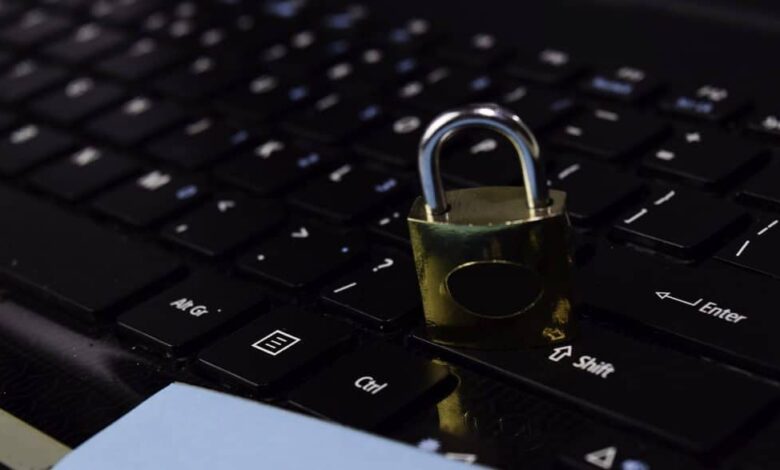
Artificial Intelligence Blocks Ryuk Ransomware Invasion
Artificial Intelligence Blocks Ryuk Ransomware Invasion: It sounds like something out of a sci-fi thriller, right? But the reality is, AI is becoming a crucial weapon in our fight against increasingly sophisticated cyber threats like the notorious Ryuk ransomware. This isn’t just about detecting malware; it’s about proactively preventing attacks, responding in real-time, and even aiding in recovery.
We’re diving deep into how AI is changing the cybersecurity landscape and making our digital lives safer, one ransomware attack at a time.
This post will explore the different ways AI is being used to combat Ryuk, from identifying malicious code patterns to predicting and preventing attacks before they even happen. We’ll look at both the successes and limitations of current AI technologies, and consider the ethical implications of using AI in this crucial area. Get ready for a fascinating look at the future of cybersecurity!
AI’s Role in Cybersecurity
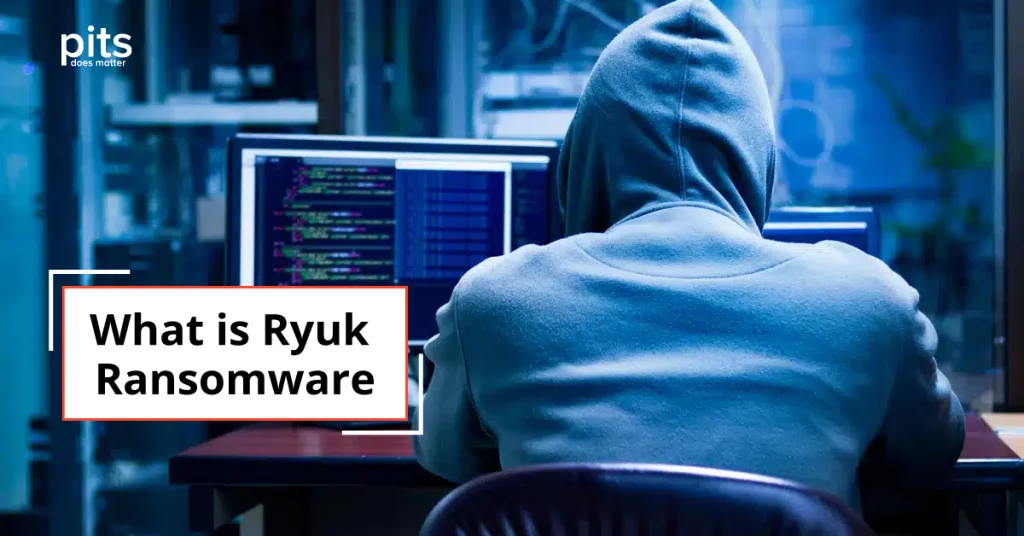
The rise of sophisticated ransomware like Ryuk demands equally sophisticated defenses, and artificial intelligence is stepping up to the challenge. AI offers a proactive and reactive approach to cybersecurity, moving beyond traditional signature-based detection to a more intelligent and adaptable system capable of identifying and neutralizing threats in real-time. This proactive approach is crucial in combating the ever-evolving tactics employed by ransomware developers.
AI-Powered Ransomware Prevention Techniques
AI can significantly enhance ransomware prevention through several key mechanisms. Machine learning algorithms can analyze network traffic patterns, identifying anomalies that might indicate malicious activity. This includes unusual file access patterns, unexpected outbound connections, or spikes in encrypted data transfer. Furthermore, AI can analyze file behavior, identifying suspicious code execution or attempts to encrypt critical data before they cause widespread damage.
Behavioral analysis is particularly effective against polymorphic ransomware, which constantly changes its code to evade traditional signature-based detection. Finally, AI can strengthen security posture by analyzing system configurations and identifying vulnerabilities that could be exploited by ransomware attacks. Regular vulnerability scanning and patching, guided by AI’s insights, are crucial for preventing attacks.
Real-Time Ryuk Ransomware Detection and Response
AI’s real-time capabilities are vital in combating Ryuk. By continuously monitoring network activity and system behavior, AI can detect the telltale signs of a Ryuk infection, such as unusual processes, encrypted files, or communication with known command-and-control servers. Upon detection, AI can trigger automated responses, such as isolating infected systems to prevent further spread, blocking malicious network connections, and initiating immediate data backups to mitigate data loss.
The speed and automation provided by AI are crucial in containing a Ryuk attack before it can encrypt a significant amount of data. Furthermore, AI can analyze the ransomware’s behavior to understand its attack vectors and develop more effective countermeasures.
Comparison of AI-Based Security Solutions
Several AI-based security solutions are available to combat ransomware, each with its strengths and weaknesses. Some solutions focus on endpoint detection and response (EDR), utilizing AI to monitor individual devices for malicious activity. Others focus on network security, analyzing network traffic to identify suspicious patterns. A hybrid approach, combining EDR and network security, is often the most effective.
The choice of solution depends on the specific needs and resources of the organization. For example, a large enterprise might benefit from a comprehensive solution encompassing multiple layers of AI-powered security, while a smaller organization might opt for a more focused solution targeting specific vulnerabilities. Crucially, the efficacy of these solutions hinges on the quality of the underlying AI models and the quantity and quality of training data used to develop them.
Poorly trained models can lead to false positives or missed detections.
Hypothetical Scenario: AI Blocking a Ryuk Ransomware Invasion
Imagine a scenario where a sophisticated Ryuk ransomware attack targets a large financial institution. The attack begins with a phishing email containing a malicious attachment. However, the institution’s AI-powered security system immediately flags the email as suspicious due to unusual language patterns and sender information, blocking it before it reaches any employees. Simultaneously, the AI system monitors network traffic and detects an attempt to exploit a known vulnerability on a server.
The AI system automatically patches the vulnerability and isolates the affected server, preventing the ransomware from spreading. Finally, the AI system continuously monitors the network for any further attempts and generates a detailed report on the attempted attack, providing valuable insights for future security improvements. The entire process happens within minutes, effectively neutralizing the threat before significant damage occurs.
This illustrates the potential of AI to provide comprehensive and proactive protection against advanced ransomware threats.
Ryuk Ransomware Tactics and AI Countermeasures: Artificial Intelligence Blocks Ryuk Ransomware Invasion
Ryuk ransomware, a particularly devastating strain, targets high-value organizations, demanding significant ransoms for data decryption. Understanding its tactics and developing effective AI-based countermeasures is crucial for bolstering cybersecurity defenses. This section delves into Ryuk’s infection vectors, explores AI techniques for detection, addresses challenges in combating sophisticated variants, and proposes a proactive AI-powered system for neutralization.
Ryuk Ransomware Infection Vectors
Ryuk primarily spreads through sophisticated, multi-stage attacks. Initial access is often gained through phishing emails containing malicious attachments or links leading to compromised websites. These initial compromises then leverage other malware, often Trickbot or Emotet, to establish persistence and lateral movement within the victim’s network. Once established, Ryuk encrypts critical data, rendering systems unusable and demanding a ransom for decryption.
The attackers carefully select their targets, focusing on organizations likely to pay due to the potential disruption and loss of sensitive data. This targeted approach makes Ryuk significantly more dangerous than many other ransomware variants.
AI Techniques for Ryuk Malware Detection
Several AI techniques can effectively detect malicious code associated with Ryuk. Machine learning models, trained on vast datasets of benign and malicious code samples, can identify patterns and anomalies indicative of ransomware behavior. These models can analyze file characteristics, network traffic, and system processes to flag suspicious activities. For example, anomaly detection algorithms can identify unusual file access patterns or encrypted network communications consistent with Ryuk’s encryption process.
Natural language processing (NLP) can also analyze the command and control (C&C) communications of Ryuk, identifying suspicious s or patterns within the encrypted messages. These techniques provide a layered approach to detection, increasing the overall effectiveness of security systems.
Challenges in AI-Based Ryuk Detection
Despite the potential of AI, detecting sophisticated ransomware like Ryuk presents significant challenges. The attackers constantly evolve their techniques, using polymorphism and obfuscation to evade detection. This necessitates continuous model retraining and adaptation to stay ahead of emerging threats. Additionally, the limited availability of labeled Ryuk samples can hinder the training of accurate and robust AI models.
Furthermore, the attackers’ focus on highly targeted attacks means that the number of observable instances might be relatively low, making it harder to train robust detection models. Finally, the need to balance detection accuracy with minimizing false positives remains a significant hurdle.
AI-Powered System for Proactive Ryuk Neutralization
To proactively identify and neutralize Ryuk’s initial infection attempts, a multi-layered AI-powered system is necessary. The following table Artikels a proposed design:
Component | Function | AI Technique | Expected Outcome |
---|---|---|---|
Email Security Gateway | Scans incoming emails for malicious attachments and links. | Machine learning-based malware detection, sandboxing, and URL analysis. | Blocks malicious emails before they reach end-users. |
Endpoint Detection and Response (EDR) | Monitors system activity for suspicious processes and file access patterns. | Anomaly detection, behavioral analysis, and machine learning-based threat classification. | Detects and isolates infected systems before widespread encryption occurs. |
Network Traffic Analysis | Analyzes network communication for suspicious patterns, such as unusual outbound connections. | Machine learning-based network anomaly detection and intrusion detection systems (IDS). | Identifies and blocks malicious communication attempts. |
Threat Intelligence Platform | Provides real-time threat intelligence and updates to the AI models. | Data aggregation, analysis, and correlation of threat information from various sources. | Improves the accuracy and effectiveness of detection and response mechanisms. |
The Impact of AI on Ransomware Mitigation
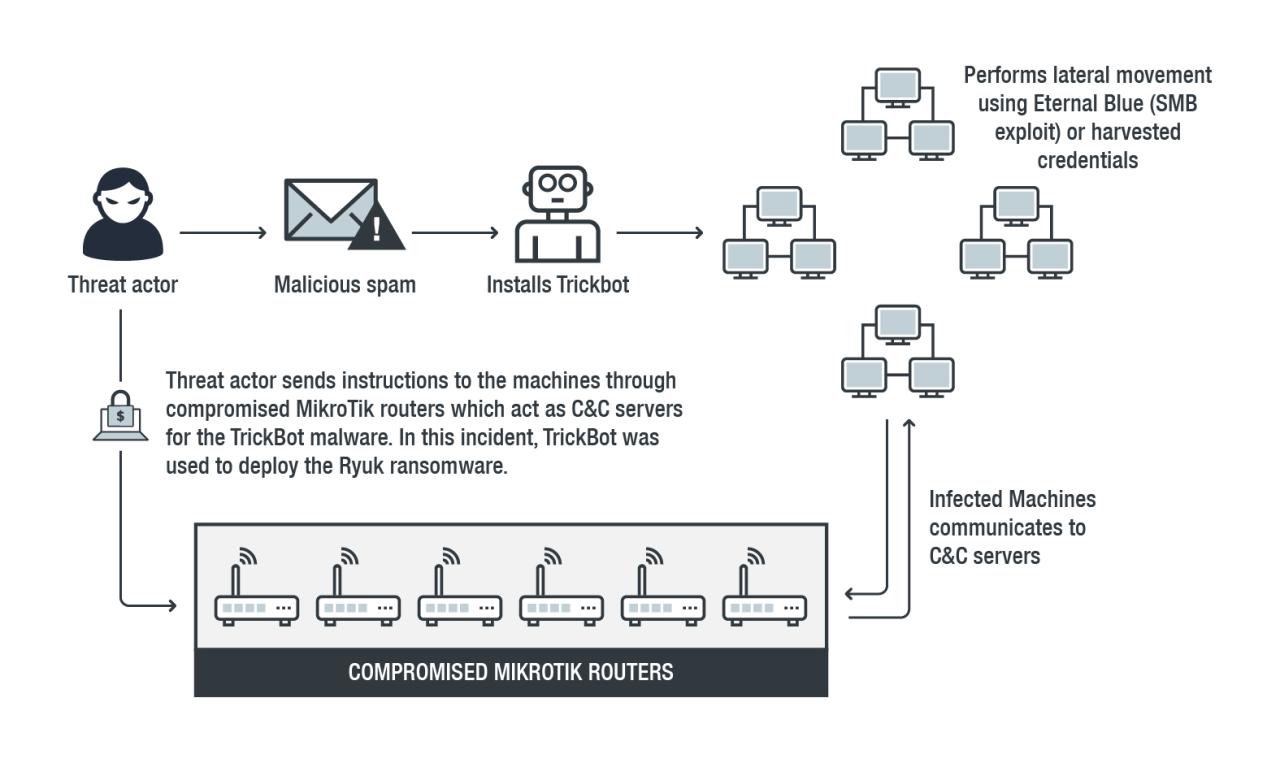
The rise of ransomware attacks has created a critical need for advanced security solutions, and Artificial Intelligence (AI) is emerging as a powerful weapon in this fight. AI’s ability to analyze vast amounts of data, identify patterns, and adapt to evolving threats makes it uniquely suited to combat the ever-changing tactics of ransomware operators. Its impact spans prevention, detection, and even recovery, significantly reducing the devastating effects of these attacks.AI improves the speed and accuracy of ransomware detection compared to traditional signature-based methods by leveraging machine learning algorithms.
Traditional methods rely on identifying known malware signatures, leaving them vulnerable to zero-day attacks and variations of existing ransomware. In contrast, AI can analyze network traffic, file behavior, and system logs to identify suspicious activities indicative of a ransomware attack, even if the specific ransomware variant is unknown. This proactive approach allows for quicker detection and response, minimizing the damage caused.
AI-Driven Ransomware Prevention Examples
Several organizations have successfully deployed AI-powered solutions to prevent ransomware attacks. For example, a major financial institution implemented an AI-based security system that analyzed network traffic in real-time, identifying unusual file access patterns and blocking malicious connections before they could compromise critical systems. Another successful case involved a healthcare provider using AI to detect and block phishing emails, a common ransomware delivery method, with a significantly higher success rate than traditional email filtering systems.
These examples highlight the effectiveness of AI in proactively preventing ransomware infections.
Speed and Accuracy of AI-Based Ransomware Detection
AI significantly enhances the speed and accuracy of ransomware detection. Traditional methods, often relying on signature-based detection, lag behind the rapid evolution of ransomware techniques. AI, however, can analyze behavioral patterns, network anomalies, and system changes to identify malicious activity far more quickly. For instance, AI can detect unusual encryption activity or the rapid proliferation of files with suspicious extensions, raising an alert far sooner than signature-based systems would.
Furthermore, AI’s ability to learn and adapt to new ransomware variants ensures ongoing accuracy, reducing false positives and improving the overall effectiveness of detection efforts. This speed and accuracy translate to a faster response time, minimizing the potential damage caused by ransomware.
Economic Benefits of AI-Based Ransomware Mitigation, Artificial intelligence blocks ryuk ransomware invasion
Implementing AI-based security solutions offers substantial economic benefits by reducing ransomware-related losses. The costs associated with ransomware attacks are staggering, including data recovery, system restoration, legal fees, and reputational damage. AI-driven prevention and detection minimize these costs by preventing infections or containing them quickly. Studies have shown that organizations utilizing AI security solutions experience significantly lower downtime and data loss compared to those relying solely on traditional methods.
The return on investment (ROI) for AI-based security is often substantial, as the cost of implementing these solutions is significantly outweighed by the savings from averted ransomware attacks. For instance, a company might invest $100,000 in an AI security system, but avoid a potential $1 million loss from a ransomware attack, representing a substantial ROI.
AI’s Role in Post-Ransomware Attack Recovery
Even with robust prevention measures, ransomware attacks can still occur. In such cases, AI can significantly aid in the recovery process. AI can analyze encrypted files to identify patterns and potentially assist in decryption efforts. Moreover, AI can automate the process of restoring systems from backups, ensuring a faster and more efficient recovery. AI can also help analyze the attack itself, identifying the attack vector and vulnerabilities exploited, to prevent future incidents.
By accelerating recovery and providing insights into the attack’s root cause, AI minimizes the overall disruption and financial impact of a successful ransomware attack.
Limitations and Future Directions of AI in Ransomware Defense
AI is rapidly transforming cybersecurity, but its application in combating sophisticated ransomware like Ryuk still faces significant hurdles. While AI offers promising solutions for detection and prevention, its effectiveness is limited by the ever-evolving nature of ransomware and the inherent complexities of data analysis. This section explores these limitations, the ethical considerations surrounding AI’s deployment, and the potential future advancements that could revolutionize ransomware defense.
AI’s role in thwarting the Ryuk ransomware is huge; it’s becoming a crucial part of cybersecurity. This rapid advancement in AI security highlights the need for equally agile development processes, which is why I’ve been diving deep into the world of domino app dev, the low-code and pro-code future , to see how it can help build more resilient systems.
Ultimately, faster, more secure app development is key to staying ahead of threats like Ryuk.
Limitations of Current AI in Ransomware Countermeasures
Current AI models, while effective in identifying known ransomware signatures and patterns, struggle with the rapid mutation and polymorphic nature of advanced ransomware like Ryuk. These threats frequently employ evasion techniques, including obfuscation and encryption, to bypass traditional signature-based detection systems and even some AI-based solutions. Furthermore, the reliance on labeled datasets for training AI models poses a challenge.
Obtaining sufficient high-quality, labeled data representing diverse ransomware variants and attack vectors is difficult and time-consuming. This data scarcity can lead to biased models that are less effective against novel attacks. Finally, the computational resources required for training and deploying complex AI models can be substantial, posing a barrier for smaller organizations.
Ethical Considerations of AI in Cybersecurity
The use of AI in cybersecurity, particularly in ransomware prevention, raises several ethical concerns. The potential for bias in AI algorithms, leading to discriminatory outcomes, is a significant issue. For example, a model trained primarily on data from one region or type of organization might perform poorly when confronted with ransomware attacks originating from different contexts. Furthermore, the deployment of AI-powered systems for automated threat response necessitates careful consideration of potential unintended consequences.
Automated actions, while efficient, could inadvertently lead to system disruptions or data loss if not properly implemented and monitored. The issue of transparency and explainability in AI-based systems is also crucial. Understanding how an AI system arrives at a particular decision is essential for building trust and ensuring accountability.
Potential Future Developments in AI for Enhanced Ransomware Defense
Future advancements in AI hold significant potential for strengthening ransomware defenses. The development of more robust and adaptable AI models, capable of handling zero-day attacks and polymorphic malware, is crucial. This could involve the use of advanced techniques like federated learning, allowing multiple organizations to collaboratively train AI models without sharing sensitive data. Improvements in explainable AI (XAI) will also be critical for building trust and ensuring the responsible deployment of AI-based security systems.
Enhanced threat intelligence sharing, facilitated by AI, could significantly improve the ability to proactively identify and mitigate emerging ransomware threats. Finally, the integration of AI with other cybersecurity technologies, such as blockchain and advanced threat hunting platforms, will further enhance overall security posture.
Potential Research Areas for Improving AI-Based Ransomware Protection
The effective application of AI in ransomware defense requires continued research and development. Key areas for future research include:
- Developing AI models capable of detecting and classifying ransomware based on behavioral analysis rather than solely relying on signatures.
- Improving the efficiency and scalability of AI-based ransomware detection systems to handle large volumes of data in real-time.
- Exploring the use of reinforcement learning to train AI agents that can proactively adapt to new ransomware tactics and techniques.
- Developing robust methods for evaluating and mitigating bias in AI-based ransomware detection models.
- Investigating the application of explainable AI (XAI) to enhance transparency and accountability in AI-powered security systems.
- Researching the integration of AI with other cybersecurity technologies for a more holistic approach to ransomware defense.
Illustrative Example: AI Blocking Ryuk
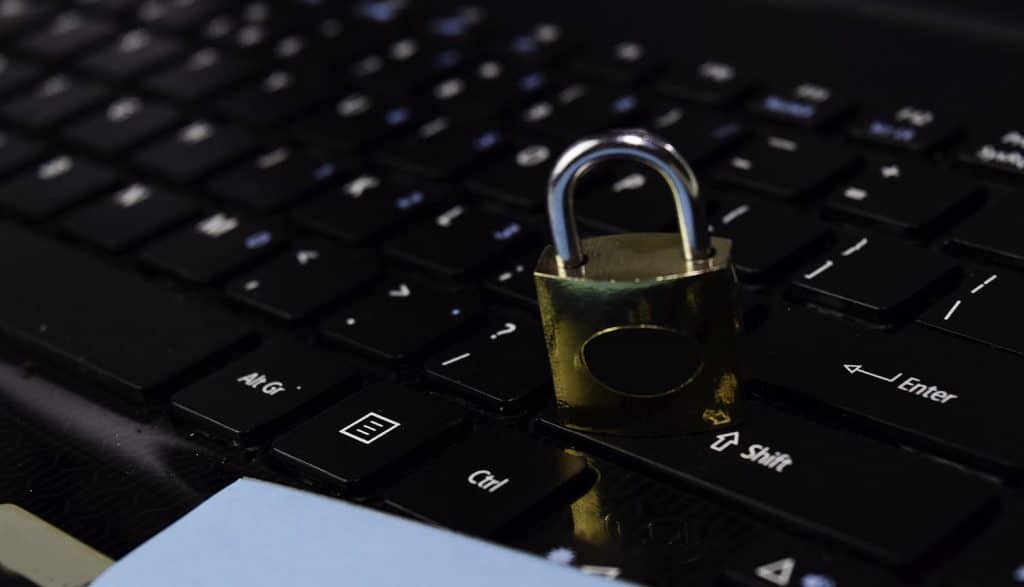
Imagine a visual representation charting the lifecycle of a Ryuk ransomware attack, contrasted with the points of AI intervention. The visualization would use a timeline format, showing distinct stages of the attack progressing from left to right, with AI’s countermeasures depicted as shields or blockades at each stage.The initial stage depicts the Ryuk ransomware attempting to infiltrate the network.
This is represented visually by a swarm of dark, shadowy figures (representing malicious code) attempting to breach a brightly lit, well-protected fortress (representing the network). Simultaneously, a graph showing network traffic spikes dramatically as the malware attempts to connect. This is where AI’s network traffic analysis comes into play. The AI system, depicted as a vigilant security guard, analyzes this traffic in real-time, identifying unusual patterns and high volumes of data flow originating from suspicious sources.
The AI’s analysis flags these anomalies, immediately triggering alerts and initiating countermeasures, preventing the malware from establishing a foothold. The visual representation shows the shadowy figures being repelled by a shimmering energy field generated by the AI.
Network Traffic Analysis and Anomaly Detection
AI’s role in preventing the initial infiltration relies heavily on its ability to analyze network traffic. The visualization shows a heatmap representing network traffic, with anomalous activity highlighted in bright red. The AI’s machine learning algorithms identify deviations from established baselines, such as unusual connection attempts, unexpected data volumes, and communication with known malicious IP addresses. This real-time analysis allows for immediate blocking of suspicious connections, preventing the ransomware from spreading laterally within the network.
The graphic would depict the AI system actively rerouting or blocking these malicious connections, represented by the shadowy figures being redirected away from the fortress. The successful blocking is shown by the heatmap calming down and returning to normal activity levels.
Behavioral Anomaly Detection and File System Monitoring
Once the initial infiltration attempt is thwarted, the focus shifts to preventing further malicious activities. The next stage of the visualization depicts the ransomware attempting to encrypt files. This is illustrated by small, dark tendrils reaching out from the already repelled figures, attempting to touch and corrupt the data represented by bright, colorful blocks. This is where AI’s behavioral anomaly detection and file system monitoring become crucial.
The AI, depicted as a watchful eye, constantly monitors file system activity, looking for unusual patterns such as mass file encryption or deletion attempts. The AI’s algorithms detect these suspicious behaviors and immediately trigger alerts. The visualization shows the AI system actively blocking these malicious actions, represented by a protective shield around the data blocks, preventing the tendrils from reaching them.
The AI also uses its analysis to identify and isolate any already affected files, limiting the extent of the damage. The graphic shows the tendrils being repelled by the shield, with the data blocks remaining safe and unharmed.
AI-Driven Response and Remediation
The final stage illustrates the AI’s role in responding to and remediating any successful infections. Even with robust preventative measures, some malware might penetrate the defenses. In this scenario, the visualization shows a few dark figures having managed to breach the outer defenses and are beginning to corrupt the data. The AI system, depicted as a swift surgical team, quickly identifies and isolates these compromised areas, preventing the spread of the infection.
The visualization showcases the AI identifying and quarantining the affected files, represented by a containment zone around the compromised data blocks, preventing further damage. The AI then initiates automated recovery procedures, using backups and data restoration techniques to recover the affected files. This is represented visually by the data blocks being repaired and restored to their original state.
The dark figures are neutralized, and the fortress remains secure.
Closure
The fight against ransomware is far from over, but the integration of artificial intelligence is proving to be a game-changer. While AI isn’t a silver bullet, its ability to analyze vast amounts of data, identify anomalies, and respond rapidly makes it an invaluable tool in our arsenal. As AI technology continues to evolve, we can expect even more sophisticated and effective defenses against threats like Ryuk.
The future of cybersecurity is intelligent, and that’s incredibly promising news for us all.
Quick FAQs
What are the ethical concerns surrounding the use of AI in cybersecurity?
Ethical concerns include potential biases in AI algorithms leading to unfair targeting, the potential for misuse of AI by malicious actors, and the need for transparency and accountability in AI-driven security decisions.
How does AI improve the speed and accuracy of ransomware detection compared to traditional methods?
AI can process massive datasets far faster than humans, identifying subtle patterns indicative of malicious activity that traditional methods might miss. This speed and accuracy significantly reduce response times and limit the damage caused by ransomware.
Can AI completely prevent all ransomware attacks?
No, AI is not a foolproof solution. Sophisticated, evolving ransomware can still find ways to bypass even the most advanced AI defenses. However, AI significantly reduces the risk and impact of successful attacks.
What are some examples of successful AI deployments in preventing ransomware?
Many companies use AI-powered endpoint detection and response (EDR) solutions to monitor system behavior, detect anomalies, and prevent malicious code execution. These solutions have proven effective in stopping ransomware before it can encrypt data.