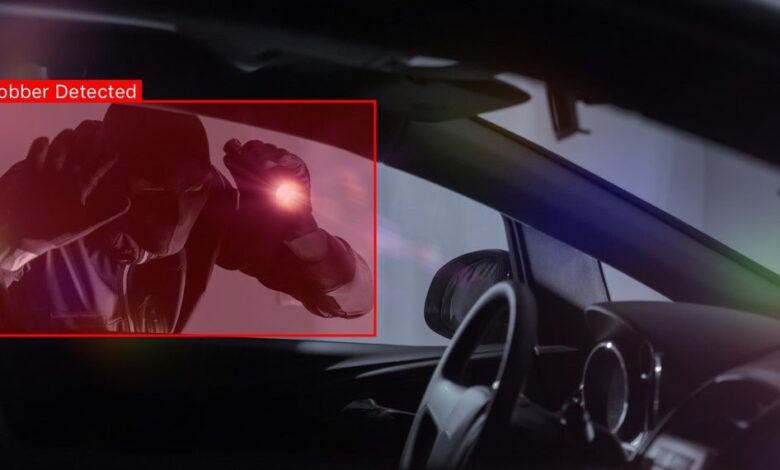
AI to Help British Police Detect Fake Crimes
AI to help British police detect fake crimes – sounds like something out of a sci-fi movie, right? But it’s a rapidly developing reality. Imagine a world where AI can sift through mountains of crime reports, instantly identifying inconsistencies in witness statements, analyzing photos for digital manipulation, and even flagging patterns suggestive of fraudulent claims. This isn’t just about saving police time; it’s about ensuring that genuine victims receive the support they need, and that resources aren’t wasted on fabricated incidents.
This post dives into the fascinating – and sometimes unsettling – world of AI’s role in crime detection.
We’ll explore how different AI technologies are being employed, the challenges of data privacy and bias in training these systems, and the ethical implications of using AI to judge the validity of crime reports. We’ll look at the potential for misidentification and the impact on police-community relations. It’s a complex issue with significant consequences, and I’m excited to unpack it with you.
Types of Fake Crimes Reported to British Police
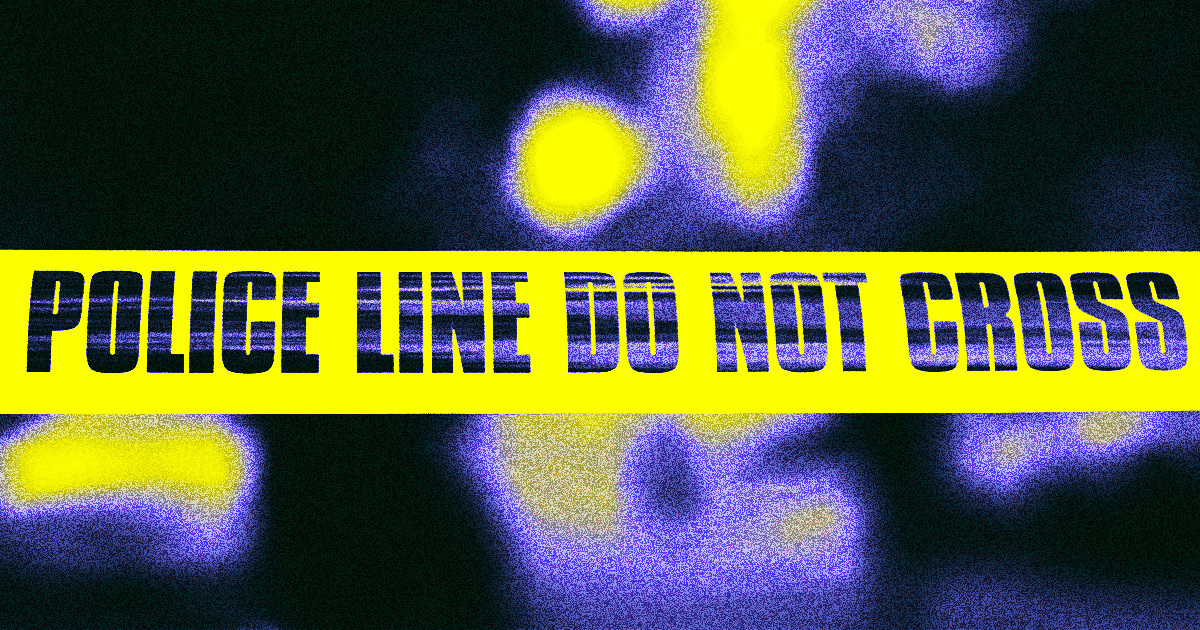
The British police force faces a significant challenge in dealing with false crime reports, which divert resources and undermine public trust. These fabricated reports range from relatively minor incidents to elaborate schemes designed for financial gain. Understanding the common types, motivations, and methods employed is crucial for effective policing and resource allocation. This exploration delves into the various categories of fake crimes, highlighting the tactics used and the difficulties in their detection.
Methods Used to Fabricate Evidence in False Crime Reports
False crime reports often rely on manufactured evidence to bolster their credibility. Common tactics include creating fake documents, such as forged receipts or altered photographs. Staging a crime scene, albeit poorly, is another method, aiming to create a convincing narrative. For example, someone claiming a burglary might deliberately scatter items around their home to mimic a break-in.
False testimonies, given either by the perpetrator or accomplices, are frequently used, often corroborated by fabricated witness statements. The use of technology, including creating fake social media posts or manipulating digital images, also plays a significant role in enhancing the plausibility of the false report. The sophistication of these methods varies greatly, from clumsy attempts to highly elaborate schemes.
Motivations Behind Reporting Fake Crimes
The reasons behind reporting fake crimes are diverse and often complex. Financial gain is a primary driver, particularly in insurance fraud cases. For instance, individuals might falsely claim their property was stolen or damaged to receive insurance payouts. Other motivations include seeking revenge against someone, attempting to cover up another crime, or escaping legal consequences. For example, someone might falsely accuse another individual of assault to divert attention from their own wrongdoing.
Sometimes, individuals might report fake crimes due to mental health issues or a desire for attention. The underlying psychology can be intricate and requires a nuanced understanding to effectively investigate and prosecute these cases.
Categorization of Fake Crimes and Detection Challenges
Crime Type | Common Tactics | Motivations | Detection Challenges |
---|---|---|---|
Insurance Fraud | Staging accidents, fabricating damage, falsifying documents. | Financial gain, avoiding premiums. | Verifying claims, identifying inconsistencies, tracing financial flows. |
Fabricated Assaults | Self-inflicted injuries, false witness statements, manipulated evidence. | Revenge, diverting attention from own actions, seeking sympathy. | Forensic evidence analysis, identifying inconsistencies in victim statements, corroborating witness accounts. |
False Burglary Reports | Staging a break-in, creating fake evidence of theft. | Insurance claims, covering up other crimes, seeking compensation. | Analyzing forensic evidence, reviewing security footage, detecting inconsistencies in victim accounts. |
False Allegations of Hate Crimes | Self-inflicted injuries, fabricated evidence of racist or homophobic abuse. | Seeking attention, sympathy, or financial compensation; revenge. | Thorough investigation, verifying witness accounts, identifying inconsistencies in the narrative. |
AI Technologies for Detecting False Crime Reports
The increasing sophistication of false crime reports necessitates the adoption of advanced technologies to assist law enforcement. Artificial intelligence offers a powerful toolkit for identifying inconsistencies and anomalies that might otherwise escape human scrutiny, allowing officers to focus their resources on genuine crimes. This involves leveraging several AI techniques to analyze various aspects of reported incidents, improving efficiency and accuracy in investigations.AI-powered image analysis and natural language processing (NLP) are particularly useful in identifying discrepancies in evidence and witness statements, respectively.
Furthermore, algorithmic anomaly detection can highlight unusual patterns in crime reporting data that might indicate fraudulent activity.
AI-Powered Image Analysis for Identifying Inconsistencies in Photographic Evidence, Ai to help british police detect fake crimes
AI algorithms can be trained to detect manipulations in photographic evidence, such as digital alterations or inconsistencies in lighting and shadows. For example, an algorithm could analyze the metadata embedded within an image file to detect inconsistencies between the claimed date and time of capture and the actual data recorded. Further analysis might reveal signs of cloning, splicing, or other digital manipulations, flagging the image for further human review.
The system could compare the image against a database of known manipulated images, identifying similarities and potential patterns of fraudulent activity. Sophisticated algorithms could even identify inconsistencies in the background of an image, such as the presence of objects that are out of place or inconsistent with the claimed location or time of the incident.
Natural Language Processing (NLP) for Detecting Inconsistencies in Witness Statements
NLP algorithms can analyze witness statements for inconsistencies, contradictions, or fabricated narratives. These algorithms can identify inconsistencies in timelines, descriptions of events, or the use of language. For instance, an NLP system might flag a statement that contains unusually high numbers of adverbs or adjectives, suggesting embellishment or exaggeration. It might also identify contradictions between different witness statements or inconsistencies between a witness’s statement and other available evidence.
The system could analyze sentence structure, word choice, and overall narrative coherence to assess the credibility of the statement. By comparing the statement against a database of known false reports, the system could also identify common patterns and red flags indicative of fabrication.
Anomaly Detection Algorithms for Identifying Unusual Patterns in Crime Reporting Data
Various AI algorithms can be employed to detect anomalies in crime reporting data. Outlier detection algorithms, for example, identify data points that significantly deviate from the norm. This could flag a surge in reports of a specific crime type from a particular location, or an unusually high number of reports submitted by a single individual. Anomaly detection algorithms, which are designed to identify unusual patterns or deviations from expected behavior, can be used to detect suspicious clusters of reports or unusual reporting patterns.
For instance, an algorithm might identify a sudden increase in reports of a specific crime type in a previously low-crime area, prompting further investigation. These algorithms can be particularly useful in identifying patterns of fraudulent reporting that might be difficult to detect through traditional methods. A real-life example might involve identifying a cluster of insurance fraud claims reported within a short timeframe, all exhibiting similar characteristics.
Data Sources and Preprocessing for AI Models
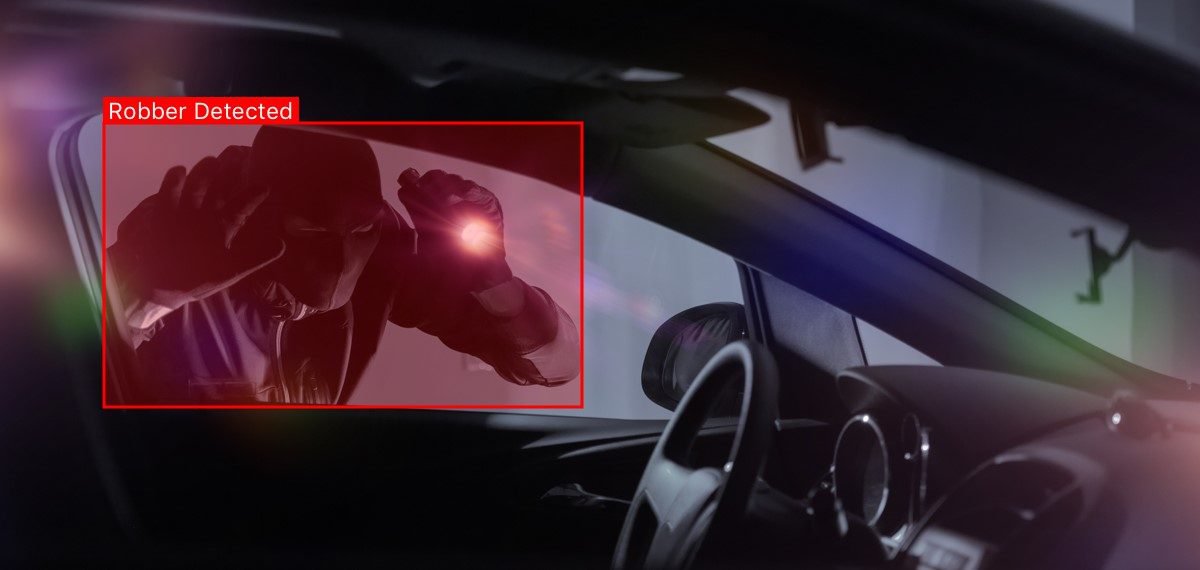
Building an effective AI system to detect false crime reports requires a robust data pipeline. This pipeline must efficiently collect, clean, and prepare data from various police databases for use in training and evaluating AI models. The quality of this data directly impacts the accuracy and reliability of the AI’s predictions. Careful consideration must also be given to ethical and privacy implications throughout this process.Data from multiple sources are necessary to provide a comprehensive picture of crime reports and their veracity.
These sources need to be integrated effectively, requiring careful planning and potentially custom data integration solutions.
Data Sources for AI Model Training
The primary data source will be the existing crime reporting databases used by British police forces. These databases contain detailed information on reported crimes, including victim statements, witness testimonies, suspect information (if available), and investigative notes. Supplementary data could include geographic information systems (GIS) data to analyze crime hotspots and patterns, potentially revealing inconsistencies that suggest false reporting.
Social media data, while requiring careful ethical consideration and legal compliance, could provide additional context and corroborating evidence in specific cases. Finally, historical data on proven false reports, if available, would be invaluable for training the AI to identify similar patterns in new reports. The integration of these diverse data sources presents a significant technical challenge, requiring expertise in data warehousing and ETL (Extract, Transform, Load) processes.
Data Preprocessing Challenges and Solutions
Preprocessing police data for AI training presents numerous challenges. Inconsistencies in data entry, missing values, and sensitive personal information require careful handling. Text data from crime reports needs to be cleaned and standardized, removing irrelevant information and converting unstructured text into a format suitable for machine learning algorithms. This might involve techniques like tokenization, stemming, and lemmatization to extract meaningful features from the text.
Numerical data might need scaling or normalization to prevent features with larger values from dominating the model.
Data Privacy and Ethical Considerations
Using police data for AI training raises significant privacy and ethical concerns. The data contains sensitive personal information, and its use must comply with data protection regulations like the UK GDPR. Anonymization techniques, such as differential privacy and data masking, are crucial to protect the identities of individuals involved in reported crimes. Transparency and accountability are paramount; the purpose of data use, the methods employed, and the potential risks must be clearly documented and communicated.
Ethical review boards should be consulted to ensure the responsible and ethical use of police data in AI development. Bias in the data itself needs careful examination and mitigation; historical biases within policing might be reflected in the data, potentially leading to unfair or discriminatory outcomes from the AI system.
Handling Missing or Incomplete Data
Missing or incomplete data is a common problem in crime reports. Several methods can be used to address this: imputation techniques (replacing missing values with estimated values based on other data points), removal of records with excessive missing data, or using algorithms robust to missing data. The choice of method depends on the nature and extent of the missing data and the specific AI model used.
For example, imputation methods like k-nearest neighbors or multiple imputation could be used to fill in missing values based on similar cases. However, these methods must be applied cautiously to avoid introducing bias or inaccuracies.
AI Model Training and Evaluation
Training an AI model to identify fake crime reports requires a robust methodology and careful evaluation. The goal is to create a system that can accurately distinguish between genuine incidents and fabricated reports, assisting police in prioritizing resources and investigations. This involves selecting appropriate algorithms, training data, and evaluation metrics, followed by a plan for continuous improvement.The selection of an appropriate training methodology is crucial.
Supervised learning, using a labelled dataset of both genuine and fake crime reports, is the most suitable approach. This dataset would need to be carefully curated, ensuring a balanced representation of various crime types and reporting styles to avoid bias. The model would learn to associate specific features within the reports (e.g., inconsistencies in narrative, unusual language, lack of corroborating evidence) with the label indicating whether the report is genuine or false.
A variety of algorithms could be employed, including Support Vector Machines (SVMs), Random Forests, or deep learning models like Recurrent Neural Networks (RNNs) or transformers, depending on the complexity of the data and desired performance. The choice would be driven by experimental evaluation on a held-out validation set.
Model Training Methodology
The training process would involve splitting the labelled dataset into training, validation, and test sets. The training set is used to train the model, the validation set is used to tune hyperparameters and prevent overfitting, and the test set provides an unbiased evaluation of the final model’s performance. The model would be trained iteratively, with performance monitored on the validation set.
Early stopping techniques would be employed to prevent overfitting, ensuring the model generalizes well to unseen data. Hyperparameter tuning would involve exploring different settings for the chosen algorithm (e.g., learning rate, regularization strength) to optimize performance.
Performance Evaluation Metrics
Several metrics are essential for evaluating the model’s performance. These include:
- Precision: The proportion of correctly identified fake crime reports out of all reports classified as fake. A high precision score indicates that the model rarely misclassifies genuine reports as fake.
- Recall: The proportion of correctly identified fake crime reports out of all actual fake crime reports. High recall indicates that the model effectively identifies most of the fake reports.
- F1-score: The harmonic mean of precision and recall, providing a balanced measure of the model’s overall performance. It is particularly useful when dealing with imbalanced datasets, where the number of genuine and fake reports differ significantly.
- Accuracy: The overall proportion of correctly classified reports (both genuine and fake). While useful, accuracy can be misleading with imbalanced datasets.
- AUC-ROC (Area Under the Receiver Operating Characteristic Curve): This metric measures the model’s ability to distinguish between genuine and fake reports across different thresholds. A higher AUC-ROC indicates better discriminatory power.
These metrics will be calculated on the held-out test set to provide an unbiased estimate of the model’s performance in a real-world scenario. For example, a model with 90% precision and 85% recall would indicate a high ability to accurately identify fake reports while minimizing false positives. The F1-score would provide a single number summarizing this performance.
Model Retraining and Improvement Plan
Ongoing model retraining and improvement are crucial for maintaining accuracy and adapting to evolving patterns in fake crime reporting. A continuous feedback loop will be established, incorporating real-world data and feedback from police officers using the system. This feedback will include:
- False positives: Genuine reports incorrectly classified as fake. Analysis of these cases will help identify areas where the model needs improvement and potential biases in the data.
- False negatives: Fake reports incorrectly classified as genuine. These cases will highlight weaknesses in the model’s ability to detect certain types of deception.
- Changes in reporting patterns: The model will need to be retrained periodically to adapt to new trends and tactics used in creating fake crime reports.
This feedback will be used to augment the training dataset with new examples, correct labelling errors, and potentially refine the model’s architecture or hyperparameters. Regular retraining, perhaps on a monthly or quarterly basis, will ensure the model remains effective and up-to-date. A robust monitoring system will track the model’s performance over time, allowing for proactive intervention if accuracy begins to decline.
For example, if the F1-score drops below a predefined threshold, a retraining cycle will be initiated. This iterative process ensures the AI system remains a valuable tool for the British police.
Integration with Existing Police Systems
Integrating an AI system for detecting false crime reports into the existing British police infrastructure requires a carefully planned approach, minimizing disruption to current workflows while maximizing the system’s effectiveness. This involves seamless data transfer, user-friendly interfaces, and robust security protocols. The ultimate goal is to provide officers with a powerful tool that enhances their investigative capabilities without adding unnecessary complexity.The AI system should integrate with existing crime reporting systems, such as the national crime recording system, allowing for automated analysis of incoming reports.
This integration could be achieved through Application Programming Interfaces (APIs), enabling the AI to access and process relevant data without requiring manual data entry or transfer. The system’s output, indicating the likelihood of a report being false, would then be presented to the investigating officer within their existing case management system, providing context and informing their decision-making process.
System Workflow and Data Flow
A flowchart visualizing the process would begin with a crime report being submitted through standard channels (phone, online portal, etc.). The report’s data is then automatically transferred via API to the AI system for analysis. The AI processes the data, considering various factors (e.g., language used, inconsistencies, location data, reporting history of the individual) and generates a probability score indicating the likelihood of the report being false.
This score, along with supporting evidence (e.g., flagged inconsistencies), is then presented to the investigating officer within their existing case management system. The officer reviews this information, and it informs their investigation strategy. The system also logs all interactions and analysis results for auditing and system improvement. A simplified representation might show a box for “Crime Report Submitted,” an arrow to “AI System Analysis,” an arrow to “Probability Score Generated,” an arrow to “Officer Review,” and finally an arrow to “Investigation Strategy Adjusted.”
Security Measures
Robust security measures are crucial to protect the AI system and its data from unauthorized access and manipulation. This includes implementing strong access controls, using encryption for data at rest and in transit, and regularly auditing system logs to detect any suspicious activity. The system should adhere to all relevant data protection regulations, such as the UK GDPR, ensuring that personal data is handled responsibly and securely.
AI is revolutionizing crime detection, helping the British police sift through mountains of data to identify fraudulent claims. Imagine streamlining this process with faster, more efficient applications built using the innovative techniques described in this article on domino app dev the low code and pro code future , which could significantly improve response times. Ultimately, this tech could free up officers to focus on real investigations, leading to a safer community.
Regular security assessments and penetration testing should be conducted to identify and address vulnerabilities. Furthermore, data access should be strictly limited to authorized personnel with appropriate roles and responsibilities, employing role-based access control (RBAC). The system should also include mechanisms for detecting and preventing attempts to manipulate or tamper with the AI model itself, potentially through adversarial attacks or data poisoning.
Regular updates and patches to address newly discovered vulnerabilities are also essential.
Ethical and Societal Implications
Deploying AI to detect false crime reports in the British police force presents a complex ethical landscape. The potential benefits – freeing up valuable police resources and ensuring a more efficient allocation of justice – must be carefully weighed against the risks associated with algorithmic bias, potential erosion of public trust, and unforeseen societal consequences. The inherent complexities of human behaviour and the nuances of criminal activity demand a cautious and responsible approach to AI implementation.The use of AI in law enforcement raises significant concerns about bias and fairness.
AI models are trained on data, and if that data reflects existing societal biases – for instance, over-policing of certain communities or disproportionate reporting of crimes against specific demographic groups – the AI system will likely perpetuate and even amplify these biases. This could lead to unfair or discriminatory outcomes, further marginalising already vulnerable populations.
Bias in AI Models and Mitigation Strategies
Addressing bias in AI models requires a multi-pronged approach. Firstly, careful curation of the training data is crucial. This involves auditing the data for existing biases, actively seeking out underrepresented groups, and employing techniques like data augmentation to balance the dataset. Secondly, algorithmic transparency is essential. Understanding how the AI model arrives at its conclusions allows for identification of potential biases within the algorithms themselves.
Finally, ongoing monitoring and evaluation of the AI system’s performance across different demographics is necessary to detect and correct for emerging biases. For example, if the AI consistently flags reports from a specific ethnic group as false with higher frequency than others, a thorough investigation into the underlying reasons is required, potentially involving human review of flagged reports and adjustments to the model’s parameters.
This ongoing feedback loop is crucial for ensuring fairness and equity.
Impact on Police-Community Relations
The introduction of AI into policing could significantly impact police-community relations, both positively and negatively. If the AI system is perceived as fair and unbiased, it could lead to increased trust and cooperation between the police and the public. However, if the AI system is perceived as discriminatory or unfair, it could exacerbate existing tensions and erode public trust.
The key to positive outcomes lies in transparency and community engagement. Open communication about the AI system’s capabilities, limitations, and potential biases is essential. Regular community consultations and feedback mechanisms can help ensure that the AI system is used responsibly and ethically, fostering trust and improving police-community relations. A successful implementation requires active engagement with the communities most likely to be affected, ensuring their concerns are heard and addressed.
Potential Unintended Consequences of AI in Crime Detection
The use of AI to detect fake crime reports, while aiming to improve efficiency, carries the potential for several unintended consequences. These consequences could have a significant societal impact.
- Increased workload for human investigators: While aiming to reduce workload, the AI might flag a large number of reports as potentially false, requiring manual review by human investigators, potentially increasing their workload rather than decreasing it.
- Discouragement of genuine crime reporting: If individuals perceive that their reports are frequently dismissed by the AI, they may become less likely to report genuine crimes in the future, leading to underreporting of crime.
- Misallocation of police resources: Incorrect flagging of genuine crimes as false could lead to a misallocation of police resources, delaying investigations and potentially impacting the safety of victims.
- Erosion of public trust in law enforcement: If the AI system is perceived as inaccurate or unfair, it could lead to a decline in public trust in the police and the justice system.
- Legal challenges and accountability issues: The use of AI in decision-making processes raises questions about legal liability and accountability. If the AI system makes an incorrect determination, who is responsible – the developers, the police force, or the AI itself?
Illustrative Case Studies
This section presents hypothetical case studies demonstrating how an AI system designed to detect false crime reports could assist British police. These examples highlight the system’s capabilities in analyzing various data points to identify inconsistencies and patterns indicative of fabricated claims. The AI’s analysis isn’t intended to replace human judgment but rather to provide valuable insights and improve investigative efficiency.
The following cases illustrate the AI’s functionality across different types of false crime reports. Each case demonstrates the AI’s ability to leverage diverse data sources and analytical techniques to flag potentially fraudulent claims.
Case Study 1: Insurance Fraudulent Car Theft
This case involves a report of a stolen vehicle used to claim insurance.
- Crime Reported: A high-value car was reported stolen from a residential driveway. The owner claimed no witnesses or security footage existed.
- AI Analysis: The AI cross-referenced the reported theft location with historical crime data, revealing a low incidence of car theft in that specific area. Furthermore, the AI detected inconsistencies between the owner’s statement and their mobile phone location data around the time of the alleged theft. The AI flagged the lack of security footage as unusual given the car’s value and the owner’s claimed affluence, and it noted inconsistencies in the description of the vehicle compared to official records.
- Outcome: The AI flagged the report as high-risk for fraud. A subsequent investigation revealed the owner had staged the theft to claim insurance.
Case Study 2: False Report of Assault
This case showcases the AI’s ability to detect inconsistencies in reports of violent crime.
- Crime Reported: An individual reported being violently assaulted in a public park, resulting in minor injuries. The victim provided a vague description of the assailant and claimed there were no witnesses.
- AI Analysis: The AI analyzed the victim’s statement for inconsistencies, comparing it to witness statements (obtained through social media analysis and CCTV footage from nearby businesses). The AI also considered the victim’s social media activity, noting a lack of posts about the alleged assault immediately following the event, despite frequent use of social media. The AI flagged the lack of corroborating evidence and inconsistencies in the victim’s timeline as suspicious.
- Outcome: The AI flagged the report as potentially false. Further investigation revealed the report was fabricated for personal reasons unrelated to an actual assault.
Case Study 3: Fabricated Burglary
This example illustrates how the AI can identify patterns and anomalies in burglary reports.
- Crime Reported: A burglary was reported at a residential property with a claim of significant items stolen. The homeowner reported no forced entry.
- AI Analysis: The AI analyzed the reported stolen items against the homeowner’s known possessions based on previous police interactions, tax records, and social media activity. The AI flagged discrepancies between the reported losses and the homeowner’s actual assets. The AI also identified that the homeowner had recently taken out a large loan and noted that the description of the stolen items was overly generic and lacked detail.
The lack of forced entry was also considered unusual.
- Outcome: The AI identified the report as potentially fraudulent. A subsequent investigation confirmed that the burglary was fabricated to cover financial debts.
Data Flow and AI Analysis for Case Study 1: Visual Representation
Imagine a flowchart. The initial input is the crime report itself, containing textual descriptions and details provided by the car owner. This data is then fed into the AI system. The AI accesses several databases: a database of historical crime data for the area, a database containing the owner’s mobile phone location data (obtained with a warrant), and a database of vehicle registration information.
The AI compares the reported theft location with the historical crime data, looking for anomalies in theft frequency. Simultaneously, it compares the owner’s stated location with their mobile phone location data at the time of the alleged theft. Finally, it compares the car’s description in the report with official registration data. The AI then processes this information using algorithms designed to identify inconsistencies and patterns indicative of fraud.
The output is a risk score, indicating the likelihood of the report being false. A high risk score triggers further investigation by human officers.
Final Thoughts
The use of AI to detect fake crimes in the UK is a double-edged sword. While the potential benefits – freeing up police resources, ensuring fair allocation of funds, and protecting genuine victims – are significant, the ethical considerations and potential for bias cannot be ignored. Ongoing monitoring, transparent development, and a commitment to mitigating bias are crucial for responsible implementation.
Ultimately, the goal is a fairer, more efficient justice system, and AI could play a powerful, albeit complex, role in achieving that.
Essential FAQs: Ai To Help British Police Detect Fake Crimes
What types of biases might be present in AI trained on police data?
AI models trained on historical police data might reflect existing societal biases, potentially leading to disproportionate flagging of certain demographics as reporting fake crimes.
How will this AI system impact police-community relations?
Successful implementation could improve trust by showing efficiency and focusing resources on genuine crimes. However, if perceived as unfair or biased, it could damage relations.
What happens if the AI system misidentifies a genuine crime report?
This is a critical concern. Human oversight and robust appeal processes are essential to prevent miscarriages of justice. Continuous model refinement and auditing will be vital.
Could this technology be used for purposes beyond detecting fake crimes?
Absolutely. The underlying technologies could be adapted for various applications, such as fraud detection in insurance claims or identifying patterns in other types of reports.