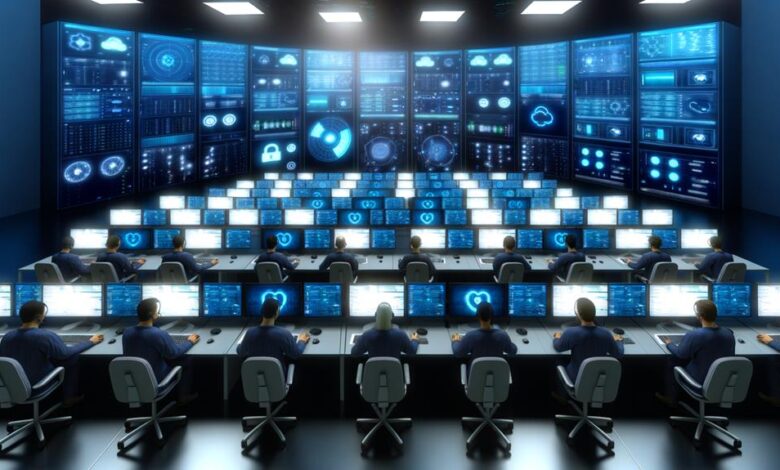
Automation Is Key to Strengthen Cybersecurity Says IBM
Automation is key to strengthen cybersecurity says IBM, and this statement couldn’t be more relevant in today’s increasingly complex threat landscape. We’re facing a deluge of sophisticated cyberattacks, from ransomware to data breaches, and traditional manual security methods are simply struggling to keep pace. This post delves into why IBM’s assertion holds true, exploring the benefits, challenges, and future of automated cybersecurity.
The sheer volume and velocity of cyber threats necessitate a shift towards automated defenses. Think of it like this: a single security analyst can only monitor so many systems manually. Automation allows us to analyze massive datasets, identify anomalies, and respond to threats in real-time – something humanly impossible at scale. This post will explore various types of automation, the role of AI, and the crucial human element that remains essential even in a highly automated security environment.
IBM’s Statement on Automation in Cybersecurity
IBM’s assertion that automation is key to strengthening cybersecurity is a powerful statement reflecting a significant shift in how we approach digital security. In today’s complex threat landscape, relying solely on human intervention is simply unsustainable. The sheer volume and sophistication of cyberattacks necessitate a more proactive and efficient defense strategy, and automation provides the tools to achieve this.The implications of this statement are far-reaching.
It signals a move away from reactive, largely manual security practices towards a more preventative, automated approach. This requires a significant investment in technology and a shift in organizational culture, but the potential rewards in terms of improved security posture and reduced risk are substantial.
Benefits of Automated Cybersecurity Defenses
Automation offers several key advantages in enhancing cybersecurity. Firstly, it significantly increases speed and efficiency. Automated systems can identify and respond to threats in real-time, often before human analysts even detect them. Secondly, automation improves consistency and reduces human error. Manual processes are prone to mistakes, while automated systems consistently apply predefined security policies and protocols.
Finally, automation allows organizations to scale their security operations to match the ever-growing volume of data and potential threats. It enables the monitoring of vast networks and systems that would be impossible to manage manually.
Examples of Automation’s Advantages in Addressing Specific Threats
Automation shines brightest when dealing with high-volume, fast-moving threats. Consider the following examples:* Distributed Denial-of-Service (DDoS) attacks: Automated systems can quickly detect and mitigate DDoS attacks by identifying anomalous traffic patterns and automatically rerouting traffic or blocking malicious sources. Manual intervention would be far too slow to be effective.
Phishing attacks
Automated systems can analyze incoming emails for suspicious characteristics, such as links to malicious websites or unusual sender addresses, flagging them for review or automatically quarantining them. Manual review of every email is impractical in large organizations.
Malware detection
Automated systems can continuously scan systems and networks for malicious software, identifying and quarantining infected files before they can cause damage. Manual scanning is time-consuming and often misses subtle indicators of compromise.
Vulnerability management
Automated vulnerability scanners can regularly assess systems for known security weaknesses, providing alerts and facilitating timely patching. Manual vulnerability assessments are slow and often incomplete.
Comparison of Manual vs. Automated Cybersecurity Approaches
Task | Manual Approach | Automated Approach | Efficiency Comparison |
---|---|---|---|
Incident Response | Human analysts investigate alerts, manually analyze logs, and implement remediation steps. This is time-consuming and prone to errors. | Automated systems detect, analyze, and respond to incidents in real-time, minimizing damage and downtime. | Automated approach is significantly more efficient, offering faster response times and reduced human error. |
Vulnerability Scanning | Manual scans are slow, incomplete, and may miss vulnerabilities. | Automated scanners regularly assess systems for known vulnerabilities, providing comprehensive reports and facilitating timely patching. | Automated approach is far more efficient, providing more complete and timely vulnerability identification. |
Log Analysis | Manual log analysis is tedious, time-consuming, and prone to overlooking critical events. | Automated systems analyze logs in real-time, identifying suspicious patterns and alerting security personnel to potential threats. | Automated approach is much more efficient, allowing for faster detection of threats and improved overall security. |
Malware Detection | Manual malware detection relies on signature-based antivirus software and is often reactive, leaving systems vulnerable. | Automated systems employ advanced techniques such as machine learning to detect both known and unknown malware, providing proactive protection. | Automated approach offers superior protection and is more efficient at detecting and responding to malware threats. |
Types of Automation in Cybersecurity
Cybersecurity automation is no longer a luxury; it’s a necessity. The sheer volume of data, the sophistication of threats, and the ever-shrinking response windows demand automated solutions. This post delves into the different types of cybersecurity automation, exploring their strengths, weaknesses, and applications within a modern security framework. We’ll examine the crucial role of AI and machine learning, and highlight key use cases to illustrate the practical benefits.Cybersecurity automation encompasses a wide range of tools and techniques designed to streamline and enhance various security functions.
These range from simple scripting to sophisticated AI-driven systems, all working towards a common goal: improving efficiency and effectiveness in protecting digital assets. The level of automation implemented depends on the organization’s specific needs, resources, and risk tolerance.
Automated Threat Detection and Response
Automated threat detection and response systems leverage various technologies to identify, analyze, and respond to security incidents. These systems typically incorporate intrusion detection systems (IDS), security information and event management (SIEM) tools, and endpoint detection and response (EDR) solutions. They use automated rules and algorithms to detect malicious activity, such as malware infections, unauthorized access attempts, or unusual network traffic patterns.
Upon detection, automated responses can be triggered, including blocking malicious connections, quarantining infected systems, or alerting security personnel. The speed and efficiency offered by automated systems are invaluable in mitigating the impact of cyberattacks. For example, a system might automatically quarantine a compromised endpoint before the malware can spread to other devices on the network, significantly reducing the damage.
Security Information and Event Management (SIEM) Automation
SIEM systems collect and analyze security logs from various sources across an organization’s IT infrastructure. Automation within SIEM enhances its capabilities by enabling automated alert generation, correlation of security events, and automated incident response. For instance, a SIEM system could be configured to automatically generate an alert when a specific pattern of suspicious activity is detected, such as multiple failed login attempts from a single IP address.
Furthermore, automation can help prioritize alerts based on severity and potential impact, allowing security teams to focus on the most critical threats. AI and machine learning can further improve SIEM automation by identifying subtle anomalies and patterns that might otherwise be missed by human analysts. This allows for proactive threat hunting and a reduction in false positives.
Vulnerability Management Automation
Automated vulnerability scanning and remediation are critical for maintaining a strong security posture. Automated vulnerability scanners regularly assess systems and applications for known vulnerabilities, providing detailed reports on identified weaknesses. This automation dramatically reduces the time and resources required for manual vulnerability assessments. Furthermore, some systems can even automatically deploy patches or apply configuration changes to mitigate identified vulnerabilities, significantly accelerating the remediation process.
A company could, for example, automate the patching of critical vulnerabilities across all their servers within hours of a patch being released, reducing their attack surface substantially.
AI and Machine Learning in Automated Cybersecurity
AI and machine learning are transforming the cybersecurity landscape by enabling more sophisticated and adaptive automated systems. These technologies can analyze vast amounts of data to identify complex patterns and anomalies that might indicate malicious activity. AI-powered systems can learn from past incidents to improve their accuracy and effectiveness over time. Machine learning algorithms can be trained to detect new and evolving threats, which are often difficult for traditional rule-based systems to identify.
This adaptive capability is crucial in today’s dynamic threat environment. For example, an AI-powered system might identify a new type of malware based on its behavior, even if it has never encountered that specific malware before.
Endpoint Protection Automation
Endpoint protection solutions, such as antivirus software and EDR tools, can be automated to improve their effectiveness. Automation can streamline tasks such as software updates, malware scanning, and incident response. For example, an automated endpoint protection system could automatically update its antivirus definitions, scan for malware on a regular basis, and quarantine any infected files. This reduces the workload on security personnel and ensures that endpoints are consistently protected against the latest threats.
Real-time threat intelligence feeds can also be integrated into automated endpoint protection systems, allowing them to proactively respond to emerging threats.
- Automated Patch Management: Automatically deploying security patches to systems and applications, minimizing vulnerabilities.
- Automated Malware Analysis: Automatically analyzing suspicious files and code to identify malicious behavior.
- Automated Incident Response: Automatically isolating infected systems, blocking malicious traffic, and restoring systems to a safe state.
- Automated Security Awareness Training: Automatically delivering security awareness training to employees, reinforcing best practices.
- Automated User Access Management: Automatically provisioning and de-provisioning user accounts, ensuring appropriate access control.
Challenges and Limitations of Automated Cybersecurity
While automation significantly boosts cybersecurity capabilities, relying solely on it presents considerable challenges. A balanced approach, combining automation with human expertise, is crucial for robust security. Over-reliance on automated systems can create vulnerabilities and blind spots, highlighting the persistent need for human oversight and critical thinking.Automation bias, the tendency to overtrust automated systems even when they are flawed, is a significant concern.
This can lead to overlooking critical security threats or misinterpreting alerts, resulting in delayed responses and increased risk. Furthermore, the automation itself can introduce new vulnerabilities, such as software bugs or misconfigurations, that attackers can exploit. Finally, the sheer complexity of modern cybersecurity landscapes often surpasses the capabilities of even the most advanced automated systems, necessitating human intervention for effective threat analysis and response.
Automation Bias and Human Oversight
The over-reliance on automated systems can lead to a phenomenon known as automation bias. This is where human operators place excessive trust in automated decisions, even when those decisions are incorrect or incomplete. In cybersecurity, this can manifest as ignoring alerts flagged by human analysts because the automated system hasn’t raised a similar flag, or failing to investigate anomalies because the automated system deems them benign.
The consequences can be severe, with critical threats going undetected or mitigated ineffectively. Therefore, maintaining a robust human-in-the-loop approach is paramount, ensuring that human analysts review automated findings, investigate anomalies, and make final decisions regarding security incidents. Effective cybersecurity requires a collaborative approach where human expertise complements and verifies automated processes.
Vulnerabilities Introduced by Automation
Automated cybersecurity systems, while beneficial, can themselves introduce vulnerabilities. For instance, a flaw in the automated system’s code could allow an attacker to bypass security controls or manipulate the system’s responses. Similarly, poorly configured automated systems might create unintended security loopholes. These automated systems are often complex pieces of software, and like any software, they are susceptible to bugs and vulnerabilities that could be exploited by malicious actors.
Furthermore, the reliance on machine learning algorithms in many automated systems means that they are only as good as the data they are trained on. Biased or incomplete training data can lead to inaccurate or ineffective threat detection, leaving the system vulnerable to novel attack vectors. Regular audits, rigorous testing, and continuous monitoring are crucial to identify and mitigate these vulnerabilities.
Scenario: Automated Security Failure Requiring Human Intervention
Imagine a scenario where a sophisticated phishing attack targets a company’s employees. The company uses an automated email filtering system to detect and block malicious emails. However, the attackers employ a novel technique that bypasses the automated system’s filters. The phishing emails successfully reach employee inboxes, and several employees fall victim to the attack, revealing sensitive company information.
The automated system, having failed to identify the threat, does not generate any alerts. Only through subsequent manual analysis of the data breach – perhaps noticing an unusual pattern of data exfiltration – do human analysts discover the attack and its extent. This illustrates how even the most advanced automated systems can fail, emphasizing the importance of human vigilance and proactive monitoring to detect and respond to sophisticated threats that evade automated defenses.
The Human Element in Automated Cybersecurity
Automation is revolutionizing cybersecurity, but it’s crucial to remember that technology alone isn’t a silver bullet. Human expertise remains indispensable, providing the critical thinking, judgment, and adaptability that automated systems currently lack. While AI and machine learning can handle repetitive tasks and detect known threats at scale, the human element is essential for navigating the complexities of evolving cyber threats and ensuring the overall effectiveness of cybersecurity strategies.The role of human cybersecurity professionals is shifting significantly in the age of automation.
Instead of manually analyzing every alert, security professionals now focus on strategic oversight, incident response, and the development and refinement of automated systems. This requires a new skillset and a deeper understanding of how automated tools operate and interact within the larger security landscape.
Human Expertise in Managing Automated Cybersecurity Systems
Effective management of automated cybersecurity systems requires a deep understanding of the technology’s capabilities and limitations. Security professionals must be able to configure, monitor, and troubleshoot automated tools, ensuring they are operating correctly and providing accurate insights. This includes understanding the underlying algorithms, identifying potential biases or vulnerabilities in the systems, and interpreting the data generated by automated tools to inform strategic decision-making.
For instance, a security analyst might need to investigate a false positive generated by an intrusion detection system, requiring them to analyze the underlying network traffic and system logs to determine if a genuine threat exists. Without human intervention, such false positives could lead to wasted resources and a decline in overall system performance.
Necessary Skills and Training for Cybersecurity Professionals
The skills required for cybersecurity professionals in an automated environment are significantly different from those needed in a purely manual environment. Training programs must adapt to reflect this change, focusing on areas such as data analysis, machine learning principles, and the ability to interpret and utilize the outputs of automated security tools. Furthermore, professionals need strong problem-solving skills to handle unexpected situations and adapt to new threats.
A strong understanding of scripting languages like Python is also increasingly valuable, allowing for automation of routine tasks and customization of security tools.
Comparison of Responsibilities Before and After Automation Implementation, Automation is key to strengthen cybersecurity says ibm
Before the widespread adoption of automated systems, cybersecurity professionals were primarily involved in manual tasks such as analyzing logs, investigating alerts, and configuring security devices. Their responsibilities were largely reactive, focusing on responding to existing threats. After the implementation of automated systems, the focus shifts towards proactive threat hunting, system optimization, and strategic planning. While reactive responses are still necessary, a larger portion of the workload involves preventing threats before they occur.
IBM’s right, automation is crucial for beefing up cybersecurity; we need faster, more efficient solutions to combat ever-evolving threats. This is where the power of streamlined development comes in, as highlighted in this insightful piece on domino app dev, the low-code and pro-code future , which shows how rapid application development can significantly enhance our ability to build and deploy automated security measures.
Ultimately, faster development cycles powered by automation are key to winning the cybersecurity battle.
This requires a deeper understanding of threat intelligence, vulnerability management, and the ability to interpret complex datasets generated by automated systems. For example, before automation, an analyst might spend hours manually analyzing logs for suspicious activity. Now, automated systems can identify these anomalies in real-time, allowing the analyst to focus on investigating the most critical threats and developing more effective security strategies.
Necessary Skills for Cybersecurity Professionals Working with Automated Systems
Skill Category | Specific Skill | Importance Level |
---|---|---|
Technical Skills | Data analysis and interpretation | High |
Technical Skills | Understanding of machine learning principles | High |
Technical Skills | Proficiency in scripting languages (e.g., Python) | Medium |
Technical Skills | Network security and system administration | High |
Soft Skills | Problem-solving and critical thinking | High |
Soft Skills | Communication and collaboration | Medium |
Soft Skills | Adaptability and continuous learning | High |
Security Expertise | Threat intelligence and vulnerability management | High |
Security Expertise | Incident response and forensics | High |
Future Trends in Automated Cybersecurity: Automation Is Key To Strengthen Cybersecurity Says Ibm
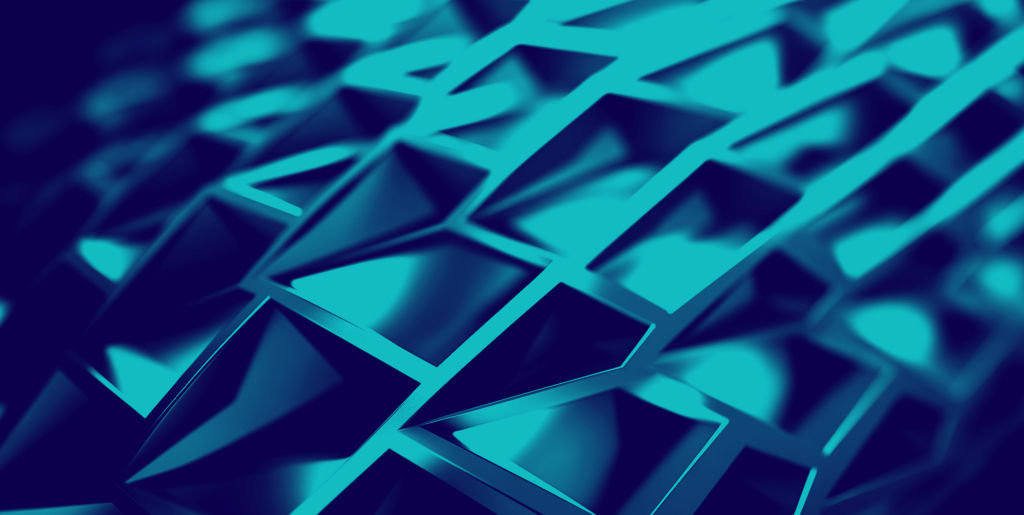
The field of automated cybersecurity is rapidly evolving, driven by the increasing sophistication of cyber threats and the need for more efficient and effective defense mechanisms. New technologies and approaches are constantly emerging, promising to reshape how we protect our digital assets in the years to come. This section explores some of the key trends shaping the future of automated cybersecurity.
The convergence of artificial intelligence (AI), machine learning (ML), and big data analytics is fundamentally altering the landscape. This powerful combination allows for the development of more proactive and adaptive security systems capable of identifying and responding to threats in real-time, even those previously unknown.
Quantum Computing’s Impact on Automated Cybersecurity
The advent of quantum computing presents both significant opportunities and challenges for automated cybersecurity. While quantum computers possess the potential to break many currently used encryption algorithms, they also offer the possibility of developing entirely new, quantum-resistant cryptographic methods. This necessitates a proactive shift towards post-quantum cryptography and the development of automated systems capable of managing and implementing these new algorithms.
For example, the National Institute of Standards and Technology (NIST) is actively evaluating and standardizing post-quantum cryptographic algorithms, a crucial step in mitigating the future risks posed by quantum computers. The integration of these algorithms into automated security systems will be paramount.
Innovative Approaches to Automation in Cybersecurity
Several innovative approaches are currently under development, pushing the boundaries of automated cybersecurity. One notable example is the use of AI-powered threat hunting systems. These systems leverage advanced machine learning algorithms to proactively search for and identify malicious activity within networks, going beyond simple signature-based detection. Another area of significant advancement is the development of automated incident response systems, which can autonomously contain and remediate security breaches with minimal human intervention.
These systems utilize AI and ML to analyze the situation, identify the root cause, and execute appropriate countermeasures, significantly reducing the time and resources required to resolve incidents.
Blockchain Technology in Automated Cybersecurity
Blockchain technology, known for its decentralized and tamper-proof nature, offers a promising approach to enhancing the security and trustworthiness of automated cybersecurity systems. Blockchain can be used to create immutable logs of security events, providing a verifiable audit trail of all activities. This enhances transparency and accountability, making it more difficult for attackers to manipulate or conceal their actions.
Furthermore, blockchain can facilitate secure and efficient sharing of threat intelligence between organizations, allowing for a more collaborative and effective approach to cybersecurity. For instance, a distributed ledger could securely track software updates and patches, ensuring only verified and trusted versions are deployed, thus mitigating vulnerabilities.
Case Studies
Real-world examples showcasing the transformative impact of automation in bolstering cybersecurity defenses are abundant. This section will delve into two specific instances where automated solutions significantly improved security posture, highlighting the technologies employed and comparing outcomes with similar situations lacking automation.
Automated Threat Detection at a Major Financial Institution
This case study focuses on a large multinational bank that implemented a Security Information and Event Management (SIEM) system augmented with machine learning (ML) capabilities. Prior to automation, the bank relied heavily on manual analysis of security logs, a process that was time-consuming, prone to human error, and often resulted in delayed responses to security incidents. The new SIEM system, however, automatically collected and analyzed security logs from various sources, identifying patterns and anomalies indicative of malicious activity far more efficiently than human analysts could manage.
Specifically, the ML algorithms were trained to detect unusual login attempts, data exfiltration patterns, and other suspicious behaviors. The system was also integrated with automated response mechanisms, enabling it to automatically block malicious IP addresses, quarantine infected systems, and initiate incident response protocols.The impact was dramatic. The bank experienced a significant reduction in the time it took to detect and respond to security incidents, from an average of several hours to mere minutes.
The number of successful breaches also decreased substantially. In contrast, a similar-sized bank that continued to rely primarily on manual log analysis experienced significantly more breaches and suffered greater financial losses. The automated system’s ability to detect subtle anomalies that might have been missed by human analysts was a key factor in its success.
The automated SIEM system, coupled with machine learning, drastically reduced incident response times, lowered the number of successful breaches, and minimized financial losses compared to organizations relying on solely manual processes. This demonstrates the critical role of automation in achieving a robust security posture.
Automated Patch Management in a Global Retail Chain
A large international retail chain implemented an automated patch management system to address vulnerabilities in its vast network of stores and online platforms. Previously, patching was a manual, labor-intensive process, often resulting in delays and inconsistencies across the network. This left the company vulnerable to attacks targeting known vulnerabilities. The new system automated the entire patch management lifecycle, from vulnerability scanning and patch downloading to deployment and verification.
It utilized a combination of technologies including vulnerability scanners, patch management software, and configuration management tools. The system was designed to prioritize patching based on the severity of vulnerabilities and the criticality of the affected systems. Furthermore, it incorporated automated testing procedures to ensure that patches did not introduce new problems.The results were impressive. The retail chain achieved significantly improved patch compliance rates, reducing its overall vulnerability footprint.
The automated system ensured that critical patches were deployed quickly and consistently across all systems, minimizing the window of vulnerability. In contrast, before automation, the company faced frequent security incidents resulting from unpatched vulnerabilities, leading to significant downtime and financial losses. The automated system’s ability to prioritize and deploy patches effectively minimized these risks.
Automating the patch management process drastically improved patch compliance, reduced the company’s vulnerability footprint, and minimized the impact of security incidents. This case study highlights the efficiency and effectiveness of automation in mitigating risks associated with software vulnerabilities.
Summary
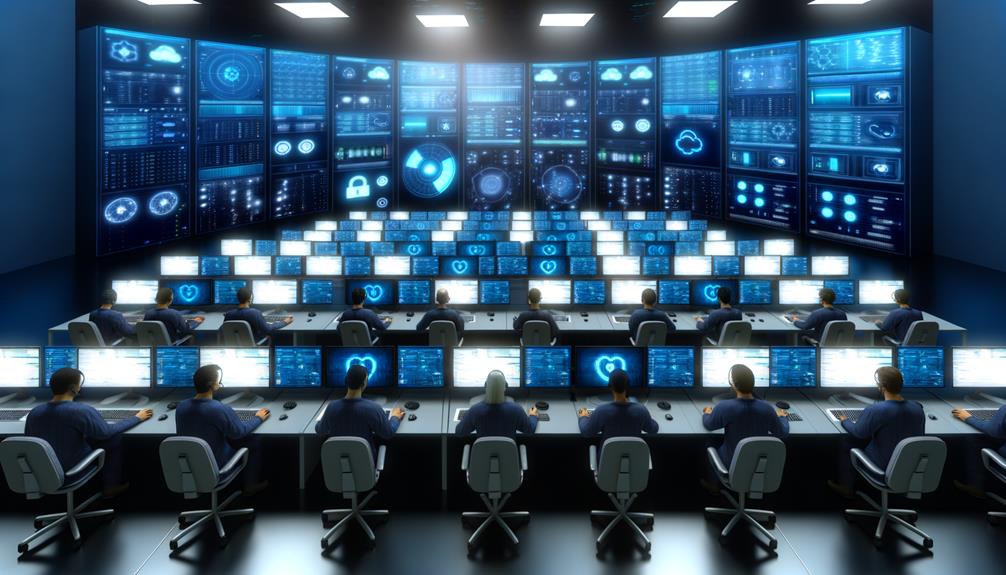
In conclusion, while IBM’s assertion that automation is key to strengthening cybersecurity is undeniably true, it’s crucial to remember that automation is a tool, not a silver bullet. A robust cybersecurity strategy requires a blend of cutting-edge technology and skilled human expertise. By embracing automation strategically and addressing its limitations, organizations can significantly enhance their security posture and better protect themselves against the ever-evolving landscape of cyber threats.
The future of cybersecurity is undoubtedly automated, but it’s a future where humans remain vital partners in the fight.
Detailed FAQs
What are the biggest risks of over-reliance on automated cybersecurity?
Over-reliance can lead to automation bias (trusting automated systems blindly), neglecting human oversight, and creating new vulnerabilities if the automation itself is compromised.
How can companies ensure human expertise remains relevant in an automated environment?
Invest in training and upskilling programs focusing on areas like AI/ML understanding, threat analysis, incident response, and security architecture design. Focus should shift from repetitive tasks to strategic oversight and complex problem-solving.
What’s the role of blockchain in automated cybersecurity?
Blockchain can enhance security and trustworthiness by providing immutable records of security events, improving auditability and transparency of automated security processes.
Can automation completely replace human cybersecurity professionals?
No. While automation handles many routine tasks, human expertise is essential for strategic decision-making, complex threat analysis, ethical considerations, and adapting to new and unforeseen threats.