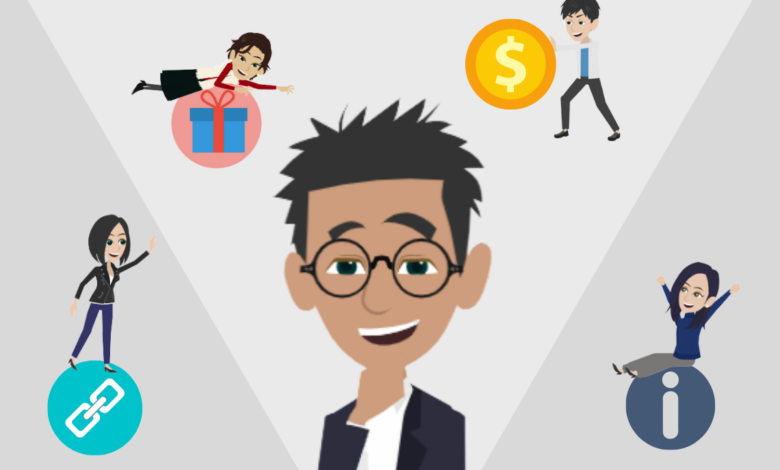
Decoding Customer Decisions The Rise of IoB
Decoding customer decisions the rise of internet of behavior – Decoding customer decisions: the rise of the Internet of Behavior (IoB) is reshaping how businesses understand and interact with their customers. It’s no longer enough to simply track website clicks; IoB delves into the rich tapestry of online and offline behaviors to paint a truly comprehensive picture of individual preferences and motivations. This means leveraging data from social media, mobile apps, and even in-store interactions to predict future actions and tailor experiences accordingly.
It’s a fascinating field, brimming with both incredible potential and important ethical considerations.
This post will explore the core principles of IoB, examining how data is collected, analyzed, and ultimately used to create more personalized and effective customer journeys. We’ll delve into the psychological factors influencing purchasing decisions, discuss the challenges of data privacy, and look ahead to the future of IoB and its implications for businesses and consumers alike. Get ready to unlock the secrets behind customer choices!
Defining the Internet of Behavior (IoB)
The Internet of Behavior (IoB) represents a significant evolution in data analysis, moving beyond simple website analytics to encompass a holistic understanding of human behavior across various digital touchpoints. It leverages the vast quantities of data generated by our increasingly interconnected lives to predict and influence actions, ultimately shaping individual and collective behavior. Unlike traditional data analysis which often focuses on isolated datasets, IoB integrates information from diverse sources to create a comprehensive profile of an individual or group.IoB’s core principle lies in the aggregation and analysis of diverse behavioral data to generate actionable insights.
This differs from traditional data analysis, which often examines data in silos. For example, a traditional approach might analyze website traffic independently of social media engagement. IoB, however, combines these data streams, providing a much richer and more nuanced understanding of customer behavior. This allows businesses to anticipate needs, personalize experiences, and optimize strategies with unprecedented accuracy.
Data Sources Utilized in IoB
IoB draws upon a wide range of data sources to build its behavioral profiles. These sources provide a multi-faceted view of individual actions and preferences. Key data sources include website analytics, tracking user interactions like page views, time spent on site, and click-through rates. Social media activity offers insights into opinions, preferences, and social connections through posts, likes, shares, and comments.
Mobile app usage data reveals patterns in app usage, location data, and in-app purchases, providing a real-time picture of user behavior. Other sources can include loyalty program data, transactional data from purchases, and even data from wearable devices monitoring physical activity and health metrics.
IoB Applications Across Industries, Decoding customer decisions the rise of internet of behavior
The applications of IoB are far-reaching and impact numerous industries. In retail, IoB helps personalize recommendations, optimize pricing strategies, and improve customer service. For example, a retailer might use IoB to identify customers likely to abandon their online shopping carts and send targeted email reminders or promotions. In finance, IoB enhances fraud detection, risk management, and personalized financial advice.
By analyzing transaction patterns and social media activity, financial institutions can identify potential fraudulent activities and tailor financial products to individual needs. Healthcare utilizes IoB for personalized medicine, disease prediction, and improved patient care. By analyzing patient data, including wearable device data and electronic health records, healthcare providers can develop more effective treatment plans and proactively manage patient health.
Ethical Considerations of IoB
The power of IoB comes with significant ethical responsibilities. The collection and use of behavioral data raise concerns about privacy, data security, and potential biases.
Ethical Consideration | Data Source | Industry Application | Potential Risks |
---|---|---|---|
Data Privacy | Website analytics, social media activity | Retail, finance, healthcare | Unauthorized access, data breaches, discriminatory profiling |
Data Security | Mobile app usage, transactional data | All industries | Data leaks, hacking, misuse of sensitive information |
Algorithmic Bias | All data sources | All industries | Unfair or discriminatory outcomes due to biased algorithms |
Transparency and Consent | All data sources | All industries | Lack of transparency about data collection and usage, insufficient informed consent |
Data Collection and Interpretation within IoB
The Internet of Behavior (IoB) relies heavily on the collection and interpretation of vast amounts of data to understand and predict human actions. This data, generated from various digital touchpoints, offers unprecedented insights into consumer preferences, trends, and future behavior. However, this power comes with significant responsibility, particularly regarding data privacy and ethical considerations. The following sections delve into the methods, challenges, and implications of data collection and analysis within the IoB ecosystem.
Understanding customer decisions in the age of the Internet of Behavior is a fascinating challenge. To build truly effective applications that anticipate and respond to these decisions, developers need powerful yet accessible tools. That’s where the advancements in application development, like those discussed in this insightful article on domino app dev the low code and pro code future , become crucial.
Ultimately, mastering this tech helps us create apps that better reflect and serve the ever-evolving needs revealed by behavioral data.
Data within the IoB is gathered from a multitude of sources, encompassing everything from website analytics and social media activity to location data from smartphones and smart devices. This diverse data landscape requires sophisticated techniques for collection, processing, and analysis. The most common methods involve passively collecting data through embedded sensors and tracking technologies, actively gathering information through surveys and questionnaires, and leveraging publicly available data sources.
Crucially, all data collection must adhere to strict privacy regulations like GDPR and CCPA, necessitating transparency and user consent. Robust security measures, including encryption and anonymization techniques, are essential to protect sensitive information from unauthorized access and misuse.
Data Aggregation and Analysis Techniques
Behavioral data, in its raw form, is often fragmented and seemingly meaningless. To extract valuable insights, it needs to be aggregated and analyzed using various techniques. This often involves combining data from multiple sources to create a comprehensive picture of individual and group behavior. For example, a company might combine website browsing history with purchase data and social media engagement to understand customer preferences for certain product categories.
Advanced analytical techniques like machine learning and artificial intelligence are then employed to identify patterns, correlations, and anomalies within the aggregated data. This allows businesses to segment their customer base, personalize marketing campaigns, and optimize product development strategies. For instance, analyzing location data alongside purchase history can reveal patterns in consumer behavior near specific retail locations, leading to optimized store placement or targeted advertising campaigns.
Pattern Identification and Predictive Modeling
The ultimate goal of IoB data analysis is often to predict future customer behavior. This involves identifying patterns and trends in past behavior and extrapolating them to forecast future actions. Several techniques are used for this purpose, including time series analysis, which identifies trends and seasonality in data over time, and machine learning algorithms, such as regression and classification models, which can predict future outcomes based on historical data.
For example, a streaming service might use machine learning to predict which shows a user is likely to watch next based on their viewing history and preferences. Similarly, a retailer might use predictive modeling to forecast demand for specific products, allowing them to optimize inventory management and avoid stockouts. The accuracy of these predictions depends heavily on the quality and completeness of the underlying data.
Challenges of Incomplete or Biased Data
Working with IoB data presents significant challenges. Data sets are often incomplete, meaning they lack information on certain aspects of customer behavior. This can lead to inaccurate or incomplete insights. Moreover, data can be biased, reflecting only certain segments of the population or containing systematic errors. For example, relying solely on online data might exclude customers who primarily shop offline.
Addressing these challenges requires careful data cleaning, validation, and the use of robust statistical methods that account for potential biases. Techniques like imputation (filling in missing values) and weighting (adjusting for over- or under-representation of certain groups) can help mitigate these issues. Understanding the limitations of the data and acknowledging potential biases is crucial for drawing accurate and reliable conclusions.
Understanding Customer Decision-Making Processes
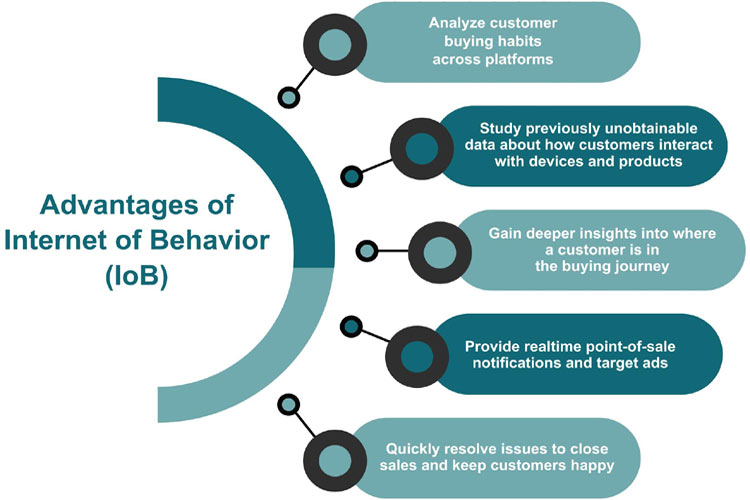
The digital age has fundamentally altered how customers make decisions. No longer constrained by geographical limitations or limited product information, consumers are now exposed to a constant barrage of choices, influenced by a complex interplay of psychological factors and readily available data. Understanding this intricate process is crucial for businesses aiming to effectively engage and convert their target audience.
The Internet of Behavior (IoB) provides unprecedented access to data illuminating these processes, offering businesses a powerful tool to understand and shape customer journeys.
Psychological factors significantly influence online purchasing behavior. Cognitive biases, such as confirmation bias (favoring information confirming pre-existing beliefs) and anchoring bias (over-relying on the first piece of information received), play a significant role. Emotional factors, including feelings of trust, excitement, or fear, also strongly impact decisions. For instance, a customer might be more likely to purchase a product if they feel a strong sense of community with the brand or if a limited-time offer creates a sense of urgency.
Psychological Factors Influencing Customer Choices
Consumers’ choices are shaped by a multitude of cognitive and emotional factors. Heuristics, or mental shortcuts, are frequently employed to simplify complex decisions. For example, a customer might choose a product based solely on its brand reputation, rather than engaging in detailed comparison shopping. Social proof, such as online reviews and testimonials, significantly impacts purchasing decisions, as consumers tend to trust the opinions of others.
Furthermore, the design and layout of websites and apps can subtly influence choices through techniques like persuasive design elements or strategically placed calls to action. Understanding these psychological nuances allows businesses to craft more effective marketing strategies and enhance user experience.
Key Behavioral Indicators Revealing Customer Needs and Motivations
Analyzing digital footprints reveals crucial insights into customer needs and motivations. Website analytics, for instance, show which pages are most visited, how long users spend on each page, and which calls to action are most effective. Social media activity, including likes, shares, and comments, provides valuable information on brand perception and customer sentiment. Search queries reveal specific product interests and needs, while purchase history indicates past preferences and buying patterns.
Combining these data points paints a comprehensive picture of customer behavior, enabling businesses to identify unmet needs and tailor their offerings accordingly.
Steps of a Typical Customer Journey and IoB Impact
A typical customer journey can be broken down into several key stages: awareness, consideration, decision, action, and loyalty. IoB data can significantly enhance understanding and optimization at each stage. For example, during the awareness phase, analyzing social media trends can help identify emerging customer needs. During the consideration phase, personalized recommendations based on browsing history can guide customers toward relevant products.
At the decision stage, targeted advertising based on demographics and online behavior can influence the final purchase. Post-purchase, IoB insights can facilitate targeted loyalty programs and improve customer service based on past interactions.
Hypothetical Customer Profile Based on IoB Data
Consider “Sarah,” a 32-year-old female living in a suburban area, identified through her online activity and purchase history. Her IoB profile reveals she frequently visits websites related to sustainable living, organic food, and fitness. She actively engages with environmental advocacy groups on social media and consistently purchases eco-friendly products online. Her purchase history shows a preference for high-quality, ethically sourced goods, even if they are slightly more expensive.
Sarah’s online behavior suggests a strong interest in health and wellness, coupled with a commitment to environmental responsibility. This detailed profile allows businesses to tailor marketing messages, product recommendations, and customer service interactions to resonate specifically with Sarah’s values and preferences.
Applying IoB Insights to Improve Customer Experiences
The Internet of Behavior (IoB) offers a treasure trove of data that, when analyzed correctly, can dramatically improve customer experiences. By understanding individual customer behaviors and preferences, businesses can move beyond generic marketing and product development, creating truly personalized and engaging interactions. This leads to increased customer loyalty, higher conversion rates, and ultimately, a stronger bottom line. This section will explore how to leverage IoB insights to achieve these goals.
IoB data provides a granular view of customer behavior, far exceeding traditional market research methods. It allows businesses to identify patterns, predict future actions, and tailor their strategies accordingly. This personalized approach fosters stronger customer relationships, leading to increased satisfaction and retention. For example, by analyzing browsing history and purchase patterns, a retailer can anticipate a customer’s need for a specific product and proactively offer it through targeted advertising or personalized recommendations.
Personalizing Marketing Campaigns and Improving Customer Engagement
Effective personalization goes beyond simply addressing a customer by name. It involves tailoring the entire customer journey, from initial contact to post-purchase engagement. IoB data allows businesses to segment their audience based on highly specific behavioral patterns, enabling the creation of highly targeted marketing campaigns. For instance, a streaming service could use IoB data to identify users who consistently watch documentaries and then recommend similar content, potentially including upcoming releases or related specials.
This level of personalization significantly improves customer engagement by delivering relevant and valuable content at the right time. Further, analyzing user interactions with marketing emails, for example, open rates and click-through rates, allows for continuous optimization of campaign messaging and delivery methods.
Leveraging IoB Data to Optimize Products and Services
IoB data provides invaluable feedback for product development and service improvement. By analyzing customer interactions with a product or service, businesses can identify pain points, areas for improvement, and unmet needs. Consider a fitness app that tracks user workouts and collects data on usage patterns. Analyzing this data could reveal that users struggle with a specific feature or find certain aspects of the app cumbersome.
This feedback can be directly used to improve the app’s design and functionality, ultimately leading to a more satisfying user experience and increased user retention. Furthermore, the identification of underutilized features can inform future product development, ensuring resources are focused on areas that will have the most significant impact on customer satisfaction.
Communicating IoB Findings to Stakeholders
Clearly communicating IoB findings to stakeholders is crucial for effective implementation. This requires translating complex data into easily understandable insights. Using clear visualizations, such as charts and graphs, is essential. Furthermore, focusing on the business impact of the findings – increased revenue, improved customer satisfaction, or reduced operational costs – makes the information more relevant and persuasive. Regular reporting on key performance indicators (KPIs) derived from IoB data provides ongoing transparency and demonstrates the value of the investment in IoB initiatives.
A well-structured presentation, incorporating both qualitative and quantitative data, will effectively communicate the implications of IoB insights and secure buy-in from stakeholders.
Implementing an IoB Strategy: A Step-by-Step Guide
Implementing a successful IoB strategy requires a structured approach.
First, define clear objectives. What specific business problems are you hoping to solve with IoB data? What are your key performance indicators (KPIs)? Setting clear objectives will guide your data collection and analysis efforts.
Second, identify relevant data sources. This could include website analytics, CRM data, app usage data, social media activity, and IoT device data. Ensure compliance with all relevant privacy regulations.
Third, establish a robust data collection and analysis infrastructure. This may involve investing in new technologies or partnering with a data analytics provider. Ensure the data is accurate, reliable, and securely stored.
Fourth, develop a data analysis plan. How will you analyze the data to extract meaningful insights? What analytical techniques will you use? Will you utilize machine learning algorithms for predictive modeling?
Fifth, implement changes based on insights. Once you’ve identified actionable insights, implement changes to your marketing campaigns, products, or services. Continuously monitor and evaluate the impact of these changes.
Sixth, monitor and iterate. The IoB landscape is constantly evolving. Regularly review your IoB strategy and make adjustments based on new data and emerging trends. This iterative approach is key to maintaining a competitive advantage.
The Future of Decoding Customer Decisions with IoB
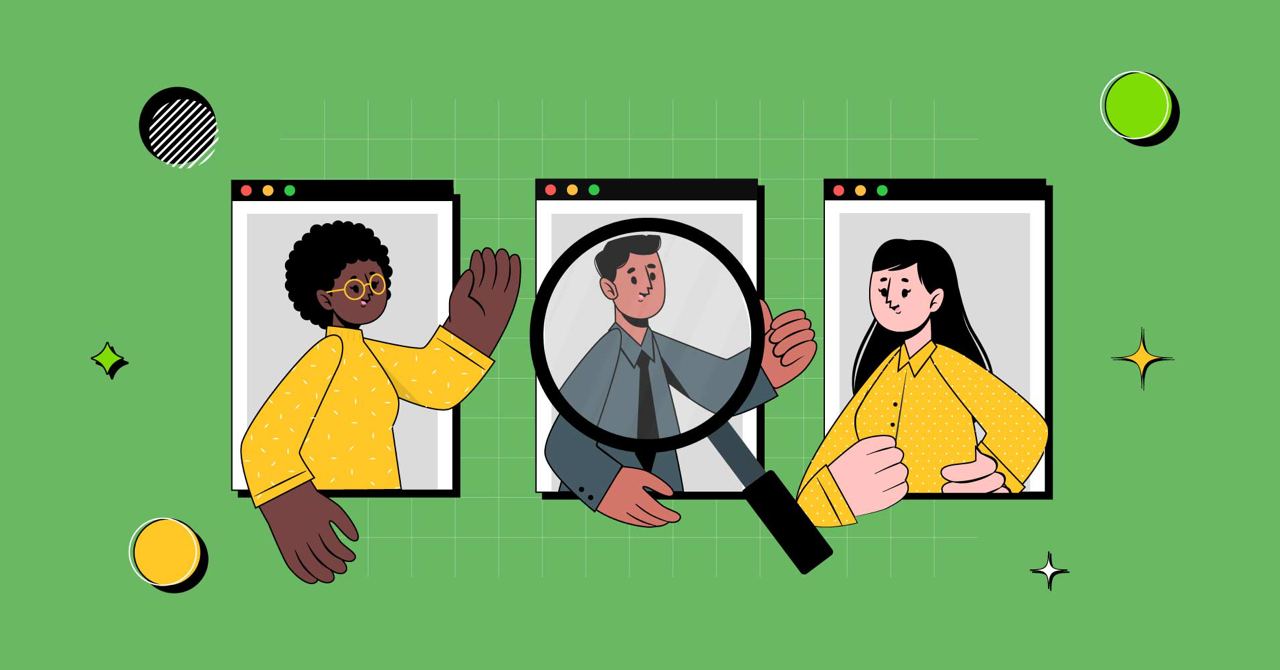
The Internet of Behavior (IoB) is rapidly evolving, promising unprecedented insights into customer decision-making. However, its future hinges on several key factors: the integration of advanced technologies, the evolving regulatory landscape, and the ethical considerations surrounding data usage. Understanding these elements is crucial for businesses seeking to leverage IoB effectively and responsibly.
The Impact of Emerging Technologies on IoB Analysis
Artificial intelligence (AI) and machine learning (ML) are poised to revolutionize IoB analysis. AI algorithms can process vast quantities of behavioral data far exceeding human capabilities, identifying subtle patterns and predicting future behaviors with increasing accuracy. For example, AI-powered predictive models can analyze website browsing history, social media activity, and purchase data to anticipate customer needs and personalize marketing campaigns with remarkable precision.
Machine learning, in particular, allows for continuous improvement of these models, adapting to changing customer behaviors and market dynamics. This continuous learning capability makes IoB systems more robust and responsive over time. Furthermore, advancements in natural language processing (NLP) enable the analysis of unstructured data like customer reviews and social media comments, providing valuable qualitative insights alongside quantitative data.
The Evolving Regulatory Landscape of Behavioral Data
The collection and use of behavioral data are increasingly subject to stricter regulations globally. The General Data Protection Regulation (GDPR) in Europe and the California Consumer Privacy Act (CCPA) in the United States are examples of legislation aimed at protecting consumer privacy and granting individuals more control over their data. These regulations mandate transparency, consent, and data security, requiring businesses to be more accountable for their data practices.
Future regulations are likely to become even more stringent, focusing on algorithmic transparency and the potential for bias in AI-driven decision-making. Companies must proactively adapt to these evolving regulations to ensure compliance and maintain consumer trust. Failure to do so can result in significant fines and reputational damage.
Challenges and Opportunities in the Future Development of IoB
The future of IoB presents both significant challenges and exciting opportunities. One major challenge is the potential for bias in algorithms, which can perpetuate existing inequalities and lead to discriminatory outcomes. Addressing this requires careful data curation, algorithm design, and ongoing monitoring for bias. Another challenge is ensuring data security and privacy in the face of increasing cyber threats.
Robust security measures and ethical data handling practices are paramount. However, the opportunities are equally compelling. IoB can enable the development of highly personalized and effective customer experiences, leading to increased customer satisfaction and loyalty. It can also drive innovation in areas such as healthcare, personalized education, and smart city development. The key is to harness the power of IoB responsibly and ethically.
Ethical Considerations in the Development and Application of IoB Technologies
Ethical considerations are paramount in the development and application of IoB technologies. Transparency and user consent are crucial. Customers should be fully informed about how their data is being collected and used, and they should have the right to opt out or access and correct their data. Furthermore, the potential for manipulation and exploitation needs to be carefully addressed.
IoB systems should be designed to avoid manipulating customer behavior in unethical ways. Finally, fairness and equity must be prioritized, ensuring that IoB technologies do not exacerbate existing social inequalities. The development and implementation of IoB must be guided by strong ethical principles and a commitment to responsible innovation. A proactive and transparent approach is essential for building and maintaining consumer trust.
Wrap-Up: Decoding Customer Decisions The Rise Of Internet Of Behavior
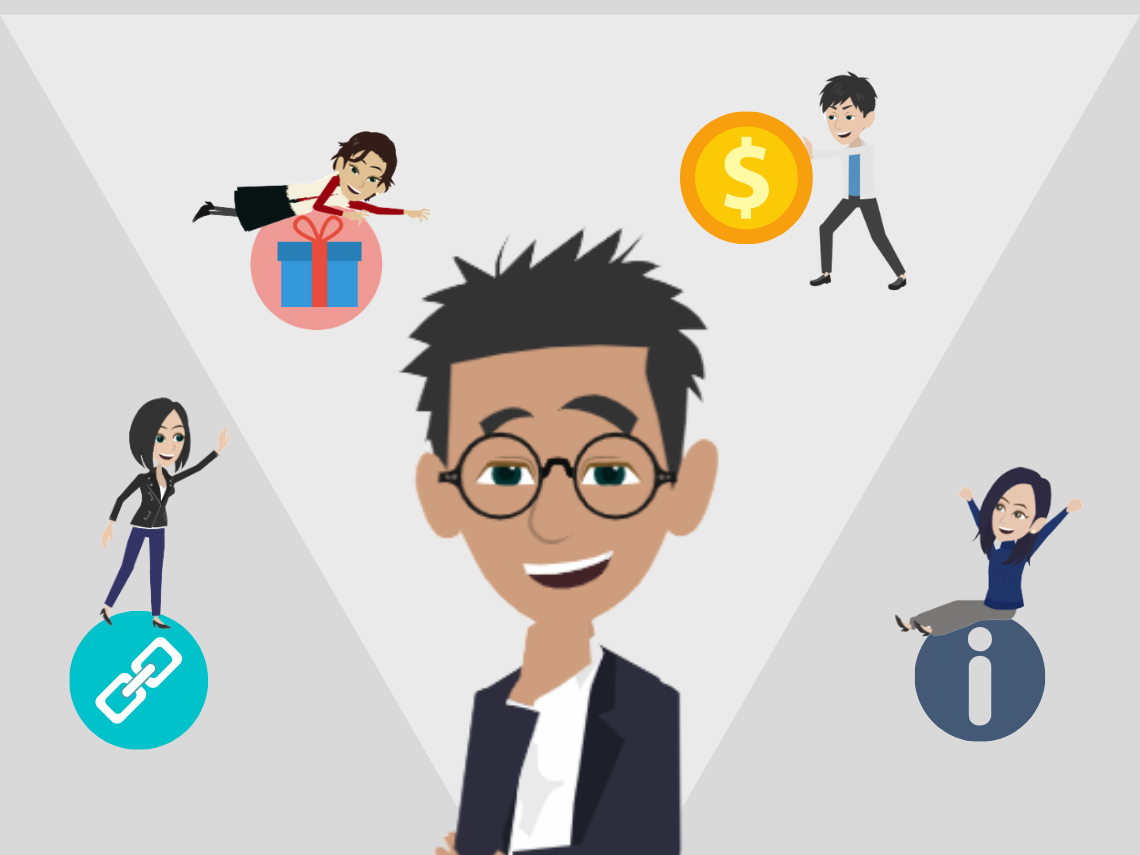
The Internet of Behavior is revolutionizing how we understand customer decisions. By ethically harnessing the power of behavioral data, businesses can create truly personalized experiences, optimize their offerings, and build stronger relationships with their customers. However, the ethical implications of IoB must be at the forefront of any implementation. The future of IoB is bright, but only if we navigate it responsibly, prioritizing data privacy and transparency.
So, are you ready to decode your customers’ decisions and unlock a world of opportunity?
User Queries
What are the biggest risks associated with IoB?
The biggest risks revolve around data privacy violations, algorithmic bias leading to unfair or discriminatory outcomes, and the potential for manipulation through highly targeted advertising.
How can I ensure ethical data collection and use within IoB?
Prioritize transparency with your customers, obtain informed consent, implement robust data security measures, and regularly audit your processes to identify and mitigate biases.
What are some common IoB data sources beyond the obvious?
Consider loyalty program data, CRM systems, sensor data (from smart devices), and even publicly available information like social media posts (with proper ethical considerations).
How can small businesses leverage IoB effectively?
Start with readily available data (website analytics, email engagement), focus on clear, measurable goals, and consider partnering with data analytics specialists if needed. Don’t try to do everything at once.