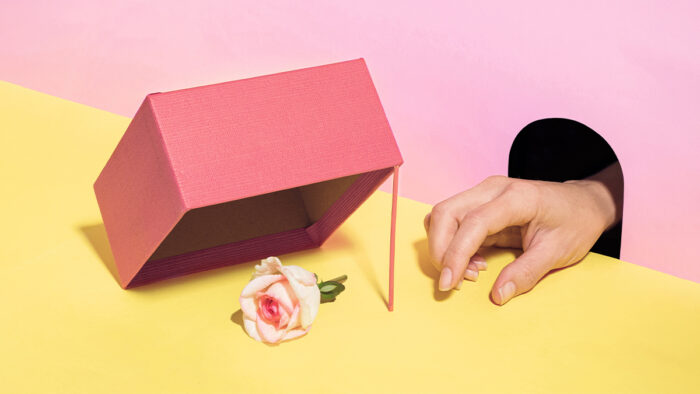
Assessing Generative AIs Impact on Cyber Risk SANS Institute
Assessing generative ais impact on cyber risk sans institute – Assessing Generative AI’s impact on cyber risk, with a focus on the SANS Institute’s perspective, is crucial in today’s rapidly evolving digital landscape. Generative AI, with its ability to create incredibly realistic content, presents both incredible opportunities and significant threats. This post delves into how these powerful AI models can be weaponized for sophisticated cyberattacks, exploring everything from crafting convincing phishing emails to developing novel malware.
We’ll also examine how the SANS Institute is addressing these challenges, providing valuable insights and resources for organizations seeking to protect themselves.
We’ll cover the vulnerabilities introduced by generative AI in software development, the potential for malicious use in social engineering, and compare traditional attacks with those powered by AI. The discussion will also include SANS Institute’s recommendations for mitigating these risks, ethical considerations surrounding responsible AI development, and a glimpse into the future of AI-driven cybersecurity threats.
Generative AI Capabilities and Cyber Risk: Assessing Generative Ais Impact On Cyber Risk Sans Institute
Generative AI, with its ability to create realistic text, images, audio, and code, presents both incredible opportunities and significant threats in the cybersecurity landscape. Its power to automate and scale tasks, previously requiring significant human effort, makes it a potent tool for both defenders and attackers. This duality necessitates a thorough understanding of its capabilities and the potential for misuse.
Generative AI’s Use in Sophisticated Cyberattacks
Generative AI can significantly enhance the sophistication and scale of cyberattacks. Malicious actors can leverage these models to automate the creation of phishing emails, crafting highly personalized and convincing messages tailored to individual victims. They can also generate realistic malware code, bypassing traditional signature-based detection systems. Furthermore, AI can be used to analyze network traffic, identify vulnerabilities, and develop highly targeted attacks with unprecedented efficiency.
The automation allows for a massive increase in the number of attacks launched, making detection and response far more challenging. For example, a generative AI could analyze thousands of past phishing campaigns to identify the most successful tactics, then generate new, highly effective phishing emails based on this analysis.
Vulnerabilities Introduced by Generative AI in Software Development and Deployment
The use of generative AI in software development, while promising increased efficiency, also introduces new vulnerabilities. AI-generated code, while functional, may contain hidden backdoors or security flaws due to biases in the training data or limitations in the AI’s understanding of security best practices. Furthermore, the reliance on AI-generated code can reduce human oversight, potentially leading to the deployment of insecure software.
A scenario might involve an AI generating code for a web application that inadvertently includes a SQL injection vulnerability because its training data lacked sufficient examples of secure coding practices for that specific scenario. This vulnerability could then be exploited by attackers to gain unauthorized access to sensitive data.
Generative AI’s Leverage for Malicious Purposes: Phishing and Social Engineering
Generative AI dramatically amplifies the effectiveness of phishing and social engineering attacks. It can generate highly personalized phishing emails, convincingly mimicking legitimate communication from banks, businesses, or even individuals known to the target. The AI can also create realistic voice recordings for voice phishing scams, making them incredibly difficult to detect. The scale at which these attacks can be launched is exponentially increased compared to traditional methods, overwhelming human defenses and increasing the likelihood of successful attacks.
For instance, an AI could generate thousands of personalized phishing emails targeting specific individuals within an organization, each tailored to their role and interests, making them much more likely to fall victim.
Hypothetical Scenario: A Successful Generative AI-Based Cyberattack, Assessing generative ais impact on cyber risk sans institute
Imagine a scenario where a malicious actor uses a generative AI to analyze the public profiles of employees at a financial institution. The AI identifies key individuals, their interests, and their communication patterns. It then crafts highly personalized phishing emails, complete with realistic attachments, designed to trick these employees into revealing their credentials. The AI further generates malicious code to exploit any vulnerabilities discovered during the initial reconnaissance phase.
This multi-pronged attack, leveraging the AI’s ability to automate and personalize attacks, successfully compromises the institution’s systems, leading to a significant data breach.
Comparison: Traditional Cyberattacks vs. Generative AI-Facilitated Attacks
Feature | Traditional Cyberattacks | Generative AI-Facilitated Attacks |
---|---|---|
Scale | Limited by human effort | Massive scale, automated attacks |
Personalization | Generic or limited personalization | Highly personalized, tailored to individuals |
Sophistication | Relies on known vulnerabilities and techniques | Can discover and exploit new vulnerabilities, create novel attack vectors |
Detection | Relatively easier to detect with signature-based systems | Difficult to detect due to novel techniques and personalization |
Cost | Relatively lower cost | Higher initial investment in AI infrastructure, but potentially higher ROI due to scale and success rate |
SANS Institute’s Perspective on Generative AI and Cybersecurity
The SANS Institute, a leading provider of cybersecurity training and certification, recognizes the transformative potential of generative AI while simultaneously acknowledging its significant implications for cybersecurity. Their research and training materials actively address the evolving threat landscape shaped by this technology, emphasizing proactive mitigation strategies for organizations of all sizes.
SANS consistently highlights the dual nature of generative AI: its ability to automate tasks and improve security defenses, alongside its potential for malicious exploitation. Their perspective is not one of fear-mongering, but rather a pragmatic assessment of the risks and opportunities, offering practical guidance for navigating this complex technological shift.
SANS Institute’s Published Research and Viewpoints
SANS Institute’s publications frequently feature articles, white papers, and blog posts analyzing the cybersecurity implications of generative AI. These resources delve into specific threats like the creation of sophisticated phishing emails, the automation of large-scale attacks, and the generation of realistic malware. They also explore the use of generative AI for defensive purposes, such as threat detection and incident response automation.
For instance, a recent SANS white paper detailed how generative AI could be used to create highly convincing social engineering attacks, targeting individuals with personalized phishing campaigns based on their online activity. This analysis provided practical recommendations for organizations to bolster their security awareness training programs.
Examples of SANS Training Materials Addressing Generative AI Threats
SANS offers various training courses and resources specifically designed to address the cybersecurity challenges posed by generative AI. These include hands-on workshops focusing on detecting and responding to AI-powered attacks, as well as online courses that explore the ethical and legal considerations of using generative AI in cybersecurity. Specific examples might include modules within existing courses, such as those focusing on incident response or advanced persistent threats (APTs), that are updated to incorporate the latest threats from generative AI.
These modules could feature case studies of real-world attacks leveraging generative AI for malicious purposes, illustrating how attackers are exploiting the technology. Furthermore, SANS likely incorporates discussions on the ethical considerations of using generative AI for both offensive and defensive security purposes within its training materials.
Adaptation of SANS Certifications and Training Programs
SANS is actively adapting its certifications and training programs to reflect the evolving threat landscape. This includes updating existing courses to incorporate the latest threats and mitigation strategies related to generative AI. New courses and certifications specifically focused on generative AI and its security implications are also being developed to provide professionals with the necessary skills and knowledge to address these emerging challenges.
This adaptation ensures that SANS-certified professionals remain at the forefront of cybersecurity expertise, capable of handling the complexities of AI-powered threats.
Key Areas for Mitigating Generative AI-Related Cyber Risks (SANS Recommendations)
The SANS Institute likely recommends focusing efforts on several key areas to mitigate generative AI-related cyber risks. These include strengthening security awareness training, investing in advanced threat detection systems capable of identifying AI-generated attacks, and developing robust incident response plans that specifically address AI-powered threats. Furthermore, they likely emphasize the importance of proactive threat intelligence gathering to stay ahead of emerging threats and adopting a strong security posture based on the principles of defense in depth.
SANS Institute’s Recommendations for Organizations
Area | Recommendation | Example | Benefit |
---|---|---|---|
Security Awareness Training | Enhance training to include specific threats from generative AI. | Simulations of AI-powered phishing attacks. | Improved employee awareness and reduced susceptibility to social engineering. |
Threat Detection | Implement advanced threat detection systems capable of identifying AI-generated attacks. | Utilizing AI-powered security information and event management (SIEM) systems. | Faster identification and response to sophisticated attacks. |
Incident Response | Develop incident response plans that specifically address AI-powered threats. | Creating playbooks for handling AI-generated malware or disinformation campaigns. | Faster containment and recovery from AI-driven incidents. |
Threat Intelligence | Proactively gather threat intelligence on emerging AI-related threats. | Subscribing to threat intelligence feeds specializing in AI-powered attacks. | Improved preparedness and proactive mitigation of future threats. |
Mitigating the Risks of Generative AI in Cybersecurity
The rapid advancement of generative AI presents both exciting opportunities and significant cybersecurity risks. While generative AI can enhance our defenses, it also empowers malicious actors with sophisticated tools for crafting more convincing phishing emails, generating realistic malware, and automating large-scale attacks. Effectively mitigating these risks requires a proactive and multi-layered approach, combining advanced detection methods, robust security protocols, and the strategic deployment of AI itself.
Detecting and Preventing Generative AI-Based Cyberattacks
Generative AI-based attacks are evolving rapidly, making traditional security measures insufficient. Detecting these attacks requires a shift towards more sophisticated techniques that can identify subtle anomalies and patterns indicative of AI-generated content. This includes analyzing the stylistic nuances of emails and other communications, detecting inconsistencies in code generated by AI, and identifying unusual patterns in network traffic that might suggest automated attacks.
Prevention involves strengthening authentication protocols, implementing robust anti-phishing measures, and regularly updating security software to counter emerging threats.
Security Protocols and Best Practices for Mitigating Generative AI Risks in Organizations
Organizations must adopt a comprehensive security strategy that addresses the unique challenges posed by generative AI. This involves establishing clear guidelines for the use of generative AI tools within the organization, implementing strict access controls to sensitive data, and regularly auditing systems for vulnerabilities. Employee training is crucial, focusing on identifying and reporting suspicious activities and understanding the potential risks associated with generative AI.
Regular security assessments and penetration testing are also vital to proactively identify and address weaknesses in the organization’s defenses. Furthermore, adopting a zero-trust security model, where access is granted based on continuous verification, is increasingly important in mitigating risks associated with both internal and external generative AI threats.
The Role of AI in Detecting and Responding to Generative AI-Based Attacks
Ironically, AI can play a crucial role in detecting and responding to attacks powered by generative AI. AI-powered security tools can analyze vast amounts of data to identify subtle patterns and anomalies that might indicate a generative AI-based attack. These tools can be used to analyze network traffic, email content, and code for signs of malicious activity, providing early warning of potential threats.
Furthermore, AI can automate incident response, accelerating the process of containment and remediation. For example, an AI system could automatically quarantine infected systems or block malicious traffic upon detection of a threat. This rapid response capability is critical in minimizing the impact of a successful attack.
A Comprehensive Cybersecurity Strategy Incorporating Defenses Against Generative AI Threats
A robust cybersecurity strategy must be adaptable and proactive, incorporating several key elements to counter generative AI threats. This strategy needs to integrate traditional security measures with advanced AI-powered detection and response capabilities. It should encompass: a strong security awareness program for employees, robust authentication and access control mechanisms, regular security audits and penetration testing, and the implementation of AI-driven security information and event management (SIEM) systems.
The strategy should also account for the potential misuse of generative AI tools within the organization, establishing clear guidelines and controls for their usage. Finally, it’s crucial to establish strong incident response plans specifically tailored to handle generative AI-based attacks, including rapid containment, recovery, and post-incident analysis.
Essential Security Tools and Technologies for Combating Generative AI-Related Risks
Implementing a comprehensive defense requires a combination of tools and technologies. This includes:
- Advanced Threat Protection (ATP) solutions: These solutions utilize AI and machine learning to detect and prevent advanced persistent threats, including those leveraging generative AI.
- Security Information and Event Management (SIEM) systems: SIEM systems aggregate and analyze security logs from various sources, providing valuable insights into potential threats. AI-enhanced SIEM systems can identify subtle patterns indicative of AI-generated attacks.
- AI-powered Intrusion Detection and Prevention Systems (IDPS): These systems leverage AI to detect and block malicious network traffic, including traffic generated by AI-powered tools.
- Data Loss Prevention (DLP) tools: DLP tools help prevent sensitive data from leaving the organization, mitigating the risk of data breaches facilitated by generative AI.
- Anti-phishing solutions: Advanced anti-phishing solutions can detect and block sophisticated phishing emails and other social engineering attacks generated by AI.
- Generative AI detection tools: These specialized tools are designed specifically to identify content generated by AI, enabling organizations to detect and mitigate the risks associated with AI-generated malware and phishing campaigns.
Ethical Considerations and Responsible AI Development
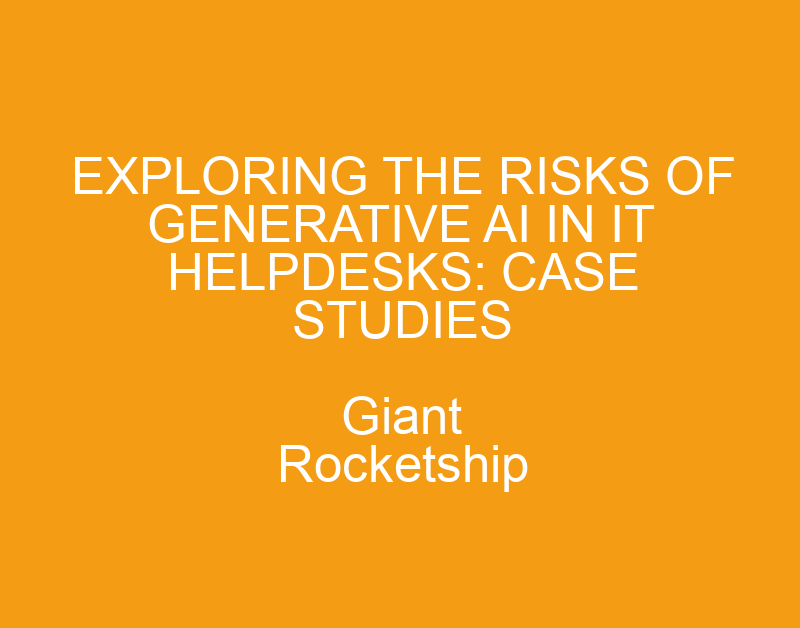
The integration of generative AI into cybersecurity presents a complex ethical landscape. While offering powerful new tools for defense, it also introduces significant risks, demanding careful consideration of potential biases, unintended consequences, and the broader societal impact. Responsible development and deployment are paramount to prevent the misuse of these technologies and ensure their benefits outweigh the inherent dangers.
Potential Biases and Unintended Consequences in Generative AI for Cybersecurity
Generative AI models are trained on vast datasets, and if these datasets reflect existing societal biases (e.g., racial, gender, socioeconomic), the AI system will likely perpetuate and even amplify those biases in its outputs. In cybersecurity, this could lead to unfair or discriminatory outcomes, such as biased threat detection systems that disproportionately target certain groups or inaccurate risk assessments based on flawed assumptions.
Assessing the impact of generative AI on cyber risk, as explored by SANS Institute, is crucial. The rapid development of applications, accelerated by trends like those discussed in this insightful article on domino app dev, the low-code and pro-code future , means we need to understand how these new tools might introduce vulnerabilities. Ultimately, this understanding is key to mitigating the growing cyber risks associated with AI’s expanding role.
Furthermore, unintended consequences can arise from the unpredictable nature of generative models; a system designed to detect malware might inadvertently flag legitimate software or generate false positives, leading to disruptions and resource wastage. For instance, an AI trained primarily on data from one geographical region might be less effective at detecting attacks originating from other regions with different attack vectors.
Approaches to Responsible AI Development in Cybersecurity
Several approaches contribute to responsible AI development. Explainable AI (XAI) techniques aim to make the decision-making processes of AI models more transparent and understandable, allowing for better scrutiny and identification of potential biases. Robust testing and validation are crucial to ensure the reliability and accuracy of AI systems before deployment. This includes rigorous evaluation across diverse datasets and scenarios to identify and mitigate potential weaknesses.
Furthermore, incorporating human oversight and feedback loops allows for continuous monitoring and adjustment of AI systems, minimizing the risk of unforeseen consequences. The development of clear ethical guidelines and standards specifically for AI in cybersecurity can also help to guide developers and users towards responsible practices. For example, a company might establish an internal review board to evaluate the ethical implications of new AI-powered security tools before their release.
Legal and Regulatory Challenges Associated with Generative AI in Cyberattacks
The use of generative AI in cyberattacks poses novel legal and regulatory challenges. Attributing responsibility for attacks involving AI becomes complex, especially when the AI system acts autonomously or unexpectedly. Existing laws may not adequately address the unique aspects of AI-enabled cybercrime, such as the creation of sophisticated malware or the automation of phishing campaigns. The rapid pace of AI development outstrips the capacity of legal frameworks to keep pace, leading to a regulatory gap that needs to be addressed through international cooperation and the development of new legislation tailored to the specific risks of AI in cybersecurity.
For example, the question of liability when a generative AI model creates novel malware used in an attack remains largely unresolved.
Transparency and Accountability in Generative AI Systems
Transparency and accountability are crucial for building trust and ensuring the responsible use of generative AI in cybersecurity. This involves making the underlying data, algorithms, and decision-making processes of AI systems accessible to relevant stakeholders, allowing for scrutiny and validation. Mechanisms for accountability need to be established to address potential harms caused by AI systems, whether through negligence or malicious intent.
This might involve establishing clear lines of responsibility for developers, deployers, and users of AI systems. Open-source development and collaborative research can promote transparency and allow for community oversight, reducing the likelihood of hidden biases or vulnerabilities. For example, open-sourcing parts of a threat detection system can allow security researchers to identify and report potential flaws.
Framework for Responsible AI Development and Deployment
A framework for responsible AI development and deployment should encompass several key elements: Firstly, a strong ethical foundation that prioritizes fairness, transparency, and accountability. Secondly, rigorous testing and validation procedures to ensure reliability and accuracy. Thirdly, mechanisms for human oversight and feedback to mitigate risks and address unintended consequences. Fourthly, clear legal and regulatory frameworks to address the unique challenges of AI in cybersecurity.
Finally, continuous monitoring and improvement to adapt to the evolving threat landscape. This framework should be adaptable and iterative, allowing for adjustments as new technologies and challenges emerge. This proactive approach is vital in navigating the ethical complexities and ensuring the safe and beneficial integration of generative AI into cybersecurity practices.
Future Implications and Research Directions
The rapid advancement of generative AI presents both unprecedented opportunities and significant challenges for cybersecurity. Its ability to automate tasks, analyze vast datasets, and generate novel content has profound implications for both offensive and defensive strategies, leading to a constantly evolving arms race in the digital realm. Understanding and proactively addressing these implications is crucial for maintaining a secure digital future.The future impact of generative AI on cybersecurity will be multifaceted and far-reaching.
We’re likely to see a dramatic increase in the sophistication and scale of cyberattacks, driven by the ease with which malicious actors can leverage generative AI to create highly personalized phishing campaigns, develop novel malware variants, and automate reconnaissance efforts. Simultaneously, generative AI offers powerful tools for defenders, enabling faster threat detection, more effective incident response, and the automation of previously manual security tasks.
This duality necessitates a proactive and adaptable approach to cybersecurity, one that embraces the potential of generative AI while mitigating its inherent risks.
Generative AI-Based Cyberattack Evolution
Generative AI will significantly alter the landscape of cyberattacks. We can anticipate a shift towards more targeted and personalized attacks, utilizing AI-generated phishing emails and social engineering campaigns tailored to individual victims. The automation capabilities of generative AI will enable the creation and deployment of malware at an unprecedented scale, potentially overwhelming traditional defense mechanisms. Moreover, the ability of generative AI to generate realistic deepfakes and synthetic media will further complicate threat identification and response.
For example, imagine a highly convincing deepfake video of a CEO authorizing a large financial transaction, used to bypass multi-factor authentication. The complexity and scale of such attacks will require equally sophisticated and adaptive defense mechanisms.
Evolution of Generative AI-Based Defense Mechanisms
In response to these evolving threats, we can expect to see the development of advanced AI-powered security solutions. These solutions will leverage generative AI’s capabilities to detect anomalies, predict potential attacks, and automate incident response. For instance, AI-powered systems could analyze network traffic in real-time to identify subtle patterns indicative of malicious activity, proactively blocking attacks before they can cause damage.
Furthermore, generative AI can be used to create synthetic datasets for training security models, improving their accuracy and resilience against adversarial attacks. This arms race between attackers and defenders will necessitate continuous innovation and adaptation.
Critical Research Areas in Generative AI Security
Several key areas require focused research and development to effectively address the challenges posed by generative AI in cybersecurity. This includes developing robust methods for detecting AI-generated malicious content, creating AI-resistant security protocols, and establishing ethical guidelines for the development and deployment of generative AI in security applications. Research into explainable AI (XAI) is crucial to understand the decision-making processes of AI-powered security systems, ensuring transparency and accountability.
Furthermore, exploring the use of differential privacy and federated learning techniques can help protect sensitive data used to train and deploy these systems. Investing in robust cybersecurity education and training programs is equally critical to prepare the workforce for this evolving threat landscape.
Predicted Growth of Generative AI-Related Cyber Threats
A textual representation of the predicted growth of generative AI-related cyber threats over the next five years could be visualized as a steeply rising curve. Imagine a graph with the x-axis representing the years (2024-2028) and the y-axis representing the number of generative AI-related cyberattacks. The curve would start relatively flat in 2024, reflecting the nascent stage of generative AI’s application in cybercrime.
However, from 2025 onwards, the curve would ascend sharply, indicating a rapid increase in the number and sophistication of these attacks. By 2028, the curve would be significantly steeper, demonstrating a substantial escalation in the threat level. This projection is based on the accelerating adoption of generative AI technologies, the decreasing cost of access to these technologies, and the increasing sophistication of malicious actors.
For example, we might see a tenfold increase in AI-powered phishing attacks from 2024 to 2028, mirroring the observed exponential growth in other areas of cybercrime. This underscores the urgent need for proactive measures to mitigate these escalating threats.
Closing Notes
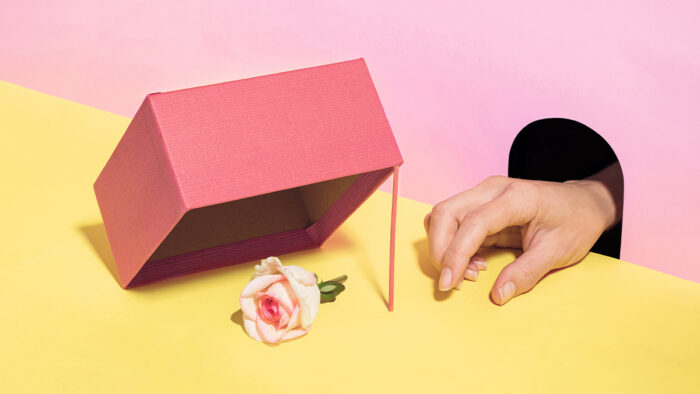
The rise of generative AI undeniably reshapes the cybersecurity landscape, presenting both unprecedented challenges and opportunities. Understanding the SANS Institute’s perspective and implementing robust mitigation strategies are paramount. By proactively addressing the ethical implications and fostering responsible AI development, we can strive towards a future where AI enhances cybersecurity rather than exacerbates its risks. Staying informed and adapting to these changes is not just a best practice; it’s a necessity for survival in the digital age.
Helpful Answers
What specific types of malware can generative AI create?
Generative AI can create highly customized and polymorphic malware, making detection and analysis significantly more difficult than traditional malware.
How can generative AI be used in social engineering attacks?
AI can create incredibly realistic phishing emails, spear-phishing campaigns, and deepfakes, making it harder to distinguish legitimate communication from malicious attempts.
What role do AI-powered security tools play in this fight?
AI-powered security tools can help detect and respond to generative AI-based attacks by identifying patterns and anomalies in network traffic and user behavior that might indicate malicious activity.
Are there any legal ramifications for using generative AI in cyberattacks?
Yes, the legal landscape is still evolving, but using generative AI for malicious purposes will likely lead to significant legal consequences, depending on the jurisdiction and the nature of the attack.