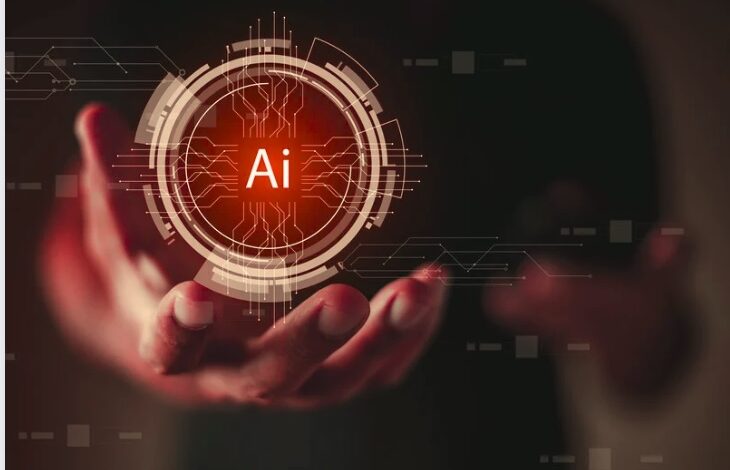
Responsible AI Balancing Innovation and Ethics
Responsible ai balancing business innovations with ethical considerations – Responsible AI: balancing business innovations with ethical considerations is no longer a futuristic concept; it’s the urgent reality shaping the technological landscape. We’re at a crossroads, where the potential for incredible advancements clashes with the potential for significant harm. This exploration dives into the critical need for ethical frameworks in AI development, examining the complexities of balancing rapid innovation with the safeguarding of human values and societal well-being.
We’ll explore the challenges businesses face, the biases inherent in algorithms, and the crucial role of transparency and accountability in building trust in this powerful technology.
From navigating the ethical dilemmas of data privacy to mitigating algorithmic bias, we’ll unpack the practical steps companies can take to integrate ethical AI into their core strategies. We’ll look at real-world examples of both responsible and irresponsible AI applications, learning from successes and failures alike. The goal? To paint a clearer picture of how we can harness the power of AI for good, ensuring a future where technology serves humanity, rather than the other way around.
Defining Responsible AI
Responsible AI is more than just building AI systems that work; it’s about building systems that work
- ethically* and
- beneficially* for society. It’s about proactively mitigating potential harms and ensuring fairness, transparency, and accountability throughout the entire AI lifecycle, from conception to deployment and beyond. This involves a careful consideration of the societal impact and potential biases embedded within algorithms and data.
The core principles of responsible AI development and deployment revolve around several key areas. These include fairness (avoiding bias and discrimination), transparency (understanding how AI systems make decisions), accountability (establishing mechanisms for addressing errors and harms), privacy (protecting sensitive data), robustness and safety (ensuring reliable and secure systems), and human oversight (maintaining human control and intervention). These principles are interconnected and interdependent; neglecting one can compromise the others.
Ethical Frameworks for AI
Different ethical frameworks offer varying perspectives on how to navigate the complex ethical challenges posed by AI. Utilitarianism, for instance, focuses on maximizing overall well-being, suggesting that AI should be designed to produce the greatest good for the greatest number of people. Deontology, on the other hand, emphasizes adherence to moral rules and duties, irrespective of the consequences.
Virtue ethics focuses on cultivating moral character, suggesting that AI developers should strive to be virtuous in their design choices. Finally, rights-based ethics emphasizes the inherent rights of individuals and seeks to protect those rights from AI-driven harms. The choice of ethical framework influences the specific guidelines and principles adopted for responsible AI development. For example, a utilitarian approach might prioritize maximizing efficiency even if it means some individuals experience minor inconveniences, while a rights-based approach would prioritize individual rights even if it compromises overall efficiency.
Examples of Responsible AI
Several real-world applications demonstrate responsible AI practices. For instance, some healthcare AI systems utilize federated learning techniques, allowing hospitals to collaboratively train models on patient data without directly sharing sensitive information, thus prioritizing patient privacy. Similarly, AI-powered loan applications often incorporate fairness mitigation strategies to avoid discriminatory outcomes based on race, gender, or other protected characteristics. These examples showcase how responsible AI can balance innovation with ethical considerations.
Another example is the use of explainable AI (XAI) techniques in medical diagnosis. XAI allows doctors to understand the reasoning behind an AI’s diagnosis, fostering trust and enabling human oversight.
Responsible AI development means carefully weighing the potential for groundbreaking business innovations against the ethical implications. This careful balance is crucial, especially as we see rapid advancements in application development; for example, check out this insightful piece on domino app dev the low code and pro code future which highlights how efficient development can impact AI implementation.
Ultimately, building ethical AI requires a thoughtful approach at every stage, from initial concept to final deployment.
Consequences of Irresponsible AI Development
Consider a hypothetical scenario: a facial recognition system deployed by law enforcement is trained primarily on images of one demographic group, leading to significantly higher rates of misidentification for other groups. This could result in wrongful arrests, increased surveillance of marginalized communities, and erosion of public trust in law enforcement. The lack of transparency and accountability in the development and deployment of this system exacerbates the problem, making it difficult to identify and rectify the biases embedded within the algorithm.
This scenario highlights the severe consequences of failing to prioritize responsible AI development, underscoring the need for rigorous ethical review and oversight throughout the AI lifecycle. The reputational damage to the organization deploying such a system, along with potential legal ramifications and social unrest, further emphasizes the importance of ethical considerations in AI development.
Balancing Innovation and Ethics in AI
The rapid advancement of artificial intelligence presents an unprecedented opportunity to revolutionize industries and improve lives. However, this breakneck speed also creates a significant tension: the need for ethical considerations often lags behind technological innovation. Businesses are grappling with the challenge of integrating ethical AI practices into their core strategies, a task made complex by the inherent conflicts between maximizing profit and adhering to responsible AI principles.
This necessitates a careful balancing act, demanding a proactive and integrated approach to ethical AI throughout the entire development lifecycle.The inherent tension between innovation and ethics in AI stems from the pressure to be first to market with new AI-powered products and services. This pressure often overshadows the crucial need for thorough ethical assessments and mitigation strategies. The allure of significant financial gains can easily outweigh the potential long-term risks associated with deploying ethically questionable AI systems.
This creates a situation where the pursuit of profit can inadvertently lead to the development and deployment of AI systems that perpetuate biases, discriminate against certain groups, or even cause harm.
Challenges in Integrating Ethical AI Practices
Integrating ethical AI practices into innovation strategies presents several significant hurdles for businesses. One major challenge is the lack of clear, universally accepted ethical guidelines and standards for AI development. This ambiguity makes it difficult for businesses to navigate the ethical landscape and to confidently implement responsible AI practices. Another key challenge lies in the difficulty of translating abstract ethical principles into concrete, actionable steps.
Furthermore, the rapid pace of technological advancement often outstrips the ability of businesses to develop and implement robust ethical frameworks, leading to a constant struggle to keep pace. Finally, the lack of skilled professionals with expertise in both AI and ethics further complicates the integration process. Companies often struggle to find individuals who can effectively bridge the gap between technical development and ethical considerations.
Conflicts Between Profit Maximization and Responsible AI
The pursuit of profit often creates direct conflicts with the principles of responsible AI. For example, a company might be tempted to use biased data to train its AI system if it leads to higher accuracy in a specific task, even if that accuracy comes at the expense of fairness and equity. Similarly, a company might choose to prioritize speed of development over thorough ethical review, potentially leading to the deployment of an AI system with unforeseen negative consequences.
The short-term gains from prioritizing profit over ethics can easily outweigh the long-term risks, especially in a competitive market environment. This highlights the need for strong internal governance structures and ethical oversight to ensure that profit maximization does not come at the cost of responsible AI practices.
A Framework for Prioritizing Ethical Considerations
To effectively prioritize ethical considerations during the AI development lifecycle, a structured framework is crucial. This framework should integrate ethical considerations at each stage, from initial concept and design to deployment and ongoing monitoring. It should include:
A clearly defined set of ethical principles and guidelines specific to the organization and its AI applications.
Regular ethical reviews at each stage of the development process, involving diverse perspectives and expertise.
Mechanisms for identifying, assessing, and mitigating potential risks and harms associated with the AI system.
Transparency and accountability mechanisms to ensure that the AI system’s decisions and actions are understandable and justifiable.
Ongoing monitoring and evaluation of the AI system’s performance and impact, with a focus on ethical considerations.
This framework necessitates a shift in organizational culture, prioritizing ethical considerations as an integral part of the innovation process, not as an afterthought. Only then can businesses truly balance the pursuit of innovation with the imperative of responsible AI development.
Bias and Fairness in AI Systems: Responsible Ai Balancing Business Innovations With Ethical Considerations
AI systems, while incredibly powerful tools for innovation, are susceptible to inheriting and amplifying biases present in the data they are trained on. This can lead to unfair or discriminatory outcomes, impacting various groups differently and undermining the very principles of equitable technology. Understanding how bias manifests and developing strategies to mitigate it is crucial for building responsible and ethical AI systems.
Bias Manifestation and Impact
Bias in AI algorithms can stem from various sources, including biased data collection, skewed data representation, and flawed algorithm design. For example, if a facial recognition system is primarily trained on images of individuals with lighter skin tones, it may perform poorly when identifying individuals with darker skin tones, leading to misidentification and potentially harmful consequences in law enforcement or security applications.
Similarly, algorithms used in loan applications might inadvertently discriminate against certain demographic groups if the training data reflects historical biases in lending practices. The impact of these biases can be far-reaching, perpetuating existing inequalities and creating new ones. This can lead to unfair access to opportunities, services, and resources, exacerbating societal divisions.
Detecting and Mitigating Bias
Detecting and mitigating bias requires a multifaceted approach, starting with the data collection phase. Careful attention should be paid to ensuring data representativeness and diversity. This includes actively seeking out and including data from underrepresented groups. Techniques like data augmentation, where synthetic data is generated to balance class representation, can be employed. During model training, techniques like fairness-aware algorithms, which explicitly incorporate fairness constraints, can help mitigate bias.
Regular auditing and monitoring of AI systems’ performance across different demographic groups are also essential to identify and address emerging biases. Transparency in the data and algorithms used is critical for accountability and building trust.
Examples of Biased AI Systems
Several real-world examples highlight the consequences of biased AI systems. One infamous case involves a recidivism prediction tool used in the US criminal justice system. This tool was found to be biased against African American defendants, leading to disproportionately harsher sentencing recommendations. Another example is in the field of hiring, where AI-powered recruitment tools have been shown to discriminate against women and minority candidates due to biases in the training data.
These examples demonstrate the tangible and harmful consequences of deploying biased AI systems without proper scrutiny and mitigation strategies.
Ensuring Fairness and Equity in AI Decision-Making
A robust procedure for ensuring fairness and equity in AI decision-making requires a comprehensive approach. This begins with establishing clear fairness criteria aligned with ethical guidelines and legal frameworks. This should include defining metrics for measuring fairness and establishing thresholds for acceptable levels of bias. Data collection and preprocessing should prioritize representativeness and address potential sources of bias.
Model selection and training should incorporate fairness-aware techniques and rigorous testing for bias. Finally, ongoing monitoring and auditing are essential to detect and address any emerging biases. Regular reviews of the AI system’s impact and continuous improvement based on feedback and data analysis are crucial to maintaining fairness and equity over time.
Bias Mitigation Techniques
Technique | Description | Advantages | Disadvantages |
---|---|---|---|
Data Augmentation | Generating synthetic data to balance class representation. | Improves data diversity, reduces bias. | Requires careful design to avoid introducing new biases. Can be computationally expensive. |
Re-weighting | Adjusting the weights of data points during training to account for class imbalances. | Simple to implement. | May not fully address complex biases. |
Fairness-Aware Algorithms | Algorithms designed to explicitly incorporate fairness constraints. | Directly addresses fairness concerns. | Can be complex to implement and may require trade-offs with accuracy. |
Adversarial Debiasing | Training a separate model to detect and counteract bias. | Can effectively mitigate bias. | Requires significant computational resources. |
Transparency and Explainability in AI
Building trust in AI systems is paramount for their widespread adoption and acceptance. Without understanding how an AI system arrives at its conclusions, users are less likely to rely on its outputs, especially in high-stakes situations like healthcare or finance. Transparency and explainability are crucial components in bridging this trust gap, ensuring accountability and allowing for effective oversight.Transparency in AI refers to the openness and clarity surrounding the data, algorithms, and decision-making processes involved.
Explainability, on the other hand, focuses on making the reasoning behind AI outputs understandable to both technical and non-technical audiences. These two concepts are closely intertwined, working together to foster confidence and responsible use.
Techniques for Enhancing AI Explainability
Several techniques aim to make the inner workings of AI models more accessible. These range from simple methods suitable for simpler models to more complex approaches needed for intricate systems like deep learning networks. The choice of technique depends heavily on the complexity of the AI system and the desired level of detail in the explanation.
- Feature Importance Analysis: This technique identifies the input features that most significantly influence the AI’s output. For example, in a loan application prediction model, feature importance might reveal that credit score and income are the most influential factors. Visualizations such as bar charts can effectively communicate these findings.
- Local Interpretable Model-agnostic Explanations (LIME): LIME approximates the behavior of a complex model locally around a specific prediction. It creates a simpler, more interpretable model that explains the prediction for that individual instance. Imagine a model predicting customer churn; LIME might explain why a specific customer is predicted to churn by focusing on their recent activity and interactions.
- SHapley Additive exPlanations (SHAP): SHAP values provide a game-theoretic approach to explaining individual predictions. They quantify the contribution of each feature to the model’s output, allowing for a comprehensive understanding of the factors influencing a specific decision. For instance, in a medical diagnosis model, SHAP values could show the relative importance of different symptoms in the diagnosis.
- Rule Extraction: This method extracts decision rules from the model, making the reasoning process more explicit. These rules can be presented in a human-readable format, such as “IF credit score > 700 AND income > $50,000 THEN approve loan.” This is particularly useful for simpler models like decision trees.
Challenges in Achieving Transparency in Complex AI Models
While the pursuit of transparency is crucial, significant challenges exist, particularly with complex models like deep learning networks. These models often involve millions or even billions of parameters, making it difficult to trace the exact path from input to output.
- Model Complexity: The sheer number of parameters and layers in deep learning models makes it extremely challenging to understand their internal workings. Tracing the influence of individual input features on the final output can be computationally expensive and practically infeasible.
- Black Box Nature: Deep learning models are often referred to as “black boxes” due to their opacity. The internal processes are not readily interpretable, making it difficult to understand why a specific decision was made.
- Data Dependence: The performance and explainability of AI models are heavily reliant on the quality and characteristics of the training data. Biases or inconsistencies in the data can lead to unpredictable and difficult-to-explain outputs.
Step-by-Step Guide for Documenting and Explaining an AI System
Effective documentation is essential for building trust and ensuring accountability. A clear explanation of the AI system’s workings to stakeholders—including technical and non-technical audiences—is crucial.
- Define the Purpose and Scope: Clearly articulate the AI system’s intended purpose, the types of decisions it makes, and the target audience for the explanation.
- Describe the Data: Detail the sources, collection methods, preprocessing steps, and any potential biases present in the training data. Include statistics about the data’s size and characteristics.
- Explain the Model: Describe the chosen model architecture, its parameters, and its training process. Use clear and concise language, avoiding technical jargon where possible. If appropriate, include visualizations of the model’s structure.
- Illustrate the Decision-Making Process: Provide concrete examples of how the AI system arrives at its conclusions. Use case studies or illustrative examples to show how the input data is processed and how the output is generated.
- Address Limitations and Biases: Acknowledge any limitations of the AI system, including potential biases or inaccuracies. Discuss how these limitations are being addressed or mitigated.
- Provide Contact Information: Include contact information for individuals or teams responsible for the AI system, enabling stakeholders to ask questions or report concerns.
Privacy and Data Security in AI
The rise of artificial intelligence presents incredible opportunities for innovation, but it also raises significant ethical concerns, particularly regarding the privacy and security of personal data. AI systems often rely on vast quantities of data to learn and function effectively, and much of this data is inherently personal, encompassing sensitive information about individuals. This dependence creates a complex interplay between technological advancement and the fundamental right to privacy, demanding careful consideration and robust safeguards.The ethical implications of collecting and using personal data for AI development are multifaceted.
Gathering and processing personal data without informed consent is a clear violation of privacy rights. Moreover, the potential for misuse, discrimination, and unintended consequences from biased or improperly secured data is substantial. The very nature of AI, with its ability to learn patterns and make predictions, can inadvertently expose sensitive details or create new vulnerabilities if data is not handled responsibly.
For instance, seemingly anonymized data can be re-identified through clever techniques, leading to privacy breaches. Furthermore, the use of AI in surveillance technologies raises profound ethical questions about the balance between security and individual liberties.
Ethical Implications of Data Collection and Use
The ethical implications stem from several key areas. First, the potential for discriminatory outcomes arises when training data reflects existing societal biases. For example, an AI system trained on a dataset predominantly featuring images of white faces might perform poorly in identifying individuals with darker skin tones, leading to unfair or inaccurate results. Second, the lack of transparency in how AI systems use personal data can erode trust and create a sense of powerlessness for individuals.
Users often have little understanding of how their data is being collected, processed, and used, making it difficult to assess the risks involved. Third, the potential for data breaches and security vulnerabilities poses a significant threat. AI systems often store and process sensitive personal information, making them attractive targets for cyberattacks. A breach could expose individuals to identity theft, financial loss, and other serious harms.
Finally, the potential for misuse of data by governments or corporations raises concerns about surveillance and control. AI-powered surveillance systems can track individuals’ movements and activities, potentially violating their privacy and freedom.
Methods for Ensuring Privacy and Security
Several methods can help ensure the privacy and security of sensitive data used in AI systems. Data minimization is crucial; only collect and process the data absolutely necessary for the AI system’s function. Strong encryption techniques should be employed to protect data both in transit and at rest. Access control mechanisms should be implemented to limit who can access sensitive data, adhering to the principle of least privilege.
Regular security audits and penetration testing can help identify and address vulnerabilities. Furthermore, robust incident response plans are essential to mitigate the impact of potential data breaches. These plans should Artikel clear procedures for containing breaches, notifying affected individuals, and recovering from the incident. The adoption of privacy-enhancing technologies (PETs) such as differential privacy and federated learning allows AI models to be trained on decentralized datasets without directly accessing or storing sensitive personal information.
Data Anonymization and Differential Privacy
Data anonymization techniques aim to remove or obscure identifying information from datasets, making it difficult to link data points back to specific individuals. However, complete anonymization is often difficult to achieve, and re-identification attacks can sometimes succeed. Differential privacy, on the other hand, adds carefully calibrated noise to the data during the analysis process, making it statistically difficult to infer individual-level information while preserving the overall statistical properties of the data.
This approach provides a stronger guarantee of privacy than simple anonymization. For example, instead of directly revealing the exact number of individuals with a specific characteristic, differential privacy would provide a range or an approximate count, introducing a small degree of uncertainty that prevents the precise identification of individuals.
Data Governance Framework
A comprehensive data governance framework is essential for responsible handling of personal data in AI applications. This framework should include clear policies and procedures for data collection, storage, processing, and disposal. It should also define roles and responsibilities for data management and security. Crucially, it must incorporate mechanisms for ensuring compliance with relevant privacy regulations, such as GDPR or CCPA.
Regular audits and assessments are necessary to verify adherence to the framework and identify areas for improvement. The framework should also establish a process for handling data subject requests, such as requests for access, correction, or deletion of personal data. Transparency is key; individuals should be informed about how their data is being used and have the ability to exercise their rights.
Finally, a robust mechanism for handling data breaches is critical, ensuring prompt notification and remediation.
Accountability and Oversight in AI
The rapid advancement of artificial intelligence necessitates robust mechanisms for accountability and oversight. Without them, the potential benefits of AI are overshadowed by the risks of misuse, bias, and unintended harm. Establishing clear lines of responsibility and effective oversight mechanisms is crucial for building public trust and ensuring the ethical development and deployment of AI systems.Accountability in AI development and deployment requires a multi-faceted approach, encompassing technical, legal, and ethical considerations.
This means identifying who is responsible when an AI system malfunctions or causes harm, and ensuring that appropriate consequences follow. This is particularly challenging in complex systems where multiple actors are involved in design, development, and deployment.
Mechanisms for Holding Developers and Deployers Accountable
Holding developers and deployers accountable requires a combination of legal frameworks, industry standards, and internal corporate policies. Legal frameworks can establish liability for AI-related harms, while industry standards can provide guidelines for ethical AI development. Internal policies can define roles and responsibilities within organizations, clarifying who is accountable for specific aspects of AI system development and deployment. Furthermore, robust auditing and testing procedures can help identify and mitigate potential risks before deployment.
Transparency in the development process is also crucial, allowing for independent scrutiny and accountability.
The Role of Regulatory Bodies and Ethical Review Boards
Regulatory bodies play a critical role in overseeing the development and deployment of AI. They can establish standards, conduct audits, and enforce regulations to ensure AI systems are developed and used responsibly. Ethical review boards, similar to those used in medical research, can provide independent assessments of the ethical implications of AI projects before they are deployed. These boards can offer valuable insights into potential biases, risks, and unintended consequences, promoting a more ethical and responsible approach to AI development.
The specific roles and powers of these bodies vary across jurisdictions, but their overarching goal is to protect individuals and society from the potential harms of AI.
Challenges in Establishing Clear Lines of Responsibility
Establishing clear lines of responsibility in complex AI systems presents significant challenges. The opacity of some AI algorithms, particularly deep learning models, makes it difficult to understand how they arrive at their decisions. This “black box” problem makes it challenging to determine who is responsible when something goes wrong. Furthermore, AI systems often involve multiple actors – developers, deployers, users – making it difficult to assign responsibility for specific outcomes.
The distributed nature of AI development and deployment, involving global supply chains and open-source components, further complicates the establishment of clear lines of accountability.
Case Study: An Ethical Violation in AI-Powered Loan Applications
A fintech company, “LoanAI,” used an AI system to assess loan applications. The system, trained on historical data, consistently denied loans to applicants from certain zip codes, disproportionately affecting minority communities. An investigation revealed that the training data reflected existing societal biases, leading to discriminatory outcomes. LoanAI was found to have insufficient oversight mechanisms in place to detect and mitigate this bias. The company faced regulatory fines, reputational damage, and legal action from affected applicants. This case highlights the need for robust testing, bias detection, and ongoing monitoring of AI systems to prevent such ethical violations. LoanAI’s failure to address potential biases in its training data, coupled with a lack of adequate oversight, demonstrates the critical need for greater accountability in AI development. The subsequent regulatory actions and legal challenges underscore the potential consequences of neglecting ethical considerations in AI.
The Future of Responsible AI
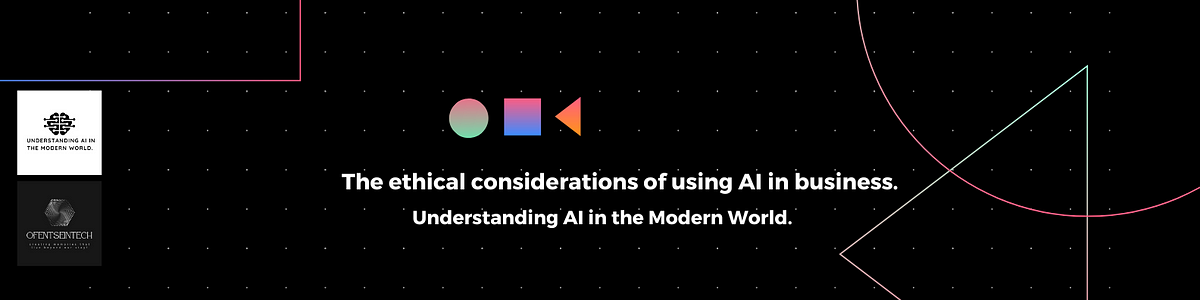
The field of artificial intelligence is rapidly evolving, bringing with it both incredible opportunities and significant ethical challenges. Responsible AI, the development and deployment of AI systems that are ethical, fair, transparent, and accountable, is no longer a niche concern but a crucial imperative for navigating this technological frontier. The future of responsible AI will be shaped by emerging trends, evolving regulations, and ongoing research efforts aimed at mitigating the risks and maximizing the benefits of this powerful technology.
Emerging Trends and Challenges in Responsible AI, Responsible ai balancing business innovations with ethical considerations
Several key trends are shaping the future landscape of responsible AI. One significant trend is the increasing focus on explainable AI (XAI), driven by the need for transparency and accountability in AI decision-making. This involves developing methods to understand how AI systems arrive at their conclusions, allowing for better scrutiny and debugging. A related challenge is ensuring fairness and mitigating bias in AI systems, which requires ongoing research into bias detection and mitigation techniques.
The increasing power and complexity of AI models also pose challenges, requiring new approaches to risk assessment and management. Furthermore, the growing reliance on AI in critical sectors like healthcare and finance necessitates the development of robust safety and security measures to prevent unintended consequences.
Predictions about the Future of AI Governance and Regulation
We can anticipate a significant increase in AI-specific regulations and governance frameworks globally. These will likely focus on areas such as data privacy, algorithmic transparency, and accountability for AI-driven decisions. Similar to the evolution of data privacy regulations like GDPR, we can expect a convergence of national and international standards to establish a more unified approach to AI governance.
For example, the EU’s AI Act is a significant step in this direction, establishing a risk-based classification system for AI systems and outlining specific requirements for high-risk applications. We might also see the emergence of industry-specific regulatory bodies focused on responsible AI practices within particular sectors. This proactive approach will be essential to fostering trust and ensuring the responsible development and deployment of AI technologies.
Key Areas Requiring Further Research and Development in Responsible AI
Several critical areas demand further research and development to ensure responsible AI. One key area is the development of robust methods for detecting and mitigating bias in training data and algorithms. This involves not only technical solutions but also a deeper understanding of the societal factors that contribute to bias. Another important area is the development of more effective explainable AI (XAI) techniques, allowing for better understanding and auditing of AI systems.
Research into AI safety and security is also crucial, focusing on the development of methods to prevent unintended consequences and ensure the robustness of AI systems against adversarial attacks. Finally, research into the ethical implications of advanced AI technologies, such as autonomous weapons systems, requires ongoing attention to ensure responsible innovation.
Potential Solutions for Addressing Ethical Dilemmas Posed by Advanced AI Technologies
Addressing the ethical dilemmas posed by advanced AI requires a multi-faceted approach. One crucial step is establishing clear ethical guidelines and principles for AI development and deployment. This requires collaboration between researchers, policymakers, and industry stakeholders to develop a shared understanding of responsible AI practices. Another important approach is promoting education and awareness about the ethical implications of AI, both within the technical community and among the broader public.
This will help foster a more informed and responsible approach to AI innovation. Furthermore, the development and implementation of robust auditing and oversight mechanisms are crucial for ensuring accountability and transparency in AI systems. This could involve independent audits of AI systems to assess their fairness, transparency, and safety. Finally, the creation of independent ethical review boards to evaluate the ethical implications of new AI technologies before their deployment is a crucial step in preventing harm and promoting responsible innovation.
Last Word
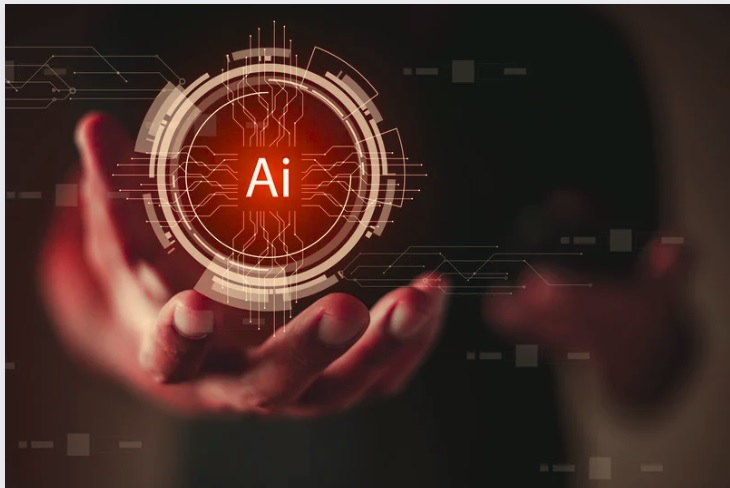
The journey toward responsible AI is a continuous one, demanding constant vigilance, adaptation, and a commitment to ethical principles. It’s not just about avoiding the pitfalls; it’s about proactively shaping a future where AI enhances human lives and contributes to a more just and equitable world. This requires collaboration between developers, policymakers, and the public to establish clear guidelines, robust regulatory frameworks, and a shared understanding of the ethical implications of this transformative technology.
The future of AI is not predetermined; it’s a future we collectively build, one ethical decision at a time.
Popular Questions
What are some common ethical concerns surrounding AI in healthcare?
Ethical concerns in AI healthcare include potential bias in diagnostic tools leading to disparities in care, privacy violations related to sensitive patient data, and the lack of transparency in AI-driven decision-making processes.
How can businesses demonstrate their commitment to responsible AI?
Businesses can demonstrate their commitment through transparent AI development practices, regular ethical audits, public reporting on AI impact, and investment in bias mitigation techniques.
What role do regulations play in promoting responsible AI?
Regulations provide a framework for accountability, setting standards for data privacy, algorithmic transparency, and bias mitigation. They help to level the playing field and encourage responsible AI practices across industries.
What is the difference between explainable AI (XAI) and transparent AI?
While both aim to increase trust, transparent AI focuses on making the entire AI system’s design and data understandable, while XAI focuses specifically on making individual AI decisions understandable.