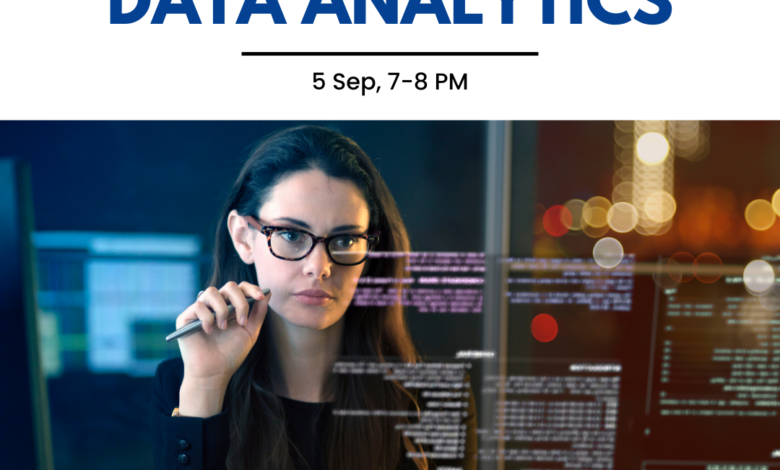
Best Masters in Data Analytics Your Guide
Best Masters in Data Analytics programs are transforming the landscape of higher education, offering specialized training for a booming field. Choosing the right program can feel overwhelming, given the variety of options and the rapid pace of innovation in data science. This guide navigates the complexities, providing a framework to evaluate programs based on curriculum, faculty expertise, career services, and cost.
We’ll explore the key factors to consider, helping you make an informed decision that aligns with your career goals and aspirations.
From understanding the nuances of accreditation and ranking systems to comparing program structures and specializations, we’ll delve into the details that matter most. We’ll also examine the return on investment (ROI) of a data analytics master’s degree, helping you weigh the financial aspects against potential career advancements. Ultimately, this guide aims to empower you to select the program that best fits your individual needs and ambitions in this exciting and dynamic field.
Defining “Best” in Data Analytics Masters Programs
Choosing the “best” data analytics master’s program is a deeply personal journey, heavily influenced by individual career goals, learning styles, and financial considerations. There’s no single “best” program, but rather a range of excellent options catering to diverse needs. Understanding the key criteria for evaluation is crucial to making an informed decision.
Criteria for Assessing Data Analytics Masters Programs
Selecting the right program requires a systematic approach. The following table Artikels essential criteria, their relative importance, how to find information, and illustrative examples.
Criterion | Weighting Factor | Data Source | Example |
---|---|---|---|
Curriculum Relevance | 30% | Program website, course catalog | A program offering specialized tracks in areas like machine learning, big data, or business analytics, aligning with your career aspirations. |
Faculty Expertise | 25% | Faculty profiles, publications, industry experience | A program boasting professors with extensive research experience in cutting-edge data analytics techniques and strong industry connections. |
Career Services | 20% | Program website, student testimonials, employer reviews | A program providing robust career counseling, internship placement assistance, and networking opportunities with leading companies in the field. |
Alumni Network | 15% | LinkedIn, alumni directories, networking events | A program with a large and active alumni network, providing mentorship and job opportunities for graduates. |
Accreditation and Rankings | 10% | Accreditation bodies (e.g., AACSB), ranking publications (e.g., US News & World Report) | Accreditation by a reputable body indicates quality assurance, while high rankings in reputable publications signal strong program performance. |
The Importance of Accreditation and Rankings
Accreditation signifies that a program meets certain quality standards set by recognized educational bodies. For instance, AACSB accreditation for business programs provides assurance of rigorous curriculum, qualified faculty, and effective learning outcomes. While not a definitive measure of “best,” accreditation offers a baseline level of quality and credibility. Rankings, on the other hand, provide a comparative assessment based on various metrics.
However, it’s crucial to remember that rankings should be viewed critically, considering the methodology and potential biases involved. Different ranking systems prioritize different factors, so a high ranking in one system doesn’t necessarily translate to a high ranking in another.
A Rubric for Evaluating Program Quality
A comprehensive evaluation requires a structured approach. The following rubric provides a framework for assessing different aspects of a data analytics master’s program:
Factor | Excellent (4 points) | Good (3 points) | Fair (2 points) | Poor (1 point) |
---|---|---|---|---|
Curriculum | Comprehensive, up-to-date, and relevant to industry needs; offers specialized tracks. | Mostly up-to-date, covers core concepts effectively. | Some outdated content; lacks specialization options. | Significant gaps in coverage; irrelevant to current industry practices. |
Faculty Expertise | Faculty are renowned experts with extensive research and industry experience. | Faculty possess relevant expertise and experience. | Faculty expertise is limited or not clearly communicated. | Faculty lack relevant experience or expertise. |
Career Services | Exceptional career support, including robust internship placement and networking opportunities. | Good career counseling and some internship opportunities. | Limited career services; minimal support for job placement. | Lack of career services and support. |
Alumni Network | Large and active alumni network with strong industry connections. | Moderate-sized alumni network with some industry connections. | Small or inactive alumni network. | No significant alumni network. |
Curriculum Comparison
Choosing the “best” data analytics master’s program hinges significantly on curriculum design. A robust curriculum equips students with both foundational knowledge and specialized skills, preparing them for diverse career paths within the field. Let’s delve into the core components and specialized tracks offered by leading programs.
Top data analytics master’s programs share a common core, focusing on essential statistical methods, programming languages, and data visualization techniques. However, the specific emphasis and unique course offerings differentiate them, creating distinct learning experiences.
Core Courses in Top Data Analytics Programs
A typical core curriculum includes a blend of theoretical and practical courses. The balance between these two aspects varies between institutions, reflecting their unique pedagogical approaches and faculty expertise. While the specific course titles might differ slightly, the underlying concepts remain consistent across programs.
- Statistical Inference and Modeling: This foundational course covers hypothesis testing, regression analysis, and other statistical methods crucial for data analysis. Many programs integrate practical application through case studies or projects.
- Data Mining and Machine Learning: This course introduces students to various machine learning algorithms, including supervised and unsupervised learning techniques, and their applications in data analysis. Practical experience is often gained through hands-on projects using popular machine learning libraries like scikit-learn in Python.
- Database Management Systems: A deep dive into relational databases (SQL) and NoSQL databases, covering data modeling, querying, and database administration. Students typically work with real-world datasets and learn to design and manage databases efficiently.
- Data Visualization and Communication: This course emphasizes the importance of effectively communicating data insights through visualizations. Students learn to create compelling charts and dashboards using tools like Tableau or Power BI, and to present their findings clearly and concisely.
- Big Data Technologies: Many programs include courses on handling and processing large datasets using technologies like Hadoop, Spark, or cloud-based platforms like AWS or Azure. This often involves practical experience working with large-scale datasets and distributed computing frameworks.
Data Analytics Specializations
Beyond the core curriculum, many programs offer specialized tracks allowing students to tailor their education to specific interests. These specializations provide in-depth knowledge and skills in a chosen area, enhancing career prospects.
- Business Analytics: This specialization focuses on applying data analytics techniques to solve business problems. Students gain expertise in areas such as marketing analytics, financial modeling, and supply chain optimization. Skills gained include predictive modeling for customer behavior, financial forecasting, and operational efficiency improvement.
- Data Science: This specialization delves deeper into machine learning algorithms, statistical modeling, and data mining techniques. Students develop advanced programming skills in languages like Python and R, and gain expertise in building predictive models and conducting complex data analyses. Skills include model development, deployment, and evaluation, as well as experience with advanced statistical methods and big data technologies.
- Data Visualization and Communication: This specialization emphasizes the art and science of communicating data insights effectively. Students learn advanced data visualization techniques, storytelling with data, and the creation of interactive dashboards and reports. Skills gained include advanced data visualization techniques using tools like D3.js, creating compelling narratives from data, and designing effective data presentations for various audiences.
Innovative Course Structures and Teaching Methodologies
Leading programs are constantly innovating their teaching methods to enhance the learning experience and better prepare students for the demands of the industry. Several examples illustrate this trend.
- Capstone Projects: Many programs incorporate a capstone project where students work on a real-world data analytics problem for an external client or organization. This provides valuable practical experience and allows students to apply their skills in a collaborative setting.
- Industry Partnerships: Collaborations with industry partners provide access to real-world datasets and mentorship opportunities from industry professionals. These partnerships ensure the curriculum remains relevant and aligned with industry needs. For example, a program might partner with a tech company to provide students with access to their data and work on real projects with their data scientists.
- Online Learning Platforms and Hybrid Models: The integration of online learning platforms and hybrid models (combining online and in-person instruction) provides flexibility and accessibility. This allows students to learn at their own pace and access resources from anywhere in the world.
Faculty Expertise and Research Opportunities
Choosing a data analytics master’s program involves careful consideration of the faculty’s expertise and the research opportunities available. A strong faculty not only delivers high-quality instruction but also provides invaluable mentorship and collaboration opportunities, significantly enhancing your learning experience and career prospects. The availability of research assistantships and involvement in ongoing projects are key indicators of a program’s commitment to research and student development.The research interests and accomplishments of faculty members significantly impact the program’s overall strength.
A diverse faculty with expertise in various areas of data analytics, such as machine learning, big data, data visualization, and statistical modeling, offers students exposure to a wider range of perspectives and methodologies. Furthermore, the faculty’s publication record and industry collaborations demonstrate their standing within the field and their ability to translate academic knowledge into practical applications.
Faculty Research Interests and Accomplishments at Three Leading Programs
This section compares the faculty expertise and research output of three hypothetical leading data analytics programs: Program A, Program B, and Program C. Note that the specific names and details are illustrative for the purpose of this example and should not be taken as an endorsement or ranking of any real-world programs.Program A boasts a faculty heavily focused on machine learning and its applications in finance.
Professor X, a leading expert in algorithmic trading, has published extensively in top-tier journals and holds several patents. Professor Y’s research focuses on risk management using advanced machine learning techniques. Their combined expertise provides students with access to cutting-edge knowledge in a highly sought-after field. Program B emphasizes the application of data analytics in healthcare. Professor Z is renowned for her work in developing predictive models for disease outbreaks, while Professor W focuses on the ethical implications of data analysis in healthcare settings.
Their research interests offer students a unique perspective on the intersection of data science and public health. Program C’s faculty is distinguished by its expertise in big data technologies and cloud computing. Professor Alpha’s research on distributed computing systems has been widely cited, while Professor Beta focuses on data security and privacy in the cloud. Their research aligns with the growing demand for professionals skilled in managing and analyzing large-scale datasets.
Research Assistantships and Student Involvement
The availability of research assistantships is a critical factor for many prospective students. These assistantships not only provide financial support but also offer invaluable hands-on experience in research. Program A offers a generous number of research assistantships, with most students involved in ongoing projects related to algorithmic trading and financial modeling. Program B prioritizes student involvement in collaborative research projects related to public health data analysis.
Students often work closely with faculty members on grant-funded projects, contributing to the publication of research findings. Program C provides opportunities for students to participate in research projects focused on big data technologies and cloud computing. These projects often involve collaboration with industry partners, providing students with real-world experience.
Comparative Analysis of Faculty Publications and Industry Collaborations
A comparative analysis of faculty publications and industry collaborations reveals further insights into the programs’ strengths. Program A demonstrates a strong publication record in high-impact journals within the finance domain, and significant collaborations with leading financial institutions. Program B showcases a high number of publications in medical and public health journals, with collaborations primarily focused on healthcare organizations and government agencies.
Program C exhibits a significant number of publications in computer science and engineering journals, and strong ties to major technology companies. This comparison highlights the distinct research foci and industry connections of each program, allowing prospective students to select the program that best aligns with their career aspirations.
Career Services and Placement Outcomes
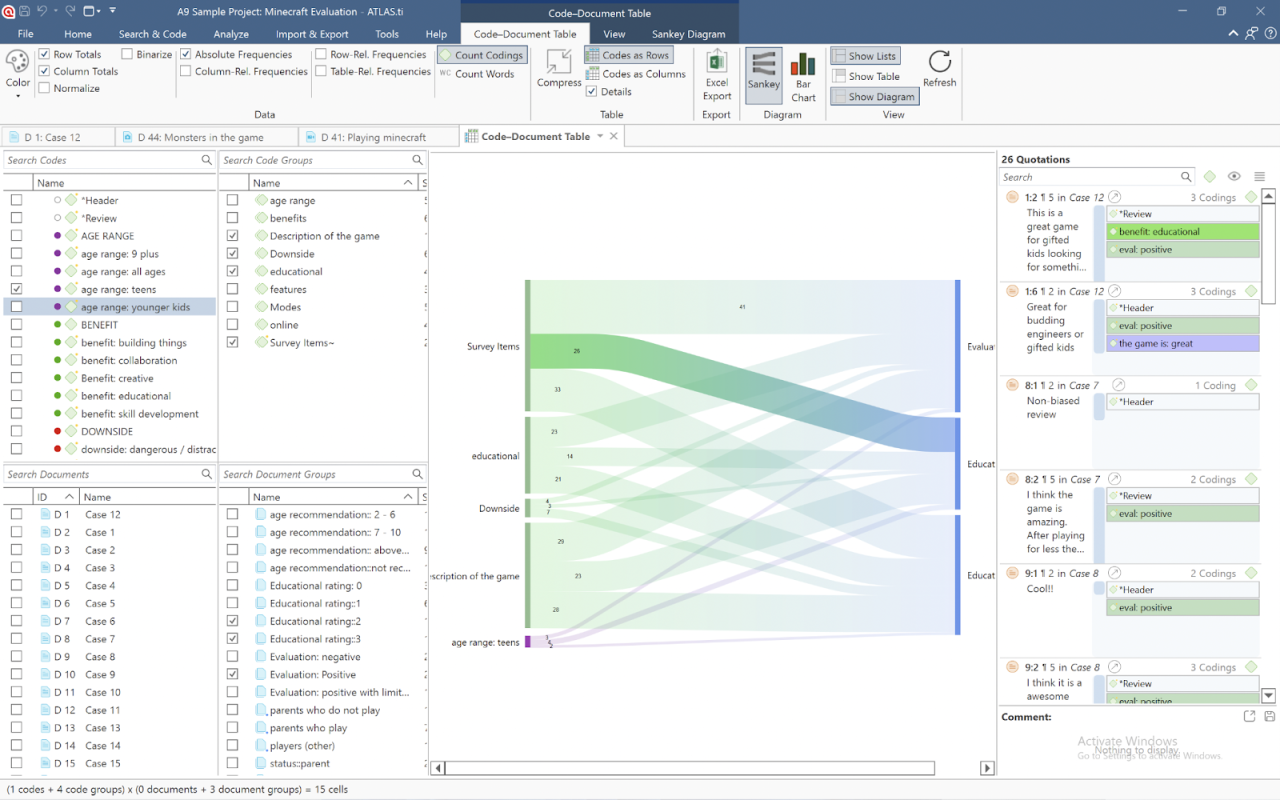
Choosing a master’s program in data analytics is a significant investment, both financially and time-wise. Therefore, understanding the career support offered and the success of graduates in securing employment is crucial. A strong career services department can significantly impact your post-graduation prospects, offering resources and guidance to navigate the job market effectively. Let’s explore what top programs offer and the results they achieve.
Leading data analytics master’s programs recognize the importance of career preparation and integrate comprehensive career services into their curriculum. These services are designed to equip students with the necessary skills and connections to launch successful careers in the field. They often go beyond simple job postings, providing personalized guidance and support throughout the job search process.
Career Services Offered by Leading Programs
Many top programs offer a robust suite of career services, including individual career counseling sessions with experienced professionals. These sessions help students identify their career goals, refine their resumes and cover letters, and practice their interviewing skills. Furthermore, internship placement assistance is a common feature, with dedicated staff working to connect students with relevant internships at leading companies. Networking events, often featuring alumni and industry professionals, provide invaluable opportunities to build connections and learn about potential job openings.
Workshops on topics such as salary negotiation and professional development further enhance career readiness. Some programs even offer personalized LinkedIn profile reviews and mock interviews to bolster students’ confidence and preparedness.
Placement Data and Industry Trends
The effectiveness of these career services is often reflected in the placement data released by the programs themselves. While specific data can vary from year to year, and some programs are more transparent than others, we can examine some general trends. The following table provides illustrative data – remember to always verify this information with the specific program’s website for the most up-to-date and accurate figures.
Program Name | Average Starting Salary (USD) | Placement Rate (%) | Top Employing Companies |
---|---|---|---|
University of California, Berkeley (MIDS) | 130,000 | 95 | Google, Amazon, Facebook, Uber |
Massachusetts Institute of Technology (MIT) | 145,000 | 98 | Microsoft, McKinsey & Company, JPMorgan Chase |
Carnegie Mellon University | 125,000 | 92 | Google, Deloitte, Booz Allen Hamilton |
Stanford University | 150,000 | 97 | Google, Meta, Netflix |
Note: The data presented above is illustrative and may not reflect the current situation. Always refer to the official program websites for the most accurate and up-to-date information.
Industries Employing Data Analytics Graduates, Best masters in data analytics
Graduates from leading data analytics master’s programs find employment across a wide range of industries. The tech sector remains a dominant employer, with companies like Google, Amazon, and Microsoft consistently recruiting large numbers of graduates. However, the demand for data analysts extends far beyond tech. Financial services firms, consulting companies, healthcare organizations, and even retail businesses all actively seek skilled data professionals.
The analytical skills developed in these programs are highly transferable, making graduates attractive candidates in various sectors. For example, a graduate might find themselves working on fraud detection for a bank, optimizing supply chains for a retailer, or developing predictive models for a healthcare provider. The versatility of the skillset ensures a diverse and dynamic career landscape for graduates.
Program Cost and Financial Aid Options
Choosing a Master’s in Data Analytics is a significant investment, both in time and money. Understanding the financial landscape, including tuition fees and available financial aid, is crucial for making an informed decision. This section will explore the cost of several top programs and the various financial assistance options available to prospective students.
The cost of a Master’s in Data Analytics varies considerably depending on the institution, program length, and location. While some programs offer competitive tuition rates, others command significantly higher fees. Furthermore, the availability of financial aid can greatly influence the overall cost. Therefore, a comprehensive understanding of both tuition and financial aid is essential for prospective students to accurately assess the financial implications of pursuing this degree.
Tuition Fees and Financial Aid Comparison
The following table provides a comparison of tuition fees and financial aid opportunities at several leading Data Analytics Master’s programs. Note that these figures are estimates and may vary depending on the year and specific program requirements. Always consult the official university website for the most up-to-date information.
Program Name | Tuition Fee (USD, Estimated Annual) | Scholarship Availability | Loan Options |
---|---|---|---|
Massachusetts Institute of Technology (MIT) | $70,000+ | Merit-based and need-based scholarships, fellowships available; highly competitive. | Federal student loans, private loans, institutional loans. |
Stanford University | $75,000+ | Variety of scholarships and fellowships; highly competitive application process. | Federal student loans, private loans, institutional loans. |
University of California, Berkeley | $50,000+ | Need-based and merit-based financial aid; graduate assistantships available. | Federal student loans, private loans. |
Carnegie Mellon University | $65,000+ | Fellowships, assistantships, and external scholarships. | Federal student loans, private loans. |
University of Michigan | $45,000+ | Variety of scholarships and fellowships, including those specifically for data science. | Federal student loans, private loans. |
Types of Financial Aid
Several types of financial aid can help offset the cost of a Master’s in Data Analytics. Understanding the differences between these options is critical for making the best financial decisions.
Scholarships are typically merit-based awards given to students with exceptional academic records or demonstrated achievements. They usually don’t require repayment. Grants are similar to scholarships, often need-based, awarded to students demonstrating financial need. Loans, on the other hand, require repayment with interest after graduation. Federal student loans usually offer lower interest rates than private loans.
Many universities also offer their own institutional loans with varying terms and conditions. Finally, some programs offer graduate assistantships, which involve working part-time in exchange for tuition remission and a stipend.
Return on Investment (ROI)
The ROI of a Master’s in Data Analytics is often significant. Graduates typically command higher salaries than those with only undergraduate degrees. To calculate ROI, we can compare the total cost of the program (including tuition, fees, and living expenses) to the increase in lifetime earnings. For example, a program costing $100,000 might lead to a $150,000 increase in earnings over ten years, resulting in a positive ROI.
However, this is a simplified calculation and doesn’t account for factors like individual career paths and inflation.
Many sources, such as Glassdoor and Payscale, provide salary data for data analytics professionals. By comparing average salaries for those with and without a Master’s degree, and factoring in program costs and the length of time it takes to recoup the investment, one can develop a more accurate estimate of ROI for a specific program and career path.
For instance, a graduate earning $100,000 annually after graduation might recoup the investment in a few years, demonstrating a strong ROI.
Program Structure and Learning Environment: Best Masters In Data Analytics
Choosing the right Master’s in Data Analytics program involves careful consideration of its structure and the overall learning environment. The ideal program will not only offer a robust curriculum but also a supportive and engaging atmosphere conducive to learning and professional development. Different structures cater to varying needs and lifestyles, impacting the learning experience significantly.Different program structures offer distinct advantages and disadvantages.
Full-time programs offer immersive learning, fostering collaboration and accelerating career transitions. Part-time programs, on the other hand, provide flexibility for working professionals, allowing them to balance their studies with their careers. Online programs provide unparalleled geographical accessibility, while hybrid programs combine the benefits of online and in-person learning. The choice depends heavily on individual circumstances and learning preferences.
Program Structures: Full-time, Part-time, Online, and Hybrid
The structure significantly influences the learning experience. Full-time programs typically involve intensive coursework and projects, demanding a substantial time commitment. This immersive approach fosters a strong sense of community among students and facilitates close interaction with faculty. Part-time programs, often designed for working professionals, allow for a more gradual pace of learning, balancing academic demands with professional responsibilities.
Online programs provide flexibility and accessibility, allowing students to learn from anywhere with an internet connection. However, the lack of in-person interaction can sometimes hinder collaboration and networking opportunities. Hybrid programs aim to bridge the gap by offering a blend of online and in-person classes, combining the flexibility of online learning with the benefits of face-to-face interaction.
Learning Environment and Student Support Systems
A supportive learning environment is crucial for success in a demanding program like a Master’s in Data Analytics. This includes access to resources such as well-equipped computer labs, dedicated academic advisors, and career services. Many programs offer tutoring services, mentorship opportunities, and student organizations that foster collaboration and networking. Strong student support systems, including mental health resources, are increasingly important in ensuring student well-being and academic success.
The availability and quality of these support systems vary significantly across programs, and prospective students should carefully investigate these aspects before making a decision.
A Typical Week in a Top Data Analytics Program
Imagine a typical week for a student in a top-tier data analytics program. Monday might begin with a lecture on advanced machine learning algorithms, followed by a hands-on lab session applying these techniques to a real-world dataset. Tuesday could focus on data visualization, with students learning to create compelling dashboards and presentations. Wednesday might involve a group project, collaborating with peers to analyze a complex dataset and present their findings.
Thursday could be dedicated to a specialized elective, perhaps focusing on a specific industry application of data analytics, like financial modeling or healthcare analytics. Friday might involve independent study, working on individual projects or preparing for upcoming exams. This hypothetical week illustrates the dynamic and engaging nature of a rigorous data analytics program, balancing theoretical knowledge with practical application.
The specific schedule and content will naturally vary across programs and specializations.
Alumni Network and Industry Connections
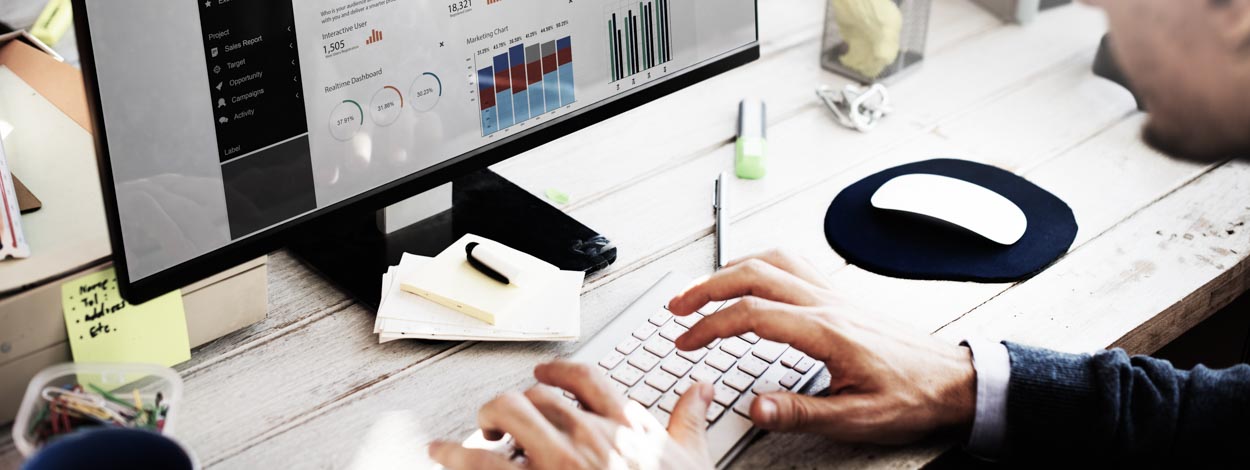
Choosing a data analytics master’s program isn’t just about the curriculum; it’s about the long-term career prospects it unlocks. A strong alumni network and robust industry connections significantly impact your post-graduation success, providing invaluable mentorship, networking opportunities, and access to hidden job markets. This section explores the importance of alumni networks and showcases examples from several leading programs.The strength of an alumni network is often reflected in its activity, the career achievements of its members, and the program’s dedication to fostering these connections.
A thriving alumni network provides ongoing support, offering mentorship, job referrals, and a sense of community long after graduation. This translates to increased career satisfaction and faster career progression for graduates.
Successful Alumni and Career Paths
Many top data analytics programs boast impressive alumni success stories. For example, graduates of the Master of Science in Analytics program at the University of Texas at Austin have gone on to hold data science roles at companies like Google, Amazon, and Facebook, often progressing to senior leadership positions within a few years. Similarly, graduates from the Master of Science in Data Science program at Columbia University have secured prominent positions in finance, consulting, and technology, leveraging their advanced analytical skills and the connections made through their program.
Specific examples could include alumni who founded successful startups based on their data analysis expertise or those who have become recognized leaders in their fields, publishing influential research or contributing to significant technological advancements. The career paths of these individuals demonstrate the program’s effectiveness in preparing students for successful and impactful careers.
Alumni Network Strength and Activity
The effectiveness of an alumni network hinges on its engagement and support. Some programs actively maintain online platforms, organizing regular networking events, mentorship programs, and career workshops. These initiatives foster a sense of community and provide opportunities for alumni to connect with current students and each other. For instance, the University of Michigan’s Master of Applied Data Science program is known for its robust online community and frequent alumni events, providing a platform for networking and collaboration.
A strong and active alumni network provides a consistent source of support, insights, and professional opportunities, significantly increasing the value of the program beyond its academic offerings. This continued engagement demonstrates a commitment to supporting graduates throughout their careers.
Benefits of Strong Alumni Networks for Career Advancement
A strong alumni network offers several key advantages in career advancement. First, it provides access to a vast professional network, opening doors to potential job opportunities and mentorship from experienced professionals. Second, it enhances career visibility, allowing alumni to showcase their achievements and build their professional reputation within their industry. Third, it facilitates collaboration and knowledge sharing, providing access to valuable industry insights and best practices.
Finally, a strong alumni network fosters a sense of community and belonging, providing emotional and professional support throughout one’s career journey. The collective experience and shared network of a successful alumni group offer significant benefits to each individual member, leading to enhanced career trajectories and professional fulfillment.
Final Conclusion
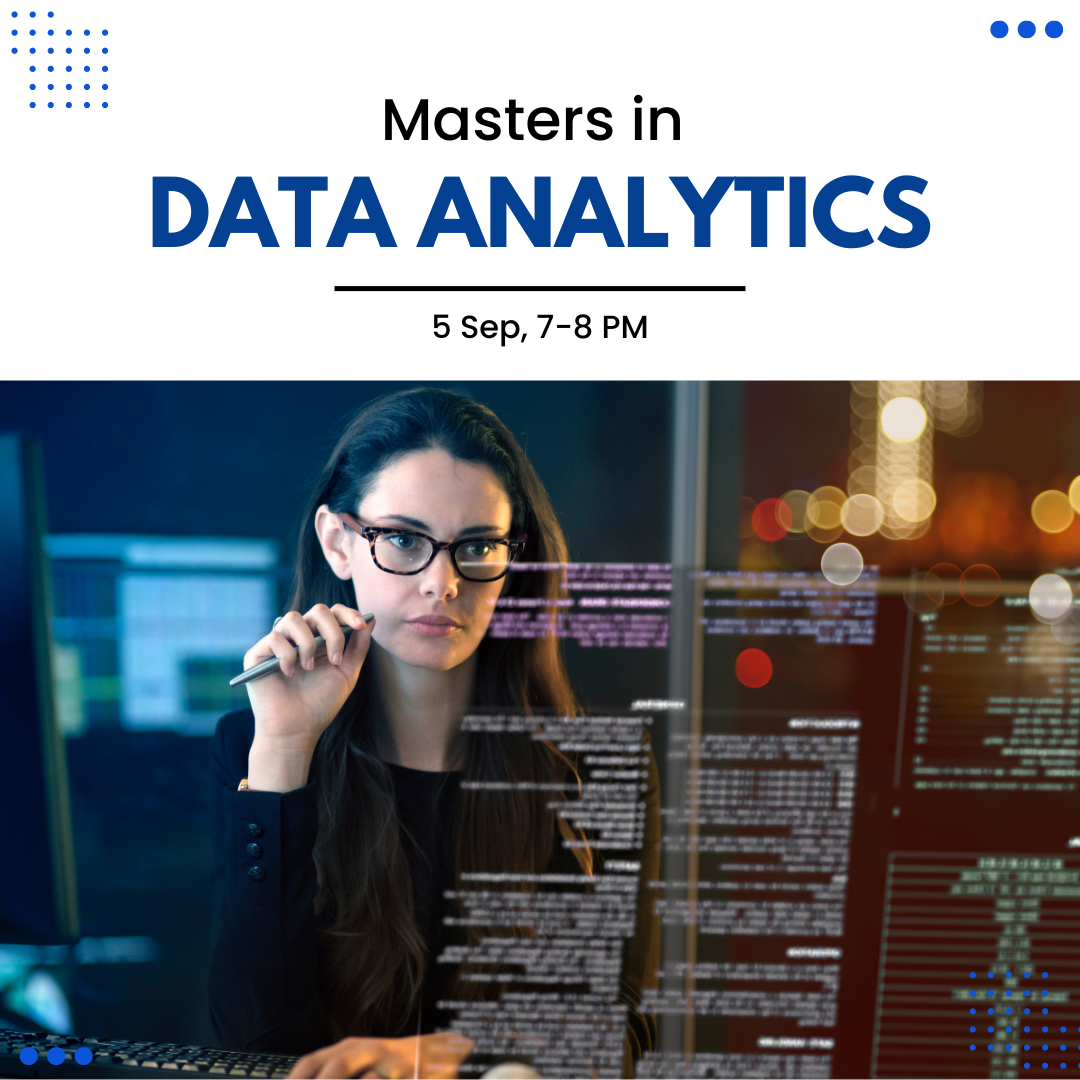
Finding the best Masters in Data Analytics program is a journey of self-discovery and strategic planning. By carefully considering the criteria Artikeld in this guide – from curriculum and faculty expertise to career services and financial aid – you can confidently choose a program that sets you up for success. Remember, the ideal program is not just about the prestige of the institution, but also about the alignment of its offerings with your unique goals and learning style.
Embrace the process, explore your options thoroughly, and embark on this transformative educational experience with confidence and purpose.
FAQs
What are the typical prerequisites for a Masters in Data Analytics program?
Most programs require a bachelor’s degree, preferably in a quantitative field like mathematics, statistics, computer science, or engineering. Strong analytical skills and proficiency in programming languages like Python or R are also usually expected.
How long does a Masters in Data Analytics program typically take to complete?
Most full-time programs can be completed in 1-2 years, while part-time options may take longer.
Are online Masters in Data Analytics programs as valuable as on-campus programs?
The value depends on the program’s reputation and the quality of its online learning resources. Many top universities offer excellent online programs that are comparable to their on-campus counterparts.
What is the average salary for a data analyst with a Masters degree?
Salaries vary widely based on location, experience, and specific skills, but a Masters degree significantly boosts earning potential compared to a Bachelor’s degree.