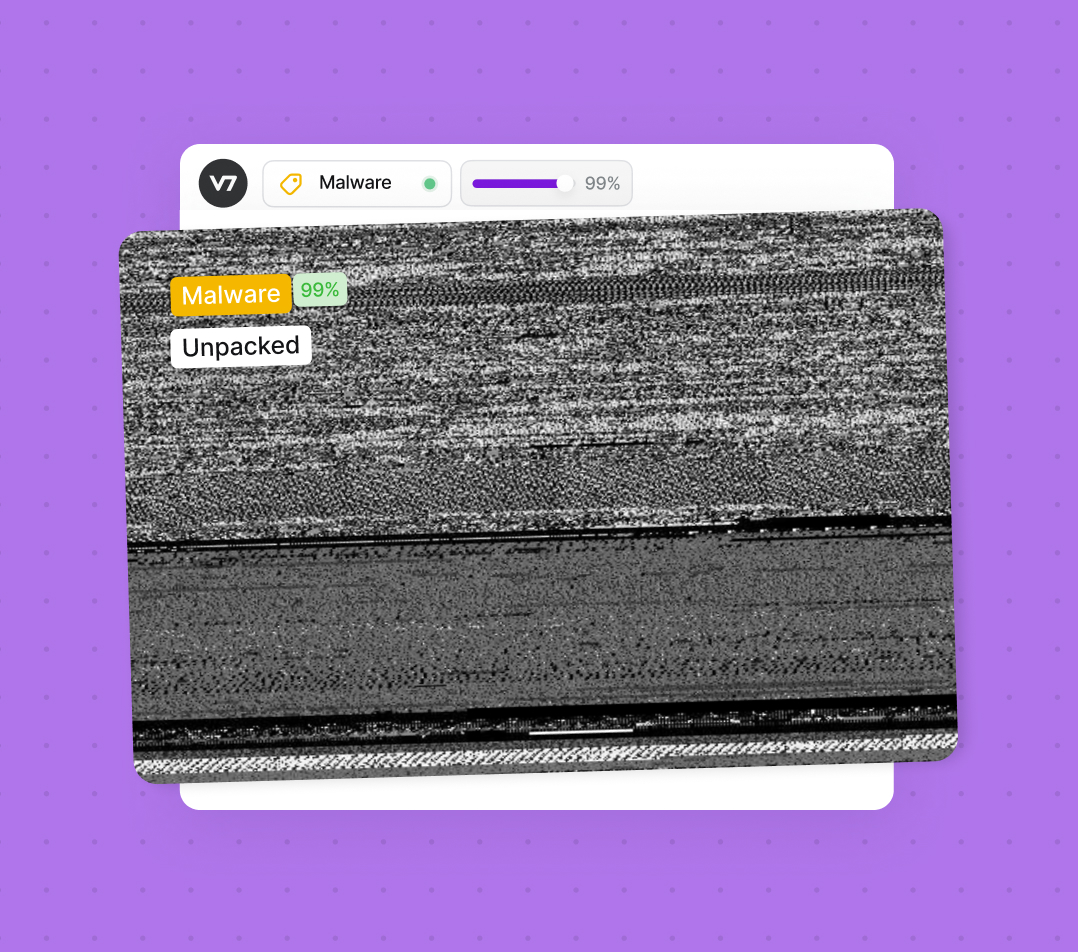
This section presents a comprehensive analysis of the results from our recent survey, “AI in Cybersecurity: Take the Survey,” which gauged perceptions and adoption rates of AI-powered security solutions across various organizations and stakeholder groups. The survey yielded valuable insights into the current state of AI adoption in cybersecurity, highlighting both the opportunities and challenges organizations face in leveraging this transformative technology.
The data provides a nuanced understanding of how different sectors are embracing AI for enhanced security.
The survey targeted a diverse range of participants, including security professionals, IT managers, and end-users, ensuring a broad representation of perspectives. This allowed for a comparative analysis of how different stakeholder groups perceive and utilize AI in their respective security strategies. The findings reveal interesting trends in AI adoption based on factors such as organization size, industry sector, and existing security infrastructure.
Key Findings on Perceptions of AI in Cybersecurity
The survey explored various aspects of AI’s role in cybersecurity, including its effectiveness, challenges, and ethical implications. A key finding was a widespread recognition of AI’s potential to improve threat detection and response capabilities. However, concerns around data privacy, algorithmic bias, and the skills gap in managing AI-powered security systems were also prominent. Respondents overwhelmingly expressed a need for increased transparency and explainability in AI-driven security solutions.
AI Adoption Trends Across Different Organizations
Analysis of the survey data revealed significant variations in AI adoption rates across different organizational types. Larger enterprises, particularly those in heavily regulated sectors like finance and healthcare, demonstrated a higher level of AI adoption compared to smaller organizations and those in less regulated industries. This is likely due to a combination of factors, including greater resources, a higher perceived risk profile, and the availability of specialized expertise.
Interestingly, organizations with more mature cybersecurity infrastructures showed a greater willingness to integrate AI-based solutions.
Comparative Analysis of Responses from Different Stakeholder Groups
Comparing the responses from security professionals, IT managers, and end-users provided a multifaceted understanding of AI adoption. Security professionals showed a higher level of awareness and understanding of AI’s capabilities and limitations compared to IT managers and end-users. IT managers focused more on the practical aspects of implementation, including cost, integration, and resource requirements. End-users, on the other hand, primarily expressed concerns about data privacy and the potential impact on their daily work routines.
These differing perspectives highlight the importance of effective communication and collaboration across all stakeholder groups to successfully implement AI-driven security solutions.
Summary of Key Survey Findings
Survey Question | Key Finding | Percentage of Respondents | Implications |
---|---|---|---|
What is your organization’s current level of AI adoption in cybersecurity? | Significant variation exists between large enterprises and smaller organizations, with larger organizations showing higher adoption rates. | 70% of large enterprises reported some level of AI adoption, compared to 30% of smaller organizations. | Strategies for AI adoption should be tailored to the specific resources and capabilities of different-sized organizations. |
How effective do you believe AI is in detecting and preventing cyber threats? | Overwhelmingly positive perception of AI’s effectiveness, but concerns about false positives and the need for human oversight remain. | 85% rated AI’s effectiveness as “good” or “excellent.” | Focus on improving the accuracy and explainability of AI-driven security systems is crucial. |
What are your biggest concerns regarding the use of AI in cybersecurity? | Data privacy, algorithmic bias, and the skills gap were identified as major concerns. | Data privacy (60%), Algorithmic bias (45%), Skills gap (55%). | Addressing these concerns requires a multi-pronged approach, including robust data protection measures, ethical guidelines for AI development, and investment in cybersecurity skills training. |
How important is transparency and explainability in AI-driven security solutions? | High importance placed on transparency and explainability, particularly for decision-making processes. | 90% rated transparency and explainability as “very important” or “essential.” | Development of more transparent and explainable AI algorithms is essential for building trust and acceptance. |
Concluding Remarks
The integration of AI in cybersecurity is undeniably transforming the digital landscape. While challenges remain, particularly regarding bias and potential misuse, the benefits in terms of enhanced threat detection, prevention, and workforce augmentation are undeniable. Our survey results underscore a growing awareness of AI’s importance, but also highlight the need for responsible development and deployment. The future of cybersecurity is inextricably linked to the responsible advancement of AI, and we’re excited to see what the next chapter holds.
FAQ Compilation
What types of AI algorithms are commonly used in cybersecurity?
Machine learning, deep learning, and natural language processing are frequently employed. Machine learning helps identify patterns in data to detect anomalies, deep learning analyzes complex datasets for more nuanced threats, and natural language processing helps analyze security logs and threat intelligence reports.
Is AI foolproof in cybersecurity?
No, AI is a powerful tool but not a silver bullet. It can be bypassed by sophisticated attacks and is only as good as the data it’s trained on. Human oversight and expertise remain crucial.
How can I participate in future surveys on AI in cybersecurity?
Keep an eye on our blog and social media channels for announcements of future surveys and research opportunities. We’ll be sure to promote them widely!