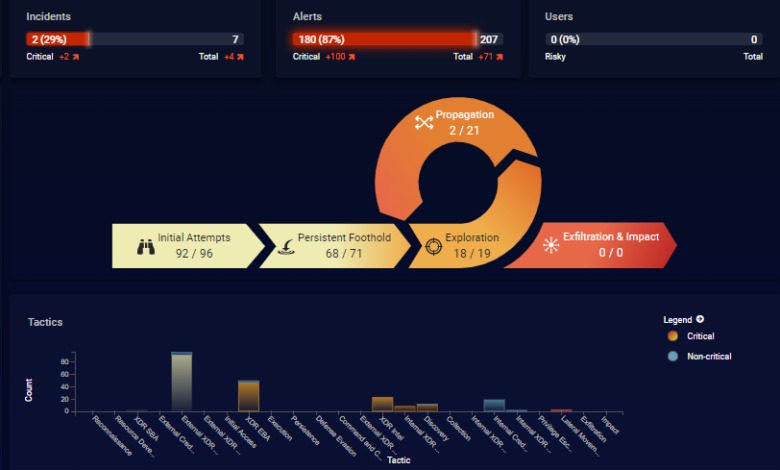
Does XDR Need a New Kill Chain?
Does XDR need a new kill chain? That’s the burning question we’re tackling today. The evolution of cyberattacks has outpaced traditional security models, leaving many wondering if the established kill chain framework still adequately reflects the reality of modern threats. We’ll delve into the limitations of current approaches, explore how XDR’s unified view changes the game, and even propose a redesigned kill chain specifically optimized for XDR’s unique capabilities.
Get ready for a deep dive into the future of threat detection and response!
This post will dissect the current limitations of the traditional kill chain in the face of increasingly sophisticated attacks. We’ll examine how XDR, with its ability to correlate data across various security tools, offers a more comprehensive approach. We’ll explore how a redesigned kill chain, tailored for XDR’s capabilities, can lead to faster threat detection, response, and ultimately, better protection.
We’ll also discuss the role of automation, AI, and human expertise in this new paradigm.
XDR Evolution and Current Kill Chain Limitations
The cybersecurity landscape is constantly evolving, with increasingly sophisticated threats demanding more robust defense mechanisms. Extended Detection and Response (XDR) represents a significant leap forward, but even this advanced technology faces limitations when confronted with the complexities of modern attacks. Understanding its evolution and the shortcomings of traditional kill chains is crucial for building truly effective security postures.XDR’s evolution is a story of integration and enhanced context.
It builds upon the foundations laid by endpoint detection and response (EDR), security information and event management (SIEM), network traffic analysis (NTA), and cloud security posture management (CSPM). While these technologies offered valuable insights into individual security domains, XDR unifies them, correlating data across various sources to provide a holistic view of the threat landscape. This unified view allows for faster threat detection, improved response capabilities, and a more efficient investigation process.
Shortcomings of Existing Kill Chains in Addressing Modern Threats
Traditional kill chains, like the Lockheed Martin Cyber Kill Chain, were designed to address more straightforward attacks. They often struggle with the speed and complexity of modern attacks, which often bypass several stages or utilize techniques that blur the lines between phases. For example, sophisticated attacks leveraging living-off-the-land binaries (LOLBins) can evade detection by using legitimate system tools for malicious purposes, making it difficult to pinpoint the precise stage of the attack within a traditional kill chain framework.
The speed of lateral movement in modern attacks also challenges the sequential nature of these kill chains, making it difficult to track the progression of the attack in real-time. Furthermore, the increasing use of evasion techniques, such as obfuscation and polymorphism, renders signature-based detection less effective.
Comparison of Traditional Security Approaches and XDR
Traditional security approaches, often relying on isolated security tools, lack the integrated view provided by XDR. A SIEM, for example, might detect suspicious activity, but it might lack the granular context provided by EDR to fully understand the impact on individual endpoints. XDR, by contrast, leverages the combined data to create a comprehensive picture, allowing for more accurate threat detection and faster response times.
The question of whether XDR needs a revamped kill chain is complex. It’s all about adapting to evolving threats, and that requires a flexible approach to development. Thinking about this, I was reminded of the innovative work happening with domino app dev the low code and pro code future , which highlights how agile development can improve response times.
Ultimately, a faster, more adaptable approach to both application development and threat response could be key to answering whether a new XDR kill chain is truly necessary.
This integrated approach leads to reduced mean time to detect (MTTD) and mean time to respond (MTTR), significantly mitigating the impact of security incidents. The centralized management and automated response capabilities of XDR also enhance operational efficiency, reducing the workload on security teams.
Limitations of Signature-Based Detection in the Context of XDR
While XDR significantly enhances threat detection, its reliance on signature-based detection, even in conjunction with other methods like behavioral analysis and machine learning, still presents limitations. Sophisticated attackers can easily modify malware to evade signature-based detection, rendering these signatures ineffective. This highlights the need for a multi-layered approach that combines signature-based detection with advanced threat detection techniques, such as anomaly detection and threat intelligence integration, to effectively combat the ever-evolving threat landscape.
A robust XDR system should incorporate multiple detection methods to account for both known and unknown threats, ensuring comprehensive protection.
Emerging Threat Landscape and XDR’s Role
The modern cyber threat landscape is evolving at an alarming rate, characterized by increasingly sophisticated and persistent attacks that easily bypass traditional security measures. These attacks leverage automation, exploit vulnerabilities in multiple systems, and often employ techniques designed to evade detection and analysis. The limitations of siloed security tools, which only offer a narrow view of the overall security posture, are painfully exposed in the face of these advanced threats.
XDR, with its unified approach, offers a powerful countermeasure.Sophisticated modern cyberattacks often involve a multi-stage process, combining various techniques to achieve their objectives. These attacks frequently leverage automation to scale their efforts, targeting multiple systems simultaneously. They often employ polymorphic malware, which constantly changes its characteristics to avoid signature-based detection. Furthermore, attackers frequently utilize social engineering tactics to gain initial access, leveraging human error as a key vulnerability.
The resulting damage can range from data breaches and financial loss to significant operational disruption and reputational harm.
Attack Circumvention of Traditional Security Measures
Traditional security tools, such as antivirus software, firewalls, and intrusion detection systems, often operate in isolation, lacking the context and visibility necessary to effectively counter sophisticated attacks. For instance, an attacker might use a spear-phishing email to deliver malware that bypasses antivirus software, then uses the compromised system to launch lateral movement across the network, evading firewall detection. The segmented nature of these tools hinders their ability to correlate events and identify the bigger picture, allowing attackers to remain undetected for extended periods.
XDR’s Unified Approach to Security
XDR’s core strength lies in its unified approach to security. By consolidating security data from various sources – endpoints, networks, cloud environments, and more – into a single platform, XDR provides a holistic view of the security posture. This unified view allows security teams to correlate events across different security domains, enabling faster threat detection and response. The platform’s ability to analyze data from disparate sources reveals attack patterns and behaviors that might otherwise remain hidden, enabling proactive threat hunting and improved incident response.
Real-world Attack Examples and XDR Improvement
Consider the NotPetya ransomware attack of 2017, which caused billions of dollars in damages globally. This attack leveraged a vulnerability in Ukrainian accounting software to spread rapidly across networks, utilizing legitimate system processes to evade detection. An XDR system, by correlating endpoint activity with network traffic and cloud logs, could have detected the unusual activity associated with the malware’s propagation far earlier, allowing for a faster response and significant mitigation of the damage.
Similarly, the SolarWinds attack, where malicious code was inserted into the company’s Orion software, highlights the need for cross-domain visibility. XDR could have identified anomalies in software updates and system behavior across multiple environments, enabling timely detection and prevention of the compromise.
Re-imagining the Kill Chain for XDR: Does Xdr Need A New Kill Chain
The traditional kill chain, while useful, struggles to keep pace with the sophisticated, multi-vector attacks enabled by modern threat actors. XDR, with its unified view of security data, offers the potential to fundamentally reshape how we approach threat detection and response. A redesigned kill chain, optimized for XDR’s capabilities, is crucial for effective threat mitigation in today’s complex landscape.
This new model shifts the focus from isolated events to a holistic understanding of the attack lifecycle, allowing for proactive and preventative measures.The following section details a redesigned kill chain model tailored to leverage the full potential of XDR, outlining its role at each stage and illustrating it with practical examples. This model emphasizes automation and proactive threat hunting, going beyond simple reactive responses.
XDR-Centric Kill Chain Model
This redesigned kill chain focuses on the interconnectedness of various attack vectors and the comprehensive visibility provided by XDR. Instead of focusing on discrete stages, this model emphasizes continuous monitoring and rapid response. The stages are less linear than the traditional model, acknowledging the often-overlapping and iterative nature of modern attacks.
Attacker Actions | XDR Detection Methods | Response Actions | Mitigation Strategies |
---|---|---|---|
Reconnaissance (e.g., network scanning, phishing emails) | Network traffic analysis, email security analysis, threat intelligence correlation | Alerting, blocking malicious traffic, quarantining suspicious emails | Implement robust network segmentation, employee security awareness training, advanced email filtering |
Weaponization (e.g., malware creation, exploit development) | Behavioral analysis, file reputation checks, sandbox analysis | Isolate infected systems, prevent malware execution | Regular software patching, application whitelisting, endpoint detection and response (EDR) integration |
Delivery (e.g., phishing attachments, malicious links) | URL analysis, file analysis, endpoint detection | Block malicious URLs, quarantine infected files | Secure email gateways, web filtering, user education |
Exploitation (e.g., exploiting vulnerabilities) | Vulnerability scanning, system log analysis, security information and event management (SIEM) integration | Patch vulnerable systems, contain the attack | Regular vulnerability assessments, penetration testing, automated patching |
Installation (e.g., malware installation, backdoor creation) | Endpoint detection and response (EDR), process monitoring | Isolate infected systems, remove malware | Strong endpoint security, regular backups, incident response plan |
Command and Control (C2) Communication (e.g., communication with attacker servers) | Network traffic analysis, DNS monitoring, threat intelligence | Block malicious communication, investigate C2 infrastructure | Firewall rules, intrusion detection systems (IDS), threat intelligence feeds |
Actions on Objectives (e.g., data exfiltration, system compromise) | Data loss prevention (DLP), user and entity behavior analytics (UEBA), file integrity monitoring | Contain the breach, recover data | Data encryption, access control, regular data backups |
Exfiltration (e.g., data theft) | Network traffic analysis, data loss prevention (DLP), cloud security posture management (CSPM) | Block data exfiltration attempts, investigate compromised accounts | Data encryption, access control, multi-factor authentication (MFA) |
XDR’s Impact on Threat Hunting and Incident Response
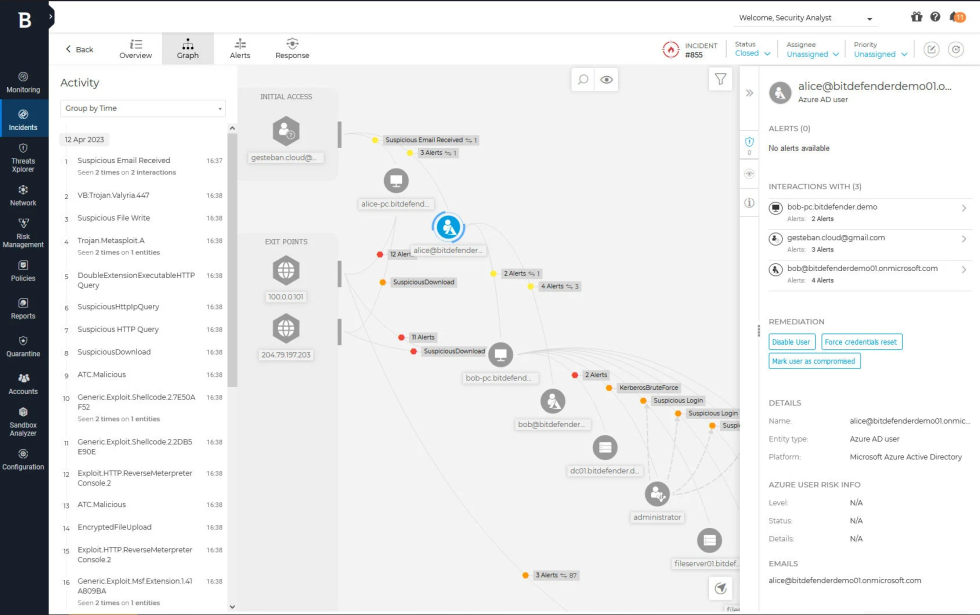
XDR’s unified view of security data across endpoints, networks, and cloud environments dramatically reshapes threat hunting and incident response. By correlating data from disparate sources, XDR provides a richer context for identifying threats, accelerating investigations, and significantly improving remediation speed. This consolidated approach allows security teams to move beyond reactive incident handling towards a more proactive and efficient security posture.XDR’s ability to analyze massive datasets and identify subtle anomalies provides a significant advantage in threat hunting and incident response.
This is especially critical in today’s complex threat landscape where attackers often employ sophisticated evasion techniques to blend in with legitimate activity. The speed and efficiency offered by XDR’s integrated approach directly translates to reduced dwell time and minimized damage from successful attacks.
Leveraging XDR Data for Proactive Threat Hunting
Effective threat hunting with XDR involves using its capabilities to proactively search for malicious activity, rather than simply reacting to alerts. This can be achieved by creating custom queries based on known indicators of compromise (IOCs), suspicious behaviors, or anomalies detected across different data sources. For instance, a security analyst might query the XDR platform to identify all devices that have communicated with a known malicious IP address in the last 24 hours, regardless of whether those devices have triggered traditional security alerts.
So, does XDR need a new kill chain to effectively handle the complexities of modern threats? I’ve been thinking a lot about this lately, especially considering the expanding attack surface. The rise of cloud-based threats necessitates a shift in our thinking, and understanding platforms like Bitglass is key; check out this insightful article on bitglass and the rise of cloud security posture management for more context.
Ultimately, a redefined kill chain for XDR might be crucial to stay ahead of the curve.
This proactive approach allows for the identification of threats before they escalate into major incidents. Another strategy involves using XDR’s machine learning capabilities to identify unusual patterns in network traffic or endpoint activity, such as unexpected outbound connections or unusual file executions. These anomalies can then be investigated further to determine whether they represent malicious activity. This proactive approach significantly reduces the time to detection and allows for a faster response.
Improving Incident Response Times with XDR Insights
XDR significantly accelerates incident response by providing a single pane of glass into the entire attack chain. When an alert is triggered, the security team can immediately access relevant data from all connected security tools, such as endpoint detection and response (EDR), network detection and response (NDR), cloud security posture management (CSPM), and security information and event management (SIEM) systems.
This eliminates the need to manually correlate data from multiple sources, saving valuable time during critical situations. For example, if a ransomware attack is detected on an endpoint, XDR can instantly provide information about the affected files, the attacker’s network activity, and the compromised user accounts, allowing for rapid isolation and containment of the threat. The consolidated view facilitates faster triage and prioritization of incidents based on severity and impact.
Facilitating Faster Threat Containment and Remediation
The integrated nature of XDR allows for swift containment and remediation of threats. Once a threat is identified, XDR can automate many of the response actions, such as isolating infected endpoints, blocking malicious network connections, and removing malicious files. This automation reduces the manual effort required by security analysts, allowing them to focus on more complex tasks. For example, XDR can automatically quarantine an infected endpoint, preventing further lateral movement of the attacker within the network.
Simultaneously, it can initiate a remediation process, such as automatically deleting malicious files or restoring the system from a backup. This automated response significantly reduces the time it takes to contain and remediate threats, minimizing the potential damage.
The Role of Automation and Orchestration in Enhancing XDR Effectiveness
Automation and orchestration are crucial for maximizing the benefits of XDR. By automating repetitive tasks, such as threat investigation, incident response, and remediation, security teams can free up valuable time and resources to focus on more strategic initiatives. Orchestration capabilities allow for the seamless integration of XDR with other security tools, enabling a more coordinated and effective security response.
For instance, an XDR platform can be configured to automatically trigger an incident response playbook when a specific threat is detected. This playbook could include steps such as isolating the infected endpoint, analyzing the threat, blocking malicious IPs, and notifying the relevant security teams. This level of automation significantly improves efficiency and reduces the risk of human error. The integration with SOAR (Security Orchestration, Automation, and Response) platforms further enhances this capability, allowing for more complex and sophisticated automated responses.
Data Sources and Integration in the New Kill Chain
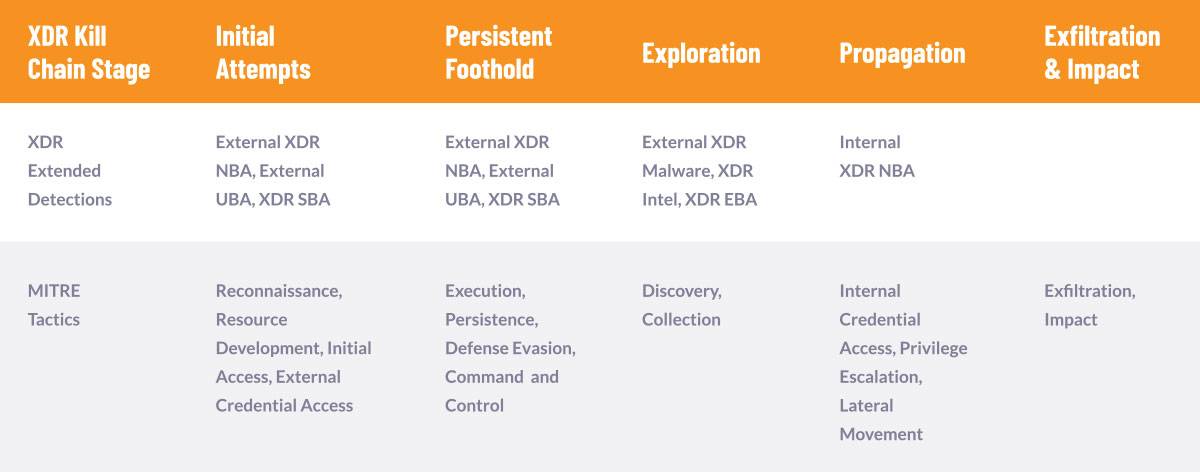
The effectiveness of an XDR solution hinges on its ability to ingest and correlate data from a wide array of sources. A comprehensive XDR platform transcends the limitations of single-vendor security tools, offering a unified view of the threat landscape across the entire IT infrastructure. This holistic approach allows for more accurate threat detection and response, leading to improved security posture.The process of integrating and correlating data from diverse sources is crucial for comprehensive threat analysis.
This involves not just collecting data but also enriching it, normalizing it, and establishing relationships between seemingly disparate events. Only then can a truly accurate picture of an attack emerge, enabling timely and effective remediation.
Data Sources Integrated within XDR
XDR solutions integrate data from a variety of sources to provide a holistic view of security events. These sources often include endpoint detection and response (EDR) systems, security information and event management (SIEM) platforms, network detection and response (NDR) tools, cloud security posture management (CSPM) solutions, and email security gateways. Each source contributes unique insights, which, when combined, offer a far more complete understanding of threats than any single source could provide.
For example, an EDR system might detect malicious activity on an endpoint, while a SIEM system might identify suspicious login attempts from a different location. Combining this information paints a clearer picture of a potential coordinated attack.
Data Correlation for Comprehensive Threat Analysis
Correlating data from diverse sources is a complex process requiring advanced analytics and machine learning. The goal is to identify patterns and relationships between events that might indicate a security breach. This process often involves analyzing timestamps, user accounts, IP addresses, and other relevant metadata to determine if events are related. For instance, a suspicious email detected by the email security gateway might be correlated with malicious activity detected on an endpoint shortly afterward, confirming a successful phishing attack.
Sophisticated algorithms are employed to weigh the significance of each event and its potential connection to other events, helping to prioritize alerts and focus investigation efforts.
Challenges Associated with Data Integration and Normalization
Integrating and normalizing data from diverse sources presents significant challenges. Different systems often use different formats, schemas, and terminologies, making it difficult to combine data seamlessly. Data volume can also be overwhelming, requiring efficient data processing and storage capabilities. Furthermore, ensuring data quality and accuracy is crucial for reliable threat analysis. Inconsistencies or errors in the data can lead to inaccurate conclusions and ineffective responses.
Data normalization, the process of transforming data into a consistent format, is vital to overcome these challenges. This involves converting data into a standardized format, cleaning up inconsistencies, and resolving conflicts between different data sources.
Data Flow within the New XDR Kill Chain
The data flow within the new XDR kill chain is a continuous process of data ingestion, correlation, analysis, and response. Data from various sources is ingested into the XDR platform in real-time or near real-time. This data undergoes enrichment and normalization before being subjected to advanced analytics and machine learning algorithms to identify potential threats. Suspicious activities are then prioritized and presented to security analysts, who can investigate further and take appropriate action.
This action might involve blocking malicious activity, quarantining infected systems, or initiating an incident response process. The entire process is iterative, with feedback loops used to improve the accuracy and effectiveness of the XDR solution over time. For example, analysts’ feedback on the accuracy of alerts can be used to refine the machine learning models, leading to improved threat detection in the future.
Future Directions and Considerations
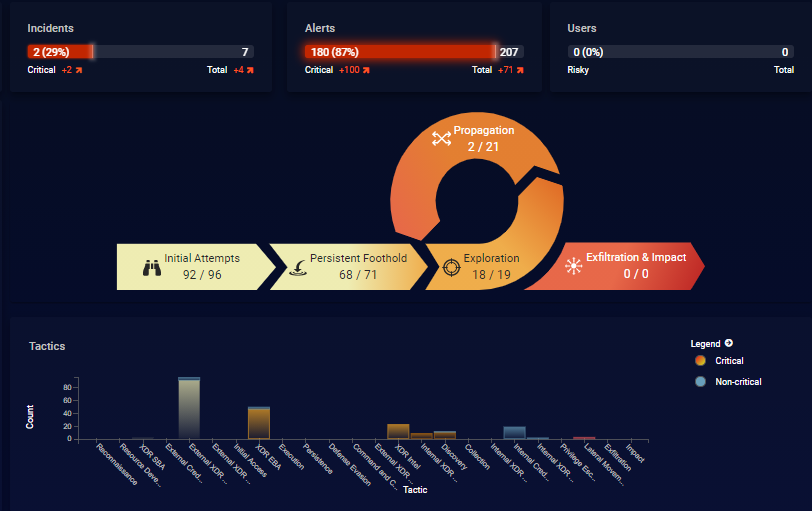
XDR is a rapidly evolving field, and its future trajectory hinges on several key factors: advancements in technology, the ever-shifting threat landscape, and the evolving needs of security professionals. Understanding these factors is crucial for organizations looking to leverage XDR to its fullest potential and prepare for the challenges ahead. The integration of AI/ML, the expansion of data sources, and the refinement of human-machine collaboration will all play significant roles in shaping the next generation of XDR.The convergence of emerging technologies and the increasing sophistication of cyberattacks will significantly influence XDR’s future development.
We’ll see a continued push for automation, enhanced threat detection capabilities, and a greater focus on proactive security measures rather than simply reacting to incidents. This shift necessitates a proactive approach to XDR implementation and ongoing refinement.
AI/ML Enhancements and Threat Detection
Artificial intelligence and machine learning are poised to revolutionize XDR. AI/ML algorithms can analyze vast quantities of data from diverse sources, identifying subtle patterns and anomalies that might escape human observation. This leads to more accurate threat detection, faster response times, and a reduction in false positives. For example, AI can be trained to recognize specific behavioral patterns indicative of ransomware attacks, allowing for preemptive mitigation strategies.
Moreover, ML algorithms can continuously learn and adapt to new threats, ensuring the XDR system remains effective against evolving attack techniques. This adaptive learning is critical in today’s dynamic threat landscape where new malware variants and attack vectors emerge constantly. Consider a scenario where a new zero-day exploit is discovered; an AI-powered XDR system could potentially identify the exploit’s signature and alert security teams before widespread damage occurs, something a rule-based system might miss.
Challenges and Considerations for XDR Implementation, Does xdr need a new kill chain
Implementing and managing an XDR solution presents several challenges. One key hurdle is data integration. Consolidating data from various sources – endpoints, networks, cloud environments, and security tools – requires careful planning and robust integration capabilities. Another significant challenge lies in ensuring data security and privacy. XDR solutions handle sensitive information, and organizations must comply with relevant regulations (like GDPR or CCPA) while maintaining the integrity and confidentiality of their data.
Finally, managing the complexity of an XDR system requires skilled personnel with expertise in both security and data analysis. Organizations need to invest in training and upskilling their security teams to effectively manage and leverage the capabilities of an XDR platform. Failure to adequately address these challenges can result in suboptimal performance, security vulnerabilities, and increased operational costs.
The Human-Machine Partnership in XDR
While automation is a cornerstone of XDR, human expertise remains indispensable. While AI/ML can automate many aspects of threat detection and response, human analysts are still needed to interpret complex situations, investigate false positives, and make critical decisions. The ideal scenario is a collaborative partnership between humans and machines, where AI/ML assists human analysts by automating repetitive tasks and providing insights, allowing human analysts to focus on more complex and strategic tasks.
This collaborative approach maximizes the effectiveness of the XDR system and minimizes the risk of human error. Think of a scenario where the XDR system flags a potential insider threat. A human analyst would be needed to investigate the alert, verify the findings, and determine the appropriate course of action. This human oversight is crucial to ensure accuracy and prevent false alarms from disrupting operations.
Final Wrap-Up
So, does XDR need a new kill chain? The answer, unequivocally, is yes. The rapid evolution of cyberattacks necessitates a more dynamic and adaptable framework, and XDR, with its integrated approach and ability to leverage diverse data sources, provides the foundation for this evolution. By reimagining the kill chain with XDR at its core, we can move towards a more proactive, efficient, and effective cybersecurity posture.
The future of security lies in embracing these changes and adapting to the ever-shifting threat landscape.
Clarifying Questions
What are the main limitations of the traditional kill chain in the context of XDR?
Traditional kill chains often struggle with the speed and complexity of modern attacks, particularly those that employ evasion techniques and lateral movement. They also typically lack the integrated view offered by XDR, making correlation of data across different security layers difficult.
How does AI/ML impact the effectiveness of an XDR-centric kill chain?
AI/ML can significantly enhance an XDR-centric kill chain by automating threat detection, improving anomaly detection, accelerating incident response, and enabling more proactive threat hunting.
What are some common challenges in implementing an XDR solution?
Challenges include data integration complexity, the need for skilled personnel to manage the system, and the potential for alert fatigue due to the volume of data processed.
What is the role of human expertise in an XDR-driven security strategy?
While automation is crucial, human expertise remains essential for interpreting complex threat scenarios, validating alerts, and making critical decisions, particularly in cases requiring nuanced judgment.