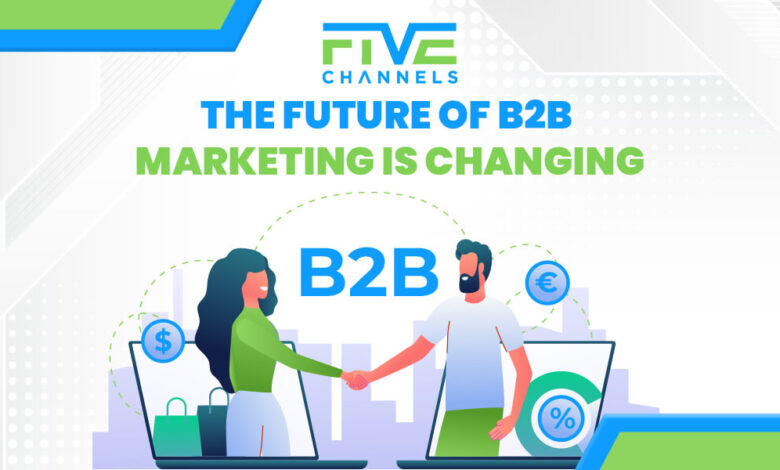
Revolutionizing B2B Research and Discovery
Revolutionizing B2B research and discovery is no longer a futuristic concept; it’s the present reality. The way businesses find, understand, and engage with potential clients is undergoing a seismic shift, driven by advancements in artificial intelligence, machine learning, and big data analytics. This transformation promises unprecedented efficiency, accuracy, and ultimately, success in the complex world of B2B sales and marketing.
We’ll explore how these technologies are reshaping the landscape, examining both the opportunities and challenges that lie ahead.
From the traditional methods of cold calling and market research surveys to the sophisticated algorithms powering today’s lead generation platforms, the journey of B2B research has been remarkable. This post delves into the specifics of AI’s role in enhancing data analysis, the power of predictive analytics, and the ethical considerations surrounding data usage. We’ll also examine how automation is streamlining workflows and making B2B research more efficient than ever before.
Prepare to discover how you can leverage these innovations to gain a competitive edge.
The Evolving Landscape of B2B Research: Revolutionizing B2b Research And Discovery
The world of B2B research has undergone a dramatic transformation, moving from rudimentary methods to sophisticated, data-driven approaches. This evolution has been fueled by technological advancements and a growing understanding of the complexities of the modern business landscape. Understanding this evolution is crucial for any B2B professional seeking to gain a competitive edge.
Historical Progression of B2B Research Methods
Early B2B research relied heavily on qualitative methods, such as in-depth interviews and focus groups. These methods provided valuable insights into customer needs and preferences but were often time-consuming and lacked the scalability necessary for large-scale research projects. The reliance on sales teams for market intelligence was also common. This approach, while offering immediate feedback, lacked the systematic analysis needed for broader strategic decisions.
Gradually, quantitative methods, such as surveys and statistical analysis, gained prominence, allowing for larger sample sizes and more objective data analysis.
Key Technological Advancements Impacting B2B Research
The advent of the internet revolutionized B2B research. The ability to collect data online through surveys, online communities, and social media listening drastically increased the speed and efficiency of research. The development of CRM (Customer Relationship Management) systems provided access to rich customer data, enabling more targeted and personalized research. Furthermore, the rise of big data analytics and artificial intelligence (AI) has allowed researchers to sift through massive datasets to identify patterns and insights that were previously impossible to detect.
These technologies facilitate predictive analytics, allowing businesses to anticipate market trends and customer behavior more effectively. For example, AI-powered tools can analyze social media sentiment to gauge customer perception of a product or service in real-time.
Comparison of Traditional and Modern B2B Research Approaches
Traditional B2B research, often relying on surveys and focus groups, was relatively slow, expensive, and limited in scope. Modern approaches, leveraging big data, AI, and advanced analytics, offer greater speed, efficiency, and depth of insight. Traditional methods provided a snapshot in time, whereas modern methods enable continuous monitoring of customer behavior and market trends. For instance, a traditional telephone survey might capture customer opinions at a single point, while modern social media listening tools provide ongoing feedback on brand perception.
The shift from a primarily qualitative approach to a more quantitative and data-driven approach is a defining characteristic of this evolution.
Timeline of Major Shifts in B2B Research Methodologies
A clear timeline highlights the significant shifts:
Era | Key Methodologies | Technological Influences |
---|---|---|
Pre-1980s | In-depth interviews, focus groups, sales team feedback | Limited technology; reliance on manual data collection |
1980s-1990s | Surveys (mail, telephone), statistical analysis | Spreadsheets, basic statistical software |
2000s-Present | Online surveys, social media listening, CRM data analysis, big data analytics, AI | Internet, CRM systems, advanced statistical software, cloud computing, AI algorithms |
AI and Machine Learning’s Role in B2B Discovery
The B2B landscape is rapidly evolving, driven by the increasing availability and sophistication of data. AI and machine learning are no longer futuristic concepts; they are powerful tools reshaping how businesses identify, qualify, and engage with potential clients. This transformation is particularly impactful in B2B research and discovery, offering unprecedented opportunities for efficiency and accuracy.
AI’s Enhancement of Data Analysis for B2B Lead Generation
AI significantly boosts the effectiveness of data analysis in B2B lead generation by automating tasks that were previously time-consuming and prone to human error. Algorithms can sift through vast datasets – encompassing CRM data, website analytics, social media activity, and market research reports – to identify patterns and insights invisible to the human eye. This includes identifying leads exhibiting high propensity to convert, based on factors such as website engagement, content downloads, and demonstrated interest in specific products or services.
For example, an AI system might analyze website traffic to identify visitors who have spent significant time on pricing pages or downloaded product specifications, flagging them as high-potential leads. This allows sales teams to focus their efforts on the most promising prospects, increasing conversion rates and optimizing resource allocation.
Machine Learning Applications in Identifying Ideal Customer Profiles
Machine learning plays a crucial role in refining and defining ideal customer profiles (ICPs). By analyzing historical sales data and customer information, machine learning algorithms can identify common characteristics among successful customers. This goes beyond simple demographics and encompasses behavioral patterns, technological usage, industry specifics, and company size. This detailed ICP allows for more targeted marketing campaigns and a more effective sales approach.
For instance, a machine learning model might reveal that companies with a specific technology stack and a certain annual revenue range are more likely to adopt a particular software solution. This precise targeting reduces wasted effort and improves the overall ROI of marketing and sales activities.
Predictive Analytics’ Impact on B2B Sales Strategies
Predictive analytics, a subset of machine learning, allows businesses to forecast future outcomes based on historical data. In B2B sales, this translates to anticipating customer behavior, predicting sales cycles, and optimizing sales strategies. For example, predictive models can identify leads likely to churn or those poised to make a significant purchase. This allows for proactive interventions – offering targeted support to at-risk customers or preparing customized proposals for high-value prospects.
Predictive analytics also helps optimize sales resource allocation by prioritizing leads with the highest probability of conversion, thus maximizing sales team efficiency and revenue generation. A company using predictive analytics might discover that contacting leads within 24 hours of a website visit significantly increases the likelihood of a successful engagement.
Comparison of AI-Powered B2B Research Tools
The following table compares several AI-powered B2B research tools, highlighting their features, pricing, and target audience. Note that pricing can vary depending on specific needs and contract terms.
Tool Name | Key Features | Pricing Model | Target Audience |
---|---|---|---|
Salesforce Einstein | Lead scoring, opportunity insights, predictive analytics | Subscription-based, tiered pricing | Large enterprises, sales and marketing teams |
HubSpot Sales Hub | Contact management, deal tracking, sales automation | Subscription-based, tiered pricing | SMBs and enterprises, sales and marketing teams |
ZoomInfo | Lead generation, company intelligence, contact data | Subscription-based, tiered pricing | Sales and marketing teams, business development |
Leadfeeder | Website visitor identification, lead scoring | Subscription-based, tiered pricing | SMBs and enterprises, marketing and sales teams |
Data-Driven Insights and Decision Making in B2B
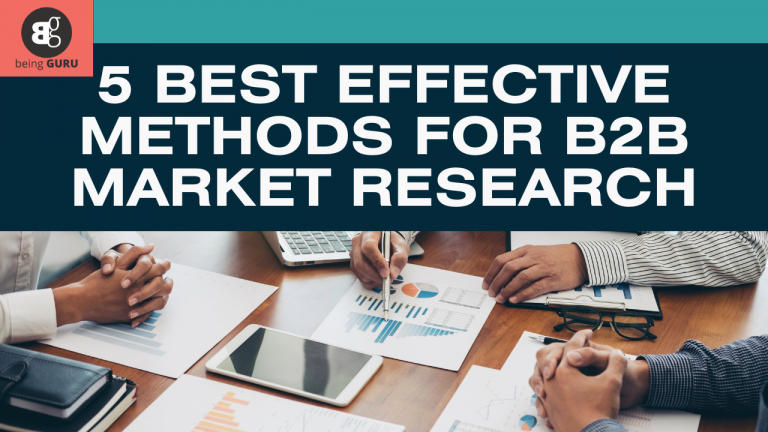
The explosion of readily available data has fundamentally altered the B2B landscape. No longer are gut feelings and anecdotal evidence sufficient; successful businesses now rely on data-driven insights to inform every aspect of their operations, from lead generation to customer retention. This shift towards data-centric decision-making empowers B2B companies to achieve greater efficiency, profitability, and a deeper understanding of their target market.Big data’s contribution to more effective B2B targeting is undeniable.
The sheer volume, variety, velocity, and veracity of available data allows for highly granular segmentation and personalized outreach.
Big Data’s Impact on B2B Targeting
By analyzing data points like website behavior, social media engagement, purchase history, and firmographic information (company size, industry, location), businesses can create detailed buyer personas. This allows for the development of highly targeted marketing campaigns that resonate with specific customer segments. For example, a SaaS company might identify a subset of prospects exhibiting high website engagement with their pricing page and actively targeting them with case studies demonstrating ROI.
This level of precision significantly improves conversion rates compared to broad, generic marketing efforts.
Real-Time Data Analysis in B2B Sales Processes
Real-time data analysis transforms the B2B sales process by providing immediate feedback and actionable insights. Sales teams can leverage tools that track lead interactions, providing insights into engagement levels and identifying potential deal blockers. Imagine a sales representative receiving a real-time alert indicating a prospect has just downloaded a pricing document. This allows for immediate follow-up, increasing the likelihood of conversion.
Furthermore, analyzing sales pipeline data in real-time allows for quicker identification of bottlenecks and the proactive adjustment of sales strategies. A sudden drop in qualified leads, for example, might prompt a review of the marketing campaign or lead generation process.
Data Visualization for Communicating B2B Research Findings
Effectively communicating research findings is crucial for securing buy-in from stakeholders and driving action. Data visualization plays a key role in this process. Instead of presenting lengthy reports filled with numbers, clear and concise visualizations – such as interactive dashboards, charts, and graphs – can communicate complex data in an easily digestible format. For example, a heatmap illustrating geographic concentration of high-value prospects can quickly convey market opportunities.
Revolutionizing B2B research and discovery requires innovative tools, and that’s where efficient application development comes in. I’ve been exploring how platforms like those discussed in this article on domino app dev the low code and pro code future can streamline the process. Ultimately, faster development cycles directly translate to quicker insights and a more competitive edge in B2B research and discovery.
Similarly, a line graph showcasing the correlation between marketing spend and lead generation can demonstrate the ROI of specific campaigns.
Translating Data Insights into Actionable Strategies
A visual representation of this process could be a flowchart. The flowchart begins with the “Data Collection” box, representing the gathering of various data points from different sources. This feeds into the “Data Analysis” box, where techniques like machine learning are used to identify patterns and insights. The “Insight Generation” box follows, showcasing key findings such as ideal customer profiles or areas for improvement.
From here, the flowchart branches into different “Actionable Strategies” boxes, each representing a specific action plan based on the insights. For example, one branch could be “Targeted Marketing Campaign,” another could be “Sales Process Optimization,” and a third could be “Product Development.” Finally, the flowchart concludes with a “Performance Measurement” box, highlighting the use of Key Performance Indicators (KPIs) to track the success of implemented strategies and to inform future iterations of the process.
This closed-loop system demonstrates the iterative nature of data-driven decision-making.
Automation and Efficiency in B2B Research
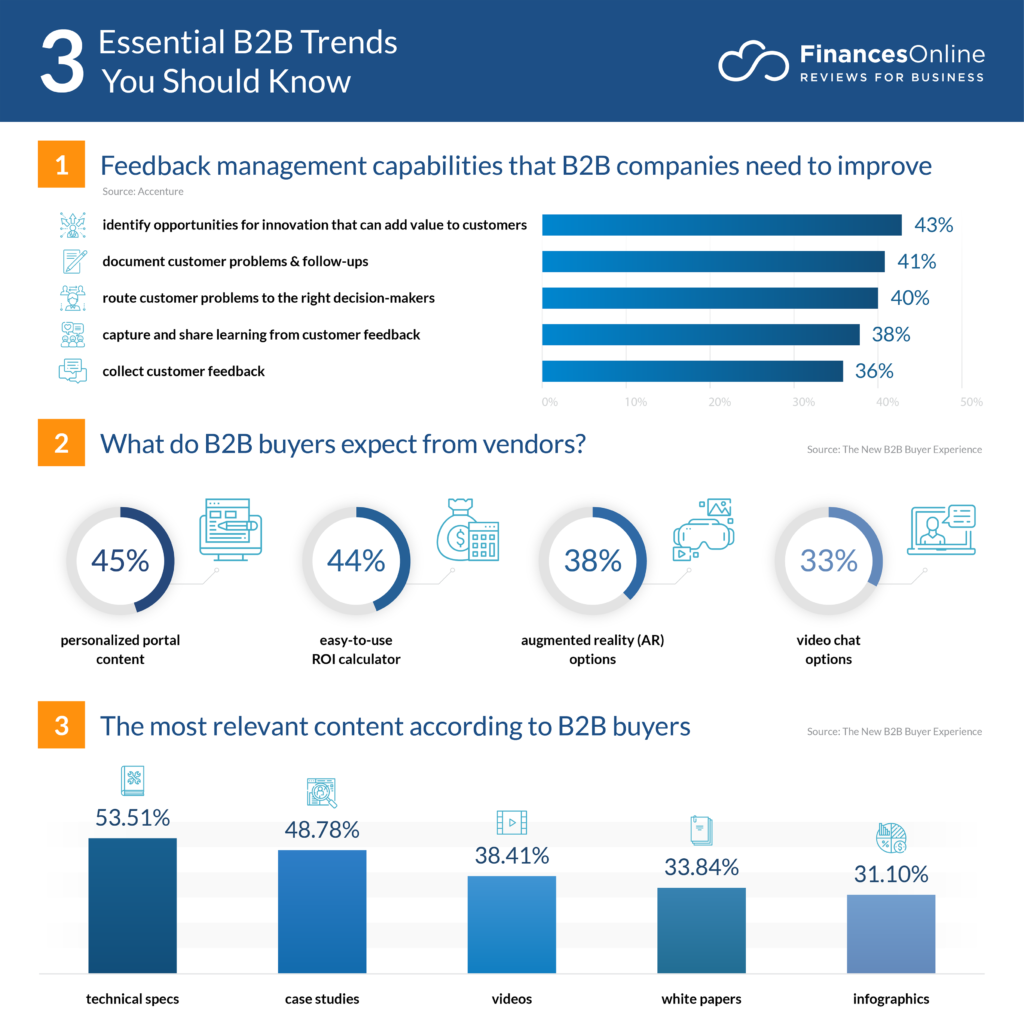
The B2B landscape is increasingly competitive, demanding faster, more efficient research processes. Manual research methods, while offering deep dives into specific areas, often fall short in terms of speed and scalability. Automation, however, offers a powerful solution, streamlining workflows and freeing up valuable time for strategic analysis. By leveraging automated tools and techniques, B2B researchers can significantly improve their efficiency and accuracy, ultimately leading to better-informed decisions.Automating various aspects of B2B research dramatically accelerates the process.
Imagine the time saved by automatically collecting and organizing data from multiple sources, instead of manually combing through websites and reports. This efficiency gain allows researchers to focus on higher-level tasks, such as interpreting data and developing strategic recommendations. The speed advantage translates directly into faster market analysis, quicker identification of potential leads, and ultimately, a competitive edge in the marketplace.
Specific Tasks Automating in B2B Research
Automation isn’t about replacing human researchers; it’s about augmenting their capabilities. Several tasks within the B2B research process lend themselves well to automation. These include data collection from various online sources, lead generation and qualification, competitor analysis, and even the initial stages of report generation. For instance, web scraping tools can automatically gather information from company websites, while CRM systems can automate lead nurturing and qualification processes.
This frees up researchers to focus on the more complex aspects of analysis and interpretation.
Cost-Effectiveness of Automated vs. Manual Research
While the initial investment in automation tools might seem significant, the long-term cost-effectiveness is undeniable. Manual research is labor-intensive, requiring significant time and resources. The cost of employing dedicated researchers, managing their time, and ensuring data accuracy adds up quickly. Automation, on the other hand, reduces labor costs, minimizes human error, and speeds up the research process, leading to significant savings in the long run.
A company conducting market research might find that automated tools reduce research time by 50%, resulting in substantial cost savings over a year. This efficiency translates into a faster return on investment (ROI) for the automation tools themselves.
Software and Tools for Automating B2B Research
The availability of powerful software and tools designed specifically for automating B2B research is constantly expanding. These tools cater to different aspects of the research process, offering a range of functionalities to streamline workflows.
Several categories of tools exist to address various needs:
- Web Scraping Tools: These tools automatically extract data from websites, such as company profiles, contact information, and news articles. Examples include ParseHub, Apify, and Octoparse. These tools can significantly reduce the time spent manually collecting data from numerous websites.
- CRM Systems: Customer Relationship Management (CRM) systems like Salesforce and HubSpot automate lead generation, qualification, and nurturing processes. They provide a centralized database for managing customer interactions and streamline communication efforts.
- Market Research Platforms: Platforms such as Statista and IBISWorld provide access to extensive market data and reports, often with automated data extraction and analysis capabilities. These reduce the need for manual data gathering and analysis.
- AI-Powered Research Assistants: Emerging AI tools can assist with tasks like summarizing lengthy documents, identifying key insights from data, and even generating initial drafts of reports. While still developing, these tools represent a significant advancement in automating complex research tasks.
Overcoming Challenges in Modern B2B Research
The rapid advancement of technology, particularly AI and machine learning, has revolutionized B2B research, offering unprecedented opportunities for data-driven insights. However, this progress also introduces new challenges that require careful consideration and proactive mitigation strategies. Successfully navigating these challenges is crucial for ensuring the ethical, accurate, and reliable use of data in B2B decision-making.
Ethical Considerations of AI in B2B Research
The application of AI in B2B research raises several ethical concerns. Algorithmic bias, for instance, can lead to unfair or discriminatory outcomes if not properly addressed. AI models trained on biased data will perpetuate and even amplify those biases in their predictions and recommendations, potentially leading to skewed market analysis or flawed customer segmentation. Furthermore, the lack of transparency in some AI algorithms can make it difficult to understand how decisions are made, raising concerns about accountability and fairness.
Robust ethical guidelines and rigorous testing are necessary to ensure AI systems are used responsibly and equitably in B2B research. Regular audits and human oversight are essential to mitigate potential biases and ensure ethical conduct.
Potential Biases in Data-Driven B2B Research and Mitigation Strategies, Revolutionizing b2b research and discovery
Data-driven B2B research is susceptible to various biases that can compromise the accuracy and reliability of findings. Sampling bias, for example, occurs when the sample used for research does not accurately represent the target population. This can lead to inaccurate generalizations and flawed conclusions. Confirmation bias, on the other hand, involves favoring information that confirms pre-existing beliefs and ignoring contradictory evidence.
To mitigate these biases, researchers should employ rigorous sampling techniques to ensure representative samples, utilize diverse data sources to cross-validate findings, and actively seek out and incorporate contradictory evidence into their analysis. Blind testing methodologies can also help minimize the influence of researcher bias. For example, in a customer satisfaction survey, the researchers should not know which customers are using a particular product before evaluating their feedback.
Data Security and Privacy in B2B Research
Protecting sensitive data is paramount in B2B research. The use of personal and business data requires strict adherence to data privacy regulations like GDPR and CCPA. Data breaches can have severe consequences, damaging reputations and leading to significant financial losses. Implementing robust security measures, such as encryption and access control, is essential to safeguard data. Furthermore, researchers must obtain informed consent from data subjects and ensure transparency about how data will be used.
Regular security audits and employee training programs on data protection best practices are vital to maintaining a secure research environment. Consider the case of a marketing firm using customer data to personalize ads; robust security protocols are crucial to prevent data leaks and maintain customer trust.
Ensuring the Accuracy and Reliability of B2B Research Data
The accuracy and reliability of B2B research data are crucial for effective decision-making. This requires careful attention to data quality throughout the research process. Data cleaning and validation techniques are essential to identify and correct errors or inconsistencies. Triangulation, the process of using multiple data sources to verify findings, enhances the credibility of research results. For instance, combining survey data with sales figures can provide a more comprehensive understanding of market trends.
Regularly updating data sources and employing statistical methods to assess data reliability further enhance the trustworthiness of B2B research. A company using outdated market research data might make poor investment decisions, highlighting the importance of data accuracy and timely updates.
Future Trends in B2B Research and Discovery
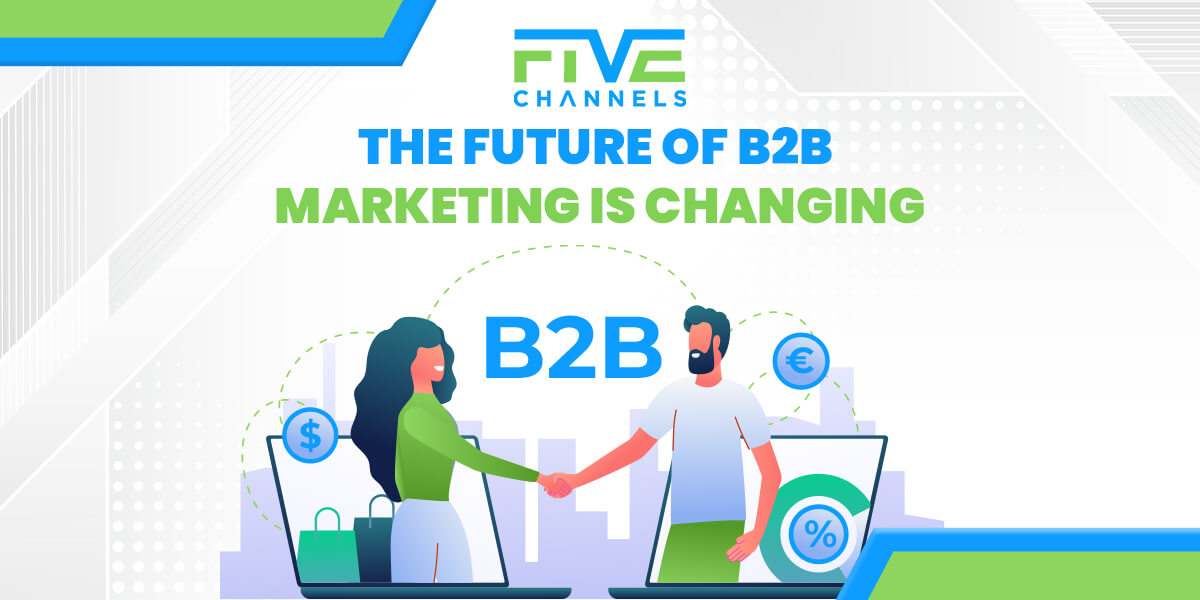
The landscape of B2B research is undergoing a rapid transformation, driven by the convergence of advanced technologies and evolving business needs. We’re moving beyond traditional methods, embracing a future where data-driven insights, automation, and human expertise work in synergy to unlock unprecedented levels of understanding and efficiency. This shift promises to reshape how businesses identify, qualify, and engage with their ideal customers.The next few years will witness a significant impact from emerging technologies, fundamentally altering B2B research methodologies.
This evolution will require B2B researchers to adapt and acquire new skills to remain competitive and effective.
The Impact of Emerging Technologies on B2B Research
Emerging technologies like artificial intelligence (AI), machine learning (ML), and natural language processing (NLP) are poised to revolutionize B2B research. AI-powered tools can automate data collection, analysis, and reporting, freeing up researchers to focus on higher-level strategic tasks. ML algorithms can identify patterns and insights hidden within vast datasets, leading to more accurate and nuanced customer segmentation and predictive analytics.
NLP enables the automated processing and analysis of unstructured data like social media posts and customer reviews, providing valuable qualitative insights. For example, a company could use AI to analyze thousands of customer service transcripts to identify recurring issues or sentiment trends, allowing for proactive improvements to products or services. This automated analysis surpasses the capacity of human researchers to sift through such volumes of data efficiently.
Disruptions and Innovations in B2B Research Methodologies
The integration of these technologies will lead to significant disruptions and innovations in B2B research methodologies. We can anticipate a rise in predictive analytics, enabling businesses to anticipate market trends and customer behavior with greater accuracy. The use of real-time data streams will allow for more agile and responsive research, enabling businesses to adapt quickly to changing market conditions.
Furthermore, the increased use of automation will reduce the reliance on manual processes, leading to significant cost savings and increased efficiency. For instance, instead of relying solely on surveys, businesses might integrate AI-powered sentiment analysis of social media conversations to understand customer perceptions in real-time, enabling faster responses to negative feedback or emerging trends.
The Evolving Role of Human Expertise in Automated B2B Research
Despite the rise of automation, the role of human expertise remains crucial. While AI and ML can process vast amounts of data and identify patterns, they lack the critical thinking, creativity, and contextual understanding that human researchers bring to the table. Humans are essential for interpreting complex data, formulating research questions, and ensuring the ethical and responsible use of data.
The future of B2B research lies in a collaborative approach, where humans and machines work together to leverage the strengths of both. Humans will focus on strategic decision-making, creative problem-solving, and ensuring the ethical and responsible use of AI-driven insights, while machines handle the more repetitive and data-intensive tasks.
Key Skills for Future B2B Researchers
The future of B2B research demands a new set of skills. Researchers will need to be proficient in data analysis, statistics, and programming languages like Python or R. A strong understanding of AI and ML principles is also becoming increasingly important, alongside expertise in data visualization and communication. Crucially, adaptability and a willingness to embrace new technologies will be essential.
Furthermore, strong critical thinking, problem-solving, and communication skills remain vital for interpreting complex data and effectively communicating findings to stakeholders. The ability to work collaboratively with AI and ML tools will be paramount, highlighting the need for a blended skillset incorporating both technical proficiency and human judgment.
Concluding Remarks
The revolution in B2B research and discovery is not just about adopting new tools; it’s about embracing a new mindset. By leveraging the power of AI, big data, and automation, businesses can move beyond guesswork and make data-driven decisions that significantly improve their sales and marketing strategies. While challenges exist – ethical concerns, data bias, and security – the potential rewards are immense.
The future of B2B research is bright, promising more efficient, accurate, and ethical ways to connect with ideal customers. Embrace the change, and watch your business thrive.
General Inquiries
What are the biggest risks of relying solely on AI for B2B research?
Over-reliance on AI can lead to biased results if the data used to train the algorithms is flawed. It also risks neglecting the crucial human element of understanding nuanced customer behaviors and market trends.
How can I ensure the data privacy and security of my B2B research?
Employ robust data encryption, comply with relevant privacy regulations (GDPR, CCPA, etc.), and use reputable, secure research platforms. Transparency with clients regarding data usage is also crucial.
What are some cost-effective ways to implement AI-powered B2B research?
Start with readily available, affordable AI tools and gradually scale up as needed. Focus on specific areas where AI can deliver the highest ROI, such as lead scoring or predictive modeling.