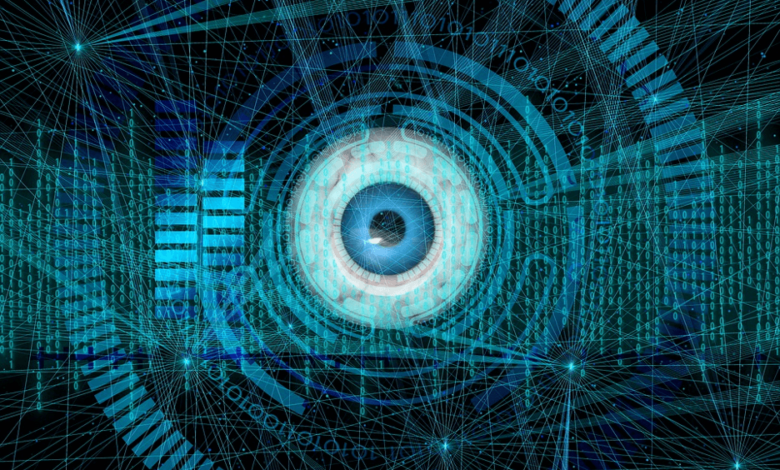
Data Governance Trends Securing Customer Data is Top Priority
Data governance trends report reveals securing customer data is top it priority content sprawl is major concern – Data governance trends report reveals securing customer data is top IT priority; content sprawl is a major concern. This report dives deep into the evolving landscape of data governance, highlighting the critical need for robust security measures in the face of increasing regulatory scrutiny and the ever-expanding digital footprint of modern businesses. We’ll explore the challenges, solutions, and emerging technologies shaping the future of data management, from AI-powered security to the crucial role of employee training.
The report underscores the escalating importance of safeguarding customer data, detailing the stringent compliance requirements driving this priority. We’ll examine successful data security strategies from industry leaders, compare different data encryption methods, and dissect the complexities of content sprawl – a significant hurdle in effective data governance. We’ll also look at emerging technologies like AI and blockchain, and how they’re transforming data security and management.
Top IT Priority: Securing Customer Data: Data Governance Trends Report Reveals Securing Customer Data Is Top It Priority Content Sprawl Is Major Concern
The increasing digitization of businesses and the ever-growing reliance on data have made customer data security a paramount concern. Data breaches not only result in financial losses and reputational damage but also erode customer trust, impacting long-term viability. This makes robust data governance and security a top IT priority, reflecting a fundamental shift in how organizations view data – not just as an asset, but as a critical component of their relationship with customers.Data security is no longer a “nice-to-have” but a “must-have” in today’s landscape.
The sheer volume of data generated and collected, coupled with the sophistication of cyber threats, necessitates a proactive and multi-layered approach to security. This extends beyond simply protecting data at rest; it encompasses data in transit and data in use, requiring a holistic strategy integrated into every aspect of the data lifecycle.
Regulatory Compliance Requirements Driving Data Security
Stringent regulations like the General Data Protection Regulation (GDPR) in Europe, the California Consumer Privacy Act (CCPA) in the US, and similar legislation worldwide, mandate robust data security measures. Non-compliance can result in hefty fines, legal action, and irreparable damage to an organization’s reputation. These regulations dictate how organizations must collect, process, store, and protect personal data, emphasizing transparency, user consent, and data minimization.
For example, GDPR requires organizations to implement appropriate technical and organizational measures to ensure a level of security appropriate to the risk, including data encryption, access control, and regular security audits. The CCPA similarly emphasizes consumer rights to access, delete, and opt-out of the sale of their personal data, necessitating strong data governance and security protocols.
Successful Data Security Strategies
Leading organizations are adopting a range of strategies to bolster their data security posture. Multi-factor authentication (MFA), which requires multiple forms of verification before granting access, is becoming standard practice. Regular security audits and penetration testing help identify vulnerabilities before they can be exploited. Implementing robust access control mechanisms, such as role-based access control (RBAC), limits access to sensitive data only to authorized personnel.
Furthermore, organizations are increasingly investing in advanced threat detection and response systems, utilizing artificial intelligence and machine learning to identify and mitigate potential threats in real-time. For example, Netflix employs a sophisticated security architecture that includes encryption, intrusion detection, and a robust incident response plan, while companies like Google continuously invest in advanced AI-powered security tools to protect their vast user data.
Comparison of Data Encryption Methods
Data encryption is a cornerstone of any robust data security strategy. Different methods offer varying levels of security and performance trade-offs.
Encryption Method | Description | Strength | Performance |
---|---|---|---|
AES (Advanced Encryption Standard) | Symmetric-key algorithm widely considered secure. | High | Good |
RSA (Rivest-Shamir-Adleman) | Asymmetric-key algorithm used for key exchange and digital signatures. | High | Relatively slower than symmetric methods |
ECC (Elliptic Curve Cryptography) | Asymmetric-key algorithm offering strong security with smaller key sizes. | High | Faster than RSA for equivalent security |
PGP (Pretty Good Privacy) | Hybrid cryptosystem using both symmetric and asymmetric encryption. | High | Moderate |
Content Sprawl
Content sprawl, the uncontrolled proliferation of digital information across an organization, is a significant challenge in today’s data-driven world. It poses a serious threat to data security, compliance, and efficient operations, particularly when dealing with sensitive customer data. Untamed content makes it incredibly difficult to locate, manage, and protect valuable information, leading to increased risks and operational inefficiencies.Content sprawl significantly impacts the ability to manage and secure customer data.
The sheer volume and variety of data scattered across various systems and locations makes it nearly impossible to maintain a consistent level of security and compliance. This lack of control increases the vulnerability to data breaches, regulatory penalties, and reputational damage. Furthermore, the inability to readily access relevant information hinders efficient decision-making and operational effectiveness.
Contributing Factors to Content Sprawl
Several factors contribute to the pervasive problem of content sprawl within organizations. These include a lack of standardized processes for content creation and management, the rapid growth of digital data, the proliferation of cloud storage solutions without proper governance, and insufficient employee training on data handling best practices. Shadow IT, where departments utilize unsanctioned applications and storage solutions, further exacerbates the issue, creating data silos that are difficult to monitor and control.
The absence of a robust data governance framework often underlies these issues, leaving organizations vulnerable to the negative consequences of uncontrolled content proliferation.
So, the latest data governance trends report highlights securing customer data as the top IT priority, with content sprawl a major headache. This makes solutions like cloud security posture management (CSPM) incredibly important, and that’s where understanding platforms such as bitglass and the rise of cloud security posture management becomes crucial. Ultimately, effective CSPM helps address the report’s concerns by providing better visibility and control over sensitive data scattered across the cloud.
Strategies for Mitigating Content Sprawl
Effective mitigation strategies for content sprawl require a multi-faceted approach. Implementing a comprehensive data governance framework is paramount. This framework should establish clear policies and procedures for content creation, storage, access, and disposal. Investing in robust content management systems (CMS) capable of centralizing and organizing information is crucial. Regular data audits and inventory assessments help identify and address data silos and redundant information.
Employee training programs focusing on data handling best practices, security awareness, and compliance requirements are also essential. Finally, regular review and updates of data governance policies ensure they remain relevant and effective in addressing evolving challenges.
Workflow Diagram for Streamlined Content Management
Data Governance Trends
So, we’ve already tackled the biggies – securing customer data and managing content sprawl. But the world of data governance is vast and ever-evolving. Let’s delve into some other key trends shaping how organizations handle their data. These trends aren’t isolated incidents; they’re interconnected and impact each other, requiring a holistic approach to data governance.
This section explores three significant data governance trends beyond the already discussed security and sprawl issues. We’ll look at how organizations are adapting and the implications for businesses.
Increased Focus on Data Quality
Data quality is no longer a “nice-to-have” but a critical business necessity. Poor data quality leads to inaccurate insights, flawed decision-making, and ultimately, lost revenue. Organizations are increasingly investing in data quality management tools and processes to ensure data accuracy, completeness, consistency, and timeliness. This includes implementing data profiling techniques to identify and address data quality issues proactively.
For example, a retail company might use data profiling to identify inconsistencies in customer addresses, leading to improved delivery accuracy and reduced shipping costs. The approach here is shifting from reactive (fixing issues as they arise) to proactive (preventing issues before they impact business operations).
Growing Adoption of Data Mesh, Data governance trends report reveals securing customer data is top it priority content sprawl is major concern
The traditional centralized data warehouse approach is proving insufficient for many organizations facing the challenges of data volume, velocity, and variety. Data mesh is an emerging architectural pattern that distributes data ownership and governance to individual domains within an organization. This allows teams to manage their own data products, fostering agility and improving data accessibility. However, this decentralized approach requires careful planning and coordination to ensure data consistency and interoperability across domains.
A financial institution, for example, might adopt a data mesh architecture, allowing different departments (e.g., loan processing, risk management) to manage their own data while adhering to common governance standards. The contrasting approach, a centralized data warehouse, can be slower to adapt to new data sources and business needs.
Rise of AI and Machine Learning in Data Governance
Artificial intelligence (AI) and machine learning (ML) are transforming data governance by automating tasks such as data discovery, classification, and quality monitoring. These technologies can help organizations identify sensitive data, enforce data policies, and detect anomalies that may indicate data breaches or other issues. The use of AI/ML is allowing for more efficient and scalable data governance, especially in organizations dealing with large volumes of data.
For instance, an AI-powered system could automatically classify documents based on their content, reducing the manual effort required for data classification and ensuring compliance with regulatory requirements. This contrasts with manual processes which are prone to errors and bottlenecks.
The implications of these trends for businesses are substantial.
- Improved data quality leads to better decision-making and increased efficiency.
- Data mesh enhances agility and responsiveness to business needs.
- AI/ML automation streamlines data governance processes and reduces costs.
- Increased compliance with data privacy regulations.
- Enhanced data security and risk management.
Emerging Technologies and Data Governance
The rise of emerging technologies is fundamentally reshaping data governance strategies. No longer a purely reactive measure, data governance is becoming proactive, leveraging the power of AI, blockchain, and other innovations to enhance security, streamline processes, and unlock the true value of data. This shift necessitates a re-evaluation of existing frameworks and the adoption of new tools and techniques to effectively manage the complexities of the modern data landscape.The integration of these technologies offers significant advantages in both data security and the overall effectiveness of data governance initiatives.
The latest data governance trends report highlights securing customer data as the top IT priority, with content sprawl a major headache. This makes efficient, secure application development crucial, and that’s where learning about domino app dev, the low-code and pro-code future , becomes incredibly relevant. Ultimately, streamlining development helps manage the very data sprawl that’s putting customer data at risk.
By automating tasks, improving data quality, and strengthening security protocols, organizations can mitigate risks, reduce costs, and achieve greater compliance with evolving regulations.
AI’s Role in Enhancing Data Security and Governance
Artificial intelligence is revolutionizing data security by automating threat detection and response. AI-powered systems can analyze vast amounts of data in real-time, identifying anomalies and potential breaches far more quickly and accurately than human analysts alone. For example, AI algorithms can detect unusual login attempts, suspicious data access patterns, and even subtle indicators of malware infections, enabling proactive intervention before significant damage occurs.
Furthermore, AI can be used to automate data classification and masking, ensuring sensitive information is appropriately protected based on predefined policies. This automation reduces the risk of human error and ensures consistent application of data protection measures across the organization.
Blockchain’s Impact on Data Transparency and Trust
Blockchain technology, known for its secure and transparent nature, offers a powerful solution for managing data provenance and ensuring data integrity. By creating an immutable record of data transactions, blockchain can enhance trust and accountability within data governance processes. Imagine a scenario where every access, modification, or transfer of sensitive customer data is recorded on a blockchain. This provides a complete audit trail, making it easier to track data usage, identify potential breaches, and comply with regulatory requirements.
Companies are beginning to explore blockchain’s potential for secure data sharing, particularly in collaborative environments where multiple organizations need to access and exchange sensitive information while maintaining strict control over access and usage.
Examples of Organizations Leveraging Emerging Technologies
Several organizations are already leading the way in integrating emerging technologies into their data governance strategies. Financial institutions are utilizing AI-powered fraud detection systems to identify and prevent fraudulent transactions, protecting both their assets and their customers’ financial data. Healthcare providers are leveraging blockchain to securely share patient medical records between different healthcare facilities, improving patient care coordination and reducing the risk of data breaches.
Retail companies are employing AI-powered analytics to personalize customer experiences while ensuring compliance with data privacy regulations like GDPR. These examples illustrate the broad applicability of these technologies and their potential to transform data governance practices across various industries.
The Human Element in Data Governance
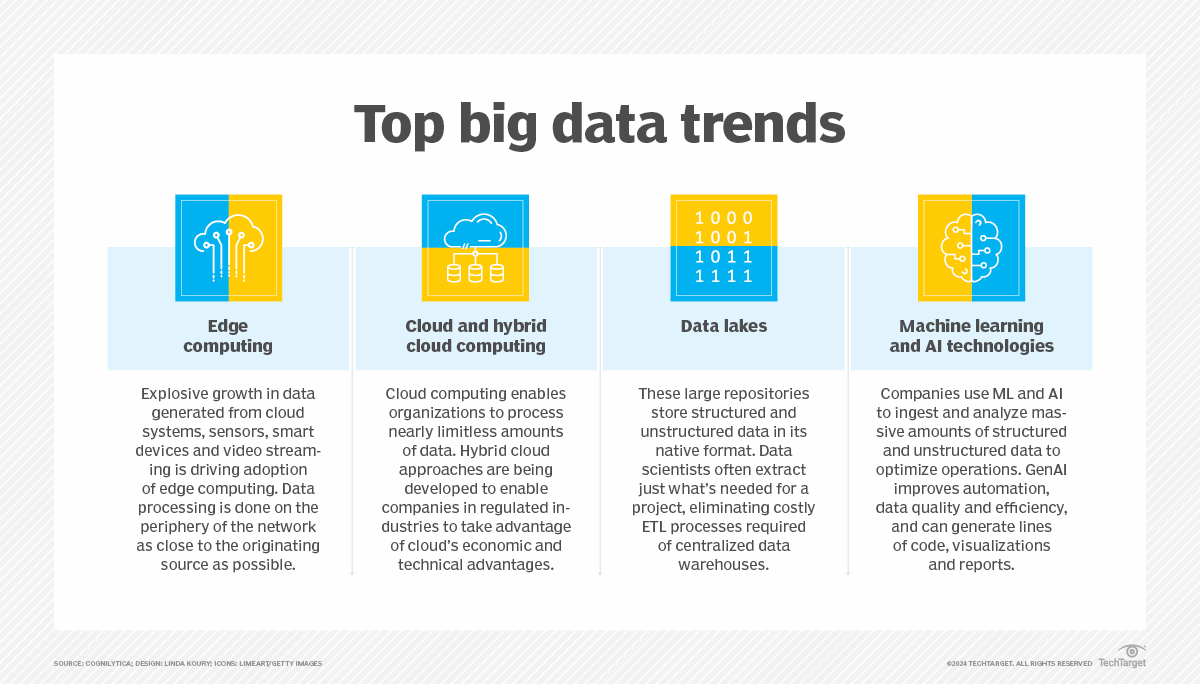
Data governance isn’t just about technology and policies; it’s fundamentally about people. The success of any data governance initiative hinges on the understanding, commitment, and responsible actions of every employee. Without a strong human element, even the most sophisticated systems and regulations will fall short. This section explores the crucial role of employee engagement in building a robust and effective data governance framework.Employee training and awareness are the cornerstones of a successful data governance program.
Effective data governance requires a workforce that understands data privacy regulations, security protocols, and the organization’s data handling policies. Without this understanding, employees might unintentionally compromise sensitive information or violate regulations, leading to significant risks and potential legal repercussions.
Employee Training and Awareness in Effective Data Governance
A comprehensive training program should cover various aspects of data governance, including data security best practices, the organization’s specific data policies, and the consequences of non-compliance. Interactive modules, scenario-based exercises, and regular refresher courses can reinforce learning and ensure employees remain up-to-date on evolving regulations and best practices. For example, a hypothetical training scenario could involve a simulated phishing email, allowing employees to practice identifying and reporting suspicious communications.
Regular quizzes and assessments can also help gauge the effectiveness of training and identify areas needing improvement. Furthermore, training should be tailored to different roles and responsibilities within the organization, ensuring that each employee receives the specific knowledge and skills relevant to their job function.
Establishing a Strong Data Governance Culture
A strong data governance culture is not merely a set of rules and regulations; it’s a shared understanding and commitment to responsible data handling throughout the organization. This culture is fostered through leadership commitment, clear communication, and consistent reinforcement of data governance principles. Leaders must actively champion data governance initiatives, demonstrating their importance through their actions and setting a positive example for their teams.
Open communication channels, regular updates, and opportunities for feedback can ensure employees understand the “why” behind data governance policies and feel involved in the process. Celebrating successes and addressing failures constructively can further strengthen this culture. For instance, a company could recognize employees who consistently demonstrate exemplary data handling practices through awards or public acknowledgment.
Fostering Data Literacy and Promoting Responsible Data Handling
Data literacy is the ability to read, work with, analyze, and argue with data. It’s crucial for employees to understand the data they handle, its importance, and the potential consequences of mishandling it. Organizations can foster data literacy through various initiatives, including workshops, online resources, and mentorship programs. These initiatives should focus on practical skills, such as data interpretation, data visualization, and data analysis techniques.
Furthermore, clear and accessible data governance policies, combined with readily available resources and support, empower employees to make responsible data handling decisions. For example, a company could provide a readily accessible internal wiki with clear explanations of data governance policies, frequently asked questions, and contact information for support. Regular communication campaigns highlighting data privacy concerns and best practices can further reinforce responsible data handling behaviors.
Data Loss Prevention (DLP) Strategies
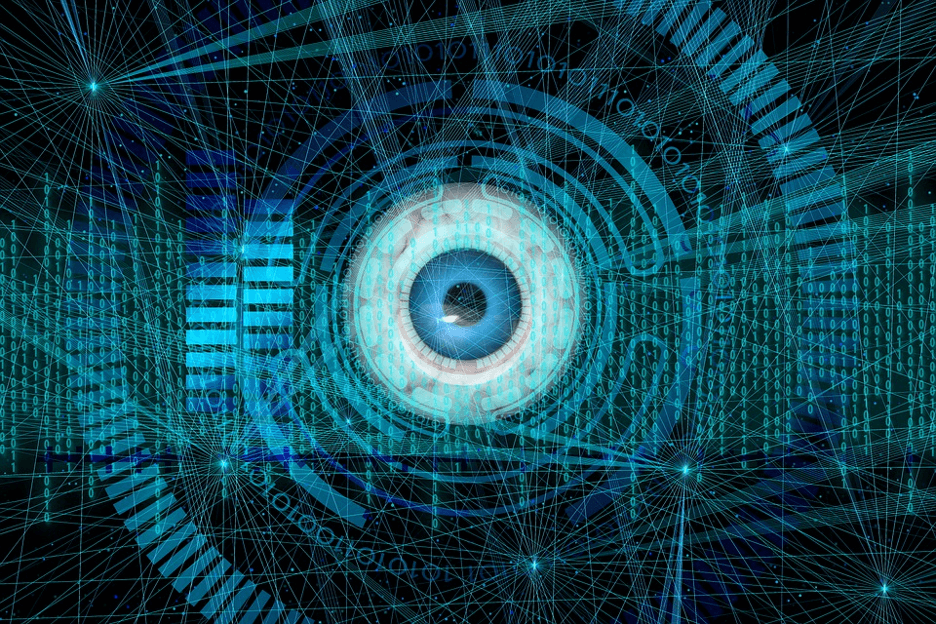
Data loss prevention (DLP) is paramount in today’s data-driven world, where breaches can lead to significant financial losses, reputational damage, and legal repercussions. Effective DLP strategies encompass a multi-layered approach, combining technological solutions with robust security policies and employee training. The goal is to identify, monitor, and prevent sensitive data from leaving the organization’s control, whether intentionally or unintentionally.
Organizations employ a variety of DLP strategies, each with its strengths and weaknesses. These strategies often work in concert to provide comprehensive protection. The selection of appropriate DLP tools and technologies depends heavily on the specific needs and risk profile of the organization, considering factors like data sensitivity, regulatory compliance requirements, and budget constraints.
Network-Based DLP
Network-based DLP solutions monitor network traffic for suspicious activity, such as unauthorized data transfers or attempts to access sensitive data. These solutions typically analyze data packets in real-time, identifying sensitive information based on predefined rules and patterns. They can block malicious traffic, generate alerts, and provide detailed logs of suspicious activity. Examples include inspecting outbound emails for confidential information or monitoring file transfers to detect unauthorized uploads to cloud storage services.
The effectiveness of network-based DLP relies heavily on accurate configuration and regular updates to keep pace with evolving threats.
Endpoint DLP
Endpoint DLP solutions focus on protecting data residing on individual devices, such as laptops, desktops, and mobile phones. These solutions monitor data at the source, preventing sensitive information from being copied, printed, or transmitted without authorization. They can scan files, applications, and clipboard contents, blocking or logging suspicious activity. Endpoint DLP offers granular control over data access and usage, but requires careful deployment and management to avoid impacting user productivity.
For instance, it might prevent an employee from copying a sensitive client list to a USB drive or emailing it to a personal account.
Cloud DLP
Cloud DLP solutions are designed to protect data stored in cloud environments, such as cloud storage services and SaaS applications. These solutions typically leverage APIs and integrations with cloud providers to monitor data access and usage, identifying and preventing unauthorized activities. The effectiveness of cloud DLP depends on the integration capabilities of the solution and the security posture of the cloud provider.
A key benefit is the ability to protect data regardless of its location, provided the cloud service provider offers appropriate APIs for integration. This might include preventing the upload of sensitive data to an unauthorized cloud storage account or detecting and preventing the sharing of sensitive data via collaborative tools.
Data Classification and Labeling
Data classification and labeling is a crucial component of any effective DLP strategy. This involves identifying and categorizing sensitive data based on its value and sensitivity, assigning appropriate labels to data assets. These labels can then be used by DLP tools to enforce access control policies and monitor data usage. For example, labeling a document as “Confidential – Client Data” allows DLP tools to automatically apply stricter access controls and monitor its usage more closely.
Effective data classification requires a clear understanding of regulatory requirements and organizational policies.
DLP Tool Comparison
Feature | Network-Based DLP | Endpoint DLP | Cloud DLP |
---|---|---|---|
Deployment | Network infrastructure | Individual devices | Cloud environment |
Monitoring Scope | Network traffic | Device activity | Cloud data |
Data Visibility | Limited to network traffic | Comprehensive device data | Dependent on cloud provider API access |
Benefits | Prevents external threats, monitors large volumes of data | Granular control, prevents data loss from devices | Protects cloud-based data, scalable and flexible |
Summary
In conclusion, navigating the ever-changing world of data governance requires a multifaceted approach. Prioritizing customer data security, tackling content sprawl, and embracing emerging technologies are crucial steps. But it’s equally important to cultivate a strong data governance culture within your organization through comprehensive employee training and a commitment to data literacy. By proactively addressing these challenges, businesses can not only meet regulatory demands but also build trust with customers and gain a competitive edge in today’s data-driven world.
Top FAQs
What are the biggest penalties for non-compliance with data privacy regulations?
Penalties vary widely depending on the regulation (GDPR, CCPA, etc.) and the severity of the violation, ranging from hefty fines to legal action and reputational damage.
How can we effectively measure the success of our data governance initiatives?
Key Performance Indicators (KPIs) such as reduced data breaches, improved data quality, faster data access, and increased employee data literacy can be used to track progress.
What are some common mistakes organizations make in data governance?
Common mistakes include neglecting employee training, lacking a clear data governance strategy, insufficient data encryption, and failing to regularly assess and update security protocols.
How can we ensure our data governance strategy remains adaptable to future changes?
Regularly review and update your strategy, incorporating emerging technologies and adapting to changes in regulations and business needs. A flexible, agile approach is key.