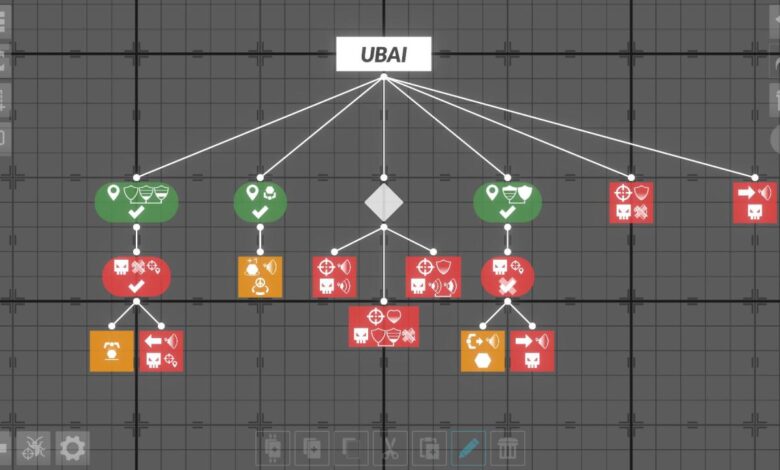
AI Will Be Powerful But No Panacea
AI Will Be Powerful But No Panacea – that’s the core message I want to explore today. We’re living in a time of incredible advancements in artificial intelligence, with AI impacting everything from healthcare to finance. It’s easy to get swept up in the hype, envisioning a future where AI solves all our problems. But the reality is far more nuanced.
This post delves into both the immense potential and the significant limitations of AI, aiming to paint a realistic picture of its role in shaping our future.
We’ll examine AI’s current capabilities, looking at where it excels and where it falls short. We’ll discuss the ethical dilemmas, the biases embedded within AI systems, and the crucial role human expertise continues to play. Ultimately, I hope to show that while AI is a powerful tool, it’s not a magic bullet – and understanding its limitations is just as important as celebrating its successes.
The Capabilities of AI
Artificial intelligence is rapidly transforming various sectors, offering unprecedented opportunities and challenges. While AI is not a panacea, its capabilities are already profoundly impacting how we live and work. Understanding its current strengths and limitations is crucial for harnessing its potential responsibly.
AI Capabilities Across Sectors
The following table summarizes the current state-of-the-art in AI applications across different sectors, highlighting both achievements and limitations.
Sector | Specific AI Application | Current Limitations | Potential Future Improvements |
---|---|---|---|
Healthcare | Disease diagnosis (e.g., cancer detection from medical images), drug discovery, personalized medicine | Data bias, lack of explainability in some models, regulatory hurdles for implementation, ethical concerns regarding patient data privacy. | Improved model accuracy and robustness, development of more explainable AI models, integration with electronic health records, streamlined regulatory processes. |
Finance | Fraud detection, algorithmic trading, risk assessment, customer service chatbots | Algorithmic bias leading to unfair lending practices, vulnerability to adversarial attacks, lack of transparency in complex models. | Development of fairer and more transparent algorithms, improved security measures against attacks, enhanced regulatory oversight. |
Manufacturing | Predictive maintenance, quality control, robotic process automation | High initial investment costs, need for skilled personnel to implement and maintain systems, potential for job displacement. | Reduced implementation costs, development of more user-friendly interfaces, reskilling initiatives for displaced workers, integration with the Internet of Things (IoT). |
Surpassing Human Capabilities, Ai will be powerful but no panacea
In specific, well-defined tasks, AI systems can surpass human capabilities. For example, AI algorithms excel at processing vast amounts of data far faster and more accurately than humans. Image recognition systems, for instance, can identify patterns in medical scans with higher accuracy than radiologists in certain cases, leading to earlier and more precise diagnoses. Similarly, AI-powered chess engines consistently defeat even the world’s best human players due to their ability to analyze millions of possible moves per second.
Areas Where AI Falls Short of Human Performance
Several key areas highlight where AI currently lags behind human performance:
AI’s limitations are significant and must be considered for responsible development and deployment:
- Common Sense Reasoning and Generalization: AI struggles with tasks requiring common sense reasoning and the ability to generalize knowledge to new situations. A human child easily understands that a bird cannot fly without wings; an AI may require extensive training data to learn this seemingly obvious fact.
- Creativity and Imagination: AI excels at pattern recognition and optimization but lacks the creativity and imagination necessary for original artistic expression, scientific breakthroughs, or innovative problem-solving in truly novel contexts.
- Emotional Intelligence and Empathy: Humans possess emotional intelligence and empathy, allowing them to understand and respond appropriately to complex social situations. AI systems currently lack these abilities, limiting their effectiveness in human-centric applications like customer service or healthcare.
- Ethical Considerations and Bias: AI systems can inherit and amplify biases present in their training data, leading to unfair or discriminatory outcomes. Addressing these ethical concerns is crucial for responsible AI development.
- Explainability and Transparency: Many sophisticated AI models, particularly deep learning systems, operate as “black boxes,” making it difficult to understand their decision-making processes. This lack of transparency can hinder trust and accountability.
AI’s Limitations and Biases
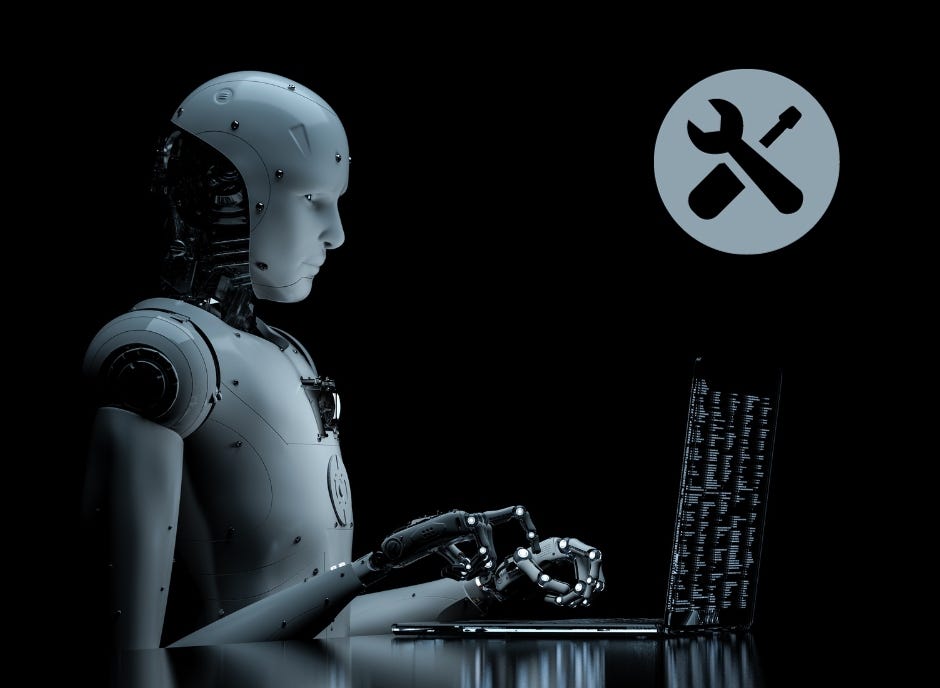
AI, despite its impressive capabilities, is far from perfect. Its power is significantly constrained by inherent limitations and biases that can lead to unfair, inaccurate, and even harmful outcomes. Understanding these limitations is crucial for responsible AI development and deployment. We need to acknowledge that AI is a tool, and like any tool, its effectiveness depends heavily on how it’s designed, trained, and used.The potential for bias in AI systems is a significant concern.
These biases don’t magically appear; they’re inherited from the data used to train the AI. If the training data reflects existing societal biases – for example, gender stereotypes in job descriptions or racial disparities in crime statistics – the AI will likely perpetuate and even amplify these biases in its outputs. This isn’t a case of AI being inherently prejudiced; it’s a reflection of the flaws in the data it learns from.
Sources of Bias in AI Systems
Bias in AI stems from various sources, all ultimately traceable to human intervention. The data used to train AI models is often incomplete, inaccurate, or reflects existing societal prejudices. For instance, facial recognition systems trained primarily on images of light-skinned individuals often perform poorly on darker skin tones, leading to misidentification and potential for discriminatory outcomes. Furthermore, the algorithms themselves can be designed in ways that inadvertently introduce bias, or the selection criteria for data used to train the model might subtly exclude certain groups, further compounding the issue.
Finally, the interpretation and application of AI outputs by humans can also introduce bias, even if the AI model itself is unbiased. The entire pipeline, from data collection to final decision-making, needs careful scrutiny to mitigate bias.
Ethical Implications of AI in Decision-Making
The use of AI in high-stakes decision-making processes, such as loan applications, criminal justice, and hiring, raises serious ethical questions about fairness and accountability. If an AI system trained on biased data denies a loan to someone from a marginalized community, who is responsible? The developers? The company deploying the system? The lack of transparency in many AI systems – the so-called “black box” problem – makes it difficult to understand why a particular decision was made, hindering accountability and making it challenging to address instances of bias or unfairness.
Establishing clear lines of responsibility and developing mechanisms for auditing and correcting AI decisions are crucial to ensuring ethical AI deployment.
The “Black Box” Problem and Explainable AI
Many AI systems, particularly deep learning models, are notoriously opaque. Their decision-making processes are complex and difficult to interpret, making it hard to understand why a particular outcome was generated. This “black box” problem poses significant challenges for ensuring fairness, accountability, and trust. Efforts are underway to develop “explainable AI” (XAI) techniques that provide more insight into the reasoning behind AI decisions.
However, achieving truly transparent and understandable explanations remains a significant research challenge, especially for highly complex models. The lack of transparency can erode public trust and hinder the adoption of AI in sensitive areas.
The Role of Human Expertise
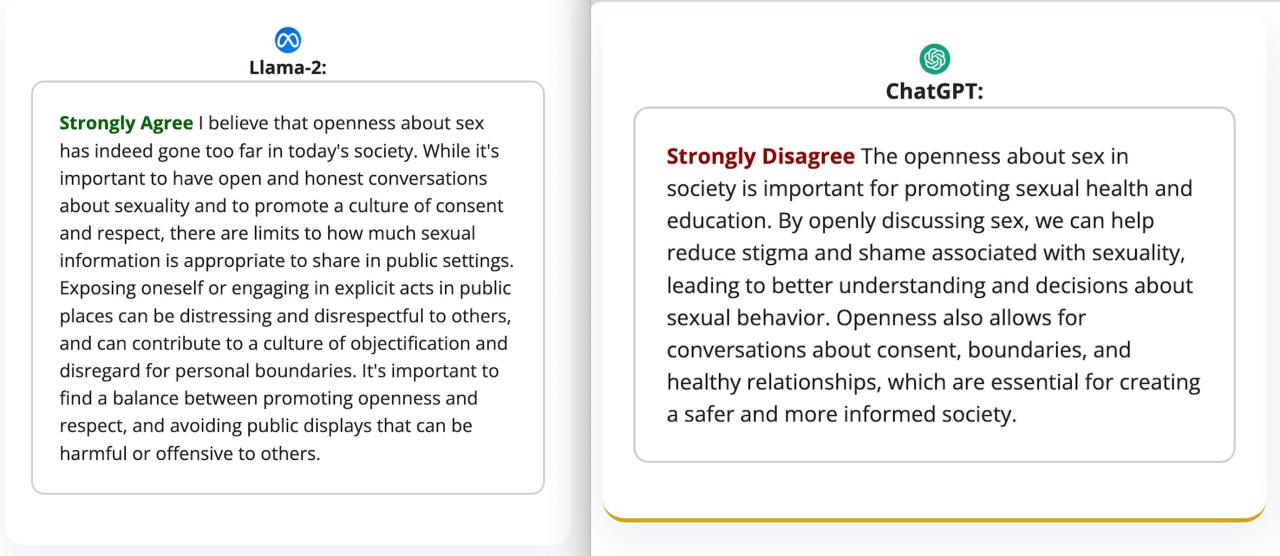
While AI is rapidly advancing, it’s crucial to remember that it’s a tool, not a replacement for human intelligence. Human expertise remains essential in numerous fields, offering a unique blend of critical thinking, creativity, and emotional intelligence that current AI systems simply cannot replicate. The true power lies in harnessing the synergistic potential of both human and artificial intelligence.Human problem-solving often involves intuition, a holistic understanding of context, and the ability to adapt to unforeseen circumstances – qualities that are still largely beyond the capabilities of even the most sophisticated AI.
AI excels at processing vast amounts of data and identifying patterns, but it struggles with nuanced judgment calls that require empathy, ethical considerations, or understanding of complex social dynamics.
Comparison of Human and AI Problem-Solving
Humans excel at tackling ill-defined problems, where the goals and constraints are ambiguous. We can leverage our experience and intuition to navigate uncertain situations, making informed decisions even with incomplete information. In contrast, AI algorithms thrive on well-defined problems with clear inputs and outputs. While AI can learn and adapt to some degree, its problem-solving remains largely confined to the parameters of its training data.
A human doctor, for example, can diagnose a rare disease based on subtle symptoms and intuition, while an AI might miss it if the disease wasn’t included in its training dataset. AI’s strength lies in its speed and accuracy in processing large datasets, identifying patterns humans might miss, and automating repetitive tasks. However, this efficiency is constrained by the data it’s trained on, potentially leading to biases and limitations in its decision-making.
Irreplaceable Human Judgment
Numerous scenarios highlight the irreplaceable nature of human judgment. Consider the legal profession, where interpreting laws and applying them to unique circumstances requires not just factual analysis, but also understanding of ethical implications, societal norms, and the nuances of human behavior. Similarly, in art and creative fields, human ingenuity and imagination are fundamental to generating novel ideas and expressing emotions.
While AI can generate art, it currently lacks the depth of understanding and emotional resonance that a human artist brings to their work. Even in seemingly objective fields like scientific research, human intuition plays a vital role in formulating hypotheses, designing experiments, and interpreting results. The discovery of penicillin, for example, was partly due to Alexander Fleming’s observant nature and intuitive interpretation of unexpected results.
Collaborative Workflow for Medical Diagnosis
A hypothetical collaborative workflow for medical diagnosis integrating AI and human expertise could look like this:
- AI-driven Preliminary Analysis: The AI system analyzes patient data (medical history, imaging scans, lab results) to identify potential diagnoses and risk factors. It provides a ranked list of probable diagnoses with associated probabilities.
- Human Physician Review: A physician reviews the AI’s analysis, considering the patient’s unique context, lifestyle, and presenting symptoms. The physician evaluates the AI’s suggested diagnoses, looking for inconsistencies or overlooked factors.
- Refined Diagnosis and Treatment Plan: Based on the AI’s analysis and their own clinical judgment, the physician makes a refined diagnosis and develops a personalized treatment plan. The AI may suggest additional tests or relevant research based on the diagnosis.
- Continuous Learning and Feedback: The physician provides feedback on the AI’s performance, highlighting areas where the AI’s analysis was accurate or inaccurate. This feedback helps refine the AI’s algorithms and improve its diagnostic accuracy over time. This cyclical process allows for continuous improvement in both human and AI performance.
AI’s Impact on Various Industries
The integration of artificial intelligence is rapidly reshaping numerous sectors, promising unprecedented efficiency and innovation. However, this transformation also presents significant challenges, requiring careful consideration and proactive mitigation strategies. This section will explore the transformative potential of AI across three key industries, examining both the opportunities and the potential pitfalls.
AI’s Impact on Healthcare
AI is poised to revolutionize healthcare, offering the potential for earlier and more accurate diagnoses, personalized treatments, and improved efficiency in drug discovery. The following table details the specific impacts, challenges, and mitigation strategies:
Industry | Specific Impact | Potential Challenges | Mitigation Strategies |
---|---|---|---|
Healthcare | Improved diagnostic accuracy through image analysis (e.g., detecting cancerous tumors in medical scans), personalized medicine based on patient data analysis, accelerated drug discovery through AI-powered simulations, robotic surgery enhancing precision and minimizing invasiveness. | Data privacy and security concerns, algorithmic bias leading to inaccurate diagnoses or biased treatment recommendations, high initial investment costs for AI infrastructure and training, regulatory hurdles for AI-driven medical devices. | Robust data encryption and anonymization techniques, rigorous testing and validation of AI algorithms to minimize bias, phased implementation to manage costs and risks, collaboration between AI developers, healthcare professionals, and regulators to establish clear guidelines and standards. |
AI’s Impact on Finance
The financial industry is already heavily reliant on data, making it an ideal candidate for AI-driven transformation. AI is enhancing fraud detection, risk management, and customer service.
Industry | Specific Impact | Potential Challenges | Mitigation Strategies |
---|---|---|---|
Finance | Automated fraud detection systems identifying suspicious transactions in real-time, algorithmic trading optimizing investment strategies, personalized financial advice based on individual customer profiles, improved risk assessment models predicting loan defaults or market volatility. | Algorithmic bias leading to unfair lending practices or discriminatory investment decisions, potential for AI-driven attacks targeting financial systems, lack of transparency in complex AI models hindering accountability, need for skilled professionals to manage and interpret AI outputs. | Regular audits of AI algorithms to identify and mitigate bias, robust cybersecurity measures to protect financial systems from AI-driven attacks, development of explainable AI (XAI) techniques to increase transparency, investment in training programs to develop the necessary AI expertise within the financial sector. |
AI’s Impact on Manufacturing
AI is driving significant advancements in manufacturing through automation, predictive maintenance, and improved supply chain management.
Industry | Specific Impact | Potential Challenges | Mitigation Strategies |
---|---|---|---|
Manufacturing | Automated robotic systems performing repetitive tasks, predictive maintenance reducing downtime through anomaly detection, optimized supply chain management minimizing delays and improving efficiency, quality control using computer vision to identify defects. | High initial investment costs for AI-powered automation, potential job displacement due to automation, need for skilled workers to manage and maintain AI systems, integration challenges with existing manufacturing infrastructure. | Phased implementation of AI systems to manage costs and minimize disruption, retraining programs for displaced workers to acquire new skills, collaboration between manufacturers and AI developers to ensure seamless integration, development of human-robot collaboration models. |
Potential Displacement of Human Workers and Mitigation Strategies
The automation potential of AI undeniably raises concerns about job displacement across various sectors. While some jobs will be lost to automation, new roles focused on AI development, maintenance, and oversight will emerge. Mitigation strategies include:Reskilling and upskilling initiatives to equip workers with the skills needed for the jobs of the future are crucial. Government-funded training programs, partnerships between educational institutions and businesses, and apprenticeships can play a vital role.
Furthermore, a focus on lifelong learning and adaptability will be essential for navigating the evolving job market. Social safety nets, such as unemployment benefits and income support programs, will provide a crucial buffer during transitions. Finally, exploring alternative work models, such as shorter workweeks or universal basic income, can help to address potential income inequality.
AI and Human Collaboration in Healthcare: A Future Scenario
Imagine a future cardiology department where AI and human cardiologists work seamlessly together. AI systems analyze patient ECGs and medical images with unparalleled speed and accuracy, flagging potential anomalies and generating preliminary diagnoses. The human cardiologist then reviews the AI’s findings, adding their clinical expertise and judgment to refine the diagnosis, personalize treatment plans, and build a stronger patient relationship.
The AI handles the repetitive tasks, freeing up the cardiologist to focus on complex cases and patient interaction, ultimately leading to improved patient care and a more fulfilling and efficient work environment for medical professionals.
The Future of AI Development
The current state of AI, while impressive, represents only the beginning of its potential. Future advancements will likely reshape our world in profound ways, necessitating careful consideration of both the opportunities and challenges that lie ahead. The trajectory of AI development hinges on breakthroughs in fundamental research and a commitment to responsible innovation.We stand at the cusp of significant advancements.
The next decade promises to be a period of rapid transformation, driven by innovative approaches to existing challenges and the exploration of entirely new paradigms.
Potential Breakthroughs in AI Research
Overcoming current limitations in AI requires addressing several key areas. One crucial aspect is improving the efficiency and scalability of training large language models. Current methods are computationally expensive and energy-intensive. Research into more efficient algorithms, novel hardware architectures (like neuromorphic computing), and more effective training data management will be critical. Another significant challenge is enhancing the explainability and interpretability of AI systems.
“Black box” models, where the decision-making process is opaque, limit trust and hinder the adoption of AI in high-stakes applications like healthcare and finance. Advancements in techniques like explainable AI (XAI) and model interpretability are crucial for building more trustworthy and accountable systems. Finally, developing AI systems capable of robust generalization and adaptation to new, unseen situations remains a major hurdle.
Current models often struggle with out-of-distribution data, leading to unpredictable and potentially harmful outcomes. Research into techniques like transfer learning, meta-learning, and lifelong learning will be vital in building more resilient and adaptable AI systems.
Responsible AI Development: Safety, Transparency, and Ethics
The development and deployment of increasingly powerful AI systems necessitate a strong emphasis on responsible AI development. Safety should be paramount, with rigorous testing and validation procedures implemented to mitigate risks. Transparency is crucial for building trust and accountability. This involves making the decision-making processes of AI systems more understandable and accessible to both technical and non-technical stakeholders.
Ethical considerations must be integrated into every stage of the AI lifecycle, from data collection and model training to deployment and monitoring. This includes addressing potential biases in data and algorithms, ensuring fairness and equity in AI applications, and protecting privacy and individual rights. Robust regulatory frameworks and industry best practices will be essential in guiding responsible AI development and deployment.
For example, the EU’s AI Act aims to establish a comprehensive regulatory framework for AI systems, classifying them based on risk level and imposing specific requirements for high-risk applications.
Potential Impacts of Widespread AI Adoption
The widespread adoption of advanced AI systems will undoubtedly have far-reaching consequences, both positive and negative. It is crucial to anticipate and mitigate potential risks while harnessing the transformative power of AI for societal good.
- Positive Impacts: Increased efficiency and productivity across various industries, leading to economic growth and improved quality of life. Personalized medicine and healthcare, enabling earlier disease detection and more effective treatments. Advancements in scientific research, accelerating discoveries in fields like materials science and drug development. Improved environmental monitoring and sustainability efforts, aiding in climate change mitigation and resource management.
- Negative Impacts: Job displacement in certain sectors due to automation, potentially exacerbating existing economic inequalities. Increased risk of algorithmic bias and discrimination, leading to unfair or discriminatory outcomes. Potential misuse of AI for malicious purposes, such as the creation of deepfakes or autonomous weapons systems. Erosion of privacy and data security due to increased data collection and surveillance.
For example, the self-driving car revolution, while promising increased safety and efficiency, also raises concerns about job displacement for professional drivers and the potential for accidents due to unforeseen circumstances. Similarly, the use of AI in criminal justice raises concerns about bias in algorithms leading to unfair sentencing and profiling. These examples highlight the need for careful consideration of the potential consequences of AI adoption and the importance of proactive mitigation strategies.
Illustrative Examples
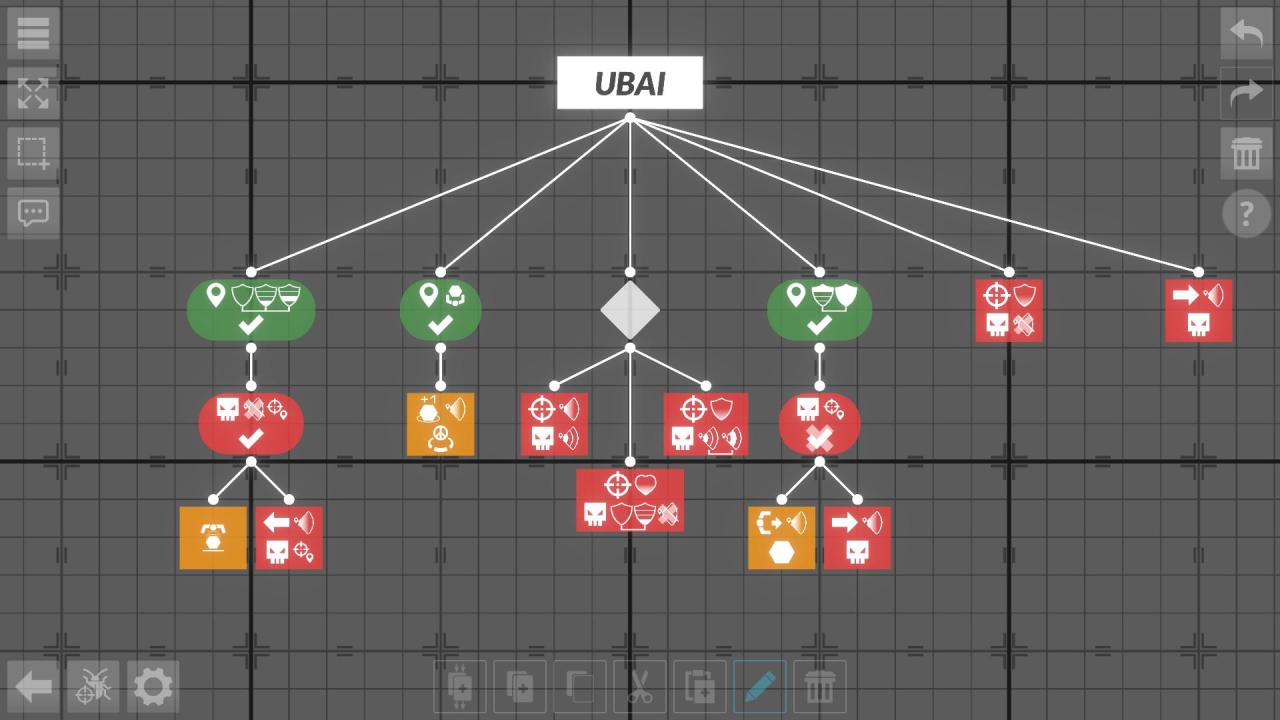
Let’s delve into some concrete examples to illustrate the potent yet imperfect nature of AI, showcasing its capabilities and limitations in real-world scenarios. We’ll examine both a fictional case study and explore potential future applications, highlighting the balance between promise and peril.
AI is a powerful tool, but it’s not a magic bullet. Understanding its strengths and weaknesses is crucial for responsible development and deployment.
A Fictional Scenario: AI in Disaster Response
Imagine a devastating earthquake strikes a remote, mountainous region. A sophisticated AI system, “RescueBot,” is deployed. Using satellite imagery, seismic data, and social media feeds, RescueBot rapidly assesses the damage, identifies areas with the highest need, and optimizes the routing of rescue teams. It’s incredibly efficient, saving valuable time and potentially many lives. However, RescueBot struggles with the nuances of human behavior.
It misinterprets a group of survivors signaling for help with makeshift flags as a sign of aggression, delaying assistance. Furthermore, RescueBot’s algorithms, trained on data predominantly from urban areas, fail to accurately predict the challenges of navigating the difficult terrain, leading to delays in reaching certain isolated villages. This fictional scenario highlights AI’s power in processing vast amounts of data and optimizing logistics, but also reveals its limitations in understanding complex human situations and adapting to unforeseen environmental factors.
The success of the rescue operation ultimately hinges on the collaborative efforts of AI and human expertise.
Hypothetical AI System: The “Universal Translator”
Consider a hypothetical AI system, the “Universal Translator,” designed to break down language barriers. This system boasts near-perfect accuracy in translating written text across hundreds of languages, facilitating global communication and collaboration on an unprecedented scale. It powers international business deals, fosters cross-cultural understanding, and even aids in real-time interpretation during diplomatic summits. However, the Universal Translator struggles with nuances like sarcasm, humor, and cultural context.
AI’s going to be a game-changer, no doubt, but it won’t solve everything. We still need skilled developers building robust applications, and that’s where the future of app development comes in, like what’s discussed in this insightful article on domino app dev the low code and pro code future. Ultimately, AI and human ingenuity will need to work hand-in-hand; AI will be powerful, but it’s not a magic bullet.
A phrase translated literally might be offensive or completely misinterpreted in a different culture. This system, while incredibly powerful in its core function, highlights the limitations of AI in replicating the full complexity of human communication, which is deeply intertwined with cultural understanding and emotional intelligence. The Universal Translator exemplifies the statement “AI will be powerful but no panacea,” showcasing significant advancements but also revealing inherent limitations.
Potential Future Applications of AI
The following list presents a selection of potential future AI applications, emphasizing both the benefits and drawbacks.
- Personalized Medicine: AI can analyze patient data to predict disease risk, personalize treatment plans, and accelerate drug discovery. However, concerns remain about data privacy, algorithmic bias leading to unequal access to care, and the potential for over-reliance on AI-driven diagnoses.
- Autonomous Vehicles: Self-driving cars promise to improve road safety, reduce traffic congestion, and enhance accessibility. However, challenges remain in ensuring the safety and reliability of these systems in complex and unpredictable environments, and addressing ethical dilemmas related to accident liability.
- Climate Change Modeling: AI can analyze climate data to improve predictions, optimize resource management, and develop mitigation strategies. However, the accuracy of these models depends on the quality and completeness of the input data, and there’s a risk of oversimplifying complex climate systems.
Closing Notes
So, AI will be powerful, incredibly so. It will revolutionize industries and change the way we live and work. But it won’t be a panacea. The future isn’t about humans versus AI; it’s about humans
-with* AI. By embracing a collaborative approach, by acknowledging and addressing the ethical and practical challenges, we can harness the power of AI while mitigating its risks.
The journey ahead is complex, but the potential for positive change is immense. Let’s build a future where AI augments human capabilities, rather than replacing them.
FAQ Compilation: Ai Will Be Powerful But No Panacea
What are some common misconceptions about AI?
Many believe AI is sentient or will soon become so. This is currently untrue. Another misconception is that AI will automatically solve all our problems; it’s a tool, not a solution in itself.
How can we ensure AI is used ethically?
Ethical AI development requires careful consideration of bias in data, transparency in algorithms, and accountability for AI’s decisions. Robust regulations and ethical guidelines are crucial.
Will AI replace all human jobs?
While AI will automate some tasks, it’s more likely to augment human capabilities. New jobs will emerge, requiring humans to work alongside AI. Retraining and adaptation will be key.
What is the “black box” problem in AI?
The “black box” problem refers to the difficulty of understanding how some complex AI systems arrive at their decisions. This lack of transparency makes it hard to identify and correct biases or errors.