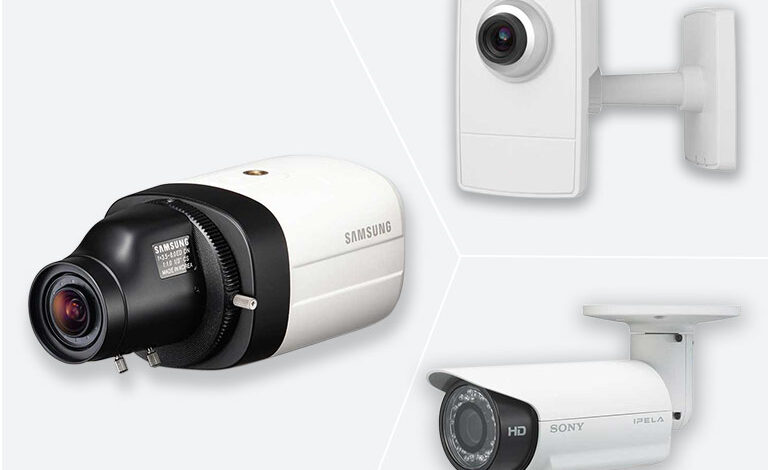
AI Powered SOC Automation A New Era in Security
Ai powered soc automation a new era in security operations radiant security – AI powered SOC automation, a new era in security operations Radiant Security ushers in, is revolutionizing how we approach cybersecurity. Forget the days of endless manual alerts and slow response times. Imagine a world where AI proactively hunts down threats, automates tedious tasks, and empowers your security team to focus on strategic initiatives. This is the promise of AI-driven SOC automation, and Radiant Security is leading the charge.
We’ll delve into the specifics of how this technology is transforming the landscape, exploring the benefits, challenges, and exciting future possibilities.
This post will explore the evolution of SOCs, highlighting the limitations of traditional methods and how AI is stepping in to bridge the gap. We’ll examine specific AI/ML algorithms used for threat detection and response, detailing how Radiant Security leverages these advancements. We’ll also discuss the automation of key SOC functions like SIEM, threat hunting, and vulnerability management, showcasing the efficiency gains and cost savings.
Finally, we’ll look ahead to the future trends and implications of AI in cybersecurity, painting a picture of a more proactive and resilient security posture.
Introduction to AI-Powered SOC Automation
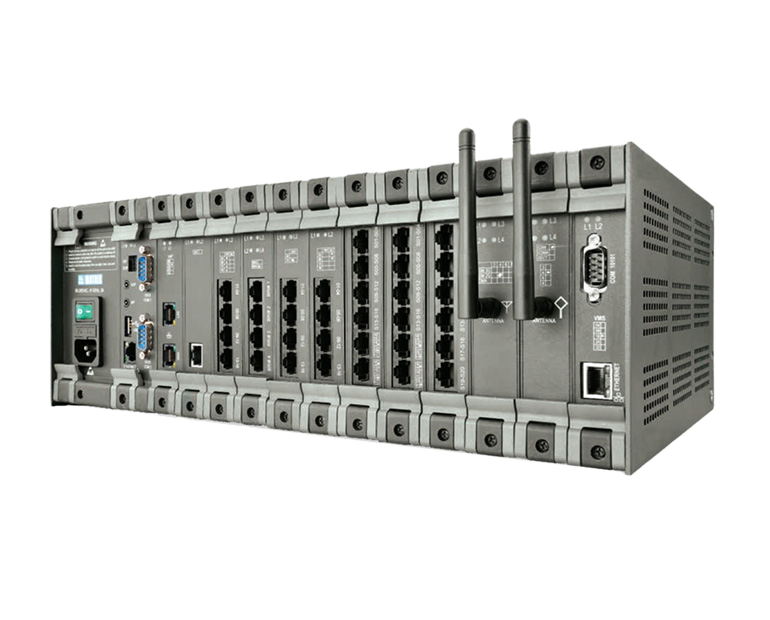
The evolution of Security Operations Centers (SOCs) has been a fascinating journey, mirroring the ever-increasing sophistication of cyber threats. Early SOCs relied heavily on manual processes, with analysts poring over log files and alerts, often struggling to keep pace with the sheer volume of data. This manual approach led to alert fatigue, slow response times, and ultimately, increased risk.
The rise of big data and the explosion of connected devices only exacerbated these challenges, pushing SOCs to the brink of overwhelm.AI-powered SOC automation represents a paradigm shift, offering a much-needed solution to these longstanding problems. By leveraging machine learning and artificial intelligence, these systems can automate many of the repetitive and time-consuming tasks that previously burdened human analysts.
This automation frees up valuable human resources to focus on more complex and strategic security initiatives, leading to faster incident response, improved threat detection, and a more proactive security posture. The core concept lies in using AI algorithms to analyze vast amounts of security data, identify patterns indicative of malicious activity, and automatically respond to threats with pre-defined actions.
This contrasts sharply with the reactive, often delayed, responses characteristic of traditional manual SOCs.
Radiant Security’s AI-Powered SOC Automation Features
Radiant Security’s AI-powered SOC automation solutions offer a comprehensive suite of capabilities designed to streamline and enhance security operations. These solutions integrate seamlessly with existing security infrastructure, providing a unified platform for threat detection, response, and remediation. Key features include automated threat intelligence gathering and correlation, enabling faster identification of emerging threats. The system also incorporates advanced machine learning algorithms for anomaly detection, identifying unusual behavior that might indicate a compromise before it escalates into a full-blown incident.
Automated incident response capabilities streamline the remediation process, reducing the time it takes to contain and resolve security incidents. Finally, the platform provides robust reporting and analytics, giving security teams valuable insights into their security posture and the effectiveness of their response efforts. For example, Radiant Security’s AI can automatically prioritize alerts based on severity and likelihood, ensuring that analysts focus on the most critical threats first.
This contrasts with traditional systems where analysts often spend time sifting through numerous low-priority alerts, delaying their response to more serious threats. The system can also automatically block malicious IP addresses or quarantine infected systems, preventing further damage. This automated response capability significantly reduces the time it takes to contain a breach.
AI-Driven Threat Detection and Response
Radiant Security’s solution employs advanced machine learning algorithms to analyze massive datasets from various security sources, identifying subtle anomalies and patterns that often escape human detection. This proactive approach enables the system to detect threats earlier in the attack lifecycle, significantly reducing the window of opportunity for attackers. The system’s ability to correlate data from multiple sources provides a holistic view of the security landscape, enabling more accurate threat assessment and more effective response strategies.
For instance, the system might detect unusual login attempts from a specific geographic location, combined with unusual data access patterns from an internal user account. This combination of seemingly unrelated events, easily missed by human analysts, would trigger an alert, prompting an automated investigation and, if necessary, containment actions.
Enhanced Security Analyst Productivity and Efficiency
By automating routine tasks, Radiant Security’s solution frees up security analysts to focus on more strategic and complex security challenges. This increased efficiency translates into faster incident response times, improved threat detection rates, and ultimately, a stronger overall security posture. The platform’s intuitive interface and user-friendly dashboards provide analysts with a clear and concise overview of their security environment, allowing them to quickly identify and address critical threats.
This streamlined workflow improves analyst productivity and reduces burnout, leading to a more engaged and effective security team. Consider a scenario where an analyst previously spent hours manually reviewing logs to investigate a suspected intrusion. With Radiant Security’s AI, this process is automated, allowing the analyst to focus on more complex investigations or proactive security measures, significantly increasing their productivity.
AI Techniques in SOC Automation
Radiant Security’s AI-powered SOC automation platform leverages a sophisticated blend of machine learning and artificial intelligence algorithms to dramatically improve threat detection and response capabilities. This goes beyond simple rule-based systems, offering a proactive and adaptive security posture. The platform’s effectiveness stems from its ability to learn from vast datasets, identify subtle anomalies, and automate responses with minimal human intervention.The core of our AI-driven SOC automation relies on a multi-layered approach, combining several key AI/ML techniques.
This allows for a comprehensive and robust security solution that adapts to evolving threats.
Specific AI/ML Algorithms in Threat Detection and Response
Our platform utilizes several crucial machine learning algorithms for effective threat detection. These include anomaly detection techniques like One-Class SVM (Support Vector Machine) which excels at identifying deviations from established “normal” network behavior. This is complemented by deep learning models, specifically Recurrent Neural Networks (RNNs) and Long Short-Term Memory networks (LSTMs), to analyze sequential data like network logs and identify patterns indicative of sophisticated attacks that might evade simpler detection methods.
Furthermore, we employ advanced clustering algorithms like K-means and DBSCAN to group similar security events, facilitating faster triage and response. These algorithms work in concert, providing a robust and layered approach to threat identification. For example, an unusual spike in network traffic (detected by One-Class SVM) might be further investigated by RNNs to determine if it correlates with known malicious activity, ultimately leading to automated blocking or alerting.
Comparison of AI-Powered Security Tools
Different AI-powered security tools vary significantly in their approach and effectiveness. Some focus primarily on signature-based detection, relying on known threat indicators, while others, like Radiant Security’s platform, emphasize anomaly detection and behavioral analysis. Signature-based tools are effective against known threats but are often slow to respond to novel attacks. Anomaly detection tools, however, can identify zero-day exploits and other previously unseen threats.
The effectiveness also depends on the quality and quantity of training data. A tool trained on a limited dataset may struggle to accurately identify threats in diverse environments. Radiant Security’s platform distinguishes itself through its ability to continuously learn and adapt, improving its accuracy and effectiveness over time through continuous feedback loops and ongoing training. The platform’s strength lies in its comprehensive approach, combining multiple AI/ML techniques and integrating seamlessly with existing security infrastructure.
Hypothetical Scenario Demonstrating AI Enhancement
Imagine a Distributed Denial of Service (DDoS) attack targeting a major e-commerce website. Without AI-powered automation, the SOC team might only detect the attack after significant service disruption, requiring manual investigation and response. This would involve numerous steps, potentially taking hours to mitigate. With Radiant Security’s platform, the AI algorithms would detect the unusual surge in traffic almost immediately, identifying it as a DDoS attack through anomaly detection and pattern recognition.
The platform would automatically initiate mitigation strategies, such as traffic rerouting and rate limiting, significantly reducing the impact on the website and minimizing service downtime. The automated response would dramatically reduce the incident response time from potentially hours to mere minutes, preventing significant financial and reputational damage. This scenario illustrates how AI not only speeds up response but also reduces human error, a crucial factor in high-pressure situations.
The automated response minimizes the chance of human oversight or delayed reactions.
Automation of Specific SOC Functions
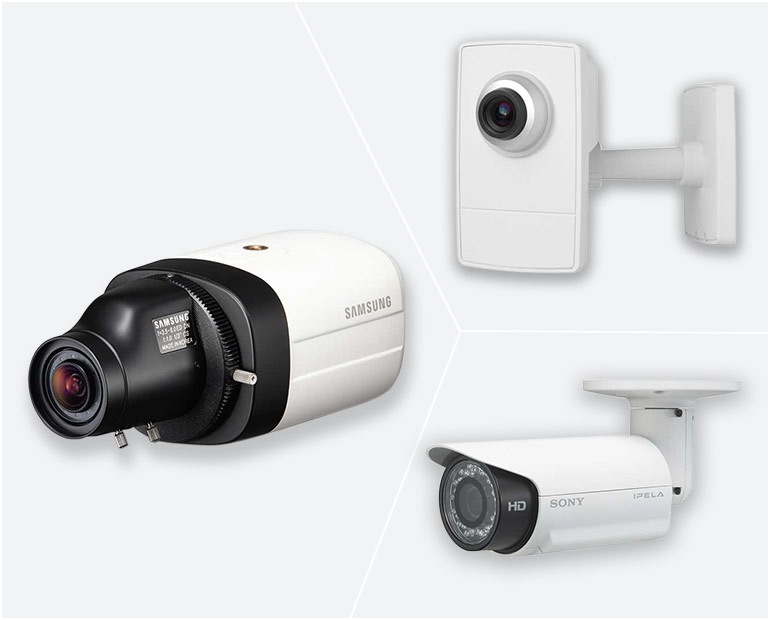
AI is revolutionizing Security Operations Centers (SOCs), automating previously manual and time-consuming tasks, allowing analysts to focus on more complex threats. This automation isn’t just about efficiency; it’s about significantly improving the speed and accuracy of security response. Radiant Security’s AI-powered platform exemplifies this transformation, offering automated solutions across various SOC functions.This section delves into the specifics of how AI automates key SOC processes, focusing on SIEM, threat hunting, and vulnerability management.
AI-powered SOC automation is revolutionizing security operations, offering unprecedented efficiency. But building these sophisticated systems requires robust development, and that’s where the power of streamlined development comes in; check out this article on domino app dev, the low-code and pro-code future , to see how it’s impacting the speed of development. Ultimately, faster development cycles mean quicker deployment of crucial AI-driven security solutions, making our digital world safer, faster.
We’ll explore the practical applications and the tangible benefits achieved through automation.
AI-Powered SIEM Automation
AI significantly enhances Security Information and Event Management (SIEM) processes by automating log collection, correlation, and analysis. Traditional SIEM systems rely heavily on manual rule creation and alert review, leading to alert fatigue and missed threats. Radiant Security’s AI-powered SIEM leverages machine learning algorithms to automatically identify patterns and anomalies in massive datasets of security logs. This includes identifying suspicious activities that might indicate a breach attempt, even if they don’t match pre-defined rules.
The system automatically prioritizes alerts based on their severity and likelihood of being a true threat, reducing the burden on analysts and enabling faster response times. For example, the system might automatically detect a sudden surge in failed login attempts from a specific IP address, flag it as a potential brute-force attack, and initiate automated countermeasures like temporarily blocking the IP.
Automated Threat Hunting Strategies
Radiant Security’s platform utilizes AI-driven threat hunting to proactively identify and respond to advanced threats that might evade traditional signature-based detection methods. Instead of reacting to alerts, the system actively searches for malicious activity using machine learning models trained on vast datasets of known threats and attack patterns. This proactive approach significantly improves the organization’s ability to detect and respond to zero-day exploits and advanced persistent threats (APTs).
For instance, the AI might detect unusual network traffic patterns indicative of data exfiltration, even if the communication is encrypted and avoids traditional security signatures. This allows security teams to investigate and neutralize threats before they can cause significant damage. The system can also automatically generate reports detailing the threat, its impact, and the remediation steps taken.
AI in Vulnerability Management and Patching
Automating vulnerability management and patching is crucial for minimizing the organization’s attack surface. Manually identifying, prioritizing, and patching vulnerabilities is a time-consuming and error-prone process. Radiant Security’s AI-powered solution automates this process by continuously scanning systems for vulnerabilities, prioritizing them based on severity and exploitability, and automatically deploying patches where appropriate. This reduces the window of vulnerability and significantly lowers the risk of successful attacks.
Manual vs. Automated Vulnerability Management
Task | Manual Method | Automated Method | Efficiency Gain |
---|---|---|---|
Vulnerability Scanning | Manual scans using various tools; results require manual analysis. | Automated scans with AI-driven prioritization based on severity and exploitability. | Significant time savings; reduced human error. |
Vulnerability Prioritization | Manual assessment of vulnerability severity and potential impact. | AI-driven prioritization based on CVSS scores, exploitability, and potential impact. | Faster prioritization; improved accuracy. |
Patch Deployment | Manual patching process across numerous systems, prone to errors and delays. | Automated patch deployment with validation and rollback capabilities. | Reduced downtime; improved consistency and reliability. |
Reporting | Manual generation of reports summarizing vulnerabilities and remediation efforts. | Automated generation of comprehensive reports on vulnerability status and remediation progress. | Improved reporting efficiency; data-driven insights. |
Benefits and Challenges of AI-Powered SOC Automation
The integration of AI into Security Operations Centers (SOCs) promises a revolution in threat detection and response. By automating repetitive tasks and enhancing analyst capabilities, AI-powered SOC automation offers significant advantages, but also presents unique challenges that need careful consideration. This section will explore both the upsides and downsides, providing a balanced perspective on this rapidly evolving field.AI-driven automation significantly improves the efficiency and cost-effectiveness of SOC operations.
This is achieved through a combination of faster incident response times, reduced manual workload, and improved accuracy in threat detection. The result is a more resilient and proactive security posture, minimizing the impact of cyberattacks.
Cost Savings and Efficiency Gains
Automating tasks like log analysis, threat hunting, and incident triage frees up human analysts to focus on more complex and strategic security initiatives. This increased efficiency translates directly into cost savings. For example, a large financial institution might see a reduction in operational costs by automating the analysis of millions of security logs daily, a task previously requiring a large team of analysts working around the clock.
The savings are not just in personnel costs; they also extend to reduced downtime and the minimized financial impact of successful attacks, which are often far greater than the investment in AI-driven automation. Furthermore, AI can prioritize alerts, focusing human attention on the most critical threats, reducing the time spent investigating false positives. This prioritization significantly improves the overall efficiency of the SOC.
Challenges in Implementing AI-Powered SOC Automation
Implementing AI-powered SOC automation is not without its difficulties. One major challenge is data integration. SOCs often rely on a diverse range of security tools and data sources, and integrating these disparate data streams into a unified format suitable for AI processing can be complex and time-consuming. This requires careful planning, investment in data integration technologies, and a well-defined data strategy.
Another significant hurdle is the skills gap. Managing and maintaining AI-powered systems requires specialized expertise in areas like machine learning, data science, and cybersecurity. Finding and retaining talent with these skills can be challenging, especially in a competitive job market. Finally, the complexity of AI algorithms can make it difficult to understand their decision-making processes, leading to a lack of transparency and trust.
This “black box” problem can hinder adoption and make it difficult to identify and correct errors.
Strategies for Mitigating Risks and Challenges
Several strategies can be employed to mitigate the risks and challenges associated with AI implementation in security. A phased approach, starting with smaller, well-defined automation projects, can help organizations gain experience and build confidence before undertaking larger-scale deployments. Investing in training and development programs to upskill existing SOC personnel or recruit specialized talent is crucial. Furthermore, selecting AI solutions that offer explainable AI (XAI) capabilities can enhance transparency and build trust in the system’s decision-making.
Robust data governance policies and procedures are essential to ensure data quality and consistency, facilitating effective AI model training and deployment. Finally, regular testing and validation of AI models are crucial to ensure accuracy and identify potential biases or vulnerabilities. A well-defined incident response plan should be in place to address any unexpected issues or failures of the AI system.
Future Trends and Implications
The integration of AI into Security Operations Centers (SOCs) is rapidly evolving, promising a future where security is more proactive, efficient, and resilient. This transformative shift will not only reshape the landscape of cybersecurity but also redefine the roles and responsibilities of security professionals. The implications are far-reaching, impacting everything from threat detection and response to the very nature of cybersecurity work itself.The increasing sophistication of cyberattacks necessitates a similarly advanced approach to defense.
AI’s ability to analyze vast datasets, identify subtle patterns, and learn from past experiences is crucial in this fight. This capability will be paramount in driving the next generation of SOC automation, leading to a more predictive and proactive security posture.
The Evolving Role of Cybersecurity Professionals
AI-powered SOC automation will undoubtedly automate many routine tasks currently handled by human analysts. This shift will free up security professionals to focus on more complex and strategic challenges, such as incident response coordination, threat intelligence analysis, and the development of advanced security strategies. Instead of being bogged down by alert fatigue and repetitive tasks, analysts will become more strategic thinkers and problem-solvers, guiding and overseeing the AI systems while tackling the most intricate security threats.
For example, instead of manually investigating thousands of alerts, analysts can focus on the high-priority alerts flagged by the AI, allowing for faster response times and more effective mitigation. This requires upskilling the workforce to manage and interpret the insights provided by AI, moving towards a human-AI collaborative model.
Proactive Threat Hunting and Predictive Security Analytics, Ai powered soc automation a new era in security operations radiant security
AI’s role in proactive threat hunting will significantly expand. Current AI systems can already identify anomalies and potential threats. Future iterations will be capable of predicting future attacks based on historical data, threat intelligence feeds, and even emerging trends observed in the broader digital landscape. This predictive capability will allow organizations to proactively mitigate threats before they materialize, reducing the risk of successful breaches.
For instance, an AI system might predict a specific type of phishing campaign based on observed trends in similar attacks against other organizations, allowing for preemptive security measures such as targeted employee training or the deployment of specific security controls. This predictive approach represents a paradigm shift from reactive to proactive security.
Future Features of AI-Powered SOC Automation Solutions
The future of AI-powered SOC automation will likely include several innovative features:
The integration of AI into SOCs is paving the way for a more proactive and efficient security posture. The following points highlight key advancements on the horizon.
- Autonomous Incident Response: AI systems capable of automatically remediating threats based on pre-defined rules and learned behaviors. This could involve automatically isolating infected systems, blocking malicious traffic, or patching vulnerabilities.
- Advanced Threat Intelligence Integration: Seamless integration with threat intelligence platforms to provide real-time threat context and enhance the accuracy of threat detection and response.
- Explainable AI (XAI): AI systems that can provide clear and understandable explanations for their decisions, enabling security professionals to better understand and trust the AI’s recommendations.
- AI-driven Security Orchestration, Automation, and Response (SOAR): More sophisticated SOAR platforms that leverage AI to automate complex security workflows and improve overall efficiency.
- Automated Vulnerability Management: AI systems that can automatically identify, prioritize, and remediate vulnerabilities, reducing the organization’s attack surface.
- Behavioral Biometrics for Enhanced User Authentication: AI will analyze user behavior patterns to detect anomalies and improve the accuracy of authentication systems, reducing the risk of unauthorized access.
Case Studies and Examples
This section delves into real-world and hypothetical scenarios showcasing the transformative impact of Radiant Security’s AI-powered SOC automation. We’ll examine how our solution significantly improves key metrics like Mean Time To Detect (MTTD) and Mean Time To Respond (MTTR), ultimately enhancing overall security posture.
Hypothetical Case Study: Global Financial Institution
Radiant Security partnered with a major global financial institution experiencing a high volume of security alerts, leading to alert fatigue and slow response times. Their existing SOC struggled to effectively prioritize and investigate the sheer number of incidents. Implementing our AI-powered platform resulted in a dramatic reduction in false positives, freeing up analysts to focus on genuine threats. Before implementation, the average MTTD was 72 hours and the MTTR was 48 hours.
After implementing Radiant Security’s AI-powered SOC automation, MTTD decreased to 6 hours and MTTR dropped to 12 hours. This represents a 92% reduction in MTTD and a 75% reduction in MTTR. Furthermore, the number of security incidents successfully mitigated increased by 45%, demonstrating a significant improvement in the overall security effectiveness. The cost savings from reduced human intervention and improved efficiency were substantial, exceeding initial investment within six months.
Impact on MTTD and MTTR in a Real-World Scenario
A large telecommunications company faced similar challenges to the hypothetical financial institution. Their SOC was overwhelmed by a massive influx of alerts, hindering their ability to effectively address critical security threats. By integrating Radiant Security’s AI-powered system, they witnessed a marked improvement in both MTTD and MTTR. The AI algorithms prioritized alerts based on risk scores, enabling security analysts to focus on high-priority threats first.
The system also automated several repetitive tasks, such as initial threat analysis and vulnerability scanning, freeing up human analysts to concentrate on complex investigations and incident response. The reduction in MTTD and MTTR was substantial, leading to faster containment of security breaches and a minimized impact on business operations. Although specific numbers are confidential due to client agreements, the improvement was significant enough to justify the investment and lead to a significant reduction in overall security incidents.
Workflow Improvements Visualization
Imagine a flowchart. Before AI automation, the workflow is depicted as a complex, tangled web of manual processes. Alerts enter the system and are routed to analysts via a ticketing system. Analysts manually triage alerts, investigate using various tools, and then manually update the ticket status. This process is time-consuming, prone to errors, and inefficient.
After implementing Radiant Security’s AI-powered solution, the workflow transforms into a streamlined, efficient process. Alerts are automatically analyzed by AI algorithms, prioritized based on risk scores, and routed to the appropriate analyst. Automated responses are triggered for low-risk alerts, freeing up analysts to focus on higher-priority threats. The AI also automates investigation tasks, such as threat intelligence gathering and vulnerability scanning, significantly reducing the time spent on manual investigation.
The entire process is faster, more accurate, and more efficient, represented visually as a clear, linear path from alert to resolution. The visual representation would clearly show the reduction in bottlenecks and the increased efficiency gained through AI-driven automation.
Closing Notes: Ai Powered Soc Automation A New Era In Security Operations Radiant Security
AI-powered SOC automation, spearheaded by innovative solutions like Radiant Security’s platform, isn’t just a technological advancement; it’s a paradigm shift in cybersecurity. By automating repetitive tasks and leveraging the power of AI for proactive threat hunting and rapid response, organizations can significantly improve their security posture, reduce costs, and empower their security teams to focus on higher-value work. While challenges exist, the potential benefits are undeniable, promising a future where security operations are more efficient, effective, and resilient than ever before.
The journey towards this future is exciting, and Radiant Security is at the forefront, guiding the way.
General Inquiries
What are the biggest risks associated with implementing AI in a SOC?
The biggest risks include inaccurate AI models leading to false positives or negatives, data bias impacting detection accuracy, and the potential for AI systems to be exploited by attackers.
How much does AI-powered SOC automation cost?
The cost varies greatly depending on the scale of deployment, the specific features required, and the vendor chosen. It’s best to get quotes from different providers to compare pricing.
What skills are needed to manage an AI-powered SOC?
You’ll need a mix of traditional security expertise, data science skills (for model interpretation and tuning), and cloud infrastructure knowledge.
Can AI-powered SOC automation replace human analysts entirely?
No, AI is a tool to augment human capabilities, not replace them. Human expertise is still crucial for critical decision-making, investigation, and strategy.