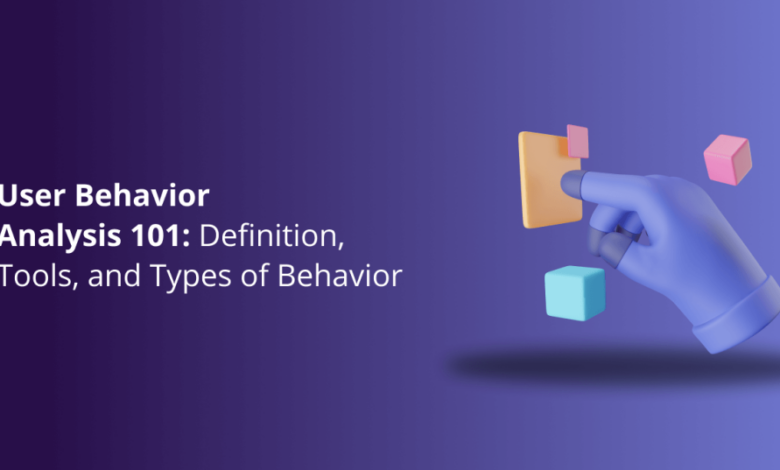
How Behavior Analytics Tools Can Help With Security
How behavior analytics tools can help with security is a game-changer in today’s digital landscape. We’re swimming in data, and sifting through it all to find genuine threats feels like searching for a needle in a digital haystack. But what if that haystack could highlight the needle itself? That’s the power of behavior analytics – it helps us understand normal user behavior, so when something deviates, we know we have a problem.
It’s proactive security, not just reactive firefighting.
Imagine instantly spotting a rogue employee accessing sensitive files outside of normal working hours, or identifying a sophisticated phishing attack before it compromises your entire system. Behavior analytics isn’t about replacing traditional security measures; it’s about adding a powerful layer of intelligence to our existing defenses, making us significantly more resilient against a constantly evolving threat landscape. This allows us to focus our resources where they matter most, preventing breaches before they happen.
Identifying Security Threats Through User Behavior
Behavior analytics tools are revolutionizing cybersecurity by shifting the focus from solely reactive threat detection to proactive threat prevention. By analyzing user behavior patterns, these tools can identify anomalies that often precede malicious activity, allowing security teams to intervene before significant damage occurs. This proactive approach is crucial in today’s sophisticated threat landscape, where traditional security measures often fall short.
These tools achieve this by establishing a baseline of “normal” user behavior. This baseline is created by observing and analyzing various user actions over a period of time. Once established, the system then continuously monitors user activity, comparing it against this baseline. Any significant deviation from this established norm triggers an alert, signaling potential malicious activity. The more data the system collects, the more accurate and refined this baseline becomes, improving the detection accuracy of anomalies.
Anomalous User Activity Indicative of Malicious Intent
Behavior analytics tools can detect a wide range of anomalous user activities that signal potential threats. These anomalies often go unnoticed by traditional security systems, which primarily focus on known threats and signatures. The power of behavior analytics lies in its ability to identify the “unknown unknowns” – the unexpected actions that deviate from established patterns and might indicate a compromise.
Types of Detectable Anomalous Behavior
Several categories of anomalous behavior are routinely flagged by behavior analytics tools. Understanding these categories is key to effectively interpreting alerts and responding to potential threats.
Method | Description | Advantages | Disadvantages |
---|---|---|---|
Statistical Anomaly Detection | Uses statistical methods like standard deviation or z-scores to identify data points that fall outside a predefined range of normal behavior. | Relatively simple to implement and computationally efficient. | Can be sensitive to outliers and may generate false positives if the normal behavior distribution is not well-defined. |
Machine Learning-based Anomaly Detection | Employs machine learning algorithms (e.g., clustering, neural networks) to learn patterns in user behavior and identify deviations from these learned patterns. | Can adapt to changing behavior patterns and detect more complex anomalies. Offers higher accuracy than statistical methods. | Requires significant amounts of training data and can be computationally expensive. Requires expertise to implement and tune. |
Rule-based Anomaly Detection | Defines specific rules based on known malicious behaviors (e.g., access to sensitive data outside of business hours). Alerts are triggered when these rules are violated. | Easy to understand and interpret. Allows for fine-grained control over what constitutes an anomaly. | Requires manual rule creation and maintenance, which can be time-consuming and prone to errors. Difficult to adapt to new types of threats. |
Hybrid Approach | Combines multiple anomaly detection methods (e.g., statistical and machine learning) to leverage the strengths of each approach and mitigate their weaknesses. | Improved accuracy and reduced false positives compared to using a single method. More robust and adaptable to changing threat landscapes. | More complex to implement and manage. Requires expertise in multiple anomaly detection techniques. |
For example, a user suddenly accessing files they’ve never accessed before, or accessing a large number of files in a short period, could indicate data exfiltration attempts. Similarly, a user logging in from an unusual geographic location or using an unfamiliar device could suggest account compromise. Attempts to modify system configurations outside of standard procedures, or unusual database queries, are also strong indicators of potential malicious activity.
Finally, a sudden increase in failed login attempts from a single IP address can be a clear sign of brute-force attacks.
Improving Incident Response with Behavior Analytics
Behavior analytics tools are revolutionizing incident response, moving us from reactive firefighting to proactive threat mitigation. By establishing a baseline of normal user activity, these tools can quickly identify deviations indicating malicious activity, significantly reducing the time it takes to detect and respond to security incidents. This proactive approach minimizes the impact of breaches, limiting data loss and reputational damage.The speed and accuracy offered by behavior analytics drastically reduce Mean Time To Detect (MTTD) and Mean Time To Respond (MTTR), key metrics in cybersecurity.
Instead of relying solely on alerts triggered by specific events, behavior analytics provides a holistic view of user activity, allowing security teams to spot anomalies that might otherwise go unnoticed. This shift towards proactive threat hunting and rapid response is crucial in today’s ever-evolving threat landscape.
Accelerated Incident Response Times
Behavior analytics accelerates incident response by providing immediate visibility into suspicious activities. For example, if an employee suddenly starts accessing sensitive files outside of normal working hours or from unusual locations, a behavior analytics system will flag this as an anomaly. This immediate alert allows security teams to investigate promptly, potentially preventing a data breach before significant damage occurs.
Consider a scenario where an employee’s account is compromised. Traditional security tools might only detect the compromise after malicious activity has already begun. Behavior analytics, however, can identify unusual login attempts or data exfiltration patternsbefore* the attacker gains full access, enabling immediate intervention and account lockdown. The difference between detecting a compromise after several hours of malicious activity versus within minutes is substantial, significantly reducing the potential impact of the attack.
Minimizing the Impact of Security Breaches
By detecting anomalies early, behavior analytics plays a crucial role in minimizing the impact of security breaches. Early detection allows for faster containment, reducing the amount of data that can be compromised and limiting the potential for lateral movement within the network. For example, if a phishing attack successfully compromises a user account, behavior analytics can identify the subsequent unusual activities, such as access to sensitive databases or unusual file transfers.
This allows security teams to quickly isolate the compromised account, preventing further damage and limiting the attacker’s access. The speed of response directly correlates with the extent of damage – the faster the response, the less opportunity for the attacker to exploit vulnerabilities.
Incident Response Procedure Using Behavior Analytics, How behavior analytics tools can help with security
A security team can effectively utilize behavior analytics data during an incident response using a structured approach:
- Initial Detection: The behavior analytics system flags an anomaly, such as unusual login attempts from a specific user account or an unusual pattern of file access.
- Investigation: The security team reviews the detailed behavior analytics data associated with the flagged anomaly. This includes timestamps, user location, accessed resources, and other relevant information.
- Verification: The team verifies the anomaly by cross-referencing the behavior analytics data with other security logs and information sources. This helps confirm the legitimacy of the anomaly and assess the potential threat level.
- Containment: Based on the investigation, the team takes appropriate containment actions, such as isolating the affected user account, blocking access to specific resources, or quarantining affected systems.
- Eradication: Once the threat is contained, the team proceeds with eradicating the threat. This might involve removing malware, patching vulnerabilities, or resetting compromised accounts.
- Recovery: The team recovers any affected systems and data, restoring them to their pre-breach state. This includes restoring backups, repairing damaged files, and ensuring the integrity of the system.
- Post-Incident Analysis: The team conducts a post-incident analysis to identify the root cause of the breach and implement preventive measures to prevent similar incidents in the future. This involves reviewing the behavior analytics data to identify any patterns or weaknesses in the security posture.
Enhancing Security Awareness Training with Behavioral Data: How Behavior Analytics Tools Can Help With Security
Security awareness training is crucial, but traditional methods often fall short. Generic training doesn’t address individual vulnerabilities, leading to ineffective learning and persistent security risks. Behavior analytics offers a powerful solution, providing insights into employee actions that can be used to personalize and optimize training programs, making them far more impactful. By analyzing user behavior data, organizations can identify specific areas where employees are most vulnerable and tailor training to directly address those weaknesses.Behavioral data offers a wealth of information to refine security awareness training.
Instead of a one-size-fits-all approach, organizations can leverage this data to create targeted training modules addressing specific risks identified within the workforce. For example, if analytics reveal a high incidence of phishing email clicks from a particular department, a specialized training module focusing on phishing techniques and detection can be developed and deployed to that specific team. This targeted approach ensures that training resources are used effectively and that employees receive the most relevant and timely information.
Identifying High-Risk Behaviors
Analyzing user behavior data allows security teams to pinpoint specific actions indicating potential vulnerabilities. For instance, repeated attempts to access unauthorized resources, unusually high login attempts from unfamiliar locations, or consistent clicking on suspicious links can all be identified and used to create targeted training modules. By understanding the patterns of risky behavior, organizations can design training that directly addresses those patterns, significantly reducing the likelihood of future security incidents.
This proactive approach helps build a more resilient security posture.
Personalizing Training Content
Behavioral data allows for the personalization of training content based on individual user profiles. Employees who consistently demonstrate risky behavior receive more focused training on the specific areas where they need improvement, while those with a strong security record can be given refresher courses or advanced training on emerging threats. This personalized approach ensures that training remains relevant and engaging for all employees, maximizing its effectiveness.
This contrasts sharply with traditional methods that often overwhelm employees with generic information, much of which is irrelevant to their individual roles and responsibilities.
Measuring Training Effectiveness
Post-training assessments alone aren’t sufficient to gauge the true effectiveness of security awareness programs. Behavior analytics provides ongoing monitoring of employee actions, allowing for a continuous evaluation of training effectiveness. If employees continue to exhibit risky behaviors after completing training, it indicates a need for further intervention, such as additional training, revised modules, or even one-on-one coaching. This continuous feedback loop allows for iterative improvements to the training program, ensuring it remains relevant and effective over time.
Prioritizing Training Topics
Instead of a generic curriculum, behavioral data enables organizations to prioritize training topics based on the most prevalent and impactful risks identified within their workforce. Resources can be allocated more effectively, focusing on areas of greatest vulnerability, rather than spreading them thinly across a range of topics. This data-driven approach ensures that training is focused on the areas that will have the greatest impact on reducing security risks.
For example, if data reveals a significant number of employees falling prey to social engineering attacks, a substantial portion of the training budget can be dedicated to modules focusing on recognizing and mitigating social engineering tactics.
Improving Training Engagement
Behavioral data can be used to identify the elements of training programs that are most effective and those that are least engaging. By analyzing user interactions with training materials, organizations can optimize the design and delivery of their training, making it more interactive, relevant, and engaging. For instance, if data reveals that employees are struggling with a particular module, the module can be redesigned or supplemented with additional resources to improve comprehension and engagement.
This iterative approach ensures that the training program remains relevant and effective for all employees.
Detecting Insider Threats Using Behavioral Analysis
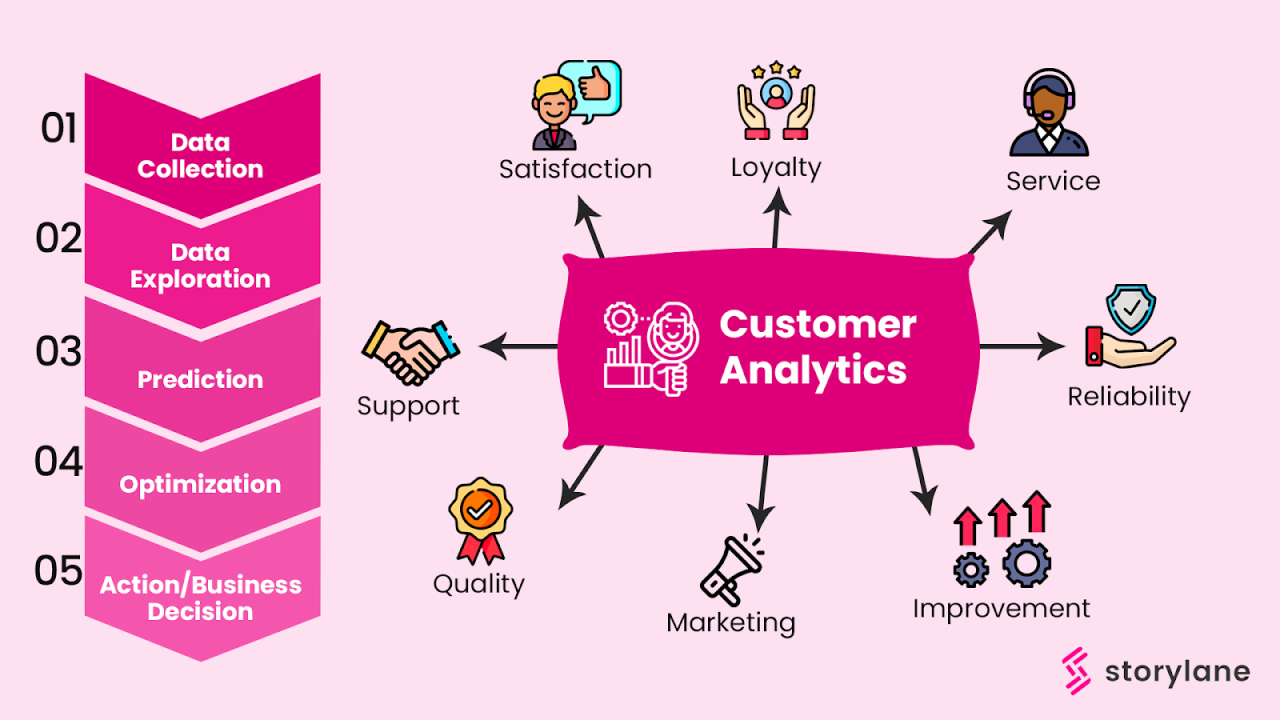
Insider threats, stemming from malicious or negligent employees, represent a significant risk to any organization. Traditional security measures often struggle to detect these threats, as they often involve authorized users leveraging their legitimate access. Behavioral analytics offers a powerful alternative, allowing organizations to identify anomalies in user behavior that may signal malicious intent or unintentional data breaches. By establishing baselines of normal activity and continuously monitoring deviations from these baselines, organizations can proactively identify and mitigate potential insider threats.
Behavioral analytics excels at detecting insider threats by focusing on the “who, what, when, where, and how” of user actions within an organization’s systems and network. It moves beyond simple rule-based systems, instead leveraging machine learning algorithms to identify subtle patterns and anomalies that might indicate malicious activity. This approach allows for the detection of threats that might otherwise go unnoticed by traditional security measures.
Key Behavioral Indicators of Potential Insider Threats
Several behavioral indicators can suggest a potential insider threat. These indicators often involve a departure from an employee’s established baseline behavior. For example, unusual access patterns, such as accessing sensitive data outside of normal working hours or from unusual locations, could be a red flag. Similarly, a sudden increase in data transfers or downloads, particularly of sensitive information, warrants investigation.
Another crucial indicator is the attempted access or modification of systems or data the employee does not typically interact with. Finally, unusual communication patterns, such as increased contact with external entities known for malicious activity, should trigger further analysis.
Techniques for Detecting Insider Threats Using Behavior Analytics
Several techniques are employed to detect insider threats using behavior analytics. These techniques often involve the combination of data collection, anomaly detection, and investigation. One common technique is user and entity behavior analytics (UEBA), which analyzes the behavior of both users and the entities they interact with, such as devices and applications. Machine learning algorithms are used to establish baselines of normal behavior and to identify deviations that may indicate malicious activity.
Another technique is to employ data loss prevention (DLP) tools integrated with behavior analytics capabilities. These tools monitor data movement and identify unusual patterns that could signal data exfiltration. Finally, security information and event management (SIEM) systems, when enhanced with behavioral analytics, can correlate various security events and identify patterns that might indicate insider threats. The choice of technique often depends on the specific needs and resources of the organization.
Visualizing User Behavior Patterns
Let’s consider a hypothetical dataset tracking employee access to sensitive financial data. The data includes timestamps, employee ID, file accessed, and location of access. We can visualize this data using a heatmap. The x-axis represents the time of day, the y-axis represents employee IDs, and the color intensity represents the frequency of access to sensitive financial data. A normal pattern would show concentrated access during regular business hours.
Behavior analytics tools are a game-changer for security, identifying unusual patterns before they escalate into full-blown breaches. Building secure apps is crucial, and that’s where understanding the evolving landscape of application development comes in, like exploring the exciting possibilities discussed in this article on domino app dev the low code and pro code future. Ultimately, proactive security measures, including robust behavior analytics, are essential for protecting these new applications and the data they manage.
However, if we observe a significantly darker color (indicating high frequency) outside of normal business hours for a specific employee, this could highlight an anomaly worthy of investigation. For example, if employee ID “1234” consistently shows high access frequency between midnight and 2 AM, it could suggest unauthorized access and warrant a deeper investigation. This visualization allows security analysts to quickly identify outliers and prioritize their investigations.
Integrating Behavior Analytics with Existing Security Systems
Behavior analytics tools offer a powerful layer of security, but their effectiveness is significantly amplified when integrated with existing security infrastructure. Seamless integration with systems like Security Information and Event Management (SIEM) platforms allows for a more holistic and proactive approach to threat detection and response. This integration leverages the strengths of both systems, creating a synergistic effect that enhances overall security posture.Integrating behavior analytics tools with SIEM systems involves connecting the two platforms to share data and insights.
This typically involves configuring APIs or using dedicated integration tools provided by the vendors. The SIEM system, which collects and analyzes security logs from various sources, provides the raw data, while the behavior analytics tool applies advanced algorithms to identify anomalies and deviations from established baselines of normal user behavior. This combined approach provides a richer context for threat detection, enabling security teams to respond more effectively.
Benefits of Integrating Behavior Analytics and SIEM
The integration of behavior analytics tools and SIEM systems delivers several key benefits, resulting in improved threat detection and response capabilities. Firstly, it enables richer context for alerts. A SIEM system might generate an alert for a suspicious login attempt, but the behavior analytics tool can provide additional context, such as whether this login attempt is consistent with the user’s typical behavior patterns.
If the login is anomalous, the behavior analytics tool can provide a risk score, alerting security teams to prioritize critical threats. Secondly, the integration facilitates proactive threat hunting. By analyzing historical user behavior data, security teams can identify subtle patterns indicative of insider threats or advanced persistent threats (APTs) that might otherwise go unnoticed. Finally, the integration streamlines incident response.
When an incident is detected, the combined data from both systems provides a comprehensive view of the attack, enabling security teams to respond faster and more effectively. For example, a compromised account identified by the SIEM can be further investigated by the behavior analytics tool to understand the extent of the compromise and the attacker’s actions.
Diagram of Behavior Analytics and SIEM Integration
Imagine a diagram depicting two interconnected boxes. The left box represents the SIEM system, labeled “Security Information and Event Management (SIEM)”. Inside this box, various icons represent different security log sources, such as firewalls, intrusion detection systems (IDS), and endpoint detection and response (EDR) solutions. Arrows point from these icons into a central processing unit within the SIEM box, indicating the collection of security logs.The right box represents the behavior analytics tool, labeled “Behavior Analytics”.
Inside this box, icons represent key functions, such as user behavior modeling, anomaly detection, and threat scoring. A thick, bidirectional arrow connects the central processing unit of the SIEM box to the behavior analytics tool, representing the data exchange between the two systems. This bidirectional flow indicates that the SIEM sends security logs to the behavior analytics tool for analysis, and the behavior analytics tool sends alerts and insights back to the SIEM for further investigation and response.
The diagram visually demonstrates the seamless flow of information between the two systems, highlighting the collaborative nature of their integration. This integrated approach provides a more comprehensive and proactive security posture, enhancing threat detection and response capabilities significantly. For example, a large financial institution might use this integration to detect and respond to fraudulent transactions, leveraging both the real-time alerts from the SIEM and the behavioral analysis to identify patterns of unusual activity.
Addressing Privacy Concerns with Behavior Analytics
The increasing use of behavior analytics in security presents a crucial ethical dilemma: how can we leverage the powerful insights these tools offer while safeguarding individual privacy? The very nature of behavior analytics – analyzing user actions to identify anomalies – inherently involves collecting and processing personal data, raising legitimate concerns about potential misuse and violations of privacy rights.
Balancing the need for enhanced security with the right to privacy requires careful consideration and proactive mitigation strategies.The collection and analysis of user data for security purposes must adhere to strict ethical guidelines and legal frameworks, such as GDPR and CCPA. Failure to do so can lead to significant reputational damage, legal repercussions, and erosion of user trust.
This necessitates a transparent and accountable approach, ensuring users are fully informed about data collection practices and have control over their data.
Data Minimization and Purpose Limitation
Effective data minimization involves collecting only the data strictly necessary for achieving the specified security objective. For instance, instead of logging every mouse click, focus on higher-level actions like login attempts, file access patterns, or unusual network activity. Similarly, purpose limitation restricts the use of collected data solely to its intended security purpose, preventing its repurposing for other, unrelated activities.
This targeted approach significantly reduces the volume of sensitive personal information processed and minimizes the risk of privacy breaches.
Data Anonymization and Pseudonymization
Data anonymization techniques aim to remove or modify personally identifiable information (PII) from datasets, making it impossible to link the data back to specific individuals. Pseudonymization, on the other hand, replaces PII with pseudonyms, allowing for data analysis while preserving a degree of privacy. While perfect anonymization is challenging, applying these techniques reduces the risk of re-identification and protects user privacy.
For example, instead of storing user names, a system might use unique identifiers, making it difficult to link specific actions to individuals.
Data Encryption and Secure Storage
Robust security measures are essential to protect collected behavioral data from unauthorized access and breaches. Data encryption, both in transit and at rest, ensures that even if a breach occurs, the data remains unreadable to unauthorized parties. Secure storage, utilizing access control mechanisms and regular security audits, further reinforces data protection. Implementing multi-factor authentication and rigorous access control policies limits access to sensitive data to authorized personnel only, minimizing the risk of insider threats.
Transparency and User Consent
Transparency is paramount in building trust and ensuring ethical data handling. Users should be clearly informed about the types of data collected, the purpose of collection, how the data is used, and the security measures implemented to protect their privacy. Obtaining explicit and informed consent before collecting and analyzing user behavior is crucial, empowering users to make informed decisions about their data.
This can be achieved through clear and concise privacy policies and consent forms, providing users with meaningful choices regarding their data.
Regular Audits and Compliance
Regular audits and compliance checks are essential to ensure ongoing adherence to privacy regulations and best practices. These audits should evaluate data handling procedures, security measures, and user consent mechanisms, identifying potential vulnerabilities and areas for improvement. Maintaining up-to-date documentation and readily available information on data processing activities allows for effective monitoring and compliance with relevant legal frameworks.
Furthermore, incorporating privacy impact assessments (PIAs) into the development and deployment lifecycle of behavior analytics systems proactively identifies and addresses potential privacy risks.
Best Practices for Responsible Use of Behavior Analytics Data
Implementing the following best practices ensures responsible and ethical use of behavior analytics data:
- Establish clear data governance policies and procedures.
- Implement data minimization and purpose limitation strategies.
- Utilize data anonymization and pseudonymization techniques where possible.
- Employ robust data encryption and secure storage mechanisms.
- Obtain explicit and informed user consent.
- Conduct regular audits and compliance checks.
- Provide transparent and accessible privacy policies.
- Establish mechanisms for data subject access requests.
- Invest in employee training on data privacy and security.
- Regularly review and update security protocols and privacy policies.
Closing Notes
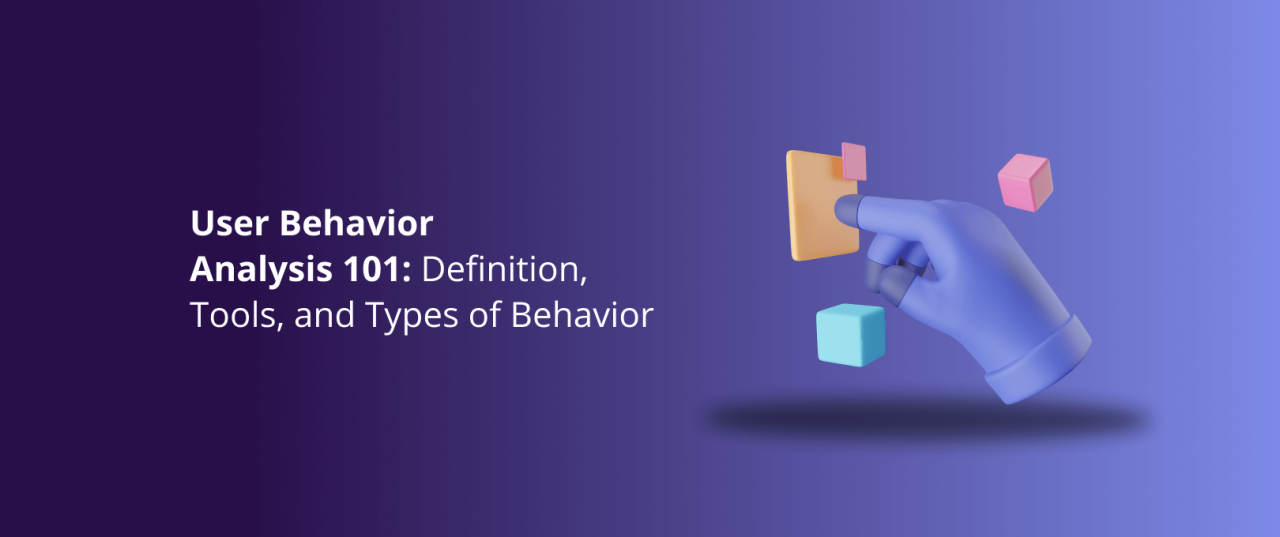
In short, behavior analytics tools are no longer a luxury, but a necessity for any organization serious about cybersecurity. By leveraging the power of data analysis to understand and anticipate threats, we can move beyond reactive security measures and into a proactive, intelligence-driven approach. It’s about shifting from simply reacting to breaches to preventing them altogether, protecting our valuable data and reputation in the process.
The future of cybersecurity is intelligent, and behavior analytics is leading the charge.
Questions Often Asked
What types of data do behavior analytics tools use?
They analyze a wide range of data, including login attempts, file access patterns, network activity, and application usage. The specific data points depend on the tool and the organization’s needs.
Are behavior analytics tools difficult to implement?
Implementation complexity varies depending on the tool and existing infrastructure. Some tools offer simpler integrations than others, and professional services are often available to assist with setup and configuration.
How much does a behavior analytics tool cost?
Pricing models differ significantly across vendors, ranging from subscription-based services to one-time license purchases. Costs depend on factors like the number of users, features, and level of support.
Can behavior analytics tools be used for compliance purposes?
Yes, the data collected can be valuable for demonstrating compliance with various regulations, such as GDPR or HIPAA, by providing evidence of security practices and incident response procedures.