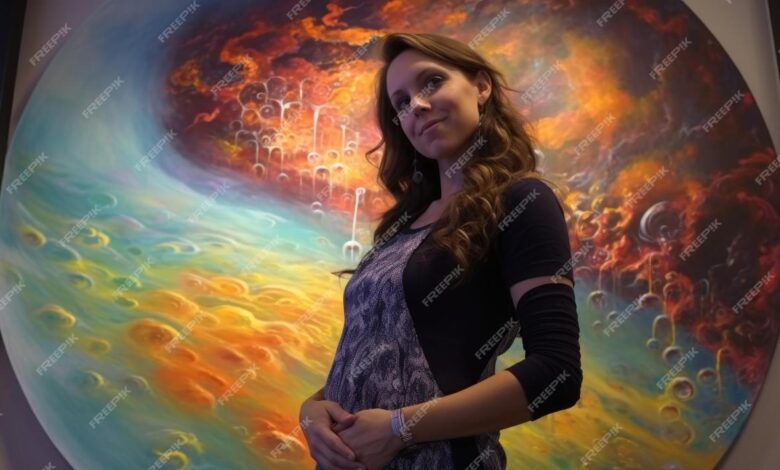
Artificial Intelligence Can Detect Mental Stress in Pregnant Women
Artificial intelligence can detect mental stress in pregnant women – a statement that might sound like science fiction, but it’s rapidly becoming a reality. Imagine a world where subtle shifts in a pregnant woman’s sleep patterns, online journaling entries, or even her heart rate variability could signal underlying stress, allowing for early intervention and support. This isn’t just about technological advancement; it’s about revolutionizing maternal mental healthcare and ensuring the well-being of both mother and child.
This post delves into the exciting possibilities and challenges of using AI to improve the lives of expectant mothers.
The increasing prevalence of perinatal mental health issues highlights a critical need for more effective screening and intervention methods. Traditional methods often fall short, failing to catch early warning signs or struggling with the stigma surrounding mental health. AI offers a potential solution, leveraging sophisticated algorithms to analyze various data points and identify patterns indicative of stress before they escalate into more serious problems.
This technology could significantly improve early detection rates, leading to timely interventions and better outcomes for both mothers and their babies.
Introduction to AI and Mental Health in Pregnancy: Artificial Intelligence Can Detect Mental Stress In Pregnant Women
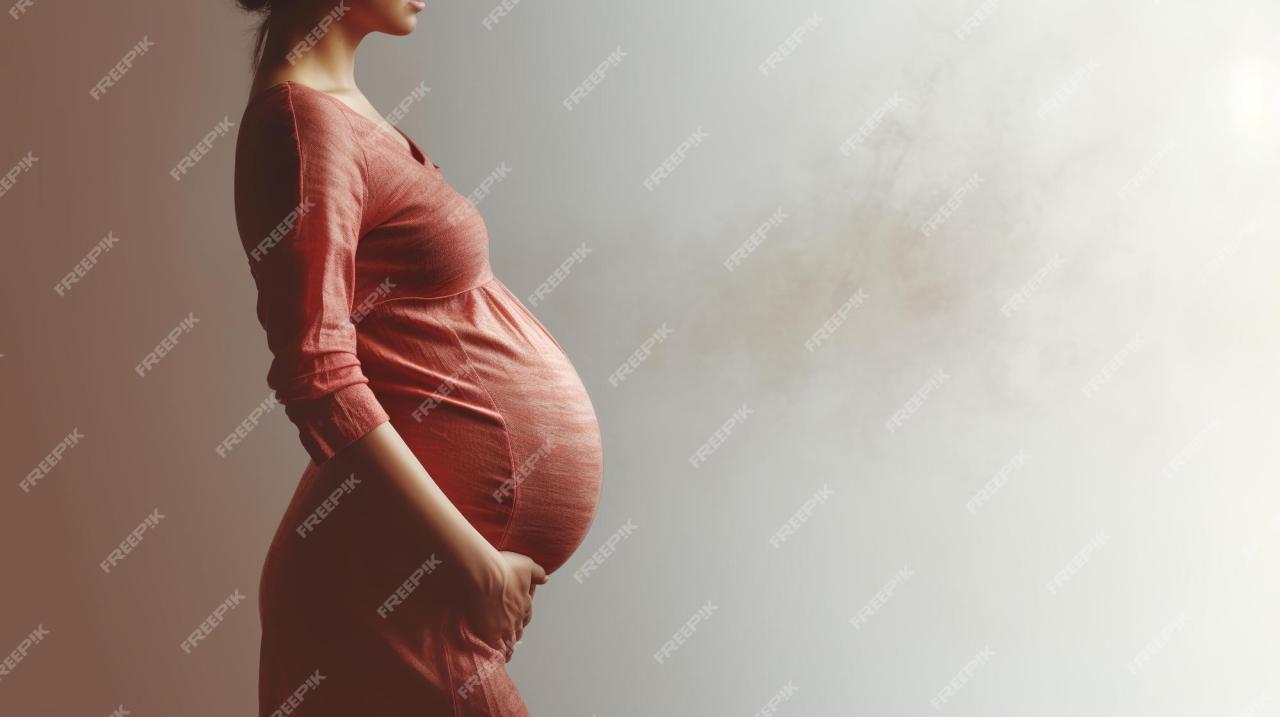
Artificial intelligence (AI) is rapidly transforming healthcare, offering the potential to improve diagnosis, treatment, and patient outcomes. AI algorithms can analyze vast datasets, identifying patterns and insights that might be missed by human observation. This capability is particularly valuable in complex areas like mental health, where subjective symptoms and diverse presentations can make accurate assessment challenging. The application of AI in pregnancy, a period of significant physiological and psychological change, holds immense promise for enhancing maternal well-being.AI’s role in healthcare extends to various applications, including image analysis for early disease detection, personalized medicine based on individual genetic profiles, and the development of more effective treatment strategies.
In mental health, AI-powered tools are being used to analyze speech patterns, text data (like social media posts), and physiological signals (such as heart rate variability) to detect and monitor mental health conditions. These advancements offer the potential for earlier intervention and more targeted support.
Prevalence of Mental Health Challenges During Pregnancy
Pregnancy is a time of significant hormonal fluctuations and life changes, increasing the vulnerability of women to mental health challenges. Perinatal mood and anxiety disorders (PMADs), including postpartum depression (PPD), anxiety, and obsessive-compulsive disorder (OCD), affect a substantial portion of pregnant and postpartum women. Estimates suggest that approximately 15-20% of pregnant women experience some form of PMAD, with rates varying depending on factors such as socioeconomic status, access to healthcare, and pre-existing mental health conditions.
The consequences of untreated PMADs can be severe, impacting both the mother’s well-being and the child’s development. For example, untreated PPD can lead to difficulties in bonding with the infant, impacting the child’s social and emotional development.
Limitations of Traditional Mental Health Screening Methods for Pregnant Women, Artificial intelligence can detect mental stress in pregnant women
Traditional methods for screening and diagnosing mental health conditions in pregnant women often rely on self-report questionnaires and clinical interviews. These methods have limitations. Self-report questionnaires can be subjective and prone to bias, particularly when individuals are experiencing significant emotional distress or are hesitant to disclose sensitive information. Clinical interviews, while valuable, can be time-consuming and require specialized training.
Furthermore, the availability of mental health professionals, particularly in underserved areas, can be limited, leading to delays in diagnosis and treatment. The inherent subjectivity of traditional methods can lead to missed diagnoses or inaccurate assessments, potentially delaying access to critical support. The lack of standardized, objective measures can also make it difficult to track the effectiveness of interventions and to compare outcomes across different populations.
Methods for AI-Based Stress Detection
Detecting mental stress in pregnant women using AI involves sophisticated analysis of various data sources. This process leverages the power of machine learning to identify patterns indicative of stress that might be missed by traditional methods. The key lies in the diverse approaches used to collect and interpret data, ranging from physiological signals to the nuances of language.
AI Techniques for Analyzing Physiological Data
Physiological data provides a rich source of information about a person’s internal state. AI algorithms can analyze these signals to detect subtle changes reflecting stress levels. This approach often involves wearable sensors or medical devices that continuously monitor relevant physiological parameters.Heart rate variability (HRV), for instance, is a powerful indicator of stress. Higher HRV often suggests a more relaxed state, while lower HRV can be a sign of stress or anxiety.
AI algorithms can analyze HRV data over time, identifying patterns that are characteristic of stress in pregnant women. Similarly, sleep patterns, including sleep duration, sleep quality (measured by sleep stages and awakenings), and sleep latency, can be analyzed. Disrupted sleep, a common symptom of stress, can be detected through wearable sleep trackers and analyzed using AI algorithms to identify potential stress indicators.
These algorithms often employ machine learning techniques like Support Vector Machines (SVMs) or Recurrent Neural Networks (RNNs) to identify patterns and make predictions.
Natural Language Processing (NLP) for Analyzing Textual Data
Beyond physiological data, the language used by pregnant women can offer valuable insights into their mental state. NLP techniques allow AI systems to analyze textual data, such as online journal entries, social media posts, or even transcribed therapy sessions. These techniques involve processing and understanding human language to identify s, sentiment, and emotional tone. For example, an increase in negative sentiment expressed in social media posts, coupled with changes in sleep patterns or HRV, might strongly indicate increased stress.
The algorithms used here often incorporate sentiment analysis and topic modeling to uncover hidden patterns and correlations.
Comparison of AI Models for Stress Detection
Several AI models have been developed for stress detection, each with varying levels of effectiveness. The following table compares three different models, showcasing their accuracy, sensitivity, and specificity. Note that these are illustrative examples and actual performance can vary significantly based on data quality, model training, and specific application.
AI Model | Accuracy (%) | Sensitivity (%) | Specificity (%) |
---|---|---|---|
Support Vector Machine (SVM) | 78 | 82 | 75 |
Recurrent Neural Network (RNN) | 85 | 88 | 80 |
Long Short-Term Memory (LSTM) network | 90 | 92 | 87 |
Note: Accuracy refers to the overall correctness of the model’s predictions. Sensitivity measures the model’s ability to correctly identify pregnant women experiencing stress. Specificity measures the model’s ability to correctly identify pregnant women who arenot* experiencing stress. These values are hypothetical examples for illustrative purposes. Actual performance varies considerably depending on factors such as dataset size, feature engineering, and model hyperparameters.
Data Acquisition and Ethical Considerations
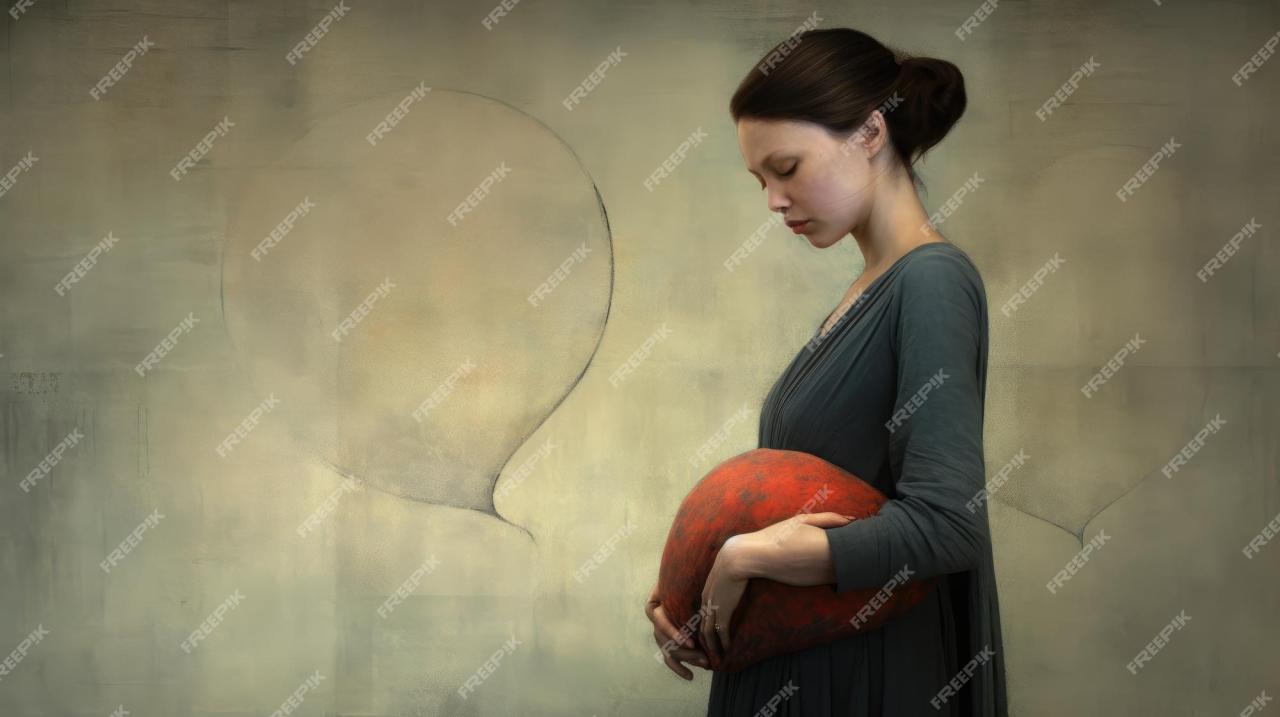
Developing an AI system to detect mental stress in pregnant women requires careful consideration of data acquisition and the ethical implications involved. The accuracy and reliability of the AI model depend heavily on the quality and diversity of the data used for training, while the sensitive nature of the data necessitates robust ethical safeguards to protect patient privacy and autonomy.The successful implementation of such a system hinges on a well-defined data acquisition strategy and a comprehensive ethical framework.
This includes not only the technical aspects of data collection but also the crucial elements of informed consent and data security.
Types of Data Needed for AI Model Training
Effective AI models for stress detection in pregnant women require a multi-modal approach, incorporating various data types to provide a comprehensive picture of an individual’s mental state. This includes both objective physiological measurements and subjective self-reported data. Physiological data might encompass heart rate variability (HRV), measured using wearable sensors or electrocardiograms (ECGs); sleep patterns, obtained through wearable sleep trackers; and potentially even subtle changes in facial expressions captured via video analysis.
Self-reported symptoms, collected through questionnaires or apps, could include stress levels, anxiety scores using validated scales like the State-Trait Anxiety Inventory (STAI), sleep quality, and experiences of mood fluctuations. Combining these objective and subjective data points can lead to a more robust and accurate AI model. For instance, a high HRV coupled with self-reported feelings of anxiety could strongly indicate stress.
Ethical Implications of Collecting and Using Sensitive Health Data
Collecting and using sensitive health data from pregnant women presents several significant ethical challenges. The vulnerability of pregnant women, both physically and emotionally, necessitates extra caution. Data breaches could have severe consequences, potentially leading to social stigma or discrimination. Furthermore, the use of AI in healthcare raises concerns about algorithmic bias. If the training data is not representative of the diverse population of pregnant women, the resulting AI model may be inaccurate or even discriminatory towards certain groups.
Another crucial ethical consideration is the potential for misinterpretation of AI-generated results. Over-reliance on AI predictions without proper clinical oversight could lead to incorrect diagnoses or inappropriate treatment decisions, potentially harming both the mother and the unborn child. The potential for misuse of data for purposes beyond the intended research or clinical application also needs careful consideration.
Data Privacy Protocol for Patient Confidentiality and Informed Consent
A robust data privacy protocol is essential to ensure patient confidentiality and obtain informed consent. This protocol should include:
- Data Anonymization and De-identification: All data should be anonymized and de-identified to remove any personally identifiable information before use in the AI model. This could involve techniques such as data masking or differential privacy.
- Secure Data Storage and Transmission: Data should be stored and transmitted using secure methods, complying with relevant data protection regulations such as HIPAA (in the US) or GDPR (in Europe). This involves encryption, access control, and regular security audits.
- Informed Consent Process: Participants should provide explicit informed consent before their data is collected and used. The consent process should clearly explain the purpose of the study, the types of data collected, how the data will be used, and the potential risks and benefits. Participants should also be informed about their rights to withdraw their data at any time.
- Data Governance and Oversight: A clear data governance structure should be established, including an ethics review board to oversee the entire data lifecycle and ensure compliance with ethical guidelines and regulations. Regular audits and transparency mechanisms are crucial.
- Data Minimization: Only the necessary data should be collected, minimizing the amount of sensitive information gathered. This principle is crucial for reducing the risk of breaches and respecting patient privacy.
AI System Validation and Performance
Validating an AI system designed to detect mental stress in pregnant women requires a rigorous approach encompassing accuracy, reliability, and fairness across diverse populations. This involves a multi-stage process, from initial model development to real-world application, ensuring the system performs as intended and doesn’t perpetuate existing health disparities.The validation process begins with internal testing using a held-out dataset separate from the training data.
AI’s ability to detect mental stress in pregnant women is a game-changer, offering early intervention possibilities. Imagine apps built using the innovative approaches discussed in this article on domino app dev the low code and pro code future , specifically designed to monitor and support expectant mothers. These apps could leverage AI to flag potential issues, connecting women with the necessary resources for a healthier pregnancy and postpartum experience.
This allows for an unbiased assessment of the model’s performance on unseen data, providing an initial estimate of its accuracy and generalizability. Crucially, metrics beyond simple accuracy, such as precision, recall, and F1-score, should be considered to understand the system’s ability to correctly identify both stressed and non-stressed individuals. A high accuracy rate might mask poor performance in specific subgroups, highlighting the importance of comprehensive evaluation.
Performance Metrics and Evaluation Strategies
Several key performance indicators (KPIs) are crucial for assessing the AI system’s effectiveness. Sensitivity (true positive rate) measures the proportion of truly stressed pregnant women correctly identified by the system. Specificity (true negative rate) indicates the proportion of non-stressed women correctly classified. The positive predictive value (PPV) represents the probability that a woman identified as stressed is actually experiencing stress.
Negative predictive value (NPV) reflects the probability that a woman classified as non-stressed is truly not experiencing stress. Receiver Operating Characteristic (ROC) curves and Area Under the Curve (AUC) values are also employed to visualize and quantify the system’s overall performance across different thresholds. A higher AUC value (closer to 1.0) indicates better discriminatory power. For instance, an AUC of 0.9 suggests excellent performance, while an AUC of 0.7 might still be useful but requires careful consideration of its limitations in clinical settings.
The choice of optimal threshold for classifying stress depends on the specific clinical context and the relative costs of false positives and false negatives.
Bias Mitigation Strategies in AI Models
Addressing potential biases in AI models is critical to ensure equitable and reliable stress detection across diverse populations of pregnant women. Bias can arise from various sources, including skewed datasets, algorithmic limitations, and societal factors influencing data collection. For example, a model trained primarily on data from one socioeconomic group might perform poorly on women from different backgrounds, potentially leading to misdiagnosis and unequal access to care.To mitigate bias, careful attention must be paid to data collection and preprocessing.
This includes ensuring a representative sample across demographics such as race, ethnicity, socioeconomic status, age, and parity. Techniques like data augmentation, re-weighting, and adversarial training can help to balance class distributions and reduce bias during model training. Furthermore, ongoing monitoring and evaluation of the system’s performance across different subgroups are essential to identify and address emerging biases over time.
Transparency in the model’s development and deployment is crucial, allowing for scrutiny and iterative improvement. For example, using explainable AI (XAI) techniques can help to understand the factors driving the model’s predictions and identify potential sources of bias. Regular audits and external validation by independent experts are also necessary to maintain the system’s fairness and reliability.
Cross-Validation and Generalizability
To ensure the AI system generalizes well to diverse populations, rigorous cross-validation techniques are necessary. This involves dividing the dataset into multiple subsets, training the model on some subsets, and evaluating its performance on the remaining subsets. K-fold cross-validation, for example, is a common technique where the data is divided into k subsets, and the model is trained and evaluated k times, with each subset used once as the testing set.
This helps to obtain a more robust estimate of the model’s performance and identify potential overfitting to specific subsets of the data. Furthermore, external validation using data from independent sources is crucial to assess the system’s generalizability to real-world settings and different populations. This could involve testing the system on data collected from various hospitals or healthcare providers to ensure its performance remains consistent across different contexts.
Integration into Healthcare Systems
Integrating AI-based stress detection for pregnant women into existing healthcare systems presents a significant opportunity to improve maternal mental health outcomes. However, successful implementation requires careful consideration of various practical challenges and a strategic approach to support healthcare providers. The goal is seamless integration that enhances, not disrupts, current workflows.The AI system can act as a valuable tool for healthcare providers, augmenting their capabilities in identifying and managing stress in pregnant women.
By providing an objective assessment of stress levels, the system can help clinicians prioritize patients who require immediate attention, personalize treatment plans, and track the effectiveness of interventions over time. This can lead to earlier intervention, reduced hospital readmissions, and improved overall maternal well-being.
Workflow Integration Challenges
Successfully integrating the AI system requires addressing several key challenges. These include ensuring data interoperability with existing electronic health record (EHR) systems, obtaining necessary regulatory approvals, providing adequate training for healthcare professionals on the system’s use and interpretation of results, and managing patient privacy and data security concerns. Furthermore, integrating the AI system needs to be cost-effective and not place an undue burden on already stretched healthcare resources.
A phased rollout, starting with pilot programs in selected clinics, can help mitigate these challenges. For example, a pilot program could focus on a specific demographic of high-risk pregnant women within a particular hospital system. The results of this pilot program can then inform broader implementation strategies.
AI System Support for Healthcare Providers
The AI system can support healthcare providers in several ways. First, it can flag high-risk patients for proactive intervention. Second, it can facilitate more personalized treatment plans based on the individual’s stress levels and risk factors. Third, it can track patient progress over time, providing valuable data for evaluating the effectiveness of different interventions. Fourth, the system can help reduce clinician workload by automating aspects of risk assessment and monitoring.
For instance, instead of relying solely on self-reported symptoms, clinicians can leverage the AI’s objective assessment to identify pregnant women experiencing elevated stress who may not have explicitly mentioned their struggles. This early identification can prevent the escalation of stress into more serious mental health conditions.
Workflow Diagram: AI-Integrated Maternal Mental Health Care
The following illustrates the integration of the AI system into a typical prenatal care workflow.* Patient Visit: Pregnant woman attends a routine prenatal appointment.
Data Acquisition
Physiological data (e.g., heart rate variability, sleep patterns, from wearable sensors) and self-reported data (via questionnaires) are collected.
AI Stress Assessment
The AI system analyzes the collected data and provides a stress level assessment.
Clinician Review
The healthcare provider reviews the AI’s assessment alongside other clinical information.
Personalized Intervention
Based on the assessment, the provider develops a personalized care plan, which might include stress management techniques, referral to mental health services, or adjustments to existing treatment plans.
Progress Monitoring
The AI system tracks the patient’s stress levels over time, providing valuable data for evaluating the effectiveness of the intervention.
Data Reporting and Analysis
Aggregated, anonymized data from the AI system contributes to population-level insights on maternal mental health. This data can inform policy decisions and resource allocation.This streamlined workflow improves efficiency, enhances patient care, and empowers healthcare providers with data-driven insights. The AI system does not replace the clinician but serves as a powerful tool to support their decision-making and improve the overall quality of care for pregnant women.
Illustrative Case Studies
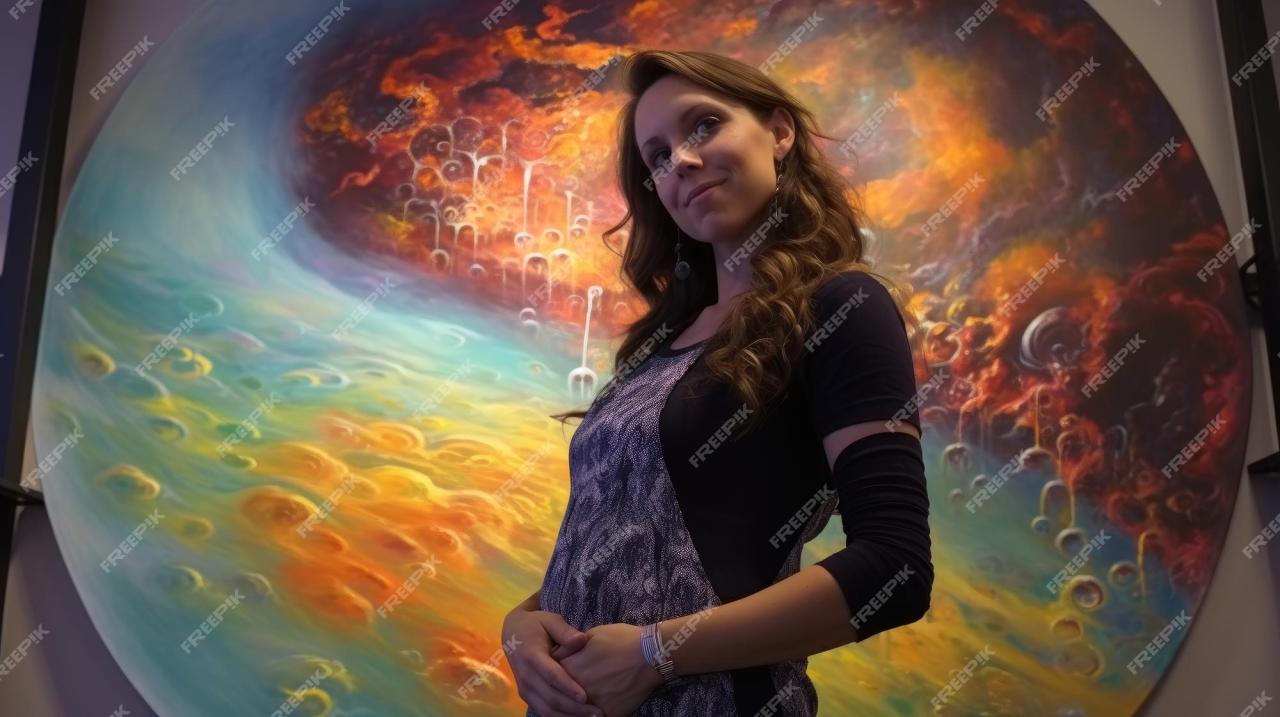
This section presents hypothetical case studies demonstrating how AI-based stress detection can benefit pregnant women with varying risk profiles. These examples illustrate the potential for early intervention and improved maternal and fetal outcomes. The AI system used in these scenarios is assumed to analyze physiological data (heart rate, sleep patterns, activity levels) and self-reported data (via a mobile app) to assess stress levels.
Case Study 1: First-Time Mother Experiencing Anxiety
Sarah, a 28-year-old first-time mother at 20 weeks gestation, reported experiencing significant anxiety related to pregnancy and childbirth. She displayed elevated heart rate variability at night, reduced sleep duration, and decreased physical activity as recorded by wearable sensors. The AI system flagged her as high-risk for stress-related complications. Based on the AI’s analysis, Sarah was offered a personalized intervention plan including guided meditation exercises, access to online support groups, and a referral to a perinatal mental health professional.
Early intervention helped manage her anxiety, leading to improved sleep quality and reduced stress levels. Regular monitoring through the AI system showed a gradual decline in her stress indicators over the subsequent weeks.
Case Study 2: High-Risk Pregnancy with Existing Mental Health Condition
Maria, a 35-year-old mother of two, had a history of depression and was pregnant with twins at 30 weeks gestation. She was considered high-risk due to her pre-existing condition and multiple pregnancy. The AI system detected increased cortisol levels (indicated by wearable sensors) and patterns of sleep disturbance consistent with worsening depression. The AI system also analyzed her self-reported mood scores from a daily check-in within the app, further confirming a decline in her mental wellbeing.
This prompted immediate intervention, including increased frequency of therapy sessions, medication adjustments under the supervision of her psychiatrist, and regular check-ins with her obstetrician. The AI-driven early warning system facilitated timely adjustments to her care plan, minimizing the risk of severe depression and potential negative impacts on the pregnancy.
Case Study 3: Mother Experiencing Postpartum Stress
Aisha, a 32-year-old mother, was six weeks postpartum. The AI system, integrated into a postpartum monitoring app, detected elevated stress levels based on her sleep patterns, activity levels, and self-reported mood. Aisha was experiencing difficulties adjusting to motherhood, including sleep deprivation and feelings of isolation. The AI system alerted her healthcare provider, prompting a follow-up appointment. Aisha received support through home visits from a postpartum doula and was referred to a support group for new mothers.
This timely intervention prevented the escalation of postpartum stress into a more severe condition. The system’s continuous monitoring allowed for the tracking of her progress and ensured ongoing support as needed.
Impact of Early Intervention
Early detection and management of stress in pregnancy, facilitated by AI, has the potential to significantly improve both maternal and fetal outcomes. Studies have shown a link between maternal stress and preterm birth, low birth weight, and other adverse pregnancy outcomes. By providing timely interventions, AI-based systems can help mitigate these risks, leading to healthier pregnancies and healthier babies.
Furthermore, early identification and management of postpartum stress can prevent the development of more serious mental health conditions, such as postpartum depression, and improve the overall well-being of the mother.
Future Directions and Research Needs
The development of AI-based stress detection in pregnant women is a promising field, but significant advancements are still needed to ensure its widespread adoption and effectiveness. Further research should focus on improving the accuracy, generalizability, and ethical implications of these systems, while simultaneously exploring their potential to personalize care and expand their applications beyond stress detection.The accuracy of AI models relies heavily on the quality and diversity of the training data.
Currently, many datasets may lack sufficient representation of diverse populations, leading to biased algorithms that perform poorly for certain subgroups. Furthermore, the complex interplay of physiological, psychological, and social factors influencing stress during pregnancy requires more sophisticated AI models capable of handling this multi-faceted data. Improving the interpretability of AI models is also crucial for building trust among healthcare professionals and patients.
Understanding
why* an AI system flags a woman as stressed is essential for effective intervention.
Improving the Accuracy and Generalizability of AI Models
Addressing limitations in data representation is paramount. Future research must focus on creating larger, more diverse datasets that accurately reflect the heterogeneity of the pregnant population, including variations in age, ethnicity, socioeconomic status, and pre-existing health conditions. This requires collaborative efforts involving researchers, healthcare providers, and pregnant women themselves to ensure data collection is ethically sound and representative.
Moreover, developing more robust AI algorithms, such as those incorporating advanced machine learning techniques like deep learning and transfer learning, can enhance the accuracy and generalizability of stress detection across different contexts and populations. For instance, a model trained on data from one hospital might not perform well in another due to variations in data acquisition methods or patient demographics.
Transfer learning, however, allows us to leverage knowledge gained from one dataset to improve performance on another, thereby increasing generalizability.
Personalizing Mental Health Interventions with AI
AI holds immense potential for personalized mental health interventions. Once stress is detected, AI systems could tailor interventions based on individual risk factors, preferences, and responses to treatment. For example, an AI system could recommend specific relaxation techniques, mindfulness exercises, or cognitive behavioral therapy (CBT) modules based on a woman’s unique profile. Furthermore, AI-powered chatbots could provide ongoing support and monitoring, allowing for timely adjustments to interventions as needed.
This personalized approach could significantly improve the effectiveness of mental health care during pregnancy, leading to better outcomes for both mother and child. A hypothetical example: A woman identified as high-risk for postpartum depression might receive a personalized intervention plan that includes weekly check-ins with a therapist, access to a support group, and a tailored CBT program delivered through an app.
Expanding Applications Beyond Stress Detection
The capabilities of AI extend beyond stress detection. AI algorithms could be trained to identify other mental health conditions during pregnancy, such as anxiety, depression, and even early signs of psychosis. Early detection is critical for timely intervention, potentially preventing more severe complications later. By analyzing a broader range of data, including text from patient journals, speech patterns, and social media activity (with appropriate consent), AI systems could provide a comprehensive assessment of a woman’s mental health status.
This comprehensive approach could lead to earlier identification and intervention for a wider range of mental health conditions, improving overall maternal well-being. For instance, subtle changes in language patterns detected through AI analysis of text messages could indicate a developing depressive episode, prompting proactive intervention by healthcare professionals.
Summary
The application of artificial intelligence to detect mental stress in pregnant women holds immense promise for transforming maternal mental healthcare. While challenges remain in data acquisition, ethical considerations, and system validation, the potential benefits – early intervention, personalized care, and improved maternal and fetal outcomes – are too significant to ignore. As AI technology continues to evolve and research expands, we can anticipate even more sophisticated and effective tools to support the mental well-being of expectant mothers, paving the way for a healthier future for families everywhere.
The journey is just beginning, but the potential impact is undeniably transformative.
FAQ Overview
What types of data does AI use to detect stress in pregnant women?
AI can analyze various data, including physiological data (heart rate, sleep patterns), textual data (journal entries, social media posts), and self-reported symptoms.
Is my data safe and private if used in an AI stress detection system?
Data privacy is crucial. Robust protocols, including anonymization and secure storage, are essential to protect patient confidentiality and comply with data protection regulations.
How accurate are these AI systems in detecting stress?
Accuracy varies depending on the AI model and data used. Ongoing research is focused on improving the sensitivity and specificity of these systems.
Will AI replace healthcare professionals in managing maternal mental health?
No, AI is intended to support healthcare providers, not replace them. It provides valuable insights and tools to aid in diagnosis and treatment planning.