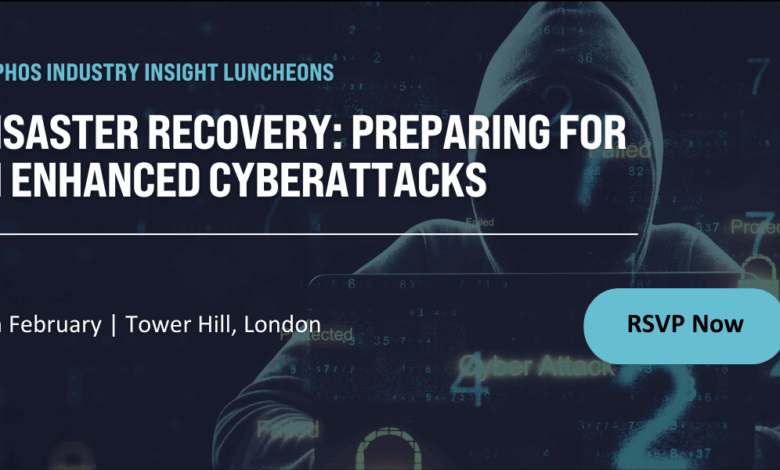
Elevating Disaster Recovery in Cybersecurity with AI-Driven Hyperautomation
Elevating disaster recovery in cybersecurity with ai driven hyperautomation – Elevating Disaster Recovery in Cybersecurity with AI-driven Hyperautomation: Imagine a world where cybersecurity breaches don’t cripple your business, but instead trigger a swift, automated recovery. That’s the promise of AI-driven hyperautomation, a game-changer in disaster recovery. This isn’t just about faster response times; it’s about building a resilient system that proactively anticipates and mitigates threats, learning and adapting with every challenge.
We’re diving deep into how this technology is revolutionizing how we approach cybersecurity disasters, moving from reactive firefighting to proactive prevention.
This post explores the core components of AI-driven hyperautomation in disaster recovery, showcasing its advantages over traditional methods. We’ll delve into how AI enhances threat detection and response, automates recovery processes, optimizes data backup and recovery, strengthens security posture, and even predicts potential disasters. Think of it as your ultimate guide to building a fortress against cyberattacks, one that’s not only strong but also incredibly smart and adaptable.
Defining AI-Driven Hyperautomation in Disaster Recovery
In today’s interconnected world, cybersecurity threats are constantly evolving, making robust disaster recovery (DR) plans more critical than ever. Traditional DR methods, while effective, often struggle to keep pace with the speed and complexity of modern attacks. This is where AI-driven hyperautomation steps in, offering a transformative approach to bolstering cybersecurity resilience. It combines the power of artificial intelligence with the efficiency of hyperautomation to create a significantly more agile and responsive DR strategy.AI-driven hyperautomation in cybersecurity disaster recovery integrates various technologies to automate and accelerate the entire DR lifecycle.
This includes AI algorithms for threat detection and response, machine learning for predictive analysis, robotic process automation (RPA) for automating repetitive tasks, and orchestration tools to manage the entire process. The synergy of these technologies enables faster recovery times, reduced downtime, and improved overall security posture.
AI-driven hyperautomation is revolutionizing cybersecurity disaster recovery, making responses faster and more efficient. This speed and efficiency are crucial, especially when considering the complexities of modern application development; check out this insightful piece on domino app dev the low code and pro code future to see how streamlined development impacts overall system resilience. Ultimately, integrating these advancements ensures a robust, adaptable disaster recovery plan capable of handling unforeseen challenges.
Core Components of AI-Driven Hyperautomation in Disaster Recovery
AI-driven hyperautomation in DR relies on several key components working in concert. First, AI and machine learning algorithms analyze security logs, network traffic, and other data sources to identify potential threats and predict potential points of failure. This proactive approach allows for preemptive actions to mitigate risks. Next, RPA automates repetitive tasks such as system backups, data replication, and the deployment of virtual machines.
Finally, orchestration platforms integrate and manage all these automated processes, ensuring seamless execution and coordination during a disaster. This integrated approach ensures that the entire DR process is automated and efficient, minimizing human intervention and potential errors.
Benefits of Integrating AI and Hyperautomation for Enhanced Disaster Recovery Processes, Elevating disaster recovery in cybersecurity with ai driven hyperautomation
The integration of AI and hyperautomation offers several significant advantages. Firstly, it dramatically reduces recovery time objectives (RTOs) and recovery point objectives (RPOs). By automating critical tasks, the system can respond to and recover from incidents much faster than traditional methods. Secondly, it improves the accuracy and efficiency of the DR process, minimizing human error and ensuring consistent application of recovery procedures.
Thirdly, AI-driven hyperautomation allows for more proactive and predictive disaster recovery. By analyzing historical data and identifying patterns, the system can predict potential failures and proactively take steps to mitigate them. This shift from reactive to proactive DR is a game-changer. Finally, it enhances scalability and flexibility, allowing organizations to easily adapt their DR plans to changing business needs and evolving threat landscapes.
Comparison of Traditional Disaster Recovery Methods with AI-Driven Hyperautomation Approaches
Traditional DR methods typically rely heavily on manual processes and predefined procedures. This often leads to slow recovery times, potential human errors, and a lack of adaptability to unexpected events. In contrast, AI-driven hyperautomation significantly accelerates the process, reduces errors, and adapts dynamically to the specific nature of the disaster. For example, while traditional methods might involve manual restoration of systems from backups, AI-driven hyperautomation can automatically identify the affected systems, orchestrate the restoration process, and even initiate failover to redundant systems without human intervention.
This difference in speed and efficiency is crucial in minimizing the impact of a cybersecurity incident.
Examples of Specific AI and Automation Tools Used in Disaster Recovery
Several tools exemplify the power of AI-driven hyperautomation in DR. For instance, Splunk’s machine learning capabilities can be used to detect anomalies and predict potential security breaches, allowing for proactive mitigation. Similarly, automation platforms like Ansible and Puppet can automate the deployment and configuration of systems during a recovery process. Furthermore, cloud-based DR solutions like AWS Disaster Recovery and Azure Site Recovery leverage AI and automation to streamline the recovery process and offer scalability.
These are just a few examples; many other vendors offer similar tools and services.
Enhancing Threat Detection and Response with AI
AI is revolutionizing disaster recovery by significantly boosting the speed and accuracy of threat detection and response. Traditional methods often rely on reactive measures, leaving organizations vulnerable during critical periods. AI, however, provides proactive capabilities, enabling faster identification of threats and more efficient resource allocation during a disaster. This proactive approach minimizes downtime and accelerates the recovery process.AI algorithms excel at analyzing massive datasets far exceeding human capabilities, identifying subtle patterns indicative of malicious activity that might otherwise go unnoticed.
This enhanced detection capacity is particularly crucial during a disaster when systems are stressed and security breaches are more likely. Furthermore, AI can prioritize critical systems for recovery based on business impact, ensuring that essential services are restored swiftly.
AI-Enhanced Threat Detection Speed and Accuracy
AI algorithms, particularly machine learning models, can process security logs, network traffic data, and system events at speeds impossible for human analysts. This allows for real-time threat detection, enabling immediate response and mitigation before significant damage occurs. For instance, an AI system could detect a surge in unusual login attempts from a specific geographic location, immediately flagging it as a potential Distributed Denial of Service (DDoS) attack during a disaster, when network resources are already strained.
The accuracy of detection is also enhanced because AI can learn from past incidents and adapt to new attack vectors, improving its ability to distinguish between genuine threats and false positives. This reduces the burden on security teams and allows them to focus on critical tasks.
AI’s Role in Prioritizing Critical Systems
During a disaster recovery, efficient prioritization of systems is paramount. AI can analyze dependencies between systems, assess their criticality to business operations, and automatically prioritize recovery efforts accordingly. This ensures that essential services, like payment processing or customer support systems, are restored first, minimizing financial losses and reputational damage. For example, an AI-driven system could determine that the customer database is more critical than the marketing email server and allocate resources accordingly, ensuring business continuity.
Hypothetical Scenario: AI-Accelerated Incident Response
Imagine a major earthquake affecting a data center. Traditional methods would involve manual checks of various systems, a time-consuming and potentially inaccurate process. However, with AI, the system automatically detects the outage, identifies the affected systems (including backups and replication status), and assesses the impact on business operations. The AI then automatically initiates a failover to a geographically dispersed backup site, prioritizing the restoration of critical applications.
Simultaneously, it analyzes network traffic for malicious activity exploiting the disaster, immediately blocking suspicious connections. The entire process, from detection to recovery, is significantly faster and more efficient than manual intervention, minimizing downtime and data loss.
Best Practices for Integrating AI-Powered Threat Intelligence into Disaster Recovery Plans
Integrating AI-powered threat intelligence into disaster recovery plans requires a strategic approach. This includes:
- Data Integration: Ensure that your AI system has access to relevant data sources, including security logs, network traffic, and system events. This requires careful planning and integration with existing security infrastructure.
- AI Model Selection: Choose AI models suitable for your specific needs and environment. Consider factors such as data volume, complexity, and the types of threats you expect to encounter.
- Regular Testing and Updates: Regularly test your AI-powered disaster recovery system to ensure its effectiveness. Keep your AI models updated with the latest threat intelligence to maintain their accuracy and relevance.
- Human Oversight: While AI can automate many tasks, human oversight remains crucial. Security professionals should monitor AI alerts, validate findings, and make critical decisions when necessary.
Automating Recovery Processes
Automating disaster recovery (DR) tasks is no longer a luxury; it’s a necessity in today’s hyper-connected world. AI-driven hyperautomation offers a powerful solution, enabling organizations to significantly reduce recovery time objectives (RTOs) and recovery point objectives (RPOs). By orchestrating a series of automated actions, we can move from reactive, manual recovery to a proactive, self-healing system. This shift minimizes downtime, reduces the impact of disruptions, and frees up valuable human resources to focus on strategic initiatives.This section details how to automate key DR tasks using hyperautomation, highlighting potential challenges and their solutions.
We will build a step-by-step guide and illustrate the process using a flowchart.
Automated Disaster Recovery Task Flow
The following table Artikels a typical automated disaster recovery process, showing how different hyperautomation tools can be used to streamline each stage. This is a simplified example, and the specific tools and steps may vary depending on your infrastructure and specific needs. Remember, a robust DR plan should be meticulously tested and updated regularly.
Task | Description | Automation Tool | Expected Outcome |
---|---|---|---|
Event Detection | Identify a triggering event (e.g., system failure, security breach). | SIEM, Monitoring tools integrated with RPA | Automated alert and initiation of DR plan. |
System Shutdown/Failover | Gracefully shut down affected systems and initiate failover to a backup system. | Orchestration platform (e.g., Ansible, Terraform), Cloud provider tools (e.g., AWS, Azure) | Minimized data loss during transition to backup systems. |
Data Replication/Restoration | Replicate or restore data from backups to the backup system. | Backup and recovery software (e.g., Veeam, Commvault), Cloud storage services | Data availability restored on the backup system. |
Application Startup | Start critical applications on the backup system. | Configuration management tools (e.g., Puppet, Chef), Container orchestration (e.g., Kubernetes) | Applications become operational on the backup system. |
Testing and Validation | Verify the functionality of restored systems and applications. | Automated testing frameworks, monitoring tools | Confirmation that the DR plan has successfully restored functionality. |
Challenges and Solutions in Automating Disaster Recovery
Automating DR processes isn’t without its hurdles. One major challenge is the complexity of integrating various systems and tools. Different components might use disparate protocols and APIs, requiring significant effort in establishing seamless communication and data exchange. A robust solution involves using a central orchestration platform that can manage and coordinate all the different components. This platform should offer a unified interface and standardized communication protocols to ensure smooth integration.
Another challenge is ensuring the accuracy and reliability of automated actions. Incorrect configuration or unforeseen errors could lead to further complications. Thorough testing and validation of automated workflows are crucial to mitigate this risk. Implementing robust logging and monitoring capabilities is also essential for identifying and rectifying any errors that might occur during the recovery process. Finally, the initial investment in implementing hyperautomation for DR can be significant.
Elevating disaster recovery in cybersecurity with AI-driven hyperautomation is crucial in today’s complex landscape. A key component of this is ensuring robust cloud security, and understanding how to manage that effectively is paramount. Check out this insightful piece on bitglass and the rise of cloud security posture management to see how better cloud security directly impacts your disaster recovery plans.
Ultimately, a strong cloud security posture is a fundamental building block for resilient AI-driven disaster recovery strategies.
However, the long-term cost savings in terms of reduced downtime and human intervention often outweigh the upfront investment.
Minimizing Human Intervention with Hyperautomation
Hyperautomation significantly reduces human intervention by automating repetitive and time-consuming tasks. For example, instead of manually shutting down servers and initiating failover, these actions can be automated through scripting and orchestration tools. Similarly, data restoration, application startup, and testing can all be automated, reducing the risk of human error and speeding up the recovery process. This allows human operators to focus on higher-level tasks such as monitoring the recovery process, troubleshooting any unexpected issues, and coordinating with stakeholders.
A well-designed hyperautomation system can even automatically notify relevant personnel of the recovery progress and any potential issues, further reducing the need for constant human monitoring. This shift towards automation ensures a faster, more efficient, and less error-prone disaster recovery process.
Improving Data Backup and Recovery: Elevating Disaster Recovery In Cybersecurity With Ai Driven Hyperautomation
AI-driven hyperautomation is revolutionizing data backup and recovery, moving beyond traditional methods to offer significantly faster, more efficient, and more resilient solutions. By leveraging machine learning and automation, organizations can drastically reduce recovery time objectives (RTOs) and recovery point objectives (RPOs), minimizing the impact of potential disasters. This enhanced approach not only safeguards valuable data but also streamlines the entire backup and recovery lifecycle.AI can optimize data backup strategies by intelligently analyzing data usage patterns, identifying redundancy, and predicting future storage needs.
This allows for the creation of more efficient backup schedules and the allocation of resources only where needed, reducing storage costs and improving backup speeds. For example, AI can identify data that is rarely accessed and prioritize backups of frequently used data, ensuring critical information is protected first. This intelligent prioritization contrasts sharply with traditional methods which often perform blanket backups, leading to wasted time and resources.
AI-Powered Data Deduplication and Compression
AI algorithms significantly enhance data deduplication and compression, resulting in smaller backup sizes and faster backup and restore times. Traditional deduplication methods rely on simple hashing techniques, whereas AI-powered solutions can leverage machine learning to identify and eliminate duplicate data more effectively, even across different file formats or versions. For instance, tools can learn to recognize semantically similar data, identifying duplicates that might be missed by simpler methods.
Furthermore, AI-driven compression techniques can dynamically adjust compression levels based on data type and importance, optimizing both storage space and recovery speed. Examples of such tools include those employing advanced algorithms like deep learning to achieve higher compression ratios than traditional methods. These tools often integrate seamlessly into existing backup infrastructures.
AI’s Role in Data Integrity and Loss Prevention
AI plays a crucial role in ensuring data integrity and preventing data loss during the recovery process. AI-powered anomaly detection systems can identify inconsistencies or corruption in backups before they lead to data loss. These systems continuously monitor backup data for anomalies, such as unusual file sizes or checksum errors, alerting administrators to potential problems and allowing for proactive intervention.
Furthermore, AI can automate the validation of backup data, verifying its integrity and ensuring that recovery will be successful. This proactive approach to data integrity minimizes the risk of data loss during recovery and significantly reduces the time spent on manual verification. In contrast to traditional methods relying on manual checks, AI provides continuous monitoring and automated validation, significantly increasing reliability.
Automating Data Restoration Processes with Hyperautomation
Hyperautomation leverages a combination of technologies, including AI, robotic process automation (RPA), and machine learning, to automate the entire data restoration process. This involves automating tasks such as identifying the necessary data, selecting the appropriate recovery point, initiating the restoration process, and verifying the data’s integrity. For example, hyperautomation can automatically trigger a recovery process in response to a disaster event, selecting the optimal recovery point based on predefined parameters and automatically initiating the restoration to a pre-configured environment.
This automated approach eliminates manual intervention, reducing recovery time and minimizing the risk of human error. The ability to orchestrate and automate these complex processes ensures faster recovery times and higher data availability. It also allows for the testing and validation of recovery plans without disrupting live systems.
Strengthening Security Posture through Automation
Disaster recovery isn’t just about restoring systems; it’s about restoring a secure operational environment. AI-driven hyperautomation provides a powerful toolset to significantly bolster our security posture during and after a disaster, minimizing the window of vulnerability and ensuring a swift, secure return to normal operations. By automating key security functions, we can reduce human error, accelerate response times, and enhance overall resilience.Hyperautomation offers a proactive approach to security during disaster recovery.
Instead of reacting to breaches after the fact, we can use automated systems to preemptively mitigate risks and rapidly respond to emerging threats. This proactive strategy shifts the focus from damage control to prevention and rapid recovery, significantly improving the overall security posture of the organization.
Automated Security Patching and Vulnerability Management Enhance Resilience
Automated patching and vulnerability management are crucial components of a robust security strategy. Traditional manual patching processes are time-consuming and prone to errors, leaving systems vulnerable for extended periods. Hyperautomation allows for the automated scanning of systems for vulnerabilities, the prioritization of patches based on risk level, and the deployment of those patches across the entire infrastructure – all with minimal human intervention.
This significantly reduces the attack surface and minimizes the risk of exploitation during a recovery period. For example, imagine a scenario where a critical vulnerability is discovered shortly after a disaster. A hyperautomated system could immediately identify affected systems, download and apply the necessary patches, and verify the success of the patch deployment – all within minutes, preventing further exploitation.
Automated System Integrity Verification Post-Disaster Recovery
After a disaster, verifying the integrity of recovered systems is paramount. Manually checking each system for inconsistencies is tedious and error-prone. AI-driven hyperautomation can automate this process by implementing checksum verification, file integrity monitoring, and system log analysis. These automated checks compare the recovered system’s state to its pre-disaster baseline, identifying any discrepancies or signs of tampering.
A discrepancy report is generated, enabling swift remediation. For instance, an automated system could compare the checksums of critical system files before and after recovery, flagging any inconsistencies that might indicate data corruption or malicious modification. This ensures the recovered systems are not only functional but also secure.
Key Security Measures Automated by AI and Hyperautomation
Automating security measures significantly improves response times and reduces human error. The following list Artikels key security functions that benefit from AI-driven hyperautomation:
- Automated vulnerability scanning and patching
- Real-time threat detection and incident response
- Automated security information and event management (SIEM) analysis
- Automated malware analysis and containment
- Automated user access control and privilege management
- Automated system hardening and configuration management
- Automated data backup and recovery verification
- Automated security log monitoring and analysis
Implementing these automated security measures minimizes the risk of exploitation, accelerates recovery times, and strengthens the overall security posture during and after a disaster. The speed and accuracy offered by AI-driven hyperautomation are critical in minimizing downtime and preventing further damage.
Predictive Analytics for Disaster Prevention
Predictive analytics, powered by AI, is transforming disaster recovery in cybersecurity by moving from reactive to proactive strategies. Instead of simply responding to breaches and outages, organizations can now leverage AI to anticipate and mitigate potential threats before they materialize, significantly reducing downtime and financial losses. This shift towards predictive capabilities is crucial in today’s complex threat landscape.AI-powered predictive analytics leverages machine learning algorithms to analyze vast amounts of data, identifying patterns and anomalies indicative of potential future events.
This allows for the prediction of cyberattacks, system failures, and other disruptive events, enabling proactive mitigation efforts.
AI Models for Predicting Cyber Threats and System Failures
Several AI models are effectively used for predicting cyber threats and system failures. For example, anomaly detection algorithms monitor network traffic and system logs, flagging unusual activity that might indicate a developing attack or impending hardware failure. These algorithms often employ techniques like clustering and classification to identify deviations from established baselines. Another powerful approach is time-series forecasting, which uses historical data to predict future system performance and identify potential bottlenecks or points of failure.
For instance, analyzing historical CPU usage and memory consumption can predict potential system crashes or slowdowns, allowing for proactive resource allocation or system upgrades. Furthermore, machine learning models can be trained on datasets of known vulnerabilities and exploits to predict which systems are most at risk and prioritize patching efforts. This proactive approach helps minimize the window of vulnerability, making attacks less likely to succeed.
Improving Proactive Disaster Mitigation Strategies with Predictive Analytics
Predictive analytics enhances proactive disaster mitigation by providing actionable insights. Instead of reacting to an incident, organizations can use predictions to prioritize security investments, allocate resources effectively, and implement preventative measures. For example, if a predictive model identifies a high probability of a ransomware attack targeting a specific system, security teams can proactively strengthen that system’s defenses, perhaps by implementing multi-factor authentication or deploying advanced endpoint detection and response (EDR) solutions.
Similarly, predicting a potential hardware failure allows for scheduled maintenance or replacement, minimizing disruption. This proactive approach significantly reduces the impact of disasters, improving overall business continuity.
Integrating Predictive Analytics into Existing Disaster Recovery Plans
Integrating predictive analytics into existing disaster recovery plans involves several key steps. First, organizations need to identify the data sources relevant to their risk profile, such as network logs, security information and event management (SIEM) data, and system performance metrics. This data is then fed into AI models, which generate predictions about potential disasters. These predictions are then incorporated into existing disaster recovery plans, updating procedures and resource allocation based on the predicted risks.
For example, a prediction of a potential denial-of-service (DoS) attack might lead to the implementation of additional network capacity or the activation of a failover system. Regularly reviewing and updating the AI models with new data is critical to maintain accuracy and relevance. The integration process should be iterative, continuously refining the models and incorporating feedback from security teams and operational staff to ensure the predictions are accurate and actionable.
This ongoing refinement process ensures that the disaster recovery plan remains relevant and effective in the face of evolving threats and system changes.
Monitoring and Reporting
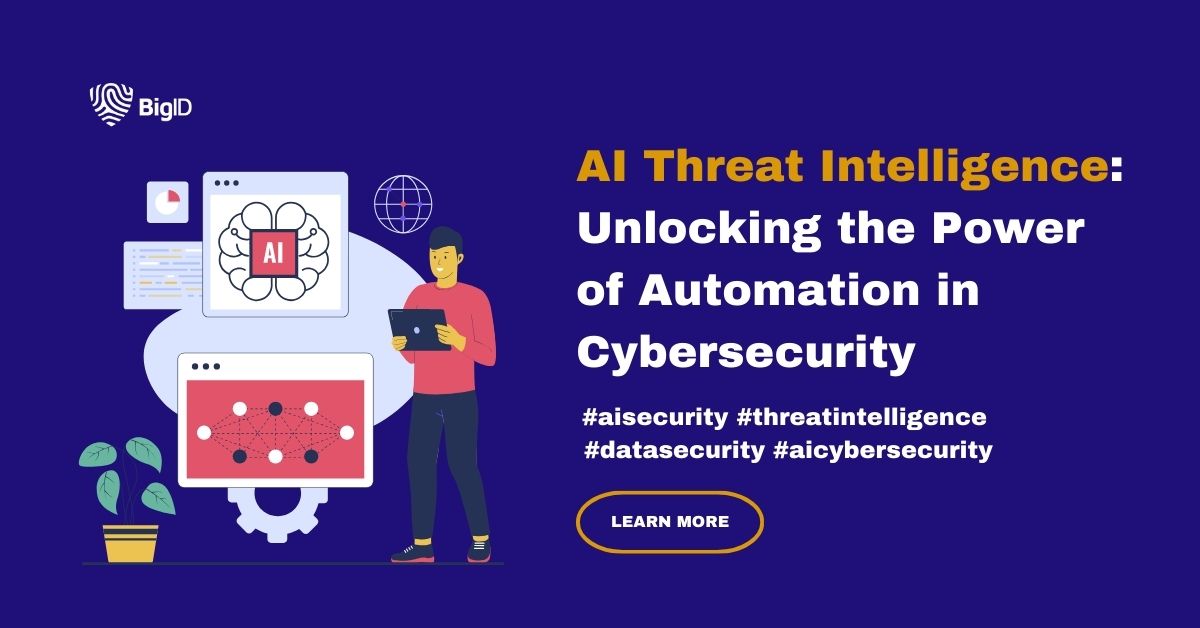
AI-driven hyperautomation fundamentally transforms disaster recovery monitoring and reporting, moving from reactive to proactive and predictive capabilities. Instead of relying solely on manual checks and sporadic reports, organizations gain real-time visibility into their recovery progress and system health, significantly improving response times and minimizing downtime. This enhanced monitoring allows for immediate identification of bottlenecks and potential issues, enabling faster remediation and reducing the overall impact of a disaster.The integration of AI allows for the continuous analysis of vast amounts of data from various sources, identifying anomalies and potential threats far more efficiently than humanly possible.
This predictive capability allows for preemptive actions to mitigate risks, even before a disaster strikes. Hyperautomation ensures that these insights are not only identified but also acted upon automatically, streamlining the entire monitoring and response process.
Real-time System Monitoring and Alerting
AI algorithms can continuously monitor critical systems, identifying deviations from established baselines and triggering alerts based on pre-defined thresholds. This real-time monitoring provides immediate notification of potential problems, allowing for rapid intervention and preventing minor issues from escalating into major outages. For example, a sudden spike in CPU utilization on a critical server might trigger an alert, allowing administrators to investigate and resolve the issue before it impacts overall system performance.
This proactive approach is a significant improvement over traditional monitoring methods that rely on periodic checks and often miss subtle yet critical indicators of impending problems.
Automated Reporting and Dashboarding
Hyperautomation facilitates the automatic generation of comprehensive reports, providing stakeholders with up-to-the-minute information on the status of the disaster recovery process. These reports can be customized to highlight key metrics and provide actionable insights. This eliminates the time-consuming manual process of compiling reports, freeing up personnel to focus on more critical tasks. Automated reporting is especially crucial during a crisis, enabling faster and more informed decision-making.
Sample Recovery Metrics Dashboard
Metric | Value |
---|---|
System Restoration Time | 15 minutes |
Data Recovery Rate | 99.9% |
Application Availability | 100% |
Number of Critical Alerts | 0 |
Recovery Cost | $5,000 |
This dashboard provides a clear and concise overview of key recovery metrics, enabling quick assessment of the recovery progress and identification of any areas needing attention. The responsive two-column layout ensures readability across various devices.
Automated Reporting for Faster Decision-Making
Automated reporting plays a vital role in accelerating decision-making during a crisis. Real-time data and insights empower incident response teams to prioritize actions effectively, allocate resources optimally, and implement the most appropriate recovery strategies. The ability to quickly access and analyze crucial information reduces response times and minimizes the impact of the disaster. For instance, an automated report showing a critical application is unavailable can trigger immediate actions to restore service, preventing significant business disruption.
Key Performance Indicators (KPIs) for AI-Driven Disaster Recovery
Several KPIs can effectively measure the performance and effectiveness of AI-driven disaster recovery systems. These include Recovery Time Objective (RTO), Recovery Point Objective (RPO), Mean Time To Recovery (MTTR), and the cost of recovery. Tracking these metrics over time allows organizations to continuously improve their disaster recovery strategies and demonstrate the return on investment (ROI) of AI and hyperautomation.
For example, a reduction in MTTR from 4 hours to 30 minutes demonstrates a significant improvement in the efficiency and effectiveness of the disaster recovery process. Similarly, a lower RTO and RPO indicates improved system resilience and data protection.
Last Word
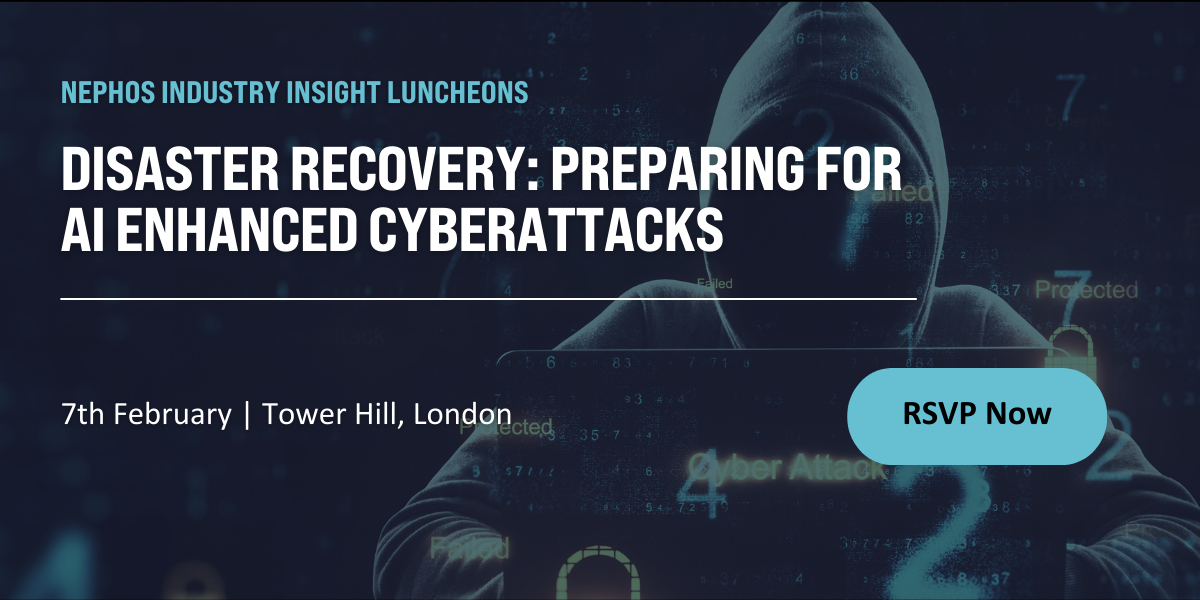
In a world of ever-evolving cyber threats, AI-driven hyperautomation isn’t just a luxury—it’s a necessity. By integrating AI and automation into your disaster recovery strategy, you’re not just reacting to attacks; you’re building a system that learns, adapts, and proactively defends against future threats. The journey to a truly resilient cybersecurity posture starts with embracing these powerful tools. This means shifting from a mindset of damage control to one of proactive prevention and rapid, automated recovery.
The future of cybersecurity is intelligent, automated, and undeniably resilient.
FAQ Corner
What are the potential downsides of implementing AI-driven hyperautomation in disaster recovery?
While powerful, AI-driven hyperautomation requires significant upfront investment in infrastructure, software, and skilled personnel. There’s also the risk of over-reliance on automation, neglecting the importance of human oversight and expertise. Careful planning and risk assessment are crucial for successful implementation.
How can I ensure the security of the AI and automation tools themselves?
The security of your AI and automation tools is paramount. This requires robust security measures, including regular patching, access control, and security monitoring. Consider employing techniques like multi-factor authentication and encryption to protect these critical components of your disaster recovery system.
What’s the difference between AI and hyperautomation in this context?
AI provides the intelligence—analyzing data, identifying patterns, and making decisions. Hyperautomation takes this intelligence and uses it to automate complex processes, orchestrating multiple tools and systems to execute recovery tasks efficiently and consistently.