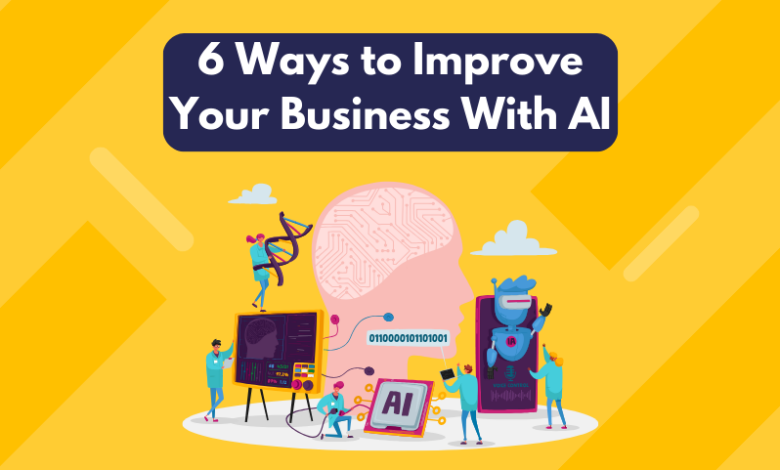
Revolutionize Your Business with AI-Powered Intelligent Operations
Revolutionize your business with ai powered intelligent operations – Revolutionize your business with AI-powered intelligent operations! It’s no longer a futuristic fantasy; it’s a tangible reality transforming how businesses operate and compete. Imagine a world where data analysis is automated, predictions are proactive, and efficiency soars to unprecedented levels. This isn’t science fiction; this is the power of AI intelligently integrated into your core business functions. We’ll explore how to harness this power, identifying key areas for implementation, choosing the right tools, and measuring the impact on your bottom line.
This journey will cover everything from understanding the core components of AI-powered intelligent operations to implementing and managing AI systems effectively. We’ll delve into specific examples, practical strategies, and future trends, equipping you with the knowledge to make informed decisions and successfully integrate AI into your business for maximum impact. Get ready to unlock a new era of productivity and profitability!
Understanding AI-Powered Intelligent Operations
AI-powered intelligent operations represent a fundamental shift in how businesses manage their processes. It’s about leveraging artificial intelligence to automate, optimize, and enhance various operational aspects, leading to significant improvements in efficiency, productivity, and overall performance. This isn’t just about adding AI to existing systems; it’s a holistic approach that integrates AI throughout the operational fabric of the organization.AI-powered intelligent operations are built upon several core components.
These include machine learning algorithms for predictive analytics and automation, robust data analytics platforms to process and interpret large datasets, advanced automation tools to streamline workflows, and a strong integration layer to connect disparate systems. Crucially, successful implementation also requires a clear understanding of business goals, a skilled workforce capable of managing and interpreting AI outputs, and a commitment to continuous improvement and adaptation.
Key Benefits of AI Integration in Business Operations
Integrating AI into business operations offers numerous advantages. Improved efficiency is a primary benefit, as AI can automate repetitive tasks, freeing up human employees to focus on more strategic initiatives. This automation also leads to reduced operational costs, as it minimizes manual labor and minimizes errors. Furthermore, AI enables data-driven decision-making, providing businesses with real-time insights and predictions to optimize resource allocation and improve strategic planning.
Enhanced customer experience is another key benefit; AI-powered chatbots and personalized recommendations can significantly improve customer satisfaction and loyalty. Finally, AI can significantly improve risk management by identifying and mitigating potential threats proactively.
Examples of Successful AI Implementations Across Industries
AI’s impact spans various sectors. In manufacturing, AI-powered predictive maintenance systems analyze sensor data from machinery to predict potential failures, minimizing downtime and maintenance costs. For example, General Electric uses AI to predict equipment failures in its jet engines, reducing maintenance costs and improving operational efficiency. In the financial services industry, AI algorithms are used for fraud detection, analyzing vast amounts of transaction data to identify suspicious activity in real-time.
Companies like PayPal leverage AI to detect and prevent fraudulent transactions, protecting both businesses and consumers. In healthcare, AI is assisting in diagnostics, analyzing medical images to identify diseases earlier and more accurately. For instance, Google’s DeepMind has developed AI algorithms that can detect eye diseases with a high degree of accuracy, aiding ophthalmologists in early diagnosis and treatment.
Comparison of Traditional Operations with AI-Enhanced Operations
Feature | Traditional Operations | AI-Enhanced Operations |
---|---|---|
Decision-Making | Primarily based on historical data and human intuition; often slow and reactive. | Data-driven, leveraging real-time insights and predictive analytics; proactive and adaptive. |
Automation | Limited automation, mostly for simple, repetitive tasks. | Extensive automation of complex tasks, including decision-making processes. |
Efficiency | Lower efficiency due to manual processes and human error. | Higher efficiency due to automation and optimization; reduced waste and errors. |
Cost | Higher operational costs due to manual labor and potential errors. | Lower operational costs due to automation and improved efficiency. |
Identifying Areas for AI Implementation
Integrating AI into your business processes can dramatically improve efficiency and profitability. However, successful implementation requires careful consideration of which areas will yield the greatest return on investment. Choosing the right processes is crucial for maximizing the benefits of AI and avoiding costly mistakes. This section will explore three key areas ripe for AI integration.
AI in Customer Service
Many businesses struggle with high customer service costs and inconsistent service quality. Long wait times, difficulty resolving complex issues, and a lack of personalized interactions are common challenges. AI can significantly alleviate these problems. AI-powered chatbots can handle routine inquiries, freeing up human agents to focus on more complex issues. Machine learning algorithms can analyze customer data to predict potential problems and proactively address them, leading to improved customer satisfaction and reduced operational costs.
For example, a telecommunications company could use AI to automatically detect and resolve billing errors, preventing frustrated customers from calling support. This leads to reduced call volume and improved customer retention.
AI in Supply Chain Optimization
Supply chain management is inherently complex, involving numerous moving parts and potential points of failure. Predicting demand, optimizing inventory levels, and ensuring timely delivery are constant challenges. AI can help by analyzing vast amounts of data to forecast demand more accurately, optimizing logistics routes, and identifying potential disruptions. Machine learning models can detect patterns and anomalies that might indicate a potential supply chain bottleneck, allowing businesses to proactively adjust their strategies and avoid costly delays.
For instance, a retail company could use AI to predict seasonal demand fluctuations, ensuring sufficient inventory without overstocking. This improves inventory management and reduces storage costs.
AI in Sales Forecasting and Lead Scoring
Accurate sales forecasting is crucial for effective resource allocation and strategic planning. Traditional methods often rely on historical data and expert intuition, which can be inaccurate and prone to bias. AI can improve sales forecasting by analyzing a wider range of data, including market trends, competitor activity, and social media sentiment. Similarly, AI-powered lead scoring systems can identify high-potential leads more effectively than manual methods, improving sales conversion rates.
A software company, for example, could use AI to analyze customer behavior and identify patterns that indicate a high likelihood of conversion, allowing sales teams to prioritize their efforts on the most promising leads. This leads to increased sales efficiency and improved ROI on marketing efforts.
AI Integration into Customer Service: A Flowchart
This flowchart illustrates the integration of AI into a customer service process:[Diagram description: The flowchart begins with a “Customer Inquiry” box. This leads to a decision box: “Is the inquiry routine?”. If yes, the flow goes to “AI Chatbot Handles Inquiry”. If no, it goes to “Human Agent Handles Inquiry”. Both paths lead to a “Customer Issue Resolved” box, which then leads to a “Data Collection and Analysis” box.
This data is then fed back into the AI system to improve its performance, completing the loop.]
Choosing the Right AI Tools and Technologies
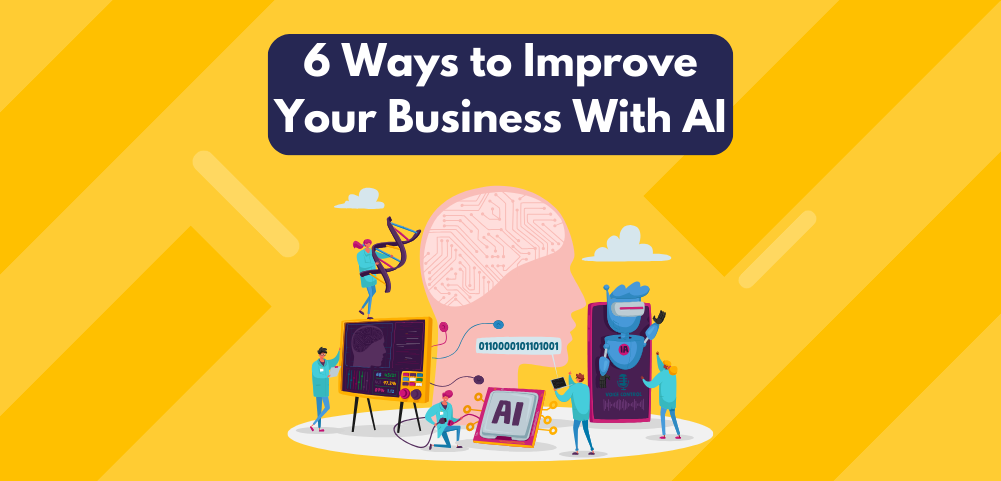
The journey to AI-powered intelligent operations isn’t just about adopting AI; it’s about selecting the right tools and technologies to meet your specific business needs. A poorly chosen AI solution can lead to wasted resources and underwhelming results. Understanding the nuances of different AI technologies and carefully evaluating vendors is crucial for success.Different AI technologies offer unique capabilities, and choosing the right one depends heavily on the problem you’re trying to solve.
Comparison of AI Technologies for Business Operations
Machine learning (ML), deep learning (DL), and natural language processing (NLP) are three prominent AI technologies frequently used in business. ML algorithms learn from data to make predictions or decisions, while DL, a subset of ML, uses artificial neural networks with multiple layers to analyze complex data patterns. NLP focuses on enabling computers to understand, interpret, and generate human language.For instance, a company aiming to predict customer churn might use ML algorithms trained on historical customer data (purchase history, engagement metrics, etc.).
If the company wants to analyze customer feedback from surveys or social media, NLP would be a more appropriate choice. Image recognition for quality control in a manufacturing process might leverage the power of deep learning to identify subtle defects. Each technology offers distinct advantages, making careful consideration vital.
Factors to Consider When Selecting AI Tools
Selecting the right AI tools involves evaluating several critical factors beyond just the core technology. Scalability, cost, and integration capabilities are paramount.Scalability refers to the ability of the AI solution to handle increasing amounts of data and processing demands as your business grows. A solution that works well today might become a bottleneck tomorrow if it lacks scalability. Cost considerations include not only the initial investment in software and hardware but also ongoing maintenance, training, and potential cloud computing expenses.
Seamless integration with existing business systems is essential to avoid disrupting workflows and ensure data compatibility. A poorly integrated AI system can create more problems than it solves.
Criteria for Evaluating AI Vendors
Choosing the right vendor is as crucial as choosing the right technology. A structured evaluation process ensures you select a partner that aligns with your business goals and provides reliable support.
- Vendor Experience and Expertise: Look for vendors with a proven track record of successful AI implementations in similar industries. Review case studies and testimonials to assess their capabilities.
- Solution Scalability and Flexibility: Ensure the solution can handle your current needs and scale to accommodate future growth. Consider whether the vendor offers flexible deployment options (cloud, on-premise, hybrid).
- Data Security and Privacy: Verify the vendor’s commitment to data security and compliance with relevant regulations (e.g., GDPR, CCPA). Understand their data handling practices and security measures.
- Integration Capabilities: Assess the vendor’s ability to integrate the AI solution with your existing systems and workflows. Check for API availability and documentation.
- Cost and Return on Investment (ROI): Obtain a detailed cost breakdown and develop a clear ROI projection based on the vendor’s claims and your business objectives. Consider factors like implementation costs, ongoing maintenance, and potential cost savings.
- Support and Maintenance: Inquire about the level of support and maintenance offered by the vendor. A responsive and knowledgeable support team is crucial for addressing issues and ensuring the smooth operation of the AI solution.
Implementing and Managing AI in Your Business: Revolutionize Your Business With Ai Powered Intelligent Operations

Successfully integrating AI into your business requires a strategic and phased approach. It’s not simply about purchasing software; it’s about transforming workflows, retraining staff, and establishing robust management systems. This process demands careful planning, meticulous execution, and ongoing monitoring to ensure optimal performance and return on investment.Implementing an AI system involves more than just installing software; it requires a holistic approach that considers your business’s unique needs and challenges.
A successful implementation is dependent on a well-defined strategy, proper resource allocation, and a commitment to continuous improvement. This process should be iterative, allowing for adjustments based on real-world performance data.
AI System Implementation Steps
A structured approach to implementation minimizes disruption and maximizes the chances of success. Each stage requires careful consideration and planning.
- Define Clear Objectives: Begin by identifying specific business problems AI can solve. This could involve automating repetitive tasks, improving customer service, or enhancing predictive analytics. For example, a retail company might aim to improve inventory management by predicting demand more accurately, while a bank might use AI to detect fraudulent transactions.
- Data Preparation and Assessment: AI systems thrive on data. Assess the quality, quantity, and accessibility of your existing data. Data cleaning, transformation, and feature engineering are often crucial steps. Insufficient or poor-quality data will significantly hamper AI performance. For example, a manufacturing company might need to standardize its sensor data from different machines before using it to predict equipment failure.
- AI Model Selection and Development: Choose the appropriate AI model based on your objectives and data. This might involve machine learning, deep learning, or natural language processing. Consider whether to build a custom model or utilize pre-trained models. A finance company might use a pre-trained model for sentiment analysis of customer reviews, while a healthcare provider might develop a custom model for medical image analysis.
- System Integration and Testing: Integrate the AI system into your existing IT infrastructure. Thorough testing is crucial to identify and rectify bugs or performance issues before full deployment. This could involve A/B testing different models or deploying the system in a limited capacity initially.
- Deployment and Monitoring: Deploy the AI system gradually, monitoring its performance closely. Continuous monitoring allows for adjustments and improvements based on real-world data. For instance, a logistics company might monitor the AI-powered route optimization system to identify and address any unexpected delays or inefficiencies.
Managing and Maintaining AI Systems
Effective management of AI systems is crucial for long-term success. This includes addressing data security and ethical considerations.
Robust data security measures are paramount. AI systems often handle sensitive data, requiring encryption, access controls, and regular security audits. Ethical considerations, such as bias in algorithms and data privacy, must be proactively addressed. Regular model retraining and updates are necessary to maintain accuracy and performance. For example, a social media platform needs to regularly update its AI algorithms to combat the spread of misinformation and hate speech.
Training Employees on AI Tools
Effective employee training is essential for successful AI adoption.
A phased approach to training is recommended. Begin with introductory sessions covering the basics of AI and its applications within the business. Follow this with hands-on training using the specific AI tools implemented. Provide ongoing support and resources to address employee questions and challenges. For example, a marketing team might receive training on using AI-powered tools for social media advertising and content creation.
Measuring the Impact of AI
Successfully integrating AI into your business operations isn’t just about implementation; it’s about demonstrable results. Measuring the impact of your AI initiatives is crucial for justifying continued investment, identifying areas for improvement, and showcasing the value proposition to stakeholders. This involves carefully selecting key performance indicators (KPIs) and developing robust methods for analyzing the data they generate.Understanding the return on investment (ROI) of AI is paramount.
While immediate results aren’t always guaranteed, a well-defined measurement strategy can illuminate the long-term benefits and demonstrate the value of AI-driven improvements. By tracking specific metrics, you can quantify the positive impact on efficiency, productivity, and ultimately, profitability.
Key Performance Indicators for AI Success
Tracking the right KPIs is essential for understanding the effectiveness of your AI implementation. These metrics should align with your overall business objectives and the specific goals of your AI initiatives. For example, if your AI is designed to improve customer service, relevant KPIs might include customer satisfaction scores, resolution times, and the volume of inquiries handled. For AI used in manufacturing, KPIs could focus on production efficiency, defect rates, and downtime.
Analyzing Data to Measure ROI
Calculating the ROI of AI involves comparing the costs of implementation (including software, hardware, personnel, and training) against the benefits realized. These benefits can be both tangible (e.g., increased revenue, reduced operational costs) and intangible (e.g., improved customer experience, enhanced decision-making). A simple ROI calculation is:
(Benefits – Costs) / Costs100%
. However, accurately assessing intangible benefits often requires more sophisticated analysis, such as comparing pre- and post-implementation performance data across relevant KPIs. For instance, a company might analyze the reduction in customer service call times after implementing an AI-powered chatbot, quantifying the cost savings from reduced labor hours.
Visual Representation of Projected Growth and Efficiency Gains
Imagine a graph charting two lines: one representing projected business growth without AI integration (a relatively steady, moderate incline), and another representing projected growthwith* AI integration (a steeper, more rapid incline). The difference between these lines visually represents the additional growth attributable to AI. Similarly, a bar chart could compare key metrics like operational costs or customer service response times before and after AI implementation.
For example, a bar representing “Average Customer Service Response Time” might show a significant decrease from 5 minutes pre-AI to 1 minute post-AI, vividly illustrating the efficiency gains. Another bar could show a dramatic reduction in operational costs due to automated processes, perhaps from $100,000 per month to $70,000, showcasing a $30,000 monthly cost savings. These visualizations provide a clear and compelling picture of the positive impact of AI on your business.
Future Trends in AI for Business Operations
The rapid advancement of artificial intelligence is poised to dramatically reshape business operations in the years to come. We’re moving beyond basic automation and into a realm of truly intelligent systems capable of complex decision-making, predictive analysis, and proactive problem-solving. This evolution will impact every sector, from manufacturing and logistics to healthcare and finance, demanding adaptation and innovation from businesses of all sizes.The integration of AI is no longer a futuristic concept; it’s a current reality with far-reaching consequences.
Emerging trends suggest an even more profound transformation is on the horizon, driven by advancements in processing power, data availability, and algorithm sophistication.
Generative AI and Enhanced Creativity
Generative AI, capable of creating new content such as text, images, and code, is rapidly maturing. This technology is transforming marketing and product development by automating content creation, personalizing customer experiences, and accelerating the design process. For instance, companies are using generative AI to create unique marketing materials tailored to individual customer segments, leading to increased engagement and conversion rates.
In product design, generative AI can explore numerous design options far beyond human capacity, optimizing for factors like weight, strength, and cost. This significantly reduces development time and enhances product innovation.
Hyperautomation and the Rise of Intelligent Workflows
Hyperautomation, the combination of AI, machine learning, and robotic process automation (RPA), is streamlining complex business processes. This technology goes beyond simple task automation, orchestrating entire workflows and adapting to changing conditions. For example, in supply chain management, hyperautomation can optimize inventory levels, predict disruptions, and automatically adjust logistics in real-time, minimizing delays and costs. In customer service, it can handle routine inquiries, escalate complex issues to human agents, and learn from past interactions to improve efficiency and customer satisfaction.
The result is a more agile and responsive business capable of reacting swiftly to market changes.
AI-Driven Predictive Maintenance and Risk Management
Predictive maintenance, using AI to anticipate equipment failures, is becoming increasingly prevalent across industries. By analyzing sensor data and historical maintenance records, AI algorithms can identify potential problems before they occur, minimizing downtime and preventing costly repairs. This is particularly impactful in manufacturing, where unexpected equipment failures can halt production lines. Similarly, AI is enhancing risk management by analyzing vast datasets to identify potential threats and opportunities, allowing businesses to make more informed decisions and mitigate risks proactively.
Financial institutions, for example, use AI to detect fraudulent transactions and assess credit risk more accurately.
Explainable AI (XAI) and Building Trust
As AI systems become more complex, the need for transparency and explainability increases. Explainable AI (XAI) aims to make AI decision-making more understandable to humans, building trust and fostering adoption. This is crucial for industries where regulatory compliance or ethical considerations are paramount, such as healthcare and finance. XAI techniques allow businesses to understandwhy* an AI system made a particular decision, enabling them to identify biases, debug errors, and ensure responsible AI deployment.
For example, in loan applications, XAI can explain why an application was approved or rejected, providing transparency and fairness.
Edge AI and Decentralized Intelligence, Revolutionize your business with ai powered intelligent operations
Edge AI, which processes data closer to the source (e.g., on devices like smartphones or sensors), is gaining traction. This reduces latency, enhances privacy, and enables real-time decision-making in environments with limited connectivity. Applications include autonomous vehicles, smart factories, and real-time fraud detection. For instance, a smart factory using edge AI can analyze sensor data from machines on the factory floor to immediately identify and address production issues, optimizing efficiency and reducing waste.
Wrap-Up
Embracing AI-powered intelligent operations isn’t just about adopting new technology; it’s about fundamentally reshaping your business strategy for a future where data-driven insights are the key to success. By carefully considering the areas ripe for AI implementation, selecting the right tools, and meticulously tracking your progress, you can unlock significant efficiency gains, reduce costs, and gain a competitive edge.
The future of business is intelligent, and this guide has equipped you with the knowledge to lead the charge.
Essential FAQs
What are the potential risks of implementing AI?
Risks include high initial investment costs, the need for skilled personnel, potential data security breaches, and ethical considerations around algorithmic bias.
How long does it typically take to see a return on investment (ROI) from AI?
The ROI timeframe varies significantly depending on the complexity of the implementation and the specific business goals. Some businesses see returns within months, while others may take years.
What if my company lacks the internal expertise to implement AI?
Many external consultants and vendors specialize in AI implementation and can provide the necessary expertise and support. Consider outsourcing specific tasks or engaging a full-service provider.
How can I ensure the ethical use of AI in my business?
Prioritize transparency, fairness, and accountability in your AI systems. Regularly audit your algorithms for bias and ensure compliance with relevant regulations and ethical guidelines.