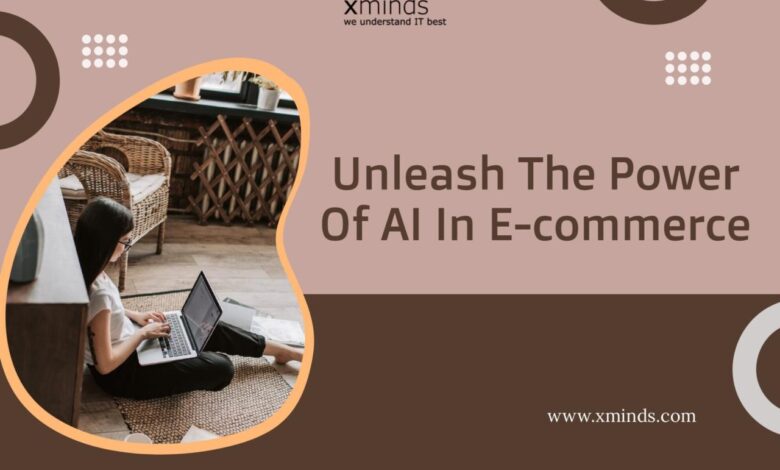
AI in E-commerce and Customer Experience
Ai in e commerce and customer experience – AI in e-commerce and customer experience is revolutionizing how businesses interact with their customers. No longer a futuristic concept, AI is actively shaping personalized shopping experiences, streamlining customer service, and enhancing the overall online journey. From AI-powered product recommendations that anticipate our needs to chatbots providing instant support, the impact is undeniable. This exploration dives into the exciting world of AI’s role in e-commerce, examining its benefits, challenges, and future implications.
We’ll delve into the specifics of AI-driven personalization, exploring how algorithms analyze vast amounts of data to create tailored product suggestions and marketing campaigns. We’ll also uncover how AI is transforming customer service through intelligent chatbots and sentiment analysis, enabling businesses to understand and respond to customer feedback more effectively. Furthermore, we’ll investigate the use of AI in search and discovery, fraud detection, and the emerging technologies poised to redefine the future of e-commerce interactions.
AI-Powered Personalization in E-commerce
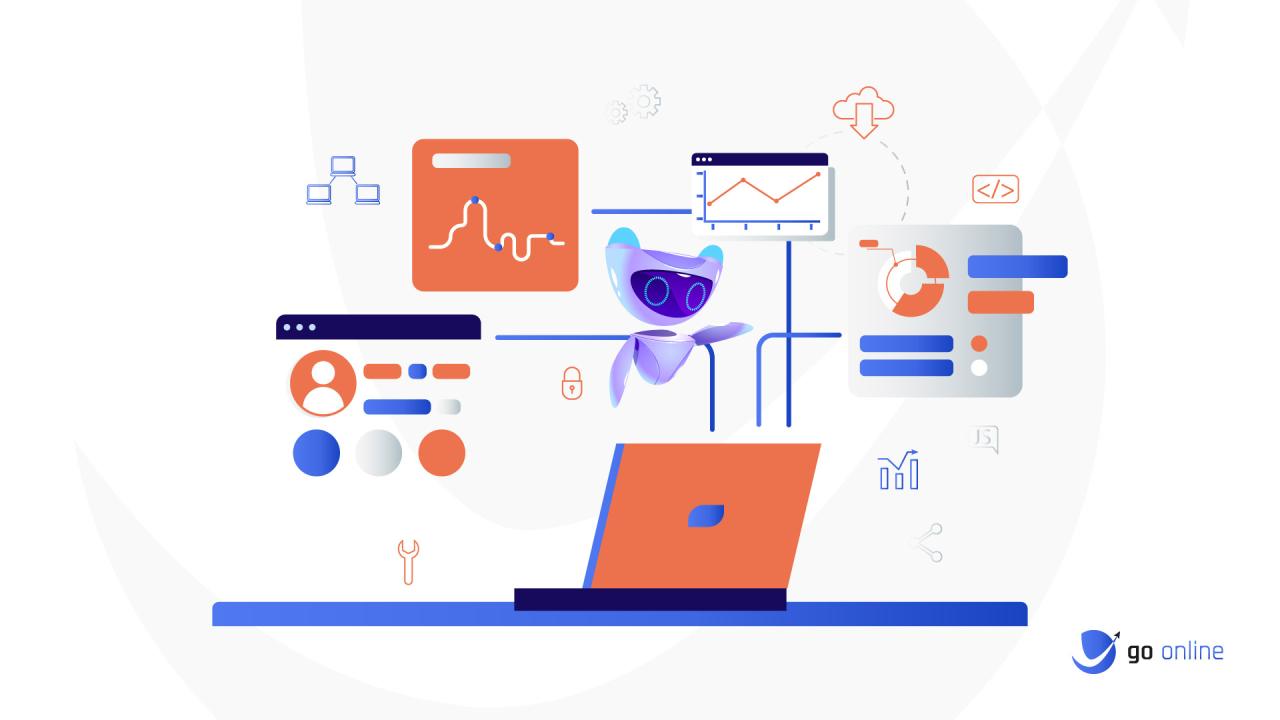
AI is revolutionizing e-commerce by enabling highly personalized experiences for customers. This personalization goes beyond simple targeted advertising; it leverages sophisticated algorithms to understand individual preferences and behaviors, ultimately leading to increased customer engagement and sales. This detailed look at AI-powered personalization will explore how it works, its implementation, and the ethical considerations involved.
AI Algorithms for Personalized Product Recommendations
AI algorithms analyze vast amounts of customer data to generate tailored product recommendations. This data includes browsing history, purchase history, demographics, and even interactions with customer service. Several key recommendation systems are employed: Collaborative filtering analyzes similarities between customers to recommend products that similar users have purchased. For example, if a user frequently buys organic products, the system might suggest similar items purchased by other organic food enthusiasts.
Content-based filtering recommends products based on a user’s past interactions with specific products. If a user consistently buys hiking boots, the system will suggest other hiking gear or similar outdoor products. Hybrid approaches combine both collaborative and content-based filtering to create even more accurate and diverse recommendations, leveraging the strengths of both methods. For instance, a hybrid system might suggest a new hiking boot based on similar purchases by other users (collaborative) and also because the user has previously shown interest in hiking-related products (content-based).
Implementation of Personalized Product Displays and Email Marketing Campaigns
AI facilitates personalized product displays by dynamically adjusting the products shown on a website or app based on individual user profiles. For example, a user browsing hiking gear might see prominently displayed high-quality boots and related accessories, while a user looking for budget-friendly options will see a different selection. In email marketing, AI allows for the creation of highly targeted campaigns.
Personalized email subject lines and body content increase open and click-through rates. A user who abandoned their shopping cart might receive an email reminding them of the items and offering a discount, increasing the likelihood of conversion. Similarly, emails showcasing products tailored to their past purchases or browsing history can drive repeat purchases and foster customer loyalty.
Comparison of AI-Driven Personalization vs. Traditional Methods
Method | Customer Engagement | Conversion Rate | Cost |
---|---|---|---|
Traditional Marketing (e.g., mass email blasts) | Low | Low | Low (initially) |
AI-Driven Personalization | High | High | Moderate to High (due to data processing and algorithm development) |
Note
The cost of AI-driven personalization can be offset by increased conversion rates and customer lifetime value.*
Ethical Considerations of AI-Powered Personalization
The use of AI for personalization raises significant ethical concerns. Data privacy is paramount. Companies must be transparent about the data they collect and how it’s used, obtaining explicit consent where necessary and adhering to data protection regulations like GDPR and CCPA. Furthermore, AI algorithms can perpetuate and amplify existing biases present in the data they are trained on.
For example, if the training data reflects gender or racial biases, the resulting recommendations might unfairly disadvantage certain groups. Mitigating these biases requires careful data curation, algorithm design, and ongoing monitoring to ensure fairness and equity. Addressing these concerns is crucial for building trust with customers and maintaining the ethical integrity of AI-powered personalization.
AI-Driven Customer Service Enhancements
The integration of artificial intelligence (AI) is revolutionizing customer service in e-commerce, moving beyond simple automation to create truly personalized and efficient support experiences. AI empowers businesses to handle a larger volume of inquiries with greater speed and accuracy, ultimately leading to increased customer satisfaction and loyalty. This enhanced efficiency allows human agents to focus on more complex issues, fostering a more effective and balanced support system.AI-powered chatbots are at the forefront of this transformation, significantly impacting response times and overall efficiency.
These intelligent virtual assistants provide immediate support, addressing common customer queries and freeing up human agents to handle more intricate problems. This not only improves response times but also reduces the workload on human agents, allowing them to concentrate on more complex issues requiring their expertise.
AI Chatbot Functionalities
AI chatbots offer a wide range of functionalities designed to streamline customer interactions. These functionalities significantly improve the customer experience by providing quick and accurate answers to frequently asked questions, offering real-time order tracking, and guiding users through troubleshooting processes. For instance, a customer can easily track their order status through a chatbot interface without needing to navigate complex website menus or wait on hold for a human agent.
Similarly, chatbots can troubleshoot simple technical issues, such as password resets or account access problems, providing immediate solutions.
AI-Powered Sentiment Analysis of Customer Feedback
Analyzing customer reviews and feedback is crucial for understanding customer sentiment and identifying areas for improvement. AI-powered sentiment analysis tools can automatically process large volumes of text data, identifying positive, negative, and neutral sentiments expressed by customers. This automated analysis allows businesses to quickly pinpoint areas of concern, such as recurring negative feedback related to a specific product or service.
For example, if numerous negative reviews mention slow shipping times, the company can prioritize improvements to its logistics processes.
Workflow Diagram: AI and Human Agent Collaboration
Imagine a workflow diagram illustrating the interaction between AI and human agents. The process begins with a customer inquiry received through various channels (e.g., website chat, email). The AI chatbot initially handles the inquiry. If the chatbot can resolve the issue (e.g., answering a simple FAQ, providing order tracking information), the interaction concludes. However, if the chatbot cannot resolve the issue, it escalates the inquiry to a human agent, providing them with the complete context of the conversation.
The human agent then takes over, leveraging the information gathered by the AI to provide a more personalized and efficient solution. This collaborative approach optimizes support processes, ensuring that customers receive timely and effective assistance, regardless of the complexity of their inquiry. This seamless handover ensures a consistent and positive customer experience.
AI in E-commerce Search and Discovery
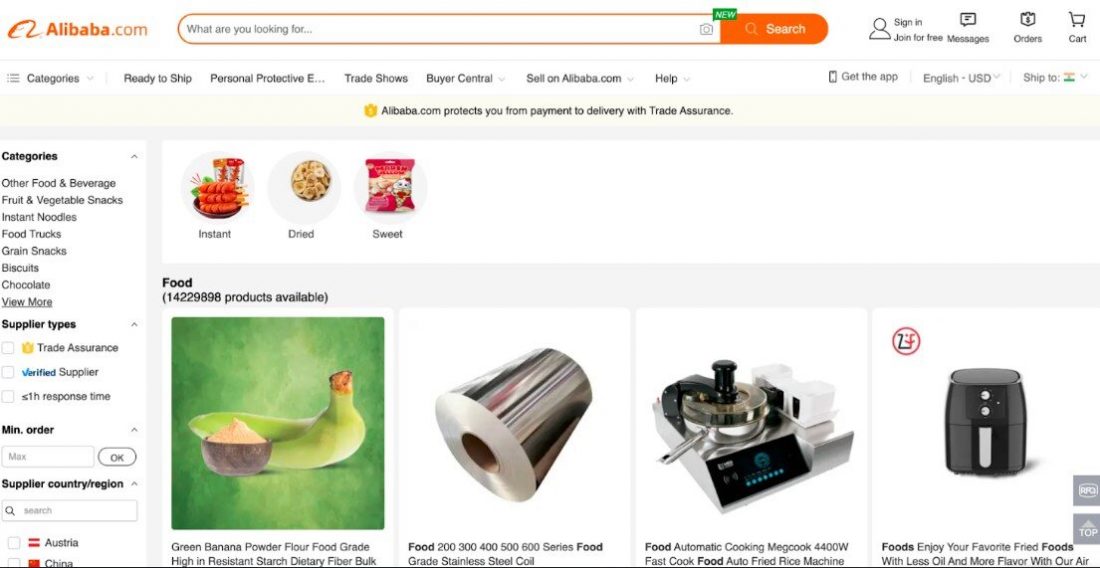
The way we find products online is undergoing a radical transformation, thanks to the power of artificial intelligence. Gone are the days of relying solely on -based searches; AI is now driving a far more intuitive and personalized shopping experience. This shift significantly impacts how consumers discover products and, ultimately, how businesses connect with their customers. Let’s delve into the exciting advancements in AI-powered e-commerce search and discovery.Traditional search algorithms, largely based on matching and ranking by relevance scores, often fall short in understanding the nuanced needs of shoppers.
They struggle with complex queries, synonyms, and the implicit meaning behind search terms. AI-powered search, on the other hand, leverages machine learning and natural language processing to provide a far more sophisticated and effective search experience. It goes beyond simple matching to understand the user’s intent and context, leading to more relevant and personalized results.
AI-Powered Search Functionalities Compared to Traditional Methods
AI-powered search significantly outperforms traditional methods by incorporating contextual understanding, personalized preferences, and advanced search capabilities. Traditional -based searches often produce generic results, even with precise s. In contrast, AI analyzes user behavior, past purchases, and browsing history to deliver highly relevant suggestions. For instance, a user searching for “running shoes” might receive different results based on their previous purchases of athletic apparel, their location, and even the time of day.
Traditional methods simply display listings based on relevance without considering such context. This contextual understanding is a key differentiator, enhancing the overall shopping experience and increasing the likelihood of a purchase.
Key Features of AI-Powered E-commerce Search
The capabilities of AI-powered search extend far beyond basic matching. Several key features significantly improve the user experience and drive sales.
- Voice Search: This allows users to search using natural language voice commands, making the process more intuitive and convenient, especially on mobile devices. For example, a user could say “Find me waterproof hiking boots size 10” and receive relevant results immediately.
- Visual Search: This enables users to upload an image of a product they like and find similar items or the exact product from different retailers. This is particularly useful when a user knows what they want but doesn’t know the product name or specific details.
- Personalized Search Results: AI algorithms analyze user data to personalize search results, displaying products that align with individual preferences, past purchases, and browsing history. This significantly improves the relevance of search results and increases the chances of conversion.
AI’s Impact on Product Discoverability and Cart Abandonment
AI dramatically improves product discoverability by presenting users with relevant products they might not have otherwise found through traditional searches. This leads to increased sales and customer satisfaction. For example, a user searching for “winter coat” might be shown a variety of styles, colors, and brands, including options they might not have considered based on their past purchase history.Furthermore, AI helps reduce cart abandonment rates by providing personalized recommendations and addressing potential issues proactively.
If a user abandons their cart, AI can send targeted email reminders, offer discounts or promotions, or highlight related products that might complete their purchase. By anticipating and addressing potential obstacles, AI contributes to a smoother and more efficient checkout process, reducing cart abandonment and increasing conversion rates. For example, Amazon’s “frequently bought together” suggestions are a prime example of AI driving increased sales by suggesting complementary products, directly addressing the potential issue of incomplete purchases.
AI for Fraud Detection and Prevention in E-commerce
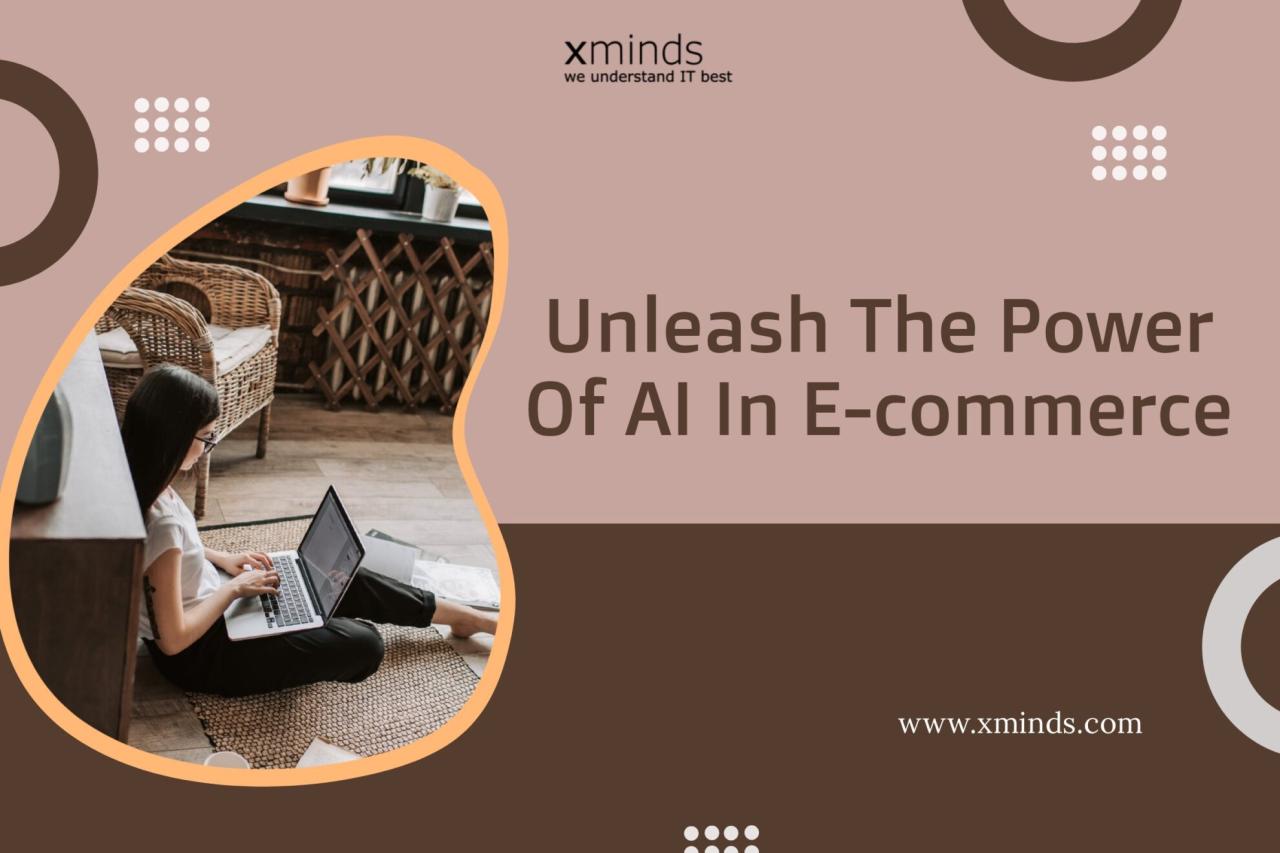
The rise of e-commerce has unfortunately brought with it a corresponding increase in fraudulent activities. From credit card theft to account takeovers, online retailers face significant financial and reputational risks. Fortunately, advancements in artificial intelligence (AI) offer powerful tools to combat these threats, enhancing security and bolstering customer trust. AI’s ability to analyze vast datasets and identify subtle patterns makes it a crucial asset in the fight against online fraud.AI plays a vital role in identifying and preventing fraudulent transactions by analyzing transactional data and user behavior to detect anomalies indicative of malicious activity.
This involves deploying sophisticated algorithms that can sift through massive amounts of data far more quickly and efficiently than human analysts, pinpointing potentially fraudulent transactions in real-time. This proactive approach minimizes financial losses and protects both the business and its customers.
Anomaly Detection Techniques
Anomaly detection forms the cornerstone of many AI-based fraud detection systems. These techniques focus on identifying transactions or user behaviors that deviate significantly from established norms. For instance, an algorithm might flag a purchase made from an unusual location or involving an unexpectedly large sum of money, especially if it’s inconsistent with the user’s past spending habits. Machine learning models, particularly unsupervised learning methods like clustering and one-class SVM, are frequently employed for this purpose.
These models can learn the characteristics of legitimate transactions and then identify outliers that are likely fraudulent.
Machine Learning Models for Fraud Detection
Various machine learning models are used to build predictive fraud detection systems. Supervised learning models, trained on historical data labeled as fraudulent or legitimate, are particularly effective. These models learn to identify patterns associated with fraudulent activities and assign probabilities to new transactions, indicating the likelihood of fraud. Commonly used models include logistic regression, support vector machines (SVMs), and decision trees, often combined in ensemble methods like random forests or gradient boosting machines for improved accuracy.
These models continuously learn and adapt as new data becomes available, enhancing their accuracy over time. For example, a model trained on past data showing a high correlation between fraudulent transactions originating from specific IP addresses and unusually high transaction values could be used to flag similar transactions in the future.
Benefits of AI-Driven Fraud Prevention
The implementation of AI in fraud prevention yields several significant benefits. Firstly, it leads to a substantial reduction in financial losses resulting from fraudulent activities. By identifying and preventing fraudulent transactions in real-time, businesses can minimize chargebacks, refunds, and other associated costs. Secondly, AI enhances customer trust and loyalty. Customers are more likely to shop with businesses that demonstrate a strong commitment to security and data protection.
Finally, AI-driven fraud prevention systems often automate many aspects of the process, freeing up human resources to focus on other critical tasks. This efficiency translates to cost savings and improved operational effectiveness.
AI-Based Fraud Detection Process Flowchart
Imagine a flowchart depicting the process. The process begins with Transaction Monitoring, where AI algorithms analyze incoming transactions against established baselines and predefined rules. This is followed by Anomaly Scoring, where each transaction receives a score based on the likelihood of fraud. Transactions exceeding a certain threshold trigger an Alert Generation, notifying relevant personnel. Finally, a Response phase ensues, involving investigation, verification, and potential blocking of suspicious transactions.
This cyclical process ensures continuous monitoring and adaptation to evolving fraud techniques. The flowchart visually represents the iterative nature of AI-driven fraud detection, highlighting the seamless integration of data analysis, risk assessment, and real-time response.
AI and the Future of E-commerce Customer Experience
The integration of artificial intelligence (AI) is rapidly transforming the e-commerce landscape, promising a future where customer experiences are hyper-personalized, seamless, and incredibly efficient. We’ve already seen the initial waves of AI-powered personalization and customer service, but the coming years will bring even more profound changes, driven by advancements in several key areas.
The evolution of AI in e-commerce isn’t just about incremental improvements; it’s a fundamental shift in how businesses understand and interact with their customers. This shift necessitates a proactive approach to understanding the implications, both positive and negative, of this technological leap.
Emerging AI Technologies Shaping Future E-commerce Experiences, Ai in e commerce and customer experience
The next generation of e-commerce will be defined by increasingly sophisticated AI technologies. These technologies will move beyond simple recommendation engines and chatbots to create truly immersive and personalized shopping experiences.
Several technologies are poised to significantly impact the future of e-commerce customer experience. These advancements will lead to more intuitive and engaging interactions between businesses and consumers.
- Augmented Reality (AR): AR overlays digital information onto the real world, allowing customers to virtually “try on” clothes, visualize furniture in their homes, or examine products in detail before purchasing. For example, an online furniture retailer could use AR to allow customers to place a virtual 3D model of a sofa in their living room using their smartphone camera, providing a realistic preview of how it would look and fit.
- Virtual Assistants (VAs): Beyond basic chatbots, future VAs will leverage natural language processing (NLP) and machine learning (ML) to understand complex customer queries, anticipate needs, and provide proactive support. Imagine a VA that not only answers product questions but also proactively suggests complementary items based on past purchases and browsing history, offering personalized styling advice or suggesting alternative products if an item is out of stock.
- Hyper-Personalization Engines: AI algorithms will analyze vast amounts of customer data to create truly individualized shopping experiences. This goes beyond simple product recommendations; it involves tailoring the entire website layout, product descriptions, and even the tone of communication to match each customer’s preferences and behavior. For example, a fashion e-commerce site might present different product categories and visual styles based on a customer’s past purchases and stated style preferences.
- Predictive Analytics for Customer Service: AI can predict potential customer issues before they arise. For example, an AI system could identify customers at risk of churning based on their browsing behavior or recent interactions, allowing proactive intervention by customer service representatives.
Challenges and Opportunities of Increased AI Adoption
The widespread adoption of AI in e-commerce presents both exciting opportunities and significant challenges. Navigating these carefully will be crucial for businesses seeking to leverage AI effectively.
Balancing the potential benefits with the inherent risks associated with AI implementation requires careful planning and execution. A thoughtful approach is necessary to ensure that AI enhances, rather than detracts from, the overall customer experience.
- Data Privacy and Security: The use of AI relies heavily on customer data, raising concerns about privacy and security. Businesses must implement robust data protection measures and be transparent about how customer data is used.
- Algorithmic Bias: AI algorithms can inherit and amplify existing biases in the data they are trained on, leading to unfair or discriminatory outcomes. Careful attention must be paid to mitigating bias in AI systems to ensure fairness and equity.
- Job Displacement: Automation through AI may lead to job displacement in certain areas of e-commerce, requiring retraining and upskilling initiatives for affected workers.
- Maintaining the Human Touch: While AI can automate many tasks, it’s crucial to maintain a human element in customer interactions. A balance between AI-powered efficiency and human empathy is essential for a positive customer experience.
- Cost of Implementation: Implementing and maintaining AI systems can be expensive, requiring significant investment in infrastructure, talent, and ongoing development.
Predictions on the Transformation of Business-Customer Relationships
The integration of AI will fundamentally reshape the relationship between businesses and customers in e-commerce. This transformation will lead to more personalized, proactive, and efficient interactions.
The future of e-commerce hinges on the successful integration of AI, and the resulting impact on the customer-business relationship will be profound. This requires a strategic approach to both implementation and ongoing management.
- Proactive Customer Service: AI will enable businesses to anticipate customer needs and provide support before problems arise, leading to increased customer satisfaction and loyalty. For example, an AI system might identify a customer who is likely to abandon their shopping cart and send a personalized email with an incentive to complete their purchase.
- Hyper-Personalized Experiences: E-commerce will move beyond generic recommendations to deliver truly individualized experiences, catering to each customer’s unique preferences and needs. This will create a stronger sense of connection and loyalty.
- Increased Customer Engagement: AI-powered features like AR and interactive chatbots will create more engaging and immersive shopping experiences, leading to increased time spent on websites and higher conversion rates. Examples include interactive product demos and virtual try-on experiences.
- Data-Driven Decision Making: Businesses will leverage AI-generated insights to make better decisions about product development, marketing, and customer service, leading to improved efficiency and profitability. This could involve analyzing customer feedback to improve product design or optimizing marketing campaigns based on predicted customer behavior.
Closing Summary: Ai In E Commerce And Customer Experience
The integration of AI in e-commerce isn’t just a trend; it’s a fundamental shift in how businesses operate and connect with their customers. By leveraging AI’s power to personalize experiences, enhance customer service, and optimize various processes, businesses can create more engaging and efficient online marketplaces. While challenges around data privacy and ethical considerations remain, the potential for AI to transform the customer experience is immense.
The future of e-commerce is undoubtedly intertwined with the continued advancement and responsible implementation of artificial intelligence.
FAQ Compilation
What are the biggest challenges in implementing AI in e-commerce?
Data privacy concerns, the cost of implementation, the need for skilled personnel, and ensuring ethical and unbiased AI algorithms are key challenges.
How can businesses ensure ethical AI practices in e-commerce?
Transparency in data usage, regular audits for bias detection, robust data security measures, and adherence to relevant regulations are crucial for ethical AI implementation.
Will AI replace human customer service agents?
No, AI is more likely to augment human agents, handling routine tasks and freeing up human agents to focus on complex issues and personalized interactions.
What are some examples of AI-powered personalization beyond product recommendations?
Personalized website layouts, targeted advertising, customized email content, and dynamic pricing are other examples of AI-driven personalization.