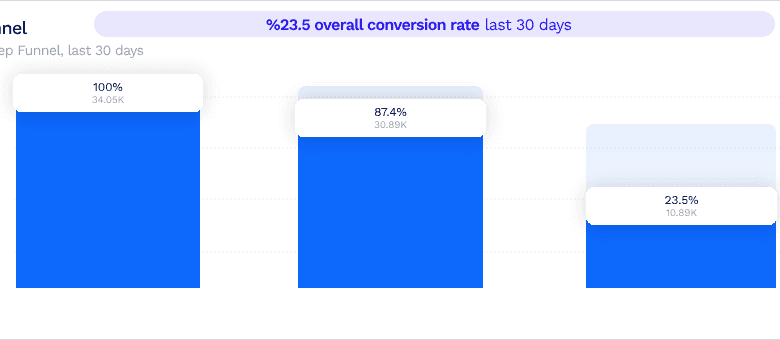
Is Customer Behaviour Analytics on Your Radar? With Increasing Digital Sales, It Should Be
Is customer behaviour analytics on your radar with increasing digital sales it should be – Is customer behaviour analytics on your radar with increasing digital sales? It should be! In today’s fiercely competitive digital landscape, understanding your customers’ online actions isn’t just beneficial – it’s essential for survival. Ignoring the wealth of data available about how your customers interact with your brand online is like sailing a ship without a map. This post dives into why understanding customer behavior is crucial, how to track key metrics, and how to use that information to boost your bottom line.
We’ll explore various data collection methods, ethical considerations, and how to transform raw data into actionable insights that improve your website design, app functionality, and marketing strategies. We’ll even touch on the exciting possibilities of predictive analytics and AI, showing you how to personalize the customer experience and stay ahead of the curve.
The Rising Importance of Understanding Digital Customer Behavior: Is Customer Behaviour Analytics On Your Radar With Increasing Digital Sales It Should Be
In today’s hyper-competitive digital landscape, understanding your customer’s online behavior isn’t just a nice-to-have; it’s a necessity. With a significant portion of sales now happening online, businesses that fail to grasp the nuances of digital customer behavior risk falling behind. The ability to track, analyze, and act upon this data is no longer a luxury but a critical component of sustainable growth.The correlation between increasing digital sales and the need for robust customer behavior understanding is undeniable.
As more transactions migrate online, the opportunity to gather rich data about customer preferences, purchase patterns, and overall online journey expands exponentially. This data allows for targeted marketing campaigns, personalized experiences, and optimized website design, all of which directly contribute to increased sales and customer loyalty. Ignoring this wealth of information is akin to navigating a vast ocean without a map – you might stumble upon some treasure, but you’re far more likely to get lost.
Risks of Ignoring Customer Behavior Analytics
Ignoring customer behavior analytics in a growing digital marketplace carries substantial risks. Businesses that fail to analyze customer data are essentially flying blind, making decisions based on guesswork rather than concrete evidence. This can lead to ineffective marketing campaigns, wasted resources, and a diminished ability to compete with more data-driven competitors. Lost opportunities to personalize the customer experience can result in lower conversion rates, increased cart abandonment, and ultimately, reduced profitability.
Furthermore, a lack of understanding of customer pain points can hinder product development and lead to the creation of products or services that fail to meet market demand.
Examples of Successful Businesses Leveraging Customer Behavior Data, Is customer behaviour analytics on your radar with increasing digital sales it should be
Many businesses have successfully leveraged customer behavior data to significantly boost their digital sales. Analyzing website traffic, user engagement, and purchase history allows for data-driven decisions that enhance the customer experience and drive revenue.
Company Name | Strategy Used | Results Achieved | Key Learnings |
---|---|---|---|
Amazon | Personalized recommendations based on browsing and purchase history; A/B testing of website design and features; targeted advertising based on user demographics and interests. | Significant increase in sales and customer engagement; higher conversion rates; improved customer retention. | Understanding individual customer preferences is crucial for personalization; continuous A/B testing is essential for optimization; data-driven decisions are more effective than intuition. |
Netflix | Recommendation engine based on viewing history and user ratings; A/B testing of content presentation and user interface; data-driven content creation. | Increased subscriber engagement and retention; higher average revenue per user; successful expansion into original content production. | Understanding user preferences is key to personalized content recommendations; continuous improvement based on data analysis is crucial for long-term success; data can inform content strategy and creation. |
Spotify | Personalized playlists and recommendations based on listening habits; targeted advertising based on musical preferences; data-driven playlist curation. | Increased user engagement and listening time; higher premium subscription rates; improved user experience. | Understanding user listening habits is key to personalized recommendations; data-driven playlist curation enhances user experience; targeted advertising increases revenue. |
Nike | Personalized marketing campaigns based on customer demographics and fitness goals; data-driven product development; use of wearable technology to track customer activity and preferences. | Increased brand loyalty and customer engagement; improved product design and functionality; higher sales conversion rates. | Integrating data from multiple sources provides a holistic view of the customer; data-driven product development leads to products that better meet customer needs; wearable technology enhances data collection and personalization. |
Key Metrics for Tracking Digital Customer Behavior
Understanding your digital customers is no longer a luxury; it’s a necessity. In today’s competitive landscape, businesses that fail to track and analyze customer behavior risk losing ground to more data-driven competitors. By focusing on key metrics, you can gain valuable insights into what works, what doesn’t, and how to optimize your online presence for maximum impact. This allows for data-driven decisions, rather than relying on gut feeling.
Website Traffic and Sources
Analyzing website traffic provides a foundational understanding of your audience reach. This involves monitoring the total number of visitors, their geographic location, and crucially, the sources driving this traffic (organic search, social media, paid advertising, email marketing, etc.). For example, a spike in traffic from a specific social media campaign indicates a successful marketing effort, while low traffic from organic search might highlight the need for improvements.
Understanding traffic sources allows for optimized resource allocation, focusing marketing spend on the most effective channels.
Conversion Rate
Conversion rate measures the percentage of website visitors who complete a desired action, such as making a purchase, signing up for a newsletter, or filling out a contact form. A low conversion rate signals potential issues with the website design, user experience, or the overall sales process. For instance, a high bounce rate (visitors leaving after viewing only one page) might suggest poor website navigation or irrelevant content.
Analyzing conversion rates helps pinpoint areas for improvement, leading to increased sales and customer acquisition. A 5% conversion rate, for example, means that for every 100 visitors, 5 complete the desired action.
Average Order Value (AOV)
Average Order Value represents the average amount spent per order. Tracking AOV helps businesses understand customer spending habits and identify opportunities to increase revenue. For example, implementing upselling and cross-selling strategies can significantly boost AOV. Offering related products or higher-priced alternatives during checkout can increase the average purchase value. A high AOV indicates successful upselling and cross-selling, while a low AOV suggests potential improvements to these strategies.
Customer Lifetime Value (CLTV)
Customer Lifetime Value predicts the total revenue a customer will generate throughout their relationship with your business. This metric is crucial for long-term strategic planning, allowing businesses to prioritize customer retention and loyalty programs. A high CLTV suggests effective customer engagement and retention strategies. For example, a loyalty program offering exclusive discounts and early access to new products can increase CLTV significantly.
By focusing on customers with high CLTV, businesses can optimize their marketing and customer service efforts.
Customer Churn Rate
Customer churn rate measures the percentage of customers who stop doing business with a company within a specific period. High churn rates indicate potential problems with product quality, customer service, or pricing. Analyzing churn rate helps businesses identify areas for improvement and implement retention strategies. For instance, proactively addressing customer concerns and improving customer support can reduce churn.
A high churn rate signals the need for a comprehensive review of the customer experience.
Visual Representation
Imagine a circular diagram, like a pie chart, with five segments, each representing one of the metrics: Website Traffic, Conversion Rate, Average Order Value, Customer Lifetime Value, and Customer Churn Rate. Arrows connect these segments to show the relationships. For instance, a larger Website Traffic segment would feed into a higher Conversion Rate segment (assuming a constant conversion rate), which in turn would influence a higher Average Order Value.
A high Customer Lifetime Value would be a result of both high conversion rates and high average order value, while a low Customer Churn Rate would reinforce a high CLTV. The size of each segment would visually represent the relative importance or magnitude of each metric for the specific business. The arrows visually represent the causal relationships and dependencies between these key performance indicators.
Data Collection and Analysis Methods
Understanding digital customer behavior requires robust data collection and analysis. The methods employed directly impact the insights gained, influencing strategic decisions and ultimately, business success. Choosing the right approach depends on factors like budget, technical capabilities, and the specific questions you’re trying to answer.
Several methods exist for gathering this crucial information, each with its strengths and weaknesses. Let’s examine three common approaches and explore best practices for responsible data handling.
Comparison of Online Customer Behavior Data Collection Methods
Three prominent methods for collecting online customer behavior data are website analytics, customer relationship management (CRM) systems, and A/B testing.
- Website Analytics: Tools like Google Analytics provide a wealth of data on website traffic, user behavior (e.g., bounce rate, time on site, pages visited), and conversions. This method offers a broad overview of user interactions with your website. However, it primarily focuses on aggregate data and may not provide detailed insights into individual customer journeys.
- Customer Relationship Management (CRM) Systems: CRMs collect data on customer interactions across multiple channels, including email, social media, and customer service interactions. This provides a more holistic view of individual customer behavior, including purchase history, preferences, and support requests. The limitation lies in the potential for data silos if not properly integrated with other data sources.
- A/B Testing: This method involves presenting different versions of a webpage or marketing message to different user segments and tracking their responses. A/B testing provides highly specific data on the effectiveness of various design choices and marketing strategies. However, it is focused on specific elements and might not capture the overall user experience.
Data Privacy and Ethical Considerations
Ethical and legal considerations are paramount when collecting and analyzing customer behavior data. Transparency and user consent are crucial.
- Obtain Informed Consent: Clearly inform users about the data you are collecting, how it will be used, and their rights regarding their data. Provide easily accessible privacy policies and ensure compliance with relevant regulations like GDPR and CCPA.
- Data Minimization: Collect only the data necessary for your specific purposes. Avoid collecting excessive or irrelevant information.
- Data Security: Implement robust security measures to protect customer data from unauthorized access, use, or disclosure. This includes encryption, access controls, and regular security audits.
- Anonymization and Pseudonymization: Where possible, anonymize or pseudonymize data to protect individual identities. This involves removing or replacing identifying information while retaining the data’s analytical value.
Step-by-Step Procedure for Analyzing Collected Data
Analyzing collected data requires a structured approach to extract actionable insights. A systematic process ensures that you derive meaningful conclusions from your data.
- Define Objectives: Clearly articulate the business questions you want to answer through data analysis. For example, “What are the most effective channels for acquiring new customers?” or “How can we improve website conversion rates?”.
- Data Cleaning and Preparation: Cleanse the data to remove inconsistencies, errors, and duplicates. Transform the data into a suitable format for analysis.
- Exploratory Data Analysis (EDA): Use visualization techniques (e.g., charts, graphs) and summary statistics to understand the data’s distribution and identify patterns and trends.
- Statistical Analysis: Apply appropriate statistical methods (e.g., regression analysis, hypothesis testing) to test hypotheses and identify significant relationships between variables.
- Develop Actionable Insights: Translate the statistical findings into practical recommendations for improving customer experience, marketing strategies, or product development.
- Monitor and Iterate: Continuously monitor the effectiveness of implemented changes and iterate on your analysis based on new data and insights.
Using Insights to Improve the Customer Journey
Understanding your digital customer’s journey is crucial for success in today’s competitive landscape. Customer behavior analytics provides the data-driven insights needed to identify friction points, optimize the user experience, and ultimately, boost conversions. By analyzing how customers interact with your website and apps, you can uncover hidden opportunities to enhance their experience and drive business growth.Customer behavior analytics offers a powerful lens through which to examine the entire customer journey, from initial awareness to final purchase (and beyond).
By tracking key metrics and analyzing user behavior patterns, businesses can pinpoint exactly where customers are encountering difficulties or abandoning their interactions. This allows for targeted improvements that directly address these pain points, leading to a smoother, more efficient, and ultimately more profitable customer journey.
Identifying Pain Points in the Digital Customer Journey
Analyzing website bounce rates, session durations, and heatmaps can reveal areas of friction. For instance, a high bounce rate on a specific product page might indicate confusing product descriptions or poor image quality. Similarly, low session durations on a checkout page suggest a cumbersome or overly complex checkout process. Heatmaps can visually show where users are clicking and scrolling, highlighting areas of interest and areas that are being ignored.
A lack of clicks on a crucial call-to-action button, for example, clearly indicates a problem with its visibility or design. By identifying these pain points, businesses can make data-driven decisions to improve the overall user experience. For example, a company selling handmade jewelry might discover through heatmap analysis that customers are consistently overlooking the “add to cart” button because it blends too seamlessly with the background.
Adjusting the button’s color or placement would directly address this issue.
Optimizing Website Design, App Functionality, and Marketing Campaigns
The insights gleaned from customer behavior analytics directly translate into actionable improvements. A high cart abandonment rate, for example, might be addressed by simplifying the checkout process, offering guest checkout options, or adding clear shipping cost information upfront. Analyzing user flows within a mobile app can identify areas where navigation is confusing or where users are struggling to complete desired actions.
This could lead to redesigning the app’s menu structure or simplifying the steps involved in a key function. In marketing, understanding which channels are driving the most qualified leads and which campaigns are resulting in the highest conversion rates allows for optimized resource allocation and more effective targeting. A company might discover that its email marketing campaigns are significantly more effective than social media ads, allowing them to focus their marketing budget on email marketing initiatives.
Improving Conversion Rates Using Customer Behavior Data
Below is a description of a flowchart illustrating how to use customer behavior data to improve conversion rates.The flowchart begins with Data Collection: This involves gathering data from various sources like website analytics, app usage data, CRM systems, and marketing automation platforms. This data is then fed into Data Analysis: This step involves using tools and techniques to identify patterns, trends, and anomalies in customer behavior.
This might include segmenting users based on demographics, behavior, or other relevant factors. The next stage is Identify Pain Points: This involves pinpointing areas in the customer journey where users are dropping off, experiencing difficulties, or failing to convert. This analysis might reveal issues with website navigation, confusing product descriptions, or a cumbersome checkout process. The identified pain points are then used to Develop Solutions: This involves brainstorming and implementing changes to address the issues identified in the previous step.
These solutions might include redesigning web pages, improving app functionality, simplifying the checkout process, or optimizing marketing campaigns. The final stage is Implementation & Monitoring: This involves putting the solutions into effect and closely monitoring their impact on key metrics such as conversion rates, bounce rates, and customer satisfaction. The results are then used to further refine and optimize the customer journey.
This cyclical process ensures continuous improvement based on real-time customer feedback and data.
Predictive Analytics and Future Trends
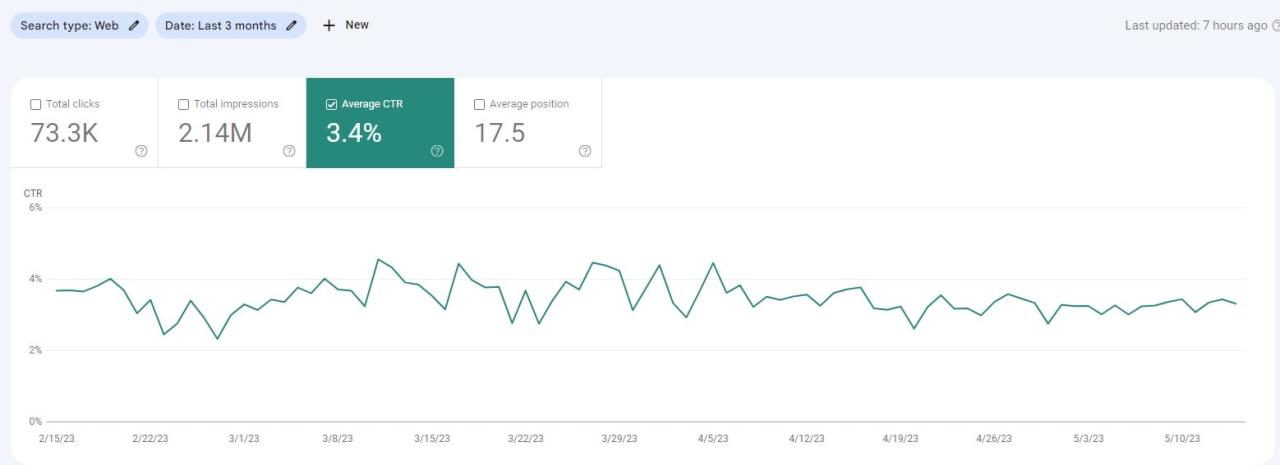
Predictive analytics is no longer a futuristic concept; it’s a vital tool for businesses navigating the increasingly complex landscape of digital customer behavior. By leveraging historical data and advanced algorithms, companies can gain powerful insights into future customer actions, allowing for proactive adjustments to strategies and a more personalized customer experience. This capability is especially crucial as digital sales continue their upward trajectory, demanding a deeper understanding of the evolving needs and preferences of online consumers.The ability to predict customer behavior offers significant competitive advantages.
Instead of reacting to market changes, businesses can anticipate them, optimizing resource allocation, improving marketing ROI, and ultimately driving revenue growth. This proactive approach allows for a more agile and responsive business model, better equipped to handle market fluctuations and evolving customer demands.
AI and Machine Learning in Predictive Analytics
Artificial intelligence (AI) and machine learning (ML) are revolutionizing predictive analytics, enabling the processing and analysis of massive datasets far beyond the capabilities of traditional methods. These technologies can identify intricate patterns and correlations in customer data that might otherwise go unnoticed, leading to more accurate predictions. For instance, AI-powered recommendation engines analyze past purchase history, browsing behavior, and demographic data to suggest relevant products or services, increasing conversion rates and customer satisfaction.
Machine learning algorithms can also predict customer churn, allowing businesses to proactively intervene with retention strategies before losing valuable customers. Netflix’s recommendation system is a prime example of successful AI-driven predictive analytics, significantly impacting user engagement and subscription retention.
Personalizing the Customer Experience Through Predictive Capabilities
Predictive analytics empowers businesses to create truly personalized customer experiences. By anticipating individual needs and preferences, companies can tailor their marketing messages, product offerings, and customer service interactions for maximum impact. Imagine a clothing retailer using predictive analytics to anticipate a customer’s upcoming need for a new winter coat based on their past purchases, location, and browsing history. The retailer could then proactively send a personalized email showcasing relevant products, potentially including a discount code to incentivize a purchase.
This level of personalization fosters stronger customer relationships, increased loyalty, and ultimately, improved profitability. Similarly, a financial institution might use predictive analytics to identify customers at risk of defaulting on a loan, allowing them to offer tailored support and financial guidance to mitigate the risk.
Predictive Modeling Techniques and Their Applications
Several powerful predictive modeling techniques are currently employed. Regression analysis, for example, helps predict a continuous outcome variable (like customer spending) based on several predictor variables (like demographics and purchase history). Classification models, on the other hand, predict a categorical outcome (like whether a customer will churn or not). These models, powered by AI and ML, are constantly being refined and improved, leading to increasingly accurate predictions.
For example, a telecom company might use a classification model to predict which customers are likely to switch providers, allowing them to implement targeted retention campaigns. The use of such models allows businesses to allocate resources efficiently and optimize marketing spend, focusing on the most promising customer segments.
Closing Summary
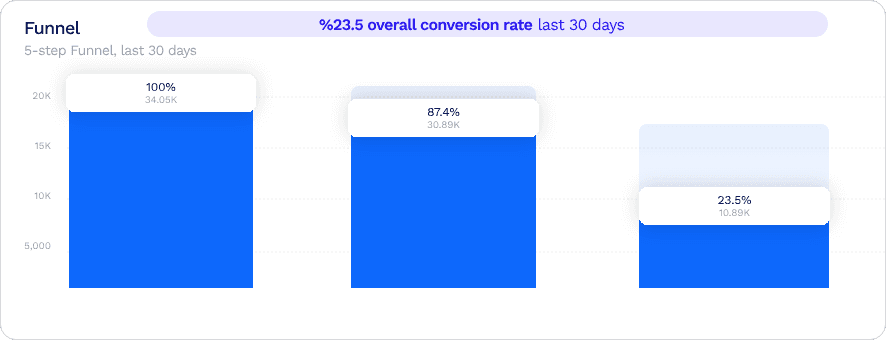
Ultimately, mastering customer behaviour analytics isn’t about simply collecting data; it’s about using that data to build stronger relationships with your customers. By understanding their motivations, pain points, and preferences, you can create a more seamless and personalized experience that drives sales, increases customer loyalty, and sets your business up for long-term success. So, are you ready to chart a course towards a data-driven future?
Let’s get started!
General Inquiries
What if I don’t have a large budget for analytics tools?
Many free or affordable tools exist, such as Google Analytics. Start with the basics and gradually add more sophisticated tools as your needs and budget grow.
How do I ensure data privacy when collecting customer information?
Prioritize transparency. Clearly explain to customers how you collect and use their data. Comply with relevant data privacy regulations (like GDPR or CCPA) and use anonymization techniques where possible.
What are some common mistakes businesses make with customer behavior analytics?
Focusing solely on vanity metrics (like website visits) instead of key performance indicators (KPIs) like conversion rates. Another common mistake is failing to act on the insights gained from data analysis.