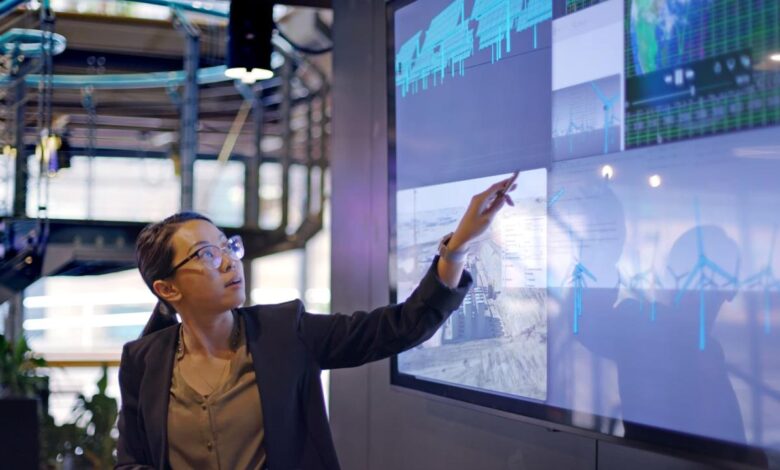
Best Data Analytics Degrees Your Path to a Rewarding Career
Best data analytics degrees are more than just academic pursuits; they’re your ticket to a future brimming with exciting opportunities and impactful work. In a world increasingly driven by data, skilled analysts are in high demand, offering lucrative salaries and the chance to make a real difference across diverse industries. This post dives deep into everything you need to know about choosing the right data analytics degree, from program types and curriculum comparisons to career prospects and financial aid options.
Get ready to unlock the power of data!
We’ll explore various degree types, from undergraduate programs to specialized master’s and certificate courses, comparing online and on-campus options. We’ll then delve into curriculum specifics, highlighting the strengths and weaknesses of different university programs. Finally, we’ll discuss career paths, salary expectations, and the application process, ensuring you’re fully equipped to make an informed decision about your future in this rapidly evolving field.
Types of Data Analytics Degrees
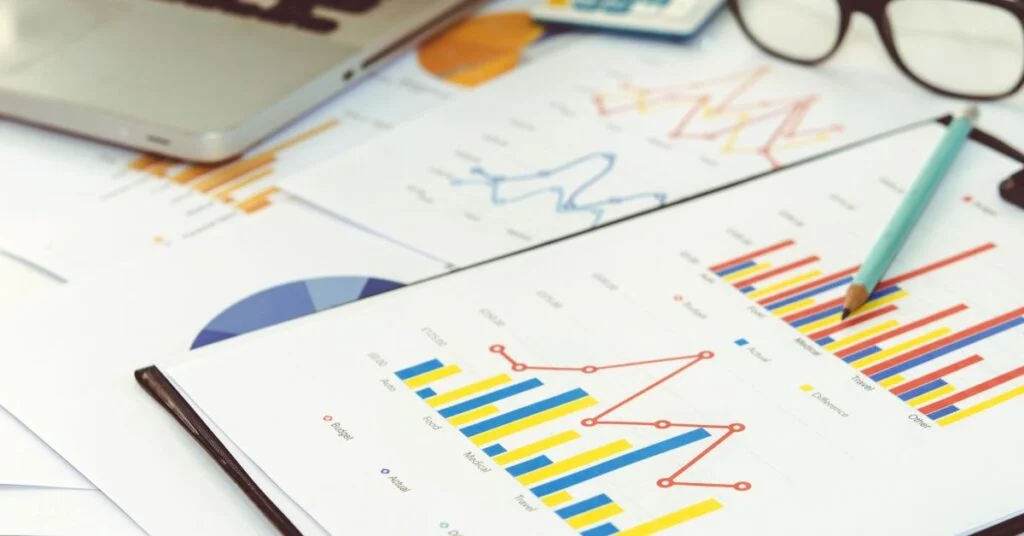
Choosing the right data analytics degree is a crucial step in launching a successful career in this rapidly growing field. The options available vary significantly in terms of duration, coursework, and ultimately, the career paths they open up. Understanding these differences is key to making an informed decision.
Data Analytics Degree Options
The data analytics field offers a range of educational pathways to suit diverse learning styles and career aspirations. Below is a table summarizing the common types of data analytics degrees.
Degree Type | Typical Duration | Common Coursework | Career Paths |
---|---|---|---|
Bachelor’s Degree (BSc/BA) in Data Analytics or a related field (e.g., Computer Science, Statistics) | 4 years | Mathematics, Statistics, Programming (Python, R, SQL), Data Visualization, Database Management, Machine Learning Fundamentals, Data Mining | Data Analyst, Junior Data Scientist, Business Analyst, Data Engineer (entry-level roles) |
Master’s Degree (MSc/MA) in Data Analytics or a related field | 1-2 years | Advanced Statistics, Machine Learning, Deep Learning, Big Data Technologies (Hadoop, Spark), Data Warehousing, Data Visualization, Predictive Modeling, Business Intelligence | Data Scientist, Data Analyst (senior roles), Machine Learning Engineer, Business Intelligence Analyst, Data Architect |
Graduate Certificate in Data Analytics | 6-12 months | Specific skills-focused courses such as data visualization, SQL, Python for data analysis, and introductory machine learning. Often tailored to professionals already in the workforce. | Upskilling for current roles, transition to data-focused roles within existing industries. |
PhD in Data Analytics or a related field | 4-7 years | Advanced research methodologies, specialized areas within data analytics (e.g., natural language processing, computer vision), significant research project and dissertation. | Research Scientist, University Professor, Lead Data Scientist in research-intensive organizations. |
Online vs. On-Campus Degree Programs
The choice between online and on-campus learning significantly impacts the learning experience. Consider these key differences:
Choosing between online and on-campus learning depends heavily on individual learning styles and preferences. Both offer unique advantages.
- Flexibility and Location: Online programs offer unmatched flexibility in terms of scheduling and location. Students can learn at their own pace and from anywhere with an internet connection. On-campus programs require consistent attendance at a specific location.
- Interaction and Collaboration: On-campus programs provide more opportunities for in-person interaction with professors and peers, fostering collaboration and networking. Online programs often utilize online forums and virtual meeting spaces to facilitate interaction, but the experience can be less immediate.
- Structure and Discipline: On-campus programs typically have a more structured learning environment, requiring regular attendance and deadlines. Online programs require greater self-discipline and time management skills.
- Cost: While tuition can vary, online programs may sometimes offer lower overall costs due to the lack of commuting expenses and on-campus housing. However, this can vary significantly between institutions.
- Resources and Access: On-campus programs offer access to on-site libraries, labs, and other resources. Online programs often provide access to digital libraries and online resources, but the availability and quality can differ.
Skills Acquired in Each Degree Type
Each data analytics degree type equips students with a unique set of skills. A Bachelor’s degree provides a foundational understanding of core concepts, while a Master’s degree delves into more advanced techniques and specializations. Certificate programs focus on specific skill sets, and PhD programs emphasize research and advanced knowledge.
The specific skills acquired are closely tied to the coursework and the level of the program. For example, a bachelor’s degree might focus on foundational programming skills in R or Python, while a master’s program would build upon this by teaching students advanced techniques in machine learning or deep learning.
Curriculum Comparison Across Institutions
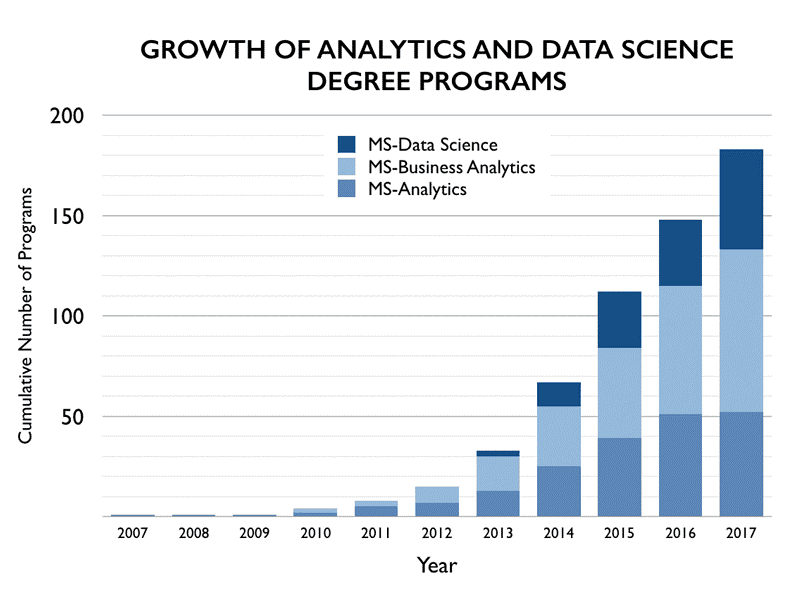
Choosing the right data analytics degree requires careful consideration of curriculum design. Top programs often share core components, but significant differences exist in their emphasis, elective offerings, and specialization tracks. This comparison highlights key distinctions between three leading universities to aid in your decision-making process.
Curriculum Comparison of Three Leading Universities
Below is a comparison of the curricula of three hypothetical top-ranked data analytics programs (University A, University B, and University C). Note that specific course titles and elective options will vary from year to year and should be verified directly with the universities. This comparison serves as a general illustration of curriculum differences.
University | Required Courses | Elective Options | Specializations Offered |
---|---|---|---|
University A | Linear Algebra, Probability & Statistics, Database Management, Data Mining, Machine Learning, Data Visualization, Big Data Analytics, Capstone Project | Advanced Machine Learning, Deep Learning, Natural Language Processing, Time Series Analysis, Cloud Computing, Business Analytics | Business Analytics, Machine Learning Engineering |
University B | Calculus, Probability & Statistics, Database Systems, Data Wrangling, Regression Analysis, Predictive Modeling, Data Visualization, Capstone Project | Spatial Data Analysis, Causal Inference, Data Ethics, Social Network Analysis, Optimization Techniques, Data Security | Data Science, Computational Social Science |
University C | Linear Algebra, Statistics, Database Management, Data Mining, Programming (Python/R), Data Visualization, Business Intelligence, Capstone Project | Advanced Data Visualization, Actuarial Science, Financial Modeling, Marketing Analytics, Healthcare Analytics, Cybersecurity Analytics | Financial Analytics, Healthcare Analytics, Marketing Analytics |
Pedagogical Approaches
University A emphasizes a strong foundation in mathematical and statistical concepts, preparing students for advanced machine learning roles. University B incorporates a broader social science perspective, exploring the ethical and societal implications of data analysis. University C focuses on practical applications within specific business sectors, offering specializations tailored to industry demands. These different pedagogical approaches reflect varying career goals and research interests.
Comparative Chart: Curriculum Strengths and Weaknesses
This chart summarizes the strengths and weaknesses of each program’s curriculum, based on the previously described information. Remember that “strength” and “weakness” are relative and depend on individual student goals.
University | Strengths | Weaknesses |
---|---|---|
University A | Strong foundation in mathematics and machine learning; well-defined specializations; industry-relevant curriculum. | Limited focus on social science and ethical considerations; fewer options for students interested in non-technical specializations. |
University B | Broader interdisciplinary approach; strong emphasis on data ethics; unique specializations in social sciences. | May lack depth in certain technical areas compared to University A; fewer purely technical specializations. |
University C | Strong focus on practical applications in various business sectors; diverse specialization options; business-oriented curriculum. | May require additional coursework for students seeking advanced theoretical knowledge in mathematics or statistics. |
Career Prospects and Salary Expectations: Best Data Analytics Degrees
Landing a data analytics job after graduation is often a rewarding experience, both professionally and financially. The field is experiencing significant growth, offering a wide array of career paths and competitive salaries. Understanding the potential earning power and job market trends is crucial for prospective students considering a data analytics degree.
Average Starting Salaries for Data Analytics Graduates
The starting salary for a data analytics graduate varies considerably depending on several factors, including the type of degree earned (Bachelor’s, Master’s), the specific skills acquired, and geographic location. Generally, Master’s degree holders command higher salaries than Bachelor’s degree holders due to their advanced knowledge and expertise. Location also plays a significant role; major tech hubs and financial centers tend to offer higher salaries than smaller cities.
The following table presents estimated average starting salaries, acknowledging that these are broad averages and individual salaries can vary widely.
Degree Type | Location | Average Starting Salary (USD) |
---|---|---|
Bachelor’s in Data Analytics | San Francisco, CA | $75,000 – $90,000 |
Bachelor’s in Data Analytics | Chicago, IL | $60,000 – $75,000 |
Master’s in Data Analytics | New York, NY | $90,000 – $110,000 |
Master’s in Data Analytics | Austin, TX | $80,000 – $100,000 |
*Note: Salary data is based on various industry reports and surveys conducted in recent years and should be considered estimates.*
Projected Growth of Data Analytics Jobs
The demand for data analysts is rapidly increasing across various sectors. The Bureau of Labor Statistics projects significant growth in jobs related to data science and analytics in the coming years. For example, the healthcare industry is increasingly relying on data analytics for improving patient care, managing costs, and developing new treatments. Similarly, the financial sector utilizes data analytics for risk management, fraud detection, and algorithmic trading.
E-commerce companies heavily depend on data analytics for understanding customer behavior, personalizing recommendations, and optimizing marketing campaigns. The growth in these and other sectors translates into a robust job market for data analytics graduates.
Career Paths for Data Analytics Graduates
A data analytics degree opens doors to a wide range of career paths. Graduates can pursue roles such as Data Analyst, Business Analyst, Data Scientist, Machine Learning Engineer, Data Engineer, and Database Administrator. The specific career path chosen often depends on the individual’s interests and the skills developed during their education. For instance, a graduate with a strong programming background might pursue a career as a Data Scientist, while someone with a keen interest in business strategy might become a Business Analyst.
The versatility of a data analytics education makes it a highly valuable asset in today’s data-driven world. Many graduates also find opportunities in specialized fields such as marketing analytics, financial analytics, or healthcare analytics, further demonstrating the breadth of career options available.
Admission Requirements and Application Process
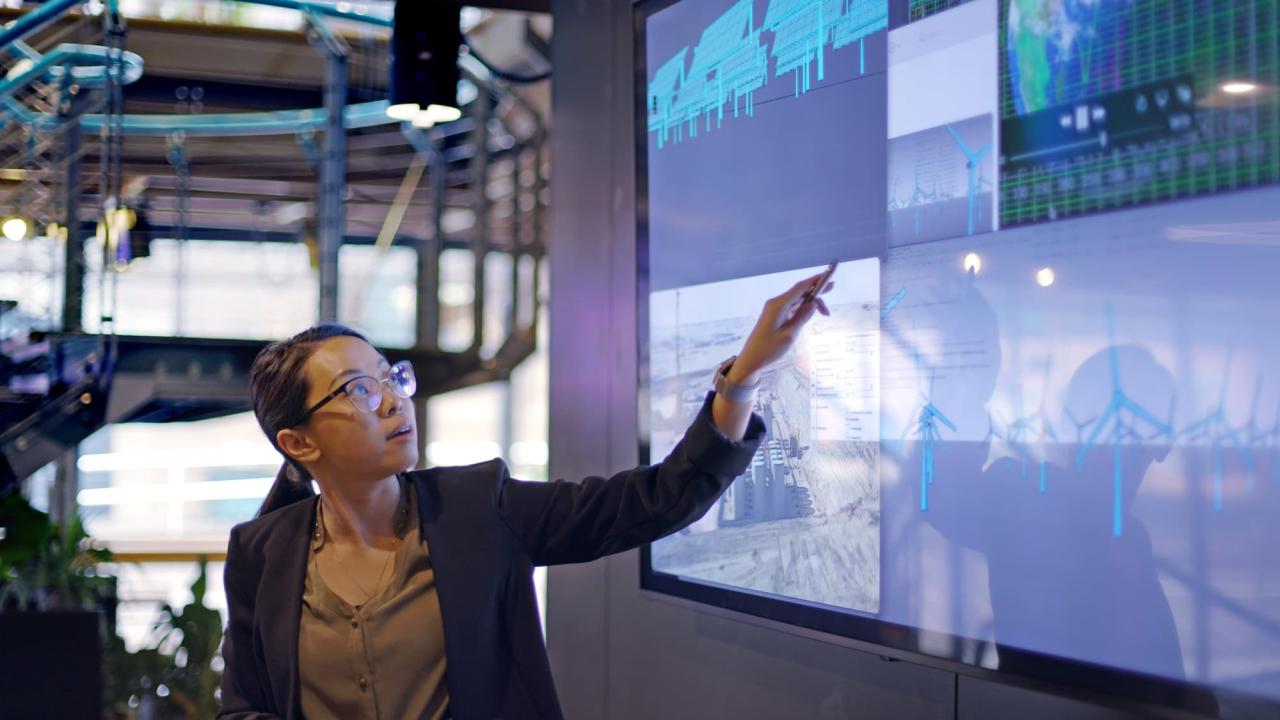
Landing your dream data analytics degree requires careful planning and a thorough understanding of the admissions process. Each program has its own specific requirements, but there are common threads that run through most applications. Knowing what to expect and how to present yourself effectively can significantly increase your chances of acceptance.The application process, while potentially daunting, is manageable with proper preparation.
Understanding the requirements and deadlines is the first step towards a successful application.
Typical Admission Requirements
Data analytics programs typically require a strong academic background, demonstrated through a high GPA and, in some cases, standardized test scores. Many programs also have prerequisite coursework, particularly in mathematics and statistics. The specific requirements vary considerably depending on the institution and the program’s level (e.g., undergraduate vs. master’s). A bachelor’s degree is usually a prerequisite for master’s programs.
For example, a competitive undergraduate program might require a minimum GPA of 3.0 or higher, while a master’s program might require a 3.5 GPA or higher. Standardized tests, such as the GRE or GMAT, are sometimes required for graduate programs, but this is becoming less common as more programs emphasize practical experience and portfolio review. Prerequisites often include courses in calculus, linear algebra, and introductory statistics.
Some programs may also require programming experience, particularly in languages like Python or R.
Application Process and Deadlines
The application process usually involves submitting an online application, transcripts, letters of recommendation, and a personal statement. There are often application fees associated with the process. Deadlines vary significantly depending on the institution and the program, with many programs having deadlines in the winter or spring for fall admission. It’s crucial to check the specific deadlines on each program’s website.
Early applications are often encouraged, as they may improve your chances of admission and securing financial aid. Late applications may not be considered, or they might be subject to reduced consideration.
Strong Application Materials: Personal Statement
A compelling personal statement is crucial for showcasing your qualifications and aspirations. It should highlight your relevant skills, experiences, and motivations for pursuing a data analytics degree. A strong personal statement goes beyond simply listing accomplishments; it demonstrates a deep understanding of the field and a clear articulation of your career goals. For instance, instead of saying “I am good at math,” a strong statement might detail a specific project where you used statistical analysis to solve a real-world problem, highlighting the analytical skills and problem-solving abilities you employed.
A compelling narrative that connects your past experiences to your future ambitions will resonate with the admissions committee.
Strong Application Materials: Letters of Recommendation
Letters of recommendation provide external validation of your abilities and character. It’s essential to request letters from individuals who can speak to your skills and potential in a meaningful way. These individuals should ideally be professors, supervisors, or mentors who can provide specific examples of your work ethic, analytical skills, and problem-solving abilities. Choosing recommenders who know you well and can speak to your strengths will strengthen your application.
Giving your recommenders ample time to write strong letters is crucial; providing them with your resume, personal statement, and a list of your accomplishments can assist them in writing a more effective recommendation.
Funding and Financial Aid Options
Pursuing a data analytics degree is a significant investment, but numerous funding options can help make it financially feasible. Understanding these options and the application process is crucial for prospective students. This section Artikels potential funding sources and provides a framework for budgeting your education expenses.
Securing funding for your data analytics education often involves a multi-pronged approach, combining several funding streams to cover the total cost. This can include scholarships, grants, loans, and even employer sponsorship in some cases. The application process for each funding source varies, but generally involves completing detailed applications, providing supporting documentation, and potentially undergoing an interview.
Potential Funding Sources
Several avenues exist for securing financial aid for a data analytics degree. These sources vary in their eligibility requirements, application processes, and award amounts. Careful research is essential to identify the most suitable options for your individual circumstances.
- Scholarships: Many organizations, universities, and private companies offer scholarships specifically for students pursuing STEM fields, including data analytics. These scholarships are often merit-based, rewarding academic excellence or specific skills. Some examples include scholarships offered by professional organizations like the Institute of Electrical and Electronics Engineers (IEEE) or specific technology companies. These scholarships typically require a strong academic record, compelling essays, and letters of recommendation.
- Grants: Grants, unlike loans, do not need to be repaid. Government agencies, non-profit organizations, and private foundations offer grants based on financial need or specific criteria. The application process for grants can be competitive and requires meticulous documentation of financial need and alignment with the grant’s objectives. Federal grants like Pell Grants are a common example, available to students demonstrating significant financial need.
- Loans: Federal student loans and private student loans provide funding for educational expenses. Federal loans often have more favorable interest rates and repayment options than private loans. However, it’s important to understand the terms and conditions of any loan before accepting it, as borrowing large sums can significantly impact your post-graduation finances. Responsible borrowing is key to avoiding future financial strain.
- Employer Sponsorship: Some employers offer tuition reimbursement or assistance programs to employees pursuing further education relevant to their roles. This is particularly true for companies with a high demand for data analytics professionals. Check with your current or prospective employer to explore this possibility. This often requires a commitment to remain employed with the company for a certain period after completing your degree.
Applying for Financial Aid
The application process for financial aid can be complex and time-consuming. It typically involves completing the Free Application for Federal Student Aid (FAFSA) for federal aid and separate applications for institutional and private scholarships and grants. Careful organization and early planning are essential to ensure a smooth application process.
- Complete the FAFSA: The FAFSA is the primary application for federal student aid, including grants and loans. It requires detailed financial information from you and your parents (if you are a dependent student). Submitting the FAFSA early is crucial, as funding is often awarded on a first-come, first-served basis.
- Apply for Institutional Aid: Many universities offer their own scholarships and grants based on merit and financial need. Check your university’s financial aid website for application deadlines and requirements.
- Search for External Scholarships and Grants: Numerous external organizations offer scholarships and grants. Utilize online scholarship databases and search engines to find opportunities that align with your profile and academic goals. Each scholarship or grant will have its own application process and requirements.
- Apply for Private Loans: If you still need additional funding after exploring other options, consider applying for private student loans. Compare interest rates and repayment terms from different lenders before making a decision.
Sample Education Budget
Creating a realistic budget is vital for managing your education expenses. This sample budget Artikels potential costs associated with a data analytics degree. Remember that these are estimates, and your actual costs may vary based on your institution, living expenses, and lifestyle.
Expense Category | Estimated Annual Cost |
---|---|
Tuition and Fees | $20,000 – $40,000 |
Books and Supplies | $1,000 – $2,000 |
Housing (on-campus or off-campus) | $8,000 – $15,000 |
Food | $4,000 – $8,000 |
Transportation | $1,000 – $3,000 |
Personal Expenses | $2,000 – $5,000 |
Total Estimated Annual Cost: $36,000 – $73,000 (This is a wide range to reflect the variability in program costs and living expenses across different locations and lifestyles).
Specializations within Data Analytics
The field of data analytics, while encompassing a broad range of skills and applications, is increasingly specialized. Understanding these specializations is crucial for students choosing a degree path and for professionals seeking career advancement. Different specializations require different skill sets and lead to distinct career opportunities, each with its own salary expectations and growth potential.
Business Analytics
Business analytics focuses on applying data analysis techniques to solve business problems. This specialization emphasizes the practical application of analytical methods to improve decision-making within organizations. Professionals in this area often work with large datasets to identify trends, predict future outcomes, and optimize business processes. Skills in statistical modeling, data mining, and business intelligence tools are highly valued.
Career paths include business analyst, market research analyst, and management consultant. A strong understanding of business principles and the ability to communicate complex findings to non-technical audiences are key differentiators. For example, a business analyst might use regression analysis to predict sales based on marketing spend, then present those findings to the marketing team to optimize their budget allocation.
Data Science
Data science is a more computationally intensive and mathematically rigorous specialization than business analytics. It involves extracting knowledge and insights from structured and unstructured data using advanced algorithms and machine learning techniques. Data scientists often work with larger and more complex datasets than business analysts, requiring expertise in programming languages like Python or R, and familiarity with various machine learning models.
Career paths include data scientist, machine learning engineer, and data architect. A recent example of data science in action is the use of natural language processing (NLP) to analyze customer reviews and identify areas for product improvement. This involves building complex models to understand sentiment and extract key themes from vast amounts of text data.
Data Visualization
Data visualization focuses on communicating data insights through compelling and effective visual representations. This specialization requires strong artistic and design skills in addition to analytical abilities. Data visualization specialists create charts, graphs, dashboards, and other visual aids to help others understand complex data quickly and easily. They need proficiency in data visualization tools like Tableau or Power BI, as well as a keen eye for design and an understanding of how to communicate effectively with different audiences.
Career paths include data visualization specialist, data journalist, and UX designer. A compelling example would be the use of interactive maps to show the spread of a disease, allowing public health officials to quickly identify hotspots and allocate resources effectively.
Data Engineering
Data engineering focuses on the infrastructure and processes required to collect, store, and manage large datasets. Data engineers design, build, and maintain the systems that support data analysis. They work with databases, cloud computing platforms, and big data technologies like Hadoop and Spark. This specialization requires strong programming skills and a deep understanding of database management systems. Career paths include data engineer, database administrator, and cloud architect.
A real-world example is the development of a data pipeline that collects data from various sources (e.g., social media, sensors, databases) and processes it to prepare it for analysis by data scientists or business analysts.
Relationship between Specializations
The following flowchart illustrates the interconnectedness of these specializations:
Data Analytics | ----------------------------------------------------- | | | Data Science Data Visualization Business Analytics | | | (Machine Learning, (Tableau, Power BI, (Statistical Modeling, Deep Learning) Data Storytelling) Business Intelligence) | | | | | | V V V Data Engineering Data Interpretation Decision Making & Strategy
Industry Trends and Future of Data Analytics
The field of data analytics is experiencing explosive growth, driven by the ever-increasing volume of data generated globally and the advancements in technology capable of processing and interpreting it.
This rapid evolution presents both exciting opportunities and significant challenges for aspiring and current data analytics professionals. Understanding the key trends shaping the industry is crucial for navigating this dynamic landscape.
The convergence of big data, artificial intelligence (AI), and machine learning (ML) is fundamentally reshaping data analytics. Big data, characterized by its volume, velocity, variety, veracity, and value (the five Vs), necessitates sophisticated tools and techniques to extract meaningful insights. AI and ML algorithms are at the forefront of this revolution, enabling the automation of complex analytical processes and the discovery of previously hidden patterns.
This synergistic relationship is pushing the boundaries of what’s possible in data-driven decision-making.
Big Data and its Impact on Data Analytics
Big data’s influence on data analytics is undeniable. The sheer volume of data available – from social media interactions to sensor readings in industrial settings – necessitates the use of distributed computing frameworks like Hadoop and Spark to process and analyze information efficiently. This has led to the development of specialized roles focusing on big data engineering and management, complementing traditional data analyst positions.
For example, companies like Netflix leverage big data analytics to personalize recommendations for their users, significantly impacting user engagement and subscription retention. The analysis of massive datasets allows for hyper-personalization, a trend expected to grow significantly in the coming years.
The Rise of Artificial Intelligence and Machine Learning
AI and ML are no longer futuristic concepts; they are integral components of modern data analytics. These technologies empower analysts to automate tasks, build predictive models with greater accuracy, and uncover complex relationships within datasets. Machine learning algorithms, such as deep learning neural networks, are particularly effective in handling unstructured data like images and text, opening up new avenues for analysis in areas like image recognition and natural language processing.
For instance, in the healthcare industry, AI-powered diagnostic tools are being developed to analyze medical images and identify potential diseases with higher accuracy than human experts in some cases.
Future Job Market for Data Analytics Professionals
The demand for skilled data analytics professionals is projected to continue its upward trajectory. The Bureau of Labor Statistics anticipates significant growth in related occupations, driven by the increasing reliance on data-driven decision-making across all sectors. While specific job titles may evolve, the core skills – data manipulation, statistical analysis, visualization, and problem-solving – will remain highly sought after.
The future will likely see a greater emphasis on roles requiring expertise in AI, ML, and cloud computing, as well as strong communication skills to effectively convey complex insights to non-technical stakeholders. Companies are increasingly seeking data scientists and analysts who can not only analyze data but also communicate their findings effectively to influence strategic business decisions.
Innovative Applications of Data Analytics Across Industries, Best data analytics degrees
Data analytics is transforming industries in remarkable ways. In finance, it’s used for fraud detection, risk management, and algorithmic trading. In retail, it powers personalized recommendations, inventory optimization, and targeted marketing campaigns. In manufacturing, predictive maintenance using sensor data minimizes downtime and optimizes production processes. The transportation industry utilizes data analytics for route optimization, traffic prediction, and autonomous vehicle development.
These are just a few examples; the applications are virtually limitless, and new innovative uses emerge constantly. For example, the use of predictive analytics in agriculture helps optimize irrigation and fertilization, leading to increased crop yields and reduced resource consumption.
Epilogue
Embarking on a journey into data analytics is an exciting and rewarding prospect. By carefully considering the various degree options, understanding the curriculum nuances, and planning for the financial aspects, you can confidently choose the path that best aligns with your aspirations and career goals. Remember, the world of data is vast and constantly evolving, so embrace the challenges, hone your skills, and prepare to make a significant impact in your chosen field.
Good luck on your data analytics adventure!
Answers to Common Questions
What is the difference between a data analyst and a data scientist?
Data analysts typically focus on interpreting existing data to inform business decisions, while data scientists often build predictive models and develop new analytical methods.
Are there any prerequisites for data analytics programs?
Prerequisites vary, but many programs require a strong foundation in mathematics and statistics. Some may also require programming experience.
How long does it take to complete a data analytics degree?
This depends on the degree type. Bachelor’s degrees typically take 4 years, while Master’s degrees can take 1-2 years, and certificates can be completed in a few months to a year.
Can I get financial aid for a data analytics degree?
Yes, many funding options exist, including scholarships, grants, loans, and employer-sponsored tuition assistance. Check with your chosen university’s financial aid office for details.