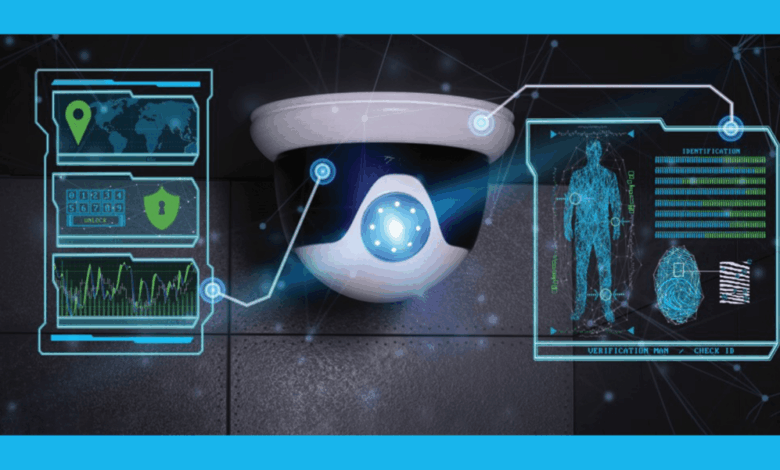
Ensuring a Secure Future Global AI Security Guidelines
Ensuring a secure future global guidelines for ai security – Ensuring a Secure Future: Global AI Security Guidelines is a topic that’s both incredibly exciting and deeply unsettling. We’re on the cusp of a technological revolution driven by artificial intelligence, but this incredible power comes with immense responsibility. The potential benefits are enormous – from curing diseases to tackling climate change – but unchecked AI development poses serious risks, from job displacement to autonomous weapons systems.
This exploration dives into the crucial need for international cooperation and the development of robust, globally accepted guidelines to ensure AI benefits humanity safely and ethically.
The challenge lies in balancing innovation with safety. How do we foster the growth of this transformative technology while mitigating the potential for misuse? This post examines the multifaceted aspects of AI security, from technical safeguards and ethical considerations to international collaboration and the crucial role of education and awareness. We’ll delve into the complexities of creating a framework for global governance, exploring potential solutions and addressing the inevitable hurdles along the way.
Defining AI Security in a Global Context
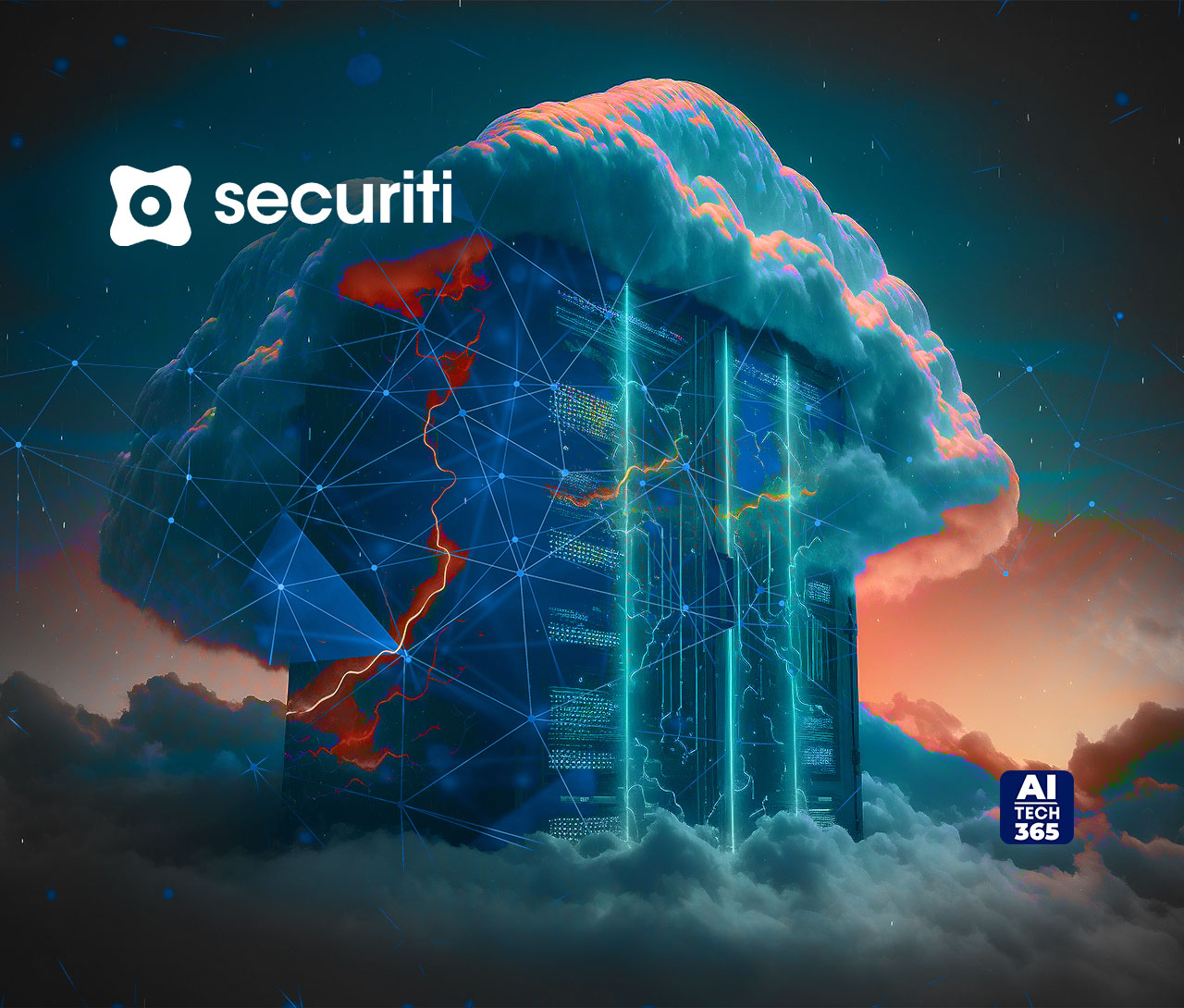
AI security, in a global context, encompasses the protection of artificial intelligence systems and their associated data from unauthorized access, use, disclosure, disruption, modification, or destruction. This definition extends beyond simple cybersecurity measures to encompass the broader societal and ethical implications of increasingly autonomous AI systems. It requires a multi-faceted approach considering the unique vulnerabilities inherent in different AI technologies, the diverse geopolitical landscapes, and the evolving nature of AI threats.The complexity of AI systems and their widespread integration into critical infrastructure pose significant challenges in establishing universally accepted standards for AI security.
These challenges include the lack of standardized terminology, the rapid pace of technological advancements outstripping regulatory frameworks, and the difficulty in balancing innovation with the need for robust security measures. Further complicating the issue is the diverse understanding of acceptable risk levels across different nations and cultures.
National Approaches to AI Security Regulations
Different nations adopt varying approaches to AI security regulations, reflecting their unique technological capabilities, economic priorities, and societal values. Some countries, like the European Union, prioritize a risk-based approach, focusing on regulating AI systems based on their potential harm. This approach often involves strict data protection regulations and rigorous ethical guidelines. Other nations, such as China, might emphasize national security and economic competitiveness, prioritizing the development and deployment of AI while focusing on internal security threats.
The United States, on the other hand, leans towards a more sector-specific approach, targeting regulation to specific industries where AI poses significant risks, such as healthcare and finance. These differing approaches highlight the difficulties in creating a truly global, harmonized regulatory framework.
A Framework for Classifying AI Security Threats
A robust framework for classifying AI security threats should consider both the likelihood and the impact of the threat. This can be visualized as a matrix, with likelihood categorized as low, medium, or high, and impact categorized similarly. For example, a low-likelihood, low-impact threat might be a minor software glitch in a simple AI application, while a high-likelihood, high-impact threat could be a sophisticated adversarial attack on a self-driving car system, potentially leading to fatalities.
This framework would allow for a prioritized approach to risk management, focusing resources on the most critical threats. Specific threat examples within each category could include data poisoning (high likelihood, medium impact), model theft (medium likelihood, high impact), and adversarial attacks (medium likelihood, high impact). The impact should be assessed across various dimensions, including economic loss, physical harm, and damage to reputation.
International Collaboration and Governance
The development and deployment of artificial intelligence (AI) presents unprecedented challenges and opportunities, demanding a coordinated global response to ensure its safe and beneficial use. Effective international collaboration and robust governance frameworks are crucial to mitigate potential risks and harness AI’s transformative power. This necessitates a multifaceted approach, involving the sharing of best practices, the establishment of common standards, and the development of mechanisms for enforcement and accountability.International collaboration on technology safety and security isn’t a new concept.
Many successful examples exist, demonstrating the feasibility of joint efforts on complex global issues. These precedents provide valuable lessons for navigating the unique challenges posed by AI.
Successful International Collaborations on Technology Safety and Security, Ensuring a secure future global guidelines for ai security
Several international collaborations highlight the potential for effective global action on technology safety. The International Atomic Energy Agency (IAEA), for example, plays a crucial role in regulating nuclear technology, promoting safety standards, and preventing nuclear proliferation. Similarly, the International Civil Aviation Organization (ICAO) sets global standards for aviation safety, fostering collaboration among nations to improve air travel security.
These organizations demonstrate the efficacy of international cooperation in addressing complex technical challenges with significant global implications. Their success lies in establishing clear guidelines, fostering transparency, and providing mechanisms for monitoring and enforcement. The challenges faced by these organizations in achieving universal adherence to standards offer valuable insights into potential obstacles for global AI governance.
Mechanisms for Establishing Global AI Security Guidelines
Establishing global AI security guidelines requires a multi-pronged approach. One potential mechanism is the development of international treaties, legally binding agreements between nations outlining shared principles and obligations regarding AI development and deployment. However, negotiating and ratifying such treaties can be a lengthy and complex process, often hindered by differing national interests and priorities. Alternatively, international organizations, such as the United Nations or specialized agencies like UNESCO, could play a crucial role in fostering collaboration, developing common standards, and facilitating the exchange of information.
These organizations can leverage their existing structures and expertise to promote dialogue, build consensus, and establish effective monitoring mechanisms. A hybrid approach, combining elements of both treaties and international organizations, might prove most effective in achieving comprehensive global AI governance.
Key Stakeholders in Global AI Governance
Global AI governance involves a diverse range of stakeholders, each with distinct roles and responsibilities. National governments play a central role in setting national AI policies, regulating AI development within their borders, and participating in international collaborations. International organizations, as mentioned above, provide platforms for dialogue, standard-setting, and monitoring. The private sector, comprising AI developers, researchers, and companies, is responsible for developing and deploying AI systems responsibly and ethically.
Civil society organizations, including NGOs and advocacy groups, play a crucial role in raising awareness, advocating for ethical AI development, and holding stakeholders accountable. Finally, academia contributes through research, education, and the development of technical expertise to inform policy decisions and guide the responsible development of AI.
Hypothetical Scenario Illustrating Challenges of Enforcing Global AI Security Regulations
Imagine a scenario where a sophisticated AI system, developed in one country, is used for malicious purposes in another. The country of origin might have robust AI security regulations in place, but these regulations may not extend to the country where the system is misused. Enforcement becomes challenging due to jurisdictional issues, differences in legal frameworks, and the difficulty of tracking and attributing malicious AI activity across borders.
This scenario highlights the need for international cooperation, mutual legal assistance treaties, and effective mechanisms for cross-border investigation and prosecution to ensure the effective enforcement of global AI security regulations. Such a situation would necessitate a collaborative effort between nations to trace the origin of the AI, identify those responsible, and take appropriate action. The difficulty in obtaining evidence across borders, and the lack of universal legal definitions for AI-related crimes, pose significant obstacles.
Technical Safeguards and Best Practices: Ensuring A Secure Future Global Guidelines For Ai Security
Building secure AI systems requires a multifaceted approach, encompassing robust development practices, rigorous testing, and proactive vulnerability management. Ignoring these aspects can lead to significant security risks, from data breaches and system malfunctions to biased outputs and malicious exploitation. This section delves into specific technical safeguards and best practices to mitigate these risks.
Secure AI Development Lifecycle Management
A secure AI system begins with a secure development lifecycle. This involves integrating security considerations into every phase, from initial design and data acquisition to deployment and ongoing monitoring. A key principle is “security by design,” embedding security not as an afterthought, but as an integral part of the system’s architecture and functionality. This proactive approach significantly reduces vulnerabilities and simplifies the mitigation process.
Techniques for Detecting and Mitigating AI Security Vulnerabilities
Various techniques exist for identifying and addressing AI security weaknesses. These include rigorous code reviews to identify vulnerabilities in the algorithms and underlying code; adversarial testing, simulating malicious attacks to expose vulnerabilities; and model explainability techniques, which enhance transparency and facilitate the detection of biases or unintended behaviors. Regular security audits and penetration testing further bolster the system’s resilience.
Furthermore, employing robust data validation and sanitization methods helps prevent malicious inputs from compromising the AI system.
Ensuring a secure future requires robust global guidelines for AI security, a task made even more critical by the expanding cloud landscape. Understanding how to manage this effectively is key, and that’s where understanding tools like Bitglass comes in; check out this insightful article on bitglass and the rise of cloud security posture management to see how it fits into the larger picture.
Ultimately, strong cloud security is a foundational element of any comprehensive AI security strategy.
Robust Security Architectures for AI Systems
A robust security architecture for AI systems encompasses several key components. Data protection involves employing encryption techniques (both in transit and at rest) and access control mechanisms to limit access to sensitive data based on the principle of least privilege. Access control ensures that only authorized personnel can access and modify the AI system and its data. Anomaly detection systems continuously monitor the system’s behavior, flagging unusual activities that could indicate malicious attacks or system malfunctions.
These systems can utilize machine learning algorithms themselves to identify deviations from normal operational patterns. Regular security updates and patching are crucial to address newly discovered vulnerabilities.
AI System Security Checklist for Developers
The following checklist provides a structured approach to ensure AI system security throughout the development process. This checklist should be adapted and expanded based on the specific needs and risks of the AI system.
Phase | Security Measure | Implementation Details | Verification Method |
---|---|---|---|
Design | Data Minimization | Collect only necessary data; anonymize or pseudonymize where possible. | Data flow diagrams and privacy impact assessments. |
Development | Secure Coding Practices | Follow secure coding guidelines; use static and dynamic code analysis tools. | Code reviews and penetration testing. |
Testing | Adversarial Testing | Simulate attacks to identify vulnerabilities; use fuzzing techniques. | Vulnerability reports and remediation efforts. |
Deployment | Access Control | Implement role-based access control (RBAC) and multi-factor authentication (MFA). | Security audits and regular access reviews. |
Monitoring | Anomaly Detection | Implement systems to detect unusual activity and potential threats. | Continuous monitoring and alert systems. |
Maintenance | Regular Updates | Apply security patches and updates promptly. | Version control and patch management systems. |
Ethical Considerations and Societal Impact
The global deployment of AI systems presents a complex tapestry of ethical challenges and societal impacts. While offering immense potential benefits, the unchecked expansion of AI necessitates a careful consideration of potential biases, unintended consequences, and the broader implications for human well-being and social structures. Failure to proactively address these issues risks exacerbating existing inequalities and creating new forms of societal disruption.The ethical implications of AI are multifaceted and deeply intertwined with its technical development and deployment.
Transparency and accountability are not merely desirable attributes; they are fundamental requirements for building trust and ensuring responsible innovation. Without mechanisms to understand how AI systems arrive at their decisions, and to hold developers and deployers accountable for their outcomes, we risk creating systems that perpetuate harm and erode public confidence.
Bias and Discrimination in AI Systems
AI systems are trained on data, and if that data reflects existing societal biases—such as gender, racial, or socioeconomic disparities—the AI will inevitably perpetuate and even amplify those biases. This can lead to discriminatory outcomes in areas like loan applications, hiring processes, and even criminal justice, disproportionately impacting marginalized communities. For example, facial recognition technology has been shown to exhibit higher error rates for individuals with darker skin tones, highlighting the urgent need for rigorous testing and mitigation strategies to address these biases before deployment.
The development of fairness-aware algorithms and diverse, representative datasets are crucial steps towards mitigating this risk.
Transparency and Accountability in AI Development
Transparency in AI involves making the inner workings of the system understandable, to the extent possible. This includes providing explanations for AI decisions, documenting the data used for training, and clearly outlining the system’s limitations. Accountability, on the other hand, focuses on establishing clear lines of responsibility for the actions and outcomes of AI systems. This requires mechanisms for identifying and addressing errors, as well as holding developers and deployers accountable for harmful outcomes.
Without transparency and accountability, it is impossible to effectively monitor and regulate the use of AI, increasing the risk of unforeseen negative consequences.
Societal Risks of Insecure AI Systems
Insecure AI systems pose a significant threat to society. Job displacement due to automation is a major concern, particularly for roles that are easily automatable. This necessitates proactive measures such as retraining and upskilling initiatives to help workers adapt to the changing job market. Furthermore, insecure AI systems can be vulnerable to manipulation, potentially leading to the spread of misinformation, social unrest, and even political instability.
The potential for deepfakes—realistic but fabricated videos or audio recordings—to manipulate public opinion and undermine trust in institutions is a particularly pressing concern.
Ethical Dilemma: Autonomous Vehicle Decision-Making
Consider a scenario involving an autonomous vehicle facing an unavoidable accident. The car must choose between two equally undesirable outcomes: hitting a pedestrian or swerving and potentially injuring its passengers. This presents a complex ethical dilemma, as different ethical frameworks may prioritize different values (e.g., minimizing harm to the greatest number of people versus prioritizing the safety of the vehicle’s occupants).
One approach might involve programming the vehicle to prioritize the safety of its occupants, based on a utilitarian calculation minimizing overall harm. Another approach might focus on a deontological perspective, emphasizing adherence to pre-defined rules and regulations regardless of the outcome. Ultimately, the resolution requires careful consideration of ethical principles, societal values, and the potential legal ramifications. A robust public discourse involving ethicists, policymakers, and technologists is crucial in establishing clear guidelines for such situations.
Future Trends and Emerging Threats
The rapid advancement of artificial intelligence (AI) brings immense benefits, but also introduces new and evolving security risks. Understanding these emerging threats is crucial for developing robust and adaptable global AI security guidelines. The interconnected nature of AI systems and their increasing integration into critical infrastructure means that vulnerabilities in one area can have cascading effects globally.The landscape of AI security is constantly shifting, demanding a proactive and dynamic approach to mitigation.
This section explores some of the most pressing future trends and emerging threats to AI security, highlighting the need for continuous adaptation and improvement of global guidelines.
Adversarial Attacks and AI-Powered Cyberattacks
Adversarial attacks exploit vulnerabilities in AI algorithms by introducing carefully crafted inputs designed to deceive the system. These attacks can manifest in various forms, such as manipulating images to fool facial recognition systems or injecting malicious code into machine learning models to alter their behavior. The sophistication of these attacks is increasing rapidly, with attackers using AI itself to develop more effective and harder-to-detect methods.
AI-powered cyberattacks represent a particularly concerning development, as malicious actors leverage AI’s capabilities for automation, scale, and adaptability to launch more sophisticated and widespread attacks against individuals, organizations, and critical infrastructure. For example, an AI could be used to autonomously scan for vulnerabilities in a network, exploit them, and deploy malware far faster than a human could. The speed and scale of such attacks necessitate a robust and proactive security posture.
The Impact of Quantum Computing on AI Security
Quantum computing holds the potential to revolutionize various fields, but it also poses a significant threat to current cryptographic methods used to secure AI systems. Quantum computers, with their vastly superior computational power, could potentially break widely used encryption algorithms, rendering current data protection measures ineffective. This could compromise the confidentiality, integrity, and availability of sensitive data used in AI development and deployment.
For example, quantum computers could easily decrypt data protected by RSA encryption, a commonly used algorithm in securing communication and data storage. The development of quantum-resistant cryptography is therefore crucial for securing AI systems in the post-quantum era. This requires a shift towards developing new algorithms that are resistant to attacks from both classical and quantum computers.
Technological Advancements Enhancing AI Security
Several technological advancements show promise in enhancing AI security. These include advancements in: Explainable AI (XAI), which allows us to understand the decision-making process of AI models, making it easier to identify and mitigate biases and vulnerabilities; Differential privacy techniques, which allow for the analysis of sensitive data while protecting individual privacy; Formal verification methods, which provide mathematical guarantees about the correctness and security of AI systems; and Blockchain technology, which can be used to enhance the security and transparency of AI data management and provenance.
These technologies offer a multi-layered approach to improving AI security, addressing different aspects of the problem. For instance, XAI can help detect adversarial attacks by revealing inconsistencies in the model’s reasoning, while differential privacy protects sensitive data used for training AI models.
Continuous Adaptation of Global AI Security Guidelines
The rapid pace of AI development necessitates the continuous evolution of global AI security guidelines. These guidelines must be flexible enough to adapt to emerging threats and technological advancements. A dynamic and collaborative approach, involving researchers, policymakers, industry stakeholders, and international organizations, is crucial for developing and updating these guidelines effectively. Regular reviews and updates, informed by ongoing research and real-world incidents, are essential to ensure their continued relevance and effectiveness in mitigating the evolving risks.
This includes mechanisms for rapid response to emerging threats and the incorporation of best practices from diverse sectors and geographical regions. International cooperation is critical for establishing consistent standards and promoting responsible AI development and deployment globally.
Education and Awareness
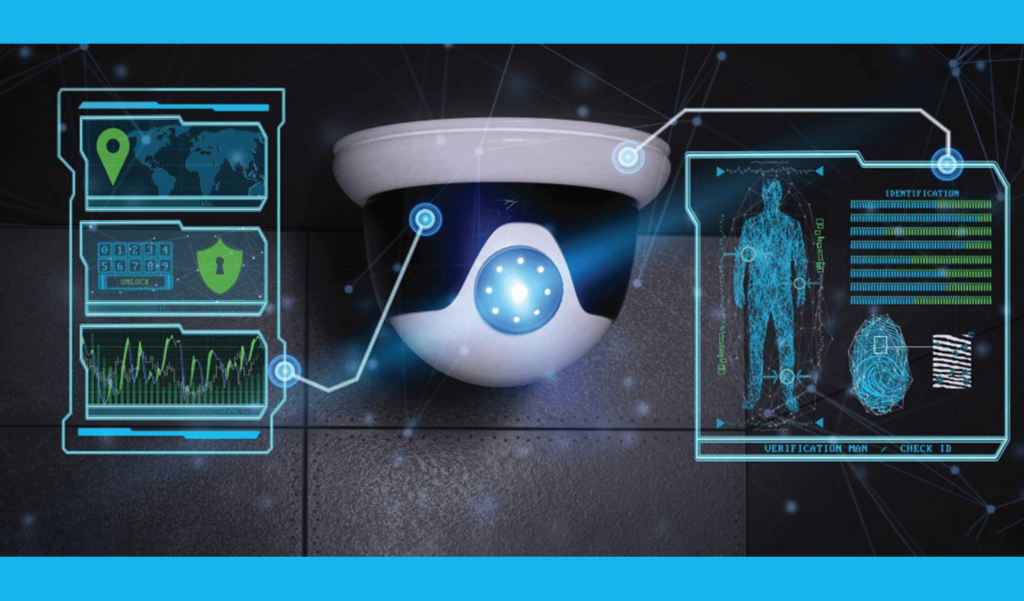
Securing the future of AI necessitates a globally coordinated effort to educate and inform both specialists and the public about the risks and responsible development of this powerful technology. A robust understanding of AI security is crucial for mitigating potential harms and fostering trust in AI systems. Without widespread education and awareness, the benefits of AI will be overshadowed by the potential for misuse and unintended consequences.AI security education and training programs are vital for creating a workforce capable of developing, deploying, and managing secure AI systems.
Crafting global AI security guidelines is crucial for a safe future, demanding robust and adaptable systems. This need for flexibility is precisely why I’ve been exploring the potential of rapid application development, like what’s discussed in this insightful article on domino app dev, the low-code and pro-code future , to help build and update security protocols quickly.
Ultimately, secure AI requires agile development practices to keep pace with evolving threats.
These programs should equip professionals with the skills and knowledge necessary to identify, assess, and mitigate AI-related risks. Public awareness campaigns, meanwhile, are essential for building a society that understands the implications of AI and can participate in shaping its future.
AI Security Training Curriculum
A comprehensive AI security training program should cover a range of topics, from foundational concepts to advanced techniques. The curriculum should be modular, allowing for customization based on the learner’s background and career goals. The learning objectives should focus on developing practical skills and critical thinking abilities.
- Module 1: Foundations of AI Security: This module introduces fundamental concepts in cybersecurity and AI, including common vulnerabilities, attack vectors, and defense mechanisms. Learning objectives include defining AI security, identifying common threats, and understanding the regulatory landscape.
- Module 2: AI System Security Assessment: This module covers methods for evaluating the security of AI systems, including vulnerability assessments, penetration testing, and risk management. Learning objectives include conducting security assessments, identifying vulnerabilities, and developing mitigation strategies.
- Module 3: Adversarial Machine Learning: This module explores the techniques used to attack and defend against adversarial machine learning attacks. Learning objectives include understanding adversarial examples, implementing defense mechanisms, and evaluating the robustness of AI models.
- Module 4: Data Security and Privacy in AI: This module focuses on the unique data security and privacy challenges posed by AI systems. Learning objectives include understanding data privacy regulations, implementing secure data handling practices, and mitigating privacy risks.
- Module 5: AI Governance and Ethics: This module examines the ethical considerations and governance frameworks relevant to AI security. Learning objectives include understanding ethical principles, applying ethical guidelines to AI development, and navigating the regulatory landscape.
Raising Public Awareness of AI Security Risks
Effective public awareness campaigns require a multi-faceted approach that utilizes various communication channels and engages diverse audiences. These campaigns should aim to educate the public about the potential risks associated with AI, while also promoting best practices for responsible AI use.The campaigns should avoid technical jargon and instead focus on clear, concise messaging that resonates with the target audience.
Real-world examples and case studies can help to illustrate the potential consequences of AI security failures. Interactive tools and resources, such as online quizzes and simulations, can enhance engagement and knowledge retention.
Lifecycle of an AI Security Awareness Campaign
A visual representation of the campaign lifecycle would be a cyclical diagram. The first stage, Planning & Research, involves defining target audiences, identifying key messages, and selecting appropriate communication channels. This is followed by Development & Production, where materials such as brochures, videos, and social media posts are created. The Implementation & Distribution phase focuses on disseminating materials through chosen channels.
Monitoring & Evaluation involves tracking campaign reach, engagement, and impact using metrics such as website visits, social media interactions, and surveys. Finally, Refinement & Iteration uses feedback from the evaluation phase to improve campaign messaging and materials for future iterations. The cycle then repeats, continuously improving the campaign’s effectiveness.
End of Discussion

Securing the future of AI requires a global, collaborative effort. It’s not just about technical solutions; it’s about forging international agreements, fostering ethical development, and educating the public about the risks and rewards. The path ahead is challenging, requiring ongoing dialogue and adaptation. But by working together, prioritizing ethical considerations, and continuously refining our approach, we can harness the power of AI for good while mitigating its inherent risks, ensuring a future where this powerful technology serves humanity.
FAQ
What are some examples of AI security vulnerabilities?
AI systems can be vulnerable to adversarial attacks (manipulating input data to produce incorrect outputs), data poisoning (corrupting training data), and model extraction (illegally copying AI models). Weaknesses in data security and access control are also significant risks.
How can we ensure AI systems are transparent and accountable?
Transparency involves making the decision-making processes of AI systems understandable. Accountability involves establishing clear lines of responsibility for the actions of AI systems, including mechanisms for redress when things go wrong. This requires technical solutions, clear regulations, and ethical frameworks.
What role does education play in AI security?
Education is vital. We need to train skilled professionals in AI security and raise public awareness of the potential risks and benefits. This includes educating developers, policymakers, and the general public about responsible AI development and usage.