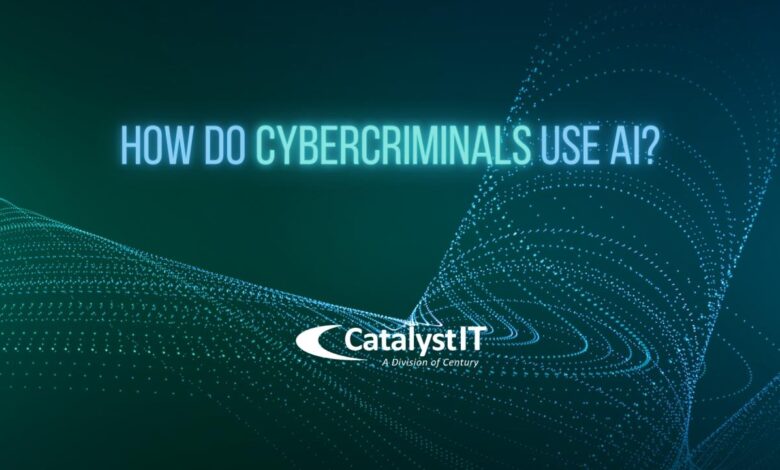
AI is the New Major Accomplice for Cyber Crimes
AI is the new major accomplice for cyber crimes. It’s a chilling thought, isn’t it? The same technology promising to revolutionize our lives is being weaponized by malicious actors to wreak havoc on a scale never before imagined. This isn’t just about a few rogue programmers; AI’s ability to automate, scale, and personalize attacks is transforming the cybercrime landscape, making it more sophisticated, more widespread, and far more difficult to defend against.
We’re diving deep into this evolving threat, exploring how AI is being used, the challenges it presents, and what we can do to fight back.
From AI-powered phishing campaigns that expertly mimic human communication to self-learning malware that constantly adapts and evades detection, the threat is real and rapidly escalating. We’ll examine specific examples, explore the ethical and legal implications, and discuss the crucial role of human expertise alongside AI in building a stronger cybersecurity posture. Get ready to uncover the dark side of artificial intelligence and learn how we can stay ahead of the curve.
The Evolving Landscape of Cybercrime
The digital age has witnessed a dramatic escalation in cybercrime, evolving from simple hacking attempts to sophisticated, AI-powered attacks. This shift represents a significant threat to individuals, businesses, and even national security. Understanding this evolution is crucial to developing effective countermeasures.
Historical Progression of Cybercrime
Early cybercrime primarily involved relatively unsophisticated methods like viruses and simple denial-of-service attacks. The motivations were often opportunistic, focusing on individual gain or vandalism. However, with the rise of the internet and increasingly interconnected systems, cybercrime became more organized and lucrative. Criminal groups began collaborating, developing more complex malware, and targeting larger organizations for significant financial gain.
The introduction of artificial intelligence marked a turning point, enabling automation, personalization, and a dramatic increase in the scale and efficiency of cyberattacks. AI’s ability to analyze vast amounts of data to identify vulnerabilities, personalize phishing attacks, and automate the creation and deployment of malware has fundamentally changed the cybercrime landscape.
Types of Cybercrime Significantly Impacted by AI
AI has significantly amplified the threat posed by several types of cybercrime. Phishing attacks, for instance, are now far more convincing due to AI’s ability to generate personalized and highly targeted messages. Malware creation and deployment have also been revolutionized; AI can automatically generate variations of malware, making it harder to detect and defend against. Furthermore, AI is increasingly used in advanced persistent threats (APTs), where attackers maintain long-term access to a system, often undetected.
The use of AI in social engineering attacks is also growing, enabling more effective manipulation of individuals to obtain sensitive information.
Comparison of Traditional and AI-Driven Cybercrime Methods
Traditional cybercrime methods often relied on manual processes, requiring significant human effort to identify targets, develop attacks, and deploy malware. These methods were often less efficient and more easily detected. AI-driven methods, however, automate many of these steps, significantly increasing the speed, scale, and sophistication of attacks. AI can analyze massive datasets to identify vulnerabilities, personalize attacks, and adapt to defensive measures, making them far more difficult to counteract.
The shift from manual to automated attacks represents a fundamental change in the nature of the threat. For example, a traditional phishing campaign might involve sending generic emails to a large number of recipients. An AI-powered campaign, however, could leverage data analysis to target specific individuals with highly personalized messages, significantly increasing the success rate.
Impact of AI on Various Cybercrime Types
Cybercrime Type | Traditional Method | AI-Enhanced Method | Impact |
---|---|---|---|
Phishing | Generic emails sent to large lists | Personalized emails targeting specific individuals based on data analysis | Increased success rate, more difficult to detect |
Malware Creation | Manual coding and deployment | Automated generation and deployment of variations of malware | Increased volume and sophistication of malware, faster adaptation to defenses |
Denial-of-Service (DoS) Attacks | Using botnets of compromised computers | Using AI to coordinate attacks and overwhelm defenses more effectively | Larger-scale and more effective attacks, harder to mitigate |
Social Engineering | Reliance on generic manipulation tactics | Highly personalized manipulation based on individual profiles and behavioral data | Increased success rate in obtaining sensitive information |
AI Techniques Used in Cybercrime
The rise of artificial intelligence has unfortunately ushered in a new era of sophisticated cybercrime. Criminals are leveraging AI’s capabilities to automate attacks, enhance their effectiveness, and evade detection at an unprecedented scale. This section delves into the specific AI techniques employed in modern cybercriminal activities.
Machine Learning in Phishing and Social Engineering
Machine learning algorithms are proving invaluable to cybercriminals in crafting highly targeted and persuasive phishing campaigns and social engineering attacks. These algorithms analyze vast datasets of personal information gleaned from various sources – social media, data breaches, and public records – to create incredibly realistic and personalized phishing emails. For example, an algorithm might identify an individual’s professional role, recent travel plans, or even their family members’ names, weaving this information into a seemingly legitimate email from a trusted source.
This level of personalization significantly increases the likelihood of a successful attack, as victims are more likely to trust communications that appear tailored to their specific circumstances. Furthermore, machine learning can be used to optimize the timing and delivery of phishing emails, maximizing their impact by sending them when a target is most likely to be receptive.
AI-Powered Malware Capabilities
AI is revolutionizing malware creation, leading to the emergence of self-learning, adaptive malware that can evade traditional security measures. One example is malware that uses reinforcement learning to modify its behavior based on its interactions with a system’s defenses. This allows it to bypass firewalls, antivirus software, and intrusion detection systems, making it significantly harder to detect and remove.
Another example is polymorphic malware that utilizes AI to constantly change its code structure, making signature-based detection methods obsolete. This adaptability makes these threats incredibly difficult to contain and neutralize, necessitating proactive and constantly evolving security strategies.
Deep Learning in Malware Creation
Deep learning, a subset of machine learning, allows for the creation of even more sophisticated and undetectable malware. Deep learning algorithms can generate highly complex and obfuscated code that is difficult for even experienced security analysts to understand and analyze. This complexity makes it challenging to identify malicious patterns and develop effective countermeasures. Furthermore, deep learning can be used to generate adversarial examples – subtly altered inputs that cause a system to misbehave in unexpected ways – enabling attackers to bypass security systems designed to detect malicious activity.
The use of generative adversarial networks (GANs) in particular allows for the creation of incredibly realistic and difficult-to-detect malware variants.
Natural Language Processing in Phishing and Identity Creation
Natural Language Processing (NLP) is a crucial component of many AI-powered cyberattacks. NLP algorithms are used to generate highly convincing phishing emails that mimic the writing style and tone of legitimate organizations. These emails are often indistinguishable from authentic communications, making them highly effective in deceiving unsuspecting victims. Beyond email creation, NLP also plays a significant role in generating believable fake identities for use in various online scams and fraudulent activities.
This involves creating realistic profiles on social media platforms and other online services, allowing attackers to build trust with their targets before launching an attack.
AI-Powered Phishing Attack Flowchart
A typical AI-powered phishing attack might follow these steps:[Imagine a flowchart here. The boxes would be:
- Data Collection (Social media scraping, data breaches, etc.)
- Target Profile Creation (AI analyzes data to identify high-value targets)
- Phishing Email Generation (AI crafts personalized emails using NLP)
- Email Delivery (Automated email sending systems)
- Victim Interaction (User clicks on malicious link or opens attachment)
- Malware Deployment (Payload is delivered and executed)
- Data Exfiltration (Sensitive data is stolen and transmitted to attacker)
- Attacker Analysis (AI analyzes success rate and refines future attacks)]
The Role of AI in Amplifying Cybercrime: Ai Is The New Major Accomplice For Cyber Crimes
AI is no longer a futuristic concept; it’s a powerful tool readily available to both benevolent and malicious actors. Its application in cybercrime is rapidly evolving, significantly increasing the scale, sophistication, and efficiency of attacks. This amplification poses a considerable threat to individuals, businesses, and national security, demanding a proactive and adaptive response from the cybersecurity community.AI’s ability to process vast amounts of data at incredible speeds allows for the automation of tasks previously requiring significant human effort.
This automation translates directly into a higher volume of attacks and a reduced response time for malicious actors. Furthermore, AI’s capacity for learning and adaptation allows it to overcome traditional security measures with increasing effectiveness. The resulting increase in the efficiency and scale of cybercrime represents a major challenge to existing security infrastructures.
AI-Powered Automation of Attacks
AI algorithms can automate various stages of a cyberattack, from reconnaissance and target selection to the execution and dissemination of malware. For example, AI-powered bots can scan networks for vulnerabilities far more quickly and efficiently than human hackers, identifying weaknesses that might otherwise go unnoticed. Similarly, AI can automate the creation and deployment of phishing emails, crafting personalized messages designed to deceive specific individuals or groups.
The result is a significant increase in the volume and success rate of phishing campaigns. Consider the example of a sophisticated botnet leveraging AI to identify and exploit zero-day vulnerabilities – this would allow for near-instantaneous exploitation of newly discovered weaknesses, significantly outpacing traditional security patching cycles.
AI’s Role in Bypassing Security Measures
AI’s capacity for adaptive learning makes it exceptionally adept at bypassing traditional security measures. Machine learning algorithms can analyze security protocols, identify patterns, and develop strategies to circumvent them. This includes techniques like evading intrusion detection systems (IDS) and antivirus software by modifying malware to avoid detection. For instance, AI can analyze the behavior of antivirus software and generate variations of malware designed to evade its detection algorithms.
The continuous adaptation and learning of these AI-powered attacks make them particularly difficult to counter with static security measures.
AI-Driven Targeted Attacks
AI significantly enhances the ability of cybercriminals to target specific individuals or organizations. By analyzing vast amounts of data from social media, online forums, and other sources, AI can build detailed profiles of potential targets, identifying their vulnerabilities and preferences. This information can then be used to create highly targeted phishing campaigns or develop custom malware tailored to exploit specific weaknesses within a target’s system.
Imagine a scenario where AI is used to analyze an organization’s internal communications, identifying key personnel and their communication patterns to launch a highly effective spear-phishing attack. The precision and personalization of these AI-driven attacks dramatically increase their effectiveness.
Vulnerabilities Introduced by AI in Security Systems
The integration of AI into security systems, while offering benefits, also introduces new vulnerabilities. These vulnerabilities stem from the inherent limitations and potential biases of AI algorithms, as well as the potential for malicious actors to exploit these systems.
- Data Poisoning: Malicious actors can introduce biased or corrupted data into the training sets of AI-powered security systems, leading to inaccurate or ineffective detection.
- Adversarial Attacks: AI models can be vulnerable to adversarial attacks, where carefully crafted inputs can cause the system to misclassify or fail to detect malicious activity.
- Model Extraction: Attackers can attempt to extract the underlying model of an AI-powered security system, allowing them to understand its limitations and develop countermeasures.
- Lack of Explainability: The “black box” nature of some AI algorithms can make it difficult to understand why a system made a particular decision, hindering troubleshooting and remediation efforts.
- Over-reliance on AI: Overdependence on AI-powered security systems without sufficient human oversight can create vulnerabilities if the AI system fails or is compromised.
Defending Against AI-Powered Cybercrime
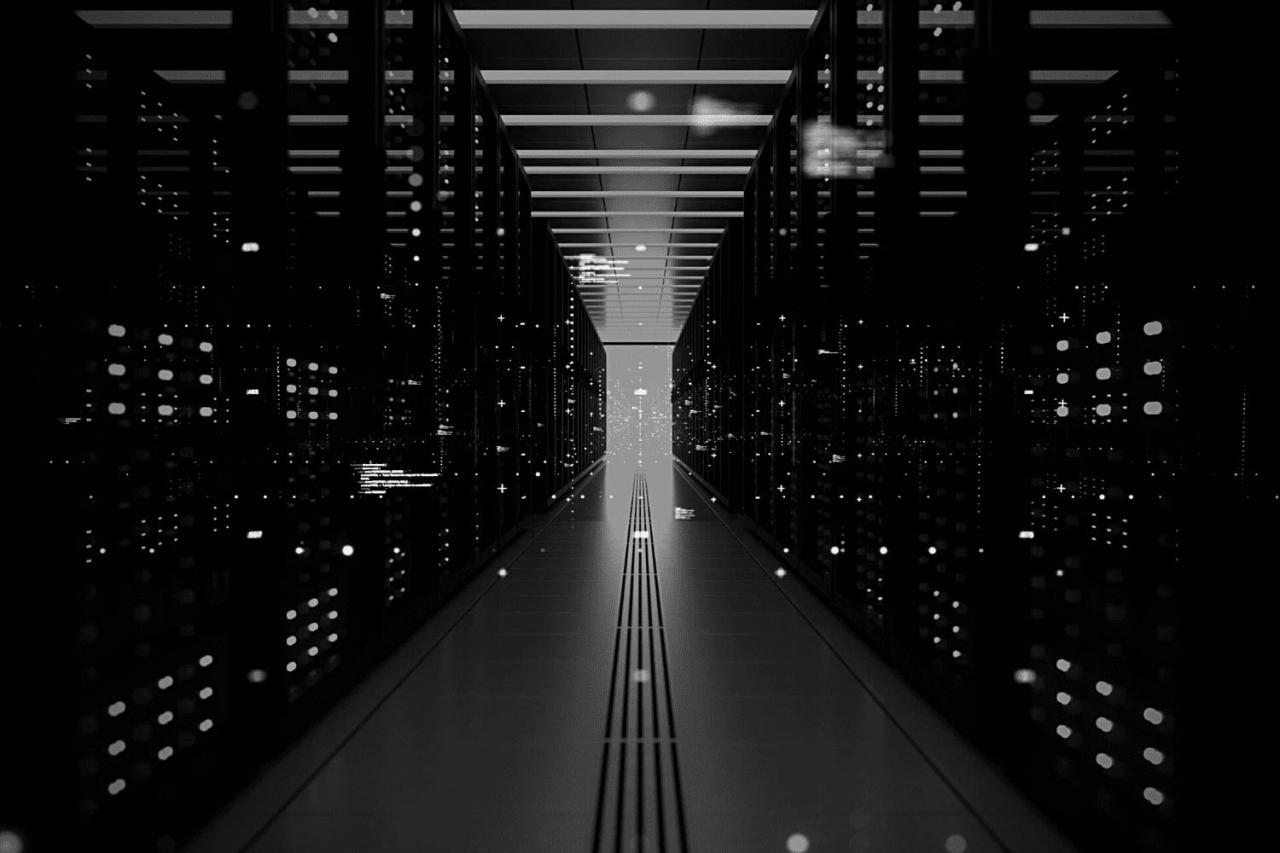
The rise of AI in cybercrime presents unprecedented challenges to cybersecurity defenses. Traditional security measures are often insufficient to combat the sophisticated and adaptive nature of AI-driven attacks. Developing robust defenses requires a multi-layered approach combining advanced technologies with human expertise.
Challenges Posed by AI in Cybersecurity Defense
AI-powered attacks are characterized by their ability to automate malicious activities at scale, learn and adapt to defensive strategies, and evade detection mechanisms. This presents significant challenges for security teams who must constantly update their defenses to keep pace. The sheer volume of data generated by AI-driven attacks can overwhelm traditional security systems, making it difficult to identify and respond to threats in a timely manner.
Furthermore, the use of AI in creating highly targeted and personalized phishing campaigns makes it harder to distinguish legitimate communications from malicious ones. The complexity of AI algorithms also makes it difficult to understand the attack mechanisms and develop effective countermeasures.
Advanced Security Measures to Counter AI-Driven Attacks, Ai is the new major accomplice for cyber crimes
Addressing the challenges requires a proactive and multifaceted approach. Advanced security measures must leverage AI and machine learning to detect and respond to threats in real-time. This includes implementing advanced threat detection systems that utilize machine learning algorithms to identify anomalies and patterns indicative of malicious activity. Behavioral analytics can monitor user and system activity, flagging suspicious behaviors that deviate from established baselines.
Sandboxing environments allow for the safe analysis of suspicious files and code without risking infection of the main system. Furthermore, robust security information and event management (SIEM) systems are crucial for aggregating and analyzing security data from various sources, providing a comprehensive view of the security landscape. Regular security audits and penetration testing help identify vulnerabilities and weaknesses in the system before they can be exploited.
Finally, proactive threat hunting, actively searching for malicious activity, is becoming increasingly important in detecting sophisticated AI-driven attacks before they cause significant damage.
Comparison of AI-Based Security Solutions
Several AI-based security solutions are available, each with its strengths and weaknesses. For example, some solutions focus on detecting malware based on its behavior, while others specialize in identifying phishing attempts through natural language processing. The effectiveness of each solution depends on factors such as the specific type of AI-driven attack, the data used to train the AI model, and the sophistication of the threat actors.
A comprehensive security strategy often involves integrating multiple AI-based solutions to provide a layered defense. Some solutions may excel at detecting known threats, while others are better suited for identifying novel and unknown attacks. The choice of solution should be based on a thorough risk assessment and consideration of the specific needs of the organization.
The Importance of Human Expertise Alongside AI in Cybersecurity
While AI plays a crucial role in enhancing cybersecurity defenses, human expertise remains indispensable. AI systems can be trained to detect known patterns, but they may struggle with novel or highly sophisticated attacks. Human analysts are needed to interpret the results of AI-based security tools, investigate suspicious activity, and make informed decisions about how to respond to threats.
The human element is also critical for developing and refining AI-based security systems, ensuring that they are effective against evolving threats. Furthermore, human judgment is necessary for ethical considerations and legal compliance in cybersecurity incident response.
AI-Driven Cybersecurity Measures
Security Measure | Description | Effectiveness against AI Attacks | Limitations |
---|---|---|---|
Advanced Threat Detection | Utilizes machine learning to identify anomalies and patterns indicative of malicious activity. | High, particularly for detecting known attack patterns. | May struggle with novel or highly sophisticated attacks; requires continuous training and updates. |
Behavioral Analytics | Monitors user and system activity to detect deviations from established baselines. | Moderate to high, effective in detecting insider threats and compromised accounts. | Can generate false positives; requires careful configuration and tuning. |
Sandboxing | Provides a safe environment for analyzing suspicious files and code. | High for analyzing malware and identifying malicious behavior. | May not be effective against attacks that use advanced obfuscation techniques. |
Security Information and Event Management (SIEM) | Aggregates and analyzes security data from various sources, providing a comprehensive view of the security landscape. | High for correlation of security events and identifying complex attacks. | Requires significant expertise to configure and manage effectively; can be resource-intensive. |
Ethical and Legal Implications
The rise of AI in cybercrime presents a complex web of ethical and legal challenges unlike anything we’ve seen before. The very nature of AI – its ability to learn, adapt, and automate – exacerbates existing vulnerabilities while creating entirely new ones. This necessitates a careful examination of the ethical dilemmas involved and a robust reassessment of our existing legal frameworks.
The speed of AI development far outpaces the legal and regulatory responses, leaving us in a constant game of catch-up.The ethical dilemmas are multifaceted. The development and deployment of AI tools for malicious purposes raise serious questions about the responsibility of creators, distributors, and users. Is the programmer of a sophisticated AI-powered phishing tool culpable if their creation is used to defraud thousands?
What about the company that provides the cloud computing resources used to train and run these tools? Determining liability in such scenarios is extraordinarily difficult, especially when AI systems exhibit emergent behavior – actions that are not explicitly programmed but arise from the complex interaction of its components. Furthermore, the anonymity and scalability afforded by AI-powered attacks raise significant ethical concerns about fairness, accountability, and the potential for disproportionate harm.
Current Legal Frameworks and Their Limitations
Existing laws, primarily focused on traditional cybercrime, struggle to keep pace with the rapid evolution of AI-enabled attacks. Laws addressing hacking, fraud, and data breaches often lack the specificity needed to prosecute AI-driven crimes effectively. The difficulty in attributing responsibility – determining who or what is legally liable for an AI-powered attack – presents a significant hurdle. For example, prosecuting the creators of an autonomous botnet that evolves its attack strategies independently presents unique legal challenges.
Current legal frameworks often focus on individual actors, while AI-powered attacks can be orchestrated by decentralized, anonymous networks. This necessitates a shift towards a more holistic approach that considers the entire ecosystem involved in the development, deployment, and use of AI in cybercrime.
Future Legal Challenges Posed by AI in Cybercrime
The future promises even greater challenges. The development of increasingly sophisticated AI, including general-purpose AI, will lead to more autonomous and adaptable cyberattacks. Imagine AI systems capable of independently identifying vulnerabilities, exploiting them, and even adapting their strategies in response to defensive measures. Attributing responsibility becomes even more complex when dealing with AI systems that can learn and evolve beyond the initial programming.
Furthermore, the use of AI in deepfakes and other forms of synthetic media presents new legal and ethical concerns, blurring the lines between reality and fabrication and potentially undermining trust in information sources. The potential for widespread social disruption and political manipulation through AI-powered disinformation campaigns also necessitates urgent attention.
Key Stakeholders and Their Roles
Addressing the challenges of AI in cybercrime requires a collaborative effort from various stakeholders. Governments must develop and implement comprehensive legal frameworks that account for the unique characteristics of AI-powered attacks. This includes defining clear lines of responsibility, establishing mechanisms for attribution, and creating effective deterrents. Law enforcement agencies need to invest in training and resources to investigate and prosecute these complex crimes.
Technology companies play a crucial role in developing secure AI systems and collaborating on the development of effective countermeasures. International cooperation is also essential, as cybercrime often transcends national borders. Academic researchers and cybersecurity experts contribute to our understanding of the risks and developing effective solutions.
Recommendations for Policy Makers and Industry Leaders
The following recommendations aim to address the growing threat of AI-powered cybercrime:
- Develop clear legal definitions of AI-enabled cyberattacks, addressing issues of attribution and liability.
- Invest in research and development of AI-based cybersecurity solutions to detect and prevent AI-powered attacks.
- Establish international cooperation frameworks to share information and coordinate responses to cross-border AI-enabled cybercrime.
- Promote ethical guidelines for the development and deployment of AI, emphasizing responsible innovation and minimizing potential harm.
- Create robust educational and training programs to equip law enforcement and cybersecurity professionals with the skills needed to combat AI-powered cybercrime.
- Incentivize the development of AI systems that are inherently secure and resistant to malicious use.
- Foster collaboration between governments, law enforcement, technology companies, and academia to address the challenges posed by AI in cybercrime.
Illustrative Examples of AI in Cybercrime
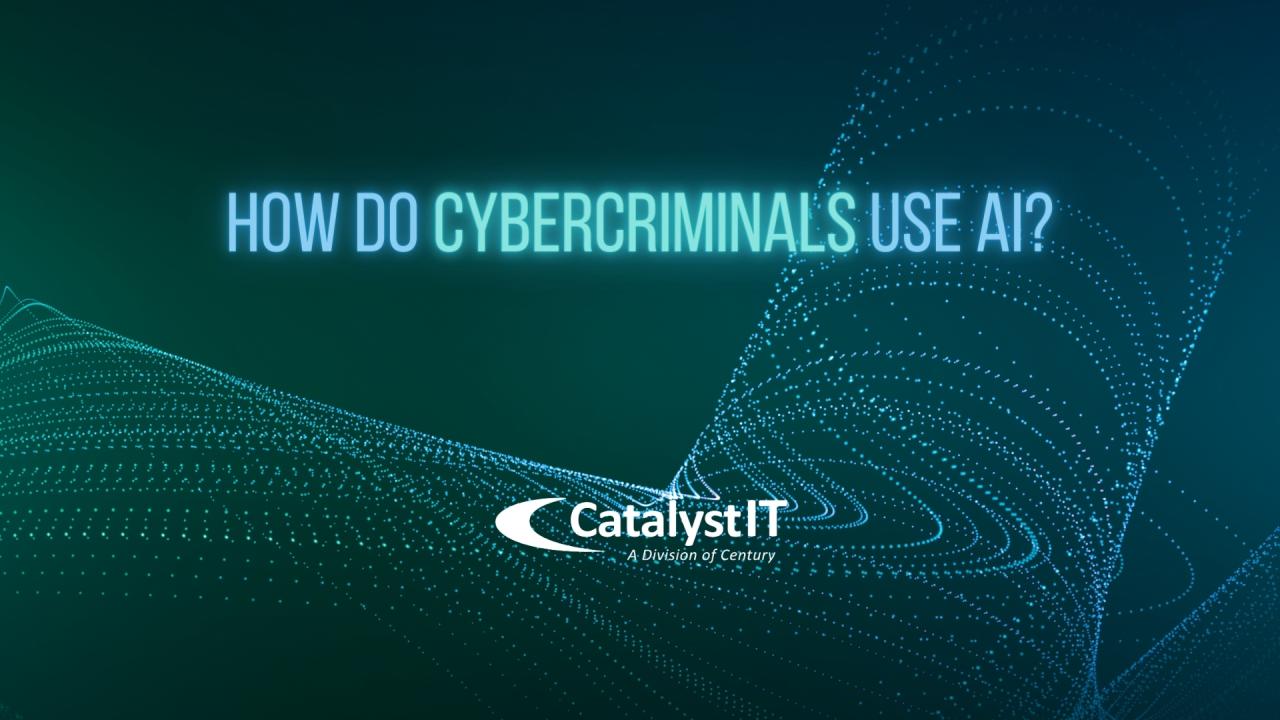
AI’s capabilities are rapidly transforming the cybercrime landscape, enabling attackers to execute sophisticated and large-scale attacks with unprecedented efficiency. The following examples illustrate how AI is being weaponized, highlighting the evolving threat and the need for robust countermeasures.
AI-Powered Large-Scale Data Breach
Imagine a scenario where a sophisticated cybercriminal group deploys an AI-powered botnet. This botnet doesn’t simply brute-force passwords; it leverages machine learning algorithms to analyze vast amounts of publicly available data – social media profiles, leaked databases, and even news articles – to build highly accurate profiles of potential targets. The AI identifies individuals with weak passwords, easily guessable security questions, or predictable behavioral patterns.
It then prioritizes targets based on the potential value of their data. The attack is highly targeted and efficient, resulting in a massive data breach affecting millions of individuals. The stolen data, ranging from financial information to personal health records, is then sold on the dark web, causing significant financial losses and reputational damage to victims. Authorities struggle to trace the attack due to the decentralized and automated nature of the botnet, highlighting the challenge of attributing responsibility and pursuing legal action against the perpetrators.
The response involves a coordinated effort by law enforcement agencies and private sector cybersecurity firms, but the damage is already done, and the long-term consequences for victims are severe.
AI-Generated Deepfakes for Social Engineering
Consider a scenario where a cybercriminal uses generative AI to create a highly realistic deepfake video of a company CEO announcing a sudden, urgent transfer of funds. The AI is trained on publicly available videos and audio recordings of the CEO, meticulously replicating their voice, mannerisms, and facial expressions. This deepfake is then sent to the company’s finance department via a seemingly legitimate email.
The realism of the deepfake is so convincing that the finance team, believing it to be a genuine instruction from their CEO, processes the fraudulent transfer. Millions of dollars are stolen before the fraud is discovered. The impact on the company is devastating, involving not only significant financial losses but also reputational damage and potential legal repercussions.
Tracing the origin of the deepfake and identifying the perpetrators becomes a complex and challenging task for law enforcement. The incident highlights the growing sophistication of social engineering attacks and the potential for AI to dramatically increase their effectiveness.
AI-Driven Automated Zero-Day Vulnerability Exploitation
In this scenario, an AI system is used to autonomously identify and exploit zero-day vulnerabilities in software. The AI employs advanced techniques like fuzzing and symbolic execution to systematically test software for weaknesses. It then automatically generates exploit code to leverage these vulnerabilities. This process is far faster and more efficient than manual methods, allowing the AI to discover and exploit zero-day vulnerabilities before they are patched by software vendors.
The consequences could range from widespread malware infections and data breaches to the disruption of critical infrastructure. The scale and speed of such attacks would make them incredibly difficult to contain and mitigate. The AI’s ability to learn and adapt would also make it challenging to develop effective defenses, necessitating a constant arms race between attackers and defenders.
The rapid evolution of AI-driven exploitation techniques poses a significant threat to cybersecurity.
Wrap-Up
The rise of AI-powered cybercrime presents a formidable challenge, but it’s not insurmountable. While the sophistication of these attacks is undeniably alarming, the innovative use of AI in cybersecurity defense offers a glimmer of hope. The future of cybersecurity hinges on a collaborative effort – governments, law enforcement, technology companies, and individuals all need to work together to develop and implement robust defenses.
By understanding the threat landscape and embracing proactive security measures, we can mitigate the risks and build a more resilient digital world. The fight is on, and the stakes are higher than ever.
Commonly Asked Questions
What are some common types of AI-powered cyberattacks?
AI is used in various attacks, including sophisticated phishing scams, the creation of highly convincing deepfakes for social engineering, automated discovery and exploitation of zero-day vulnerabilities, and large-scale data breaches.
How can I protect myself from AI-powered cyberattacks?
Strengthening passwords, being wary of suspicious emails and links, keeping software updated, using multi-factor authentication, and regularly backing up data are crucial first steps. Investing in robust security software and staying informed about the latest threats are also essential.
Is AI being used to defend against cyberattacks as well?
Yes, AI is increasingly used for cybersecurity defense. AI-powered systems can analyze vast amounts of data to detect anomalies, predict threats, and automatically respond to attacks. However, human expertise remains crucial for oversight and strategic decision-making.
What legal and ethical implications does the use of AI in cybercrime raise?
The use of AI in cybercrime raises complex ethical and legal questions surrounding accountability, responsibility, and the potential for misuse. Existing legal frameworks are often inadequate to address the novel challenges posed by AI-enabled attacks, necessitating the development of new laws and regulations.