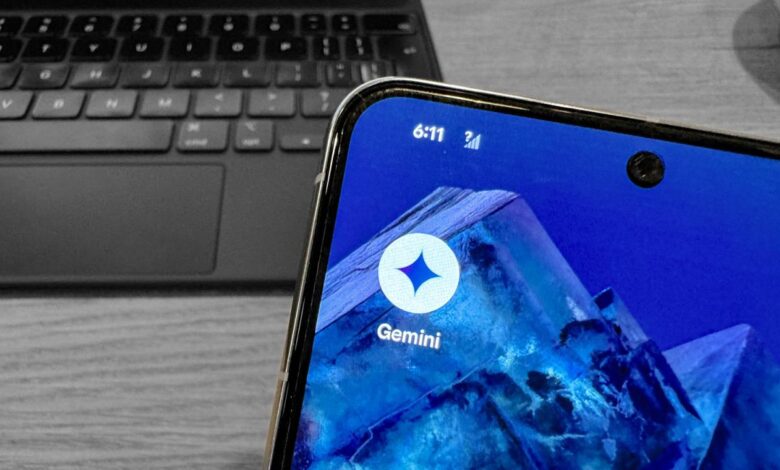
Googles Gemini AI Data Privacy Invasion?
Google to do data privacy invasion in the name of gemini ai development – Google’s Gemini AI: Data Privacy Invasion? The question hangs heavy in the air. Google’s ambitious leap into the world of advanced AI, specifically with Gemini, raises serious concerns about the extent of data collection and its potential impact on our privacy. This isn’t just about Google’s stated policies; it’s about the practical implications of feeding a massive AI with our personal data.
We’ll delve into the specifics of Google’s data collection practices, the transparency (or lack thereof) surrounding these practices, and the potential legal and ethical quagmires involved. Are we willingly handing over our digital lives for the sake of technological advancement, or is something more sinister at play?
We’ll examine the types of data Google collects, how it uses that data, and whether the current consent mechanisms are adequate. We’ll also explore the security measures in place to protect this sensitive information and discuss the potential risks associated with both data breaches and the inherent biases that can be baked into AI models trained on biased data.
Ultimately, we’ll try to answer the crucial question: is Google’s pursuit of Gemini AI worth the price of our privacy?
Google’s Data Collection Practices for Gemini AI
Google’s Gemini AI, like other large language models (LLMs), relies heavily on vast amounts of data for training and improvement. Understanding Google’s data collection methods is crucial for evaluating the privacy implications of this powerful technology. This exploration delves into the types of data collected, the stated justifications, comparisons with competitors, and a potential alternative approach.Google’s data collection for Gemini AI is multifaceted, drawing from a variety of sources.
Publicly available data, such as books, articles, and code from open-source repositories, forms a significant portion of the training dataset. However, Google also leverages data from its own services. This includes text and code from Google Search, Google Docs, and other Google products used by millions of users worldwide. The exact nature and extent of this internal data usage are not fully transparent, leading to ongoing privacy concerns.
Furthermore, user interactions with Gemini itself, including prompts, queries, and feedback, contribute to its ongoing development and refinement. This feedback loop, while beneficial for improving the model’s performance, raises questions about the long-term storage and potential use of this personalized data.
Google’s Stated Privacy Policies Regarding Gemini AI Data, Google to do data privacy invasion in the name of gemini ai development
Google’s privacy policy addresses data collection for AI development in a general sense, emphasizing its commitment to user privacy and data security. However, the specifics regarding Gemini AI data are less clear. The policy mentions data anonymization and aggregation techniques, aiming to minimize the identification of individual users. It also Artikels data retention policies, although the exact duration of data storage for Gemini AI training remains undefined.
The lack of granular detail regarding Gemini’s data usage, coupled with the sheer volume of data involved, leaves room for interpretation and raises concerns about the potential for indirect identification and re-identification of individuals.
Comparison with Other LLM Developers
Other large language model developers, such as OpenAI and Meta, also employ extensive data collection practices. However, the specifics vary significantly. OpenAI, for instance, has been more transparent about its data sources, although the exact composition of its training datasets remains proprietary. Meta’s approach is often tied to its social media platforms, raising different privacy concerns related to the volume and sensitivity of user-generated content.
A consistent theme across these developers is the challenge of balancing the need for vast datasets for training with the ethical and legal obligations to protect user privacy. A comparative analysis across these companies requires more detailed public disclosures from each.
Hypothetical Alternative Data Collection Strategy
A more privacy-conscious approach to Gemini AI development could involve a greater emphasis on synthetic data generation. Instead of relying heavily on real-world user data, the training could incorporate larger quantities of artificially generated text and code that mimics the characteristics of real data without containing personally identifiable information. This strategy, while challenging to implement perfectly, would significantly reduce the privacy risks associated with using real user data.
Furthermore, increased transparency about data sources and usage, along with robust data anonymization and minimization techniques, could further enhance user trust and confidence. This approach would likely require a shift in development strategies and increased investment in synthetic data generation technologies.
User Consent and Transparency in Data Usage
Google’s Gemini AI, like other large language models, relies heavily on user data for training and improvement. However, the mechanisms Google employs to obtain and manage user consent for this data collection are crucial for maintaining user trust and adhering to data privacy regulations. The clarity and comprehensiveness of these mechanisms are subjects of ongoing debate and scrutiny.Google primarily relies on its privacy policies and terms of service to inform users about data collection practices related to Gemini AI.
Users are generally presented with these lengthy documents during account creation or when using Google services integrated with Gemini AI. The challenge lies in the accessibility and understandability of this information for the average user. Many users may not fully comprehend the extent of data collection, particularly the nuances of how their data contributes to the training of Gemini AI.
Google’s Consent Mechanisms
Google’s consent mechanisms primarily involve incorporating data collection practices within its broader privacy policies. Users implicitly consent to data collection by continuing to use Google services. While Google does provide information on data usage, it’s often buried within extensive legal documents, making it difficult for users to easily grasp the specific implications for Gemini AI. This approach lacks the explicit and granular consent that many argue is necessary for such sensitive data processing.
For example, a user might consent to personalized advertising, but that same data might also be used for training Gemini AI without specific, separate consent.
Google’s Gemini AI development, fueled by data from Google ToDo, raises serious privacy concerns. It makes you wonder about the ethical implications of such vast data collection, especially when considering alternative development approaches. For instance, the article on domino app dev the low code and pro code future highlights how focusing on user privacy and control might influence app development choices.
Ultimately, the question remains: is the potential of Gemini worth the cost of our privacy?
Improving Transparency in Data Usage Communication
Google could significantly improve transparency by implementing clearer, more concise, and user-friendly explanations of its data usage practices for Gemini AI. This could involve: (1) Providing separate, easily accessible consent forms specifically addressing Gemini AI data usage, outlining the types of data collected and their purpose; (2) Employing visual aids, such as infographics, to illustrate data flows and processing; (3) Offering interactive tutorials or FAQs that address common user concerns; and (4) providing more granular control over what data is used for training purposes.
For instance, instead of a blanket consent for “improving services,” users could opt in or out of specific data usage categories relevant to Gemini AI.
Legal and Ethical Implications of Current Data Consent Practices
Google’s current data consent practices face potential legal and ethical challenges. Data protection regulations like the GDPR in Europe and CCPA in California require explicit and informed consent for data processing. The implicit consent model employed by Google may fall short of these requirements, particularly considering the sensitive nature of the data used to train AI models. Ethically, concerns arise regarding the potential for bias in the training data and the lack of user control over how their data is used.
This could lead to unforeseen consequences and potential harm to individuals whose data is incorporated without their full knowledge or explicit consent. Furthermore, the opacity of the process makes it difficult to assess the fairness and accountability of the AI system’s outputs.
Types of Data Collected and Consent Levels
Data Type | Purpose in Gemini AI Development | Consent Level | Example |
---|---|---|---|
Search Queries | Improving language understanding and response generation | Implicit (through Google Search Terms of Service) | A user searching for “best Italian restaurants near me” |
Gmail Emails (with user consent for data analysis) | Enhancing language model’s understanding of context and communication styles | Explicit (through Gmail settings) | Emails containing conversations, work documents, etc. |
YouTube Viewing History | Improving the model’s knowledge base and understanding of diverse topics | Implicit (through YouTube Terms of Service) | Videos watched on various subjects, including educational content |
Google Docs Content (with user consent for data analysis) | Improving writing assistance and text generation capabilities | Explicit (through Google Docs settings) | Documents created and edited by users, including formal and informal writing styles |
Data Security and Protection Measures: Google To Do Data Privacy Invasion In The Name Of Gemini Ai Development
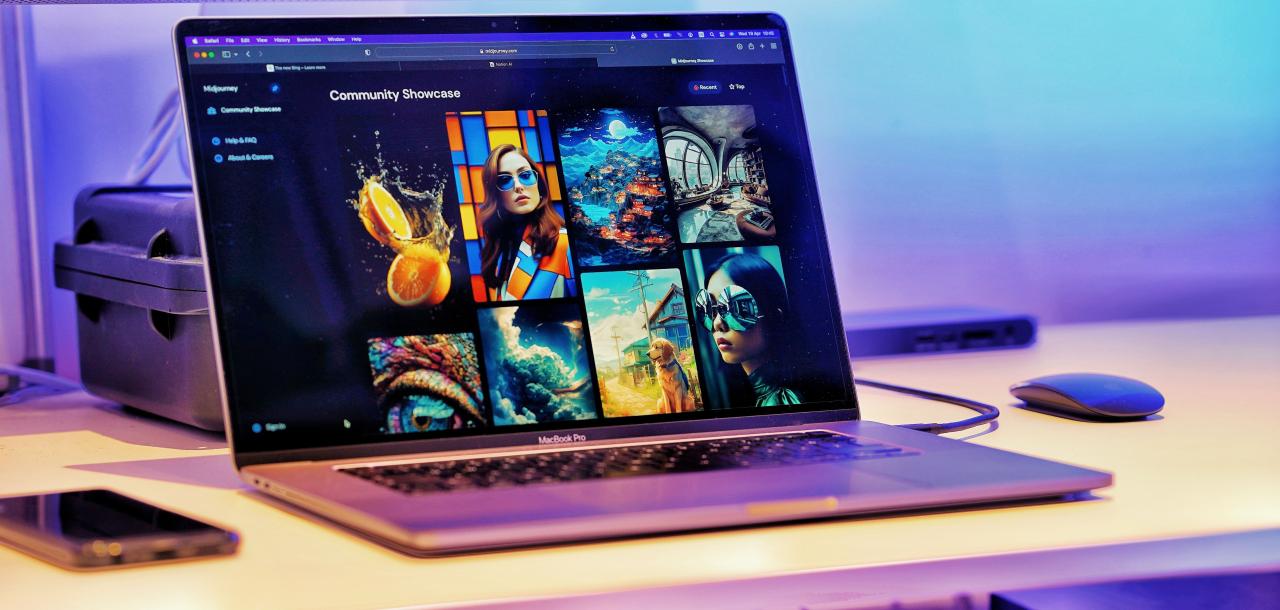
Google’s Gemini AI development relies heavily on user data, raising crucial questions about the security measures in place to protect this information. While Google touts robust security, a thorough examination of their practices, potential vulnerabilities, and comparisons to best practices is necessary for a complete understanding of the risks involved.Google employs a multi-layered approach to data security, encompassing physical security of data centers, robust network security protocols, and data encryption both in transit and at rest.
They utilize advanced threat detection systems, regularly conduct security audits, and invest heavily in personnel dedicated to cybersecurity. However, the sheer scale of data collected for Gemini AI presents unique challenges.
Data Encryption and Access Control
Google uses encryption to protect data at rest and in transit. Data at rest, meaning data stored on servers, is encrypted using strong encryption algorithms. Data in transit, meaning data moving between servers or devices, is also encrypted using secure protocols like HTTPS. Access control mechanisms, including role-based access control (RBAC), limit access to sensitive data to authorized personnel only.
This system aims to prevent unauthorized access and data breaches. However, the complexity of this system also means there’s potential for human error or vulnerabilities in the implementation to compromise the effectiveness of these measures.
Vulnerabilities in Google’s Data Security Infrastructure
Despite Google’s substantial investments, vulnerabilities remain a possibility. Sophisticated cyberattacks, such as zero-day exploits targeting software vulnerabilities, could potentially bypass security measures. Internal threats, such as malicious insiders, also pose a risk. Moreover, the sheer volume of data processed for Gemini AI increases the attack surface, making it a more attractive target for malicious actors. A successful breach could expose sensitive user information, leading to identity theft, financial losses, or reputational damage for both users and Google.
The scale of a potential breach involving Gemini AI training data would be significantly larger than most other data breaches. For example, a breach affecting millions of user search queries, voice commands, or other personal data used in Gemini AI training would have widespread consequences.
Risks Associated with Large-Scale Data Storage and Processing
Storing and processing massive datasets for AI training introduces inherent risks. The larger the dataset, the greater the potential impact of a data breach. Furthermore, the complexity of AI training processes introduces new vulnerabilities. For instance, adversarial attacks, where malicious actors manipulate input data to cause the AI to produce incorrect or harmful outputs, are a growing concern.
Data leakage during the training process, potentially revealing sensitive information about individual users, is another significant risk. The potential for bias amplification within the training data, leading to discriminatory outcomes from the AI, is also a major concern. Consider the hypothetical scenario of a bias in the training data leading to the AI unfairly disadvantaging a specific demographic group in a loan application process – the consequences could be severe.
Best Practices for Data Security in AI Development and Comparison to Google’s Practices
Best practices for data security in AI development include employing differential privacy techniques to protect individual user data while still enabling useful AI training, utilizing federated learning to train AI models on decentralized data sources without directly accessing the data, and implementing robust data anonymization and de-identification techniques. Regular security audits and penetration testing are crucial, as is maintaining transparent and accountable data governance practices.
While Google employs many of these best practices, the scale of its operation necessitates continuous improvement and transparency regarding its security measures to maintain user trust. For example, the level of detail regarding the specific encryption algorithms used and the frequency of security audits could be enhanced to provide greater transparency and assurance.
The Impact of Gemini AI on User Privacy
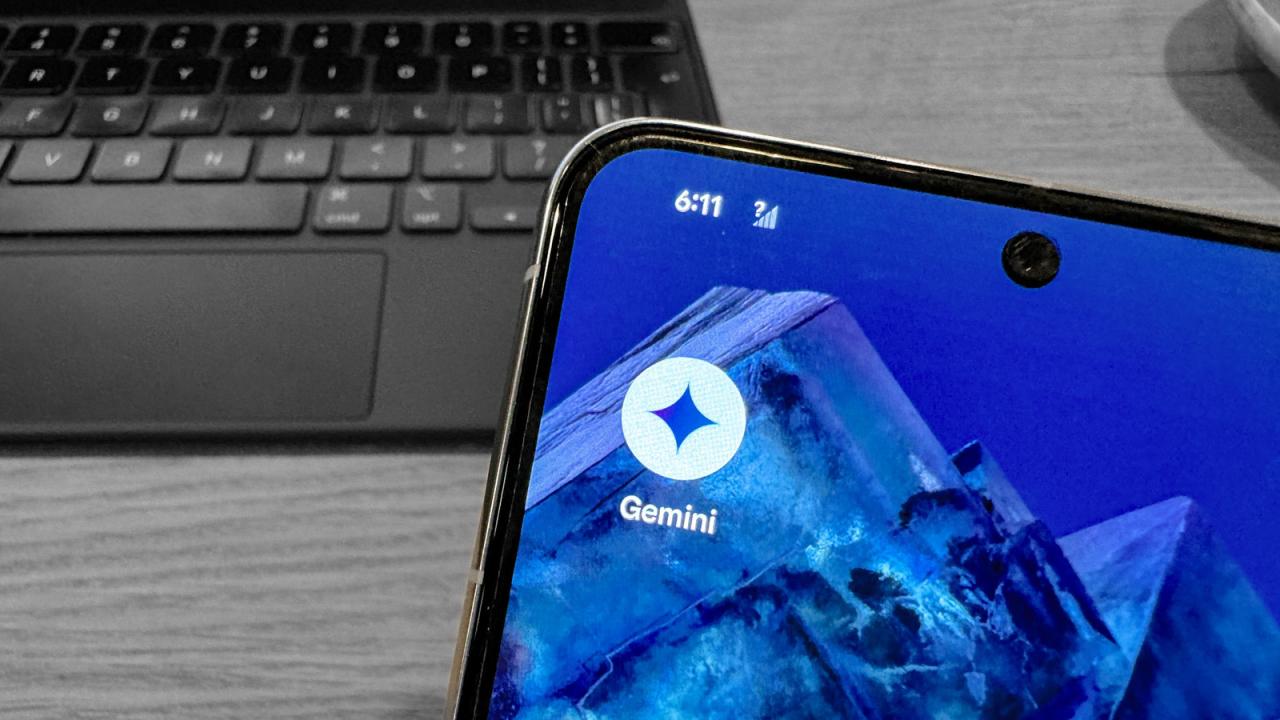
Gemini AI, with its impressive capabilities, raises significant concerns regarding user privacy. The sheer volume of data used to train and operate this powerful AI model, coupled with its advanced analytical abilities, creates a potential for privacy violations that extend beyond the typical risks associated with data collection. Understanding these risks is crucial for navigating the ethical and practical implications of this technology.The use of user data in Gemini AI’s training process inherently presents a privacy challenge.
The model learns patterns and relationships from vast datasets, which often include personally identifiable information (PII) or data that can be used to indirectly identify individuals. Even if PII is anonymized or pseudonymized, sophisticated techniques could potentially re-identify individuals based on unique combinations of seemingly innocuous data points. This process, while improving the AI’s performance, simultaneously increases the risk of exposing sensitive information.
Examples of Gemini AI’s Potential for Privacy Infringement
Gemini AI’s advanced capabilities, such as natural language processing and image recognition, can be exploited to infringe on user privacy in various ways. For instance, a malicious actor could feed the AI system with specific prompts designed to extract sensitive information from user-generated content, such as medical records, financial details, or private communications. Similarly, the AI’s ability to analyze images could lead to the unintended disclosure of location data or identification of individuals in photos that were meant to remain private.
Consider a scenario where an AI is trained on images of a specific individual’s house, and then is used to identify that house in publicly available street view imagery, potentially revealing the location to unwanted individuals. Another example is the potential for generating realistic deepfakes using the model, which could be used for identity theft or reputational damage.
Inferences from User Data Revealing Sensitive Information
The inferences made by Gemini AI based on seemingly innocuous user data can reveal sensitive information about individuals. For example, analyzing a user’s search history, location data, and online purchases could reveal their political affiliations, religious beliefs, health conditions, or sexual orientation—all without explicit consent. Even seemingly benign data points, when combined and analyzed by the AI, can create a detailed profile of an individual that surpasses what would be possible through manual analysis.
This raises concerns about the potential for discrimination, profiling, and surveillance.
Potential Privacy Risks Associated with Gemini AI Deployment
The deployment of Gemini AI presents a multitude of potential privacy risks. A comprehensive understanding of these risks is vital for responsible development and implementation.
- Data breaches: The large datasets used to train Gemini AI are vulnerable to breaches, potentially exposing sensitive user information.
- Unintended data leakage: The AI’s ability to infer sensitive information from seemingly innocuous data poses a significant risk of unintended data leakage.
- Malicious use: The AI’s capabilities could be exploited by malicious actors to target individuals for phishing, surveillance, or other harmful activities.
- Bias and discrimination: Biases present in the training data could be amplified by the AI, leading to discriminatory outcomes.
- Lack of transparency: The lack of transparency in how Gemini AI processes and uses user data makes it difficult to assess and mitigate privacy risks.
- Surveillance and profiling: The AI’s ability to analyze user data could facilitate mass surveillance and detailed profiling of individuals.
Regulatory and Legal Considerations
Google’s development and deployment of Gemini AI, a powerful large language model, raises significant concerns regarding data privacy and its compliance with existing regulations. The sheer volume of data collected and processed necessitates a thorough examination of the legal landscape and potential repercussions for non-compliance. This analysis will focus on key regulations and the potential implications for Google’s practices.Google’s data collection practices for Gemini AI are subject to a complex web of international and national laws.
Key regulations include the General Data Protection Regulation (GDPR) in the European Union, the California Consumer Privacy Act (CCPA) in California, and numerous other similar laws globally. These regulations establish strict requirements concerning data collection, processing, storage, and user consent. The potential for non-compliance carries significant financial and reputational risks for Google.
GDPR Compliance
The GDPR mandates that personal data be processed lawfully, fairly, and transparently. It requires explicit consent for processing, data minimization, and the right to be forgotten. Google must demonstrate that its data collection for Gemini AI adheres to these principles. Failure to do so could result in hefty fines, potentially reaching up to €20 million or 4% of annual global turnover, whichever is higher.
For example, a failure to adequately inform users about the extent of data collection for training Gemini AI, or a lack of readily available mechanisms for data deletion, would be clear violations. Google must provide clear and concise information about its data practices in easily accessible privacy policies, and ensure these policies are compliant with GDPR’s stipulations.
CCPA Compliance
The CCPA grants California residents specific rights regarding their personal data, including the right to know what data is collected, the right to delete data, and the right to opt-out of data sales. Google’s data practices must be compliant with these rights. Non-compliance could lead to significant fines and legal challenges from California’s Attorney General. For instance, if Google fails to provide a clear and accessible mechanism for California residents to exercise their right to delete their data used in Gemini AI training, it could face legal action and penalties.
This necessitates a robust system for identifying and responding to data subject requests.
Potential Penalties and Legal Repercussions
Non-compliance with data privacy regulations can result in a range of penalties, including substantial fines, legal injunctions requiring changes to data practices, and reputational damage. Class-action lawsuits from affected users are also a significant risk. The reputational damage from a data privacy scandal could be devastating, impacting user trust and potentially affecting Google’s market share. The cumulative cost of fines, legal fees, and reputational harm could be substantial.
Consider the example of Facebook’s Cambridge Analytica scandal; the reputational damage and resulting fines significantly impacted the company’s financial performance and public perception.
A Hypothetical Legal Framework for Enhanced AI Privacy Protection
A more robust legal framework for AI development should emphasize proactive privacy-by-design principles. This would require AI developers to incorporate data privacy considerations from the initial stages of development, rather than treating it as an afterthought. The framework should include stricter requirements for data minimization, enhanced transparency regarding data usage, and stronger enforcement mechanisms. Furthermore, independent audits of AI systems’ data handling practices should be mandatory to ensure compliance.
This proactive approach, coupled with stronger penalties for non-compliance, would create a more effective deterrent against privacy violations in the rapidly evolving field of AI. This could involve the creation of a specialized regulatory body with the power to oversee and enforce AI data privacy standards globally or at a national level.
Last Recap
The development of Gemini AI, and similar large language models, presents a fascinating paradox. We crave the convenience and capabilities these technologies offer, yet we simultaneously grapple with the very real threat to our privacy. Google’s data collection practices, while seemingly justified by the need for training data, raise critical questions about transparency, consent, and the potential for misuse.
The discussion surrounding data privacy in the age of AI is far from over; it’s an ongoing conversation that demands our attention and vigilance. We must demand greater transparency and accountability from tech giants like Google, ensuring that the pursuit of innovation doesn’t come at the expense of our fundamental right to privacy.
Question Bank
What specific data does Google collect for Gemini AI?
Google’s data collection for Gemini likely includes search history, location data, online activity, and potentially data from other Google services you use. The exact scope remains somewhat opaque.
What happens if I don’t want Google to use my data for Gemini AI?
Currently, options to completely opt out of data usage for AI training are limited. However, you can adjust your Google privacy settings to control the extent of data Google collects.
How does Google protect my data from unauthorized access?
Google employs various security measures, including encryption and access controls, but the scale of data involved makes complete protection challenging. Data breaches remain a possibility.
What legal recourse do I have if Google violates my privacy?
Depending on your location, you may have legal recourse under data privacy laws like GDPR (in Europe) or CCPA (in California). Filing a complaint with the relevant authorities might be an option.