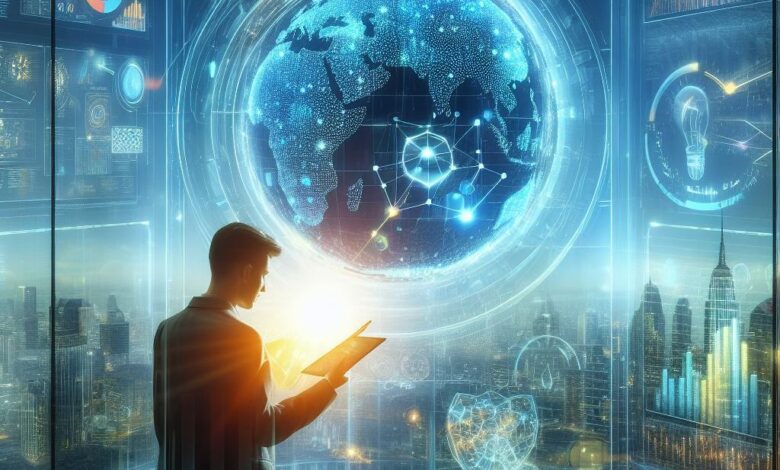
The AI Revolution Driving Enterprise Growth
The AI revolution in a modern enterprise driving competitive advantage and growth is no longer a futuristic fantasy; it’s the present reality reshaping how businesses operate and thrive. This isn’t just about adding a few AI tools; it’s a fundamental shift in strategy, demanding a holistic approach that integrates artificial intelligence into every facet of the organization, from marketing and sales to operations and strategic planning.
Prepare to explore how this transformative technology is empowering businesses to not just compete, but to dominate.
We’ll delve into the practical applications of AI across various industries, examining real-world examples of successful implementations and exploring the tangible benefits, such as enhanced efficiency, reduced costs, and data-driven decision-making. We’ll also address the potential challenges, including ethical considerations and the need for workforce adaptation, offering strategies to mitigate risks and ensure responsible AI integration. Get ready for a journey into the heart of the AI revolution and discover how your enterprise can harness its power for unprecedented growth.
Defining the AI Revolution in Modern Enterprises
The AI revolution isn’t just hype; it’s fundamentally reshaping how modern enterprises operate, compete, and grow. Driven by exponential advancements in computing power, data availability, and algorithmic sophistication, AI is no longer a futuristic concept but a tangible force impacting every facet of business. This transformation is characterized by the seamless integration of intelligent systems into core business processes, leading to significant efficiency gains and innovative solutions previously unimaginable.The current AI revolution is fueled by several key technological advancements.
Deep learning, a subset of machine learning, allows computers to learn from vast amounts of unstructured data, such as images, text, and audio, without explicit programming. This has led to breakthroughs in areas like image recognition, natural language processing, and speech synthesis. The rise of cloud computing provides the scalable infrastructure needed to train and deploy complex AI models, making AI accessible to even smaller businesses.
Furthermore, advancements in hardware, particularly specialized processors like GPUs and TPUs, significantly accelerate the training and execution of AI algorithms.
AI Applications Across Enterprise Departments
AI’s impact spans various departments, offering tailored solutions to optimize workflows and drive strategic advantage. In marketing, AI-powered tools analyze customer data to personalize campaigns, predict customer behavior, and optimize ad spending. Sales teams leverage AI for lead scoring, sales forecasting, and chatbots that provide instant customer support. Operations benefit from AI-driven predictive maintenance, optimizing supply chains, and automating repetitive tasks, improving efficiency and reducing costs.
Human Resources utilizes AI for talent acquisition, employee engagement analysis, and performance management. Finally, in finance, AI enhances fraud detection, risk management, and algorithmic trading.
Examples of Successful AI Implementations
Several industries showcase the transformative power of AI. In healthcare, AI algorithms analyze medical images for early disease detection, personalize treatment plans, and accelerate drug discovery. Manufacturing utilizes AI for predictive maintenance of equipment, optimizing production processes, and improving quality control. The financial services sector employs AI for fraud detection, risk assessment, and algorithmic trading, improving efficiency and mitigating risks.
Retailers leverage AI for personalized recommendations, inventory management, and customer service chatbots, enhancing customer experience and driving sales. For instance, Netflix uses AI to personalize movie recommendations, increasing user engagement and retention. Similarly, Amazon uses AI for optimizing its logistics and supply chain, leading to faster and more efficient delivery.
Competitive Advantage Through AI Adoption
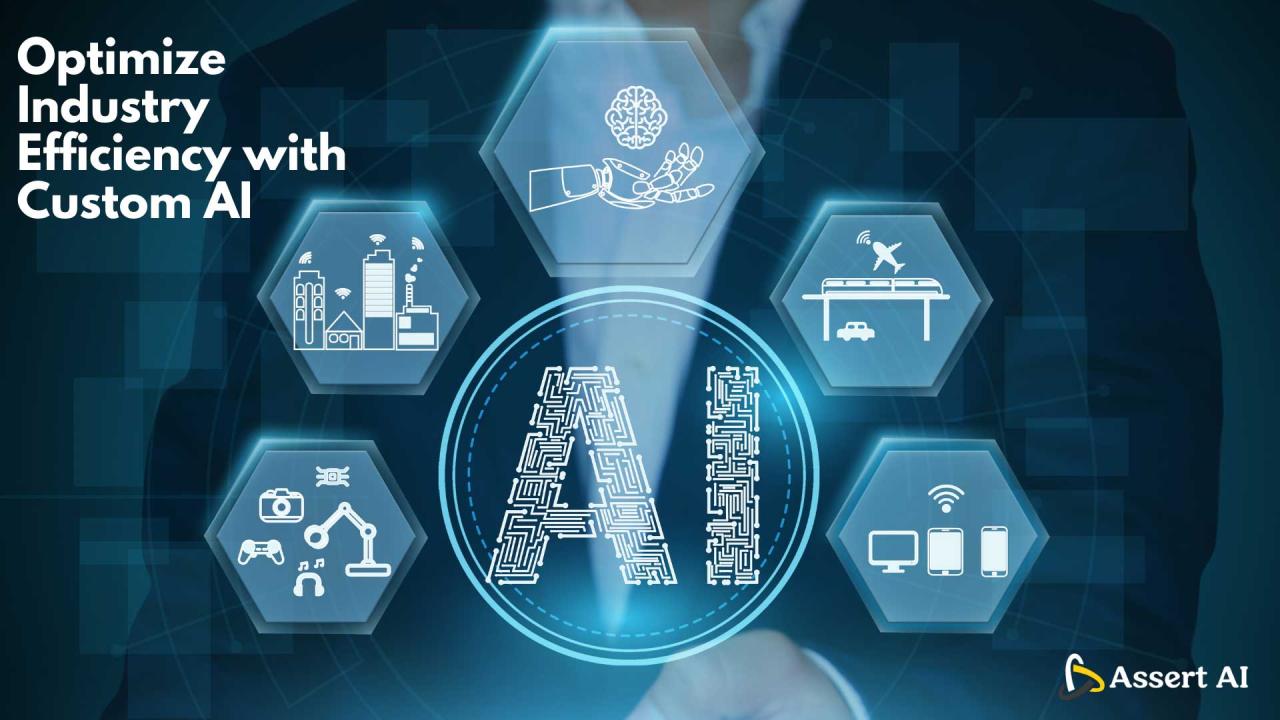
The integration of artificial intelligence (AI) is no longer a futuristic concept; it’s a critical factor determining success in today’s fiercely competitive business landscape. Companies that embrace AI strategically are transforming their operations, enhancing customer experiences, and ultimately, achieving a significant competitive edge over those lagging behind. This competitive advantage stems from a multitude of factors, ranging from increased efficiency to improved decision-making.AI significantly alters the competitive landscape.
Businesses with substantial AI integration operate with a level of agility and precision that’s difficult for their less-technologically advanced counterparts to match. They can adapt to market changes faster, personalize customer interactions more effectively, and unlock insights hidden within vast datasets – all leading to a more robust and resilient business model. Conversely, companies without significant AI integration often struggle to keep pace with these advancements, facing challenges in areas such as cost optimization, innovation, and customer satisfaction.
The AI revolution is reshaping modern enterprises, boosting efficiency and creating new revenue streams. To keep pace, businesses need agile development processes, which is where the power of low-code/no-code platforms comes in. Check out this insightful piece on domino app dev the low code and pro code future to see how it accelerates AI integration. Ultimately, embracing these tools is crucial for staying ahead in today’s competitive landscape and fueling sustainable growth.
AI-Driven Operational Efficiency and Cost Reduction
AI technologies automate repetitive tasks, streamline workflows, and optimize resource allocation, resulting in substantial cost savings and increased efficiency. For example, AI-powered robotic process automation (RPA) can handle mundane administrative tasks, freeing up human employees to focus on higher-value activities. Predictive maintenance, another AI application, uses sensor data to anticipate equipment failures, minimizing downtime and reducing maintenance costs. Furthermore, AI algorithms can optimize supply chains by predicting demand fluctuations and improving inventory management, leading to significant reductions in waste and storage costs.
The cumulative effect of these efficiencies translates to a leaner, more profitable operation.
AI’s Enhancement of Decision-Making and Strategic Planning
AI empowers businesses to make data-driven decisions with greater speed and accuracy. Advanced analytics tools leverage AI to identify trends, patterns, and anomalies within massive datasets, providing insights that would be impossible to uncover through manual analysis. This improved data visibility allows for more informed strategic planning, enabling companies to anticipate market shifts, identify emerging opportunities, and proactively adapt their strategies.
AI can also assist in risk management by identifying potential threats and vulnerabilities, allowing for timely mitigation strategies. The result is a more proactive and resilient approach to business management.
Hypothetical Case Study: AI in Retail
Imagine a large retail chain struggling with high inventory costs and inconsistent customer service. By implementing an AI-powered system, they can analyze historical sales data, weather patterns, and social media trends to predict demand more accurately. This allows for optimized inventory management, reducing storage costs and minimizing waste from unsold goods. Simultaneously, AI-powered chatbots provide 24/7 customer support, answering frequently asked questions and resolving simple issues, freeing up human staff to handle more complex customer inquiries.
This improved efficiency and customer satisfaction leads to increased sales and brand loyalty, giving the retail chain a clear competitive advantage over its rivals that haven’t adopted similar AI solutions. The result is a significant boost in profitability and market share.
AI-Driven Growth Strategies
AI is no longer a futuristic concept; it’s a powerful engine driving growth for modern enterprises. By strategically integrating AI into various business functions, companies can unlock new revenue streams, optimize operations, and gain a significant competitive edge. This section delves into how businesses can leverage AI to fuel substantial growth.
Key Performance Indicators (KPIs) for AI-Driven Growth
Measuring the success of AI initiatives requires focusing on specific KPIs that directly reflect their impact on business growth. These metrics should be aligned with overall business objectives and tracked consistently to assess the return on investment (ROI) of AI projects. Simply implementing AI isn’t enough; demonstrating its positive impact on the bottom line is crucial.
Examples of AI-Driven Growth
Several companies have successfully harnessed the power of AI to achieve remarkable growth. Netflix, for example, uses AI-powered recommendation engines to personalize user experiences, leading to increased engagement and subscription renewals. This targeted approach significantly contributes to their user retention and overall revenue growth. Similarly, Amazon leverages AI for efficient inventory management, optimized pricing strategies, and personalized marketing campaigns, all of which contribute to their market dominance and profitability.
These successes highlight the transformative potential of AI when implemented strategically.
Comparison of AI-Driven Growth Strategies
Strategy | Description | Outcome | Example |
---|---|---|---|
AI-Powered Personalization | Utilizing AI to tailor products, services, and marketing messages to individual customer preferences. | Increased customer engagement, higher conversion rates, improved customer lifetime value. | Netflix’s recommendation system. |
Predictive Analytics for Sales Forecasting | Employing AI to analyze historical data and predict future sales trends. | Improved inventory management, optimized resource allocation, reduced waste. | Walmart’s supply chain optimization. |
AI-Driven Customer Service Automation | Implementing AI-powered chatbots and virtual assistants to handle customer inquiries and support requests. | Reduced customer service costs, improved response times, enhanced customer satisfaction. | Many Banks and Telecom Companies |
AI-Enabled Process Automation | Automating repetitive tasks and processes using AI-powered robotic process automation (RPA). | Increased efficiency, reduced operational costs, improved accuracy. | Many Insurance and Finance Companies |
Implementing an AI-Driven Growth Strategy in the Retail Industry
A step-by-step plan for implementing an AI-driven growth strategy within the retail industry might look like this:
- Assess Current State: Conduct a thorough analysis of existing data, processes, and technologies to identify areas where AI can add value. This includes understanding customer behavior, inventory management, and supply chain operations.
- Define Clear Objectives: Establish specific, measurable, achievable, relevant, and time-bound (SMART) goals for AI initiatives. Examples include increasing online sales by 15% within six months or reducing customer service response time by 20% within one year.
- Select Appropriate AI Technologies: Choose AI solutions that align with identified objectives and available resources. This might involve implementing recommendation engines, predictive analytics tools, or chatbots.
- Data Integration and Preparation: Ensure that relevant data is collected, cleaned, and prepared for AI model training. This often requires integrating data from various sources and addressing data quality issues.
- Develop and Deploy AI Solutions: Develop and deploy chosen AI solutions, ensuring seamless integration with existing systems and processes. This may involve working with internal teams or external vendors.
- Monitor and Evaluate Performance: Track key performance indicators (KPIs) to monitor the effectiveness of AI initiatives. Regularly evaluate results and make necessary adjustments to optimize performance.
- Continuous Improvement: Continuously refine AI models and strategies based on performance data and evolving business needs. AI is an iterative process; continuous learning and improvement are essential.
Challenges and Risks of AI Implementation
Embracing the AI revolution presents numerous opportunities for modern enterprises, but it’s crucial to acknowledge the significant challenges and risks involved. A successful AI implementation requires careful planning, proactive risk management, and a commitment to ethical considerations. Ignoring these aspects can lead to costly failures, reputational damage, and even legal repercussions.The integration of AI systems into existing business processes isn’t simply a matter of plugging in new software.
It requires a holistic approach that addresses data infrastructure, workforce skills, and the potential for unforeseen consequences. This section will delve into the key challenges and risks, along with strategies for mitigating them.
Data Security and Privacy Concerns
AI systems are heavily reliant on data – often vast quantities of sensitive data. This creates significant vulnerabilities. Data breaches, unauthorized access, and misuse of personal information are serious risks. For example, a healthcare provider using AI for diagnosis could face severe penalties if patient data is compromised. Mitigating these risks requires robust cybersecurity measures, including data encryption, access control, and regular security audits.
Compliance with relevant data privacy regulations, such as GDPR and CCPA, is also paramount. Furthermore, implementing differential privacy techniques can help protect individual data points while still allowing for useful aggregate analysis.
Ethical Considerations in AI Development and Deployment
AI systems, particularly those using machine learning, can inherit and amplify biases present in their training data. This can lead to unfair or discriminatory outcomes. For example, an AI-powered recruitment tool trained on historical data might perpetuate gender or racial biases in hiring decisions. Addressing these ethical concerns requires careful selection and curation of training data, algorithmic transparency, and ongoing monitoring for bias.
Implementing explainable AI (XAI) techniques can help understand the decision-making processes of AI systems and identify potential biases. Furthermore, establishing ethical guidelines and principles for AI development and deployment is crucial.
Workforce Adaptation and Reskilling
The introduction of AI can lead to significant changes in job roles and responsibilities. Some jobs may be automated, while others will evolve to require new skills. This necessitates proactive workforce planning, including reskilling and upskilling initiatives. For example, a manufacturing company implementing robotic process automation needs to train its employees on how to work alongside and manage these robots.
Investing in employee training programs, providing opportunities for career transitions, and fostering a culture of continuous learning are vital for a smooth transition.
Risks of Over-Reliance on AI Systems
Over-dependence on AI systems can lead to a decline in human oversight and critical thinking. This can be particularly problematic in situations requiring nuanced judgment or ethical decision-making. For instance, relying solely on an AI-powered fraud detection system without human review could lead to false positives, impacting legitimate transactions. Maintaining a balance between AI augmentation and human expertise is crucial.
Regular audits of AI system performance, establishing clear protocols for human intervention, and fostering a culture of questioning AI-driven recommendations are essential safeguards.
Strategies for Mitigating Challenges and Risks
Effective risk mitigation requires a multi-faceted approach. This includes establishing clear AI governance structures, implementing robust data security measures, fostering a culture of ethical AI development, and investing in workforce training. Regular risk assessments, scenario planning, and continuous monitoring are also crucial for identifying and addressing emerging challenges. Collaboration with external stakeholders, such as regulators and ethical experts, can provide valuable insights and guidance.
Transparency in AI development and deployment is also vital for building trust and accountability.
Best Practices for Responsible AI Implementation
Implementing AI responsibly requires a commitment to ethical principles and best practices. Here are some key considerations:
- Prioritize data privacy and security throughout the AI lifecycle.
- Establish clear ethical guidelines and principles for AI development and deployment.
- Use diverse and representative datasets to mitigate bias.
- Implement explainable AI (XAI) techniques to enhance transparency and accountability.
- Invest in workforce training and reskilling programs.
- Maintain human oversight and critical thinking in AI-driven decision-making.
- Conduct regular audits of AI system performance and identify potential risks.
- Foster collaboration and communication with stakeholders.
- Promote transparency and accountability in AI development and deployment.
- Continuously monitor and adapt to evolving ethical and regulatory landscapes.
The Future of AI in Enterprise: The Ai Revolution In A Modern Enterprise Driving Competitive Advantage And Growth
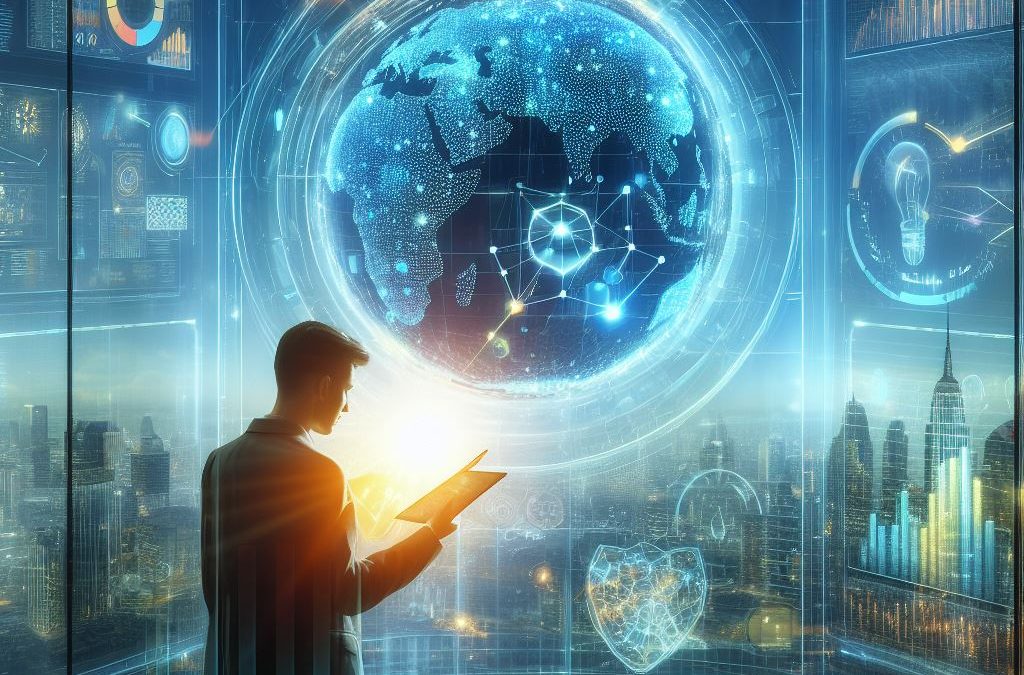
The rapid advancements in artificial intelligence are poised to dramatically reshape the business landscape over the next decade. We’re moving beyond the initial stages of AI adoption, where it served primarily as a tool for automation, towards a future where AI becomes deeply integrated into every facet of enterprise operations, driving unprecedented levels of efficiency, innovation, and competitive advantage.
This integration will be characterized by a shift from narrow AI applications to more sophisticated, generalized AI systems capable of handling complex, nuanced tasks.AI’s impact on businesses will be multifaceted, affecting everything from product development and customer service to risk management and strategic decision-making. We can expect to see a significant increase in the adoption of AI-powered tools and platforms, leading to a more data-driven and intelligent enterprise.
This transition will require significant investment in infrastructure, talent, and data management capabilities.
Generative AI and its Enterprise Applications
Generative AI, capable of creating new content like text, images, and code, will become increasingly prevalent in enterprise settings. Imagine marketing departments leveraging AI to generate personalized advertising campaigns tailored to individual customer preferences, or software development teams using AI to accelerate the coding process and reduce errors. The increased efficiency and creative potential unlocked by generative AI will significantly boost productivity and innovation across various industries.
For example, companies like Jasper and Copy.ai are already demonstrating the power of generative AI in content creation, allowing marketing teams to produce high-quality content much faster. This is just the beginning; we can anticipate even more sophisticated applications emerging in the coming years.
The Rise of Explainable AI (XAI)
As AI systems become more complex, the need for transparency and explainability will become paramount. Explainable AI (XAI) focuses on developing AI models whose decision-making processes are easily understandable by humans. This is crucial for building trust, ensuring accountability, and mitigating potential biases in AI-driven systems. Without XAI, the widespread adoption of complex AI systems within critical business functions would be hindered due to concerns about potential errors and lack of oversight.
The financial industry, for instance, will increasingly rely on XAI to ensure regulatory compliance and build confidence in AI-powered trading algorithms.
Hyperautomation and the Intelligent Enterprise
The future enterprise will be characterized by hyperautomation, where AI seamlessly integrates with robotic process automation (RPA) and other technologies to automate entire business processes. This will lead to significant improvements in efficiency, reduced operational costs, and enhanced productivity. Imagine a supply chain optimized by AI-powered predictive analytics, anticipating disruptions and automatically adjusting logistics to maintain optimal flow. This level of intelligent automation will transform industries like manufacturing, logistics, and retail, creating more agile and responsive businesses.
A Visual Representation of AI’s Evolution in Enterprise
Imagine a graphic depicting three distinct stages of AI adoption. The first stage, representing the present, shows isolated AI applications within specific departments (e.g., a chatbot in customer service, a predictive model in marketing). The second stage, representing the near future (next 3-5 years), shows interconnected AI systems working collaboratively across departments, sharing data and insights. The final stage, representing the future (5-10 years), depicts a fully integrated, intelligent enterprise where AI permeates all aspects of the business, from strategic planning to operational execution, creating a self-learning and adaptive organization.
The visual progression emphasizes the increasing interconnectedness and sophistication of AI systems within the enterprise over time.
Case Studies
AI’s transformative potential isn’t just theoretical; numerous companies have successfully integrated AI into their operations, achieving significant improvements in efficiency, profitability, and customer satisfaction. Examining these real-world examples provides valuable insights into effective AI implementation strategies and the tangible benefits they deliver. The following case studies illustrate the diverse applications and impactful results of AI across different industries.
Netflix’s AI-Powered Recommendation System, The ai revolution in a modern enterprise driving competitive advantage and growth
Netflix’s success is significantly attributed to its sophisticated recommendation engine. This system analyzes vast amounts of user data, including viewing history, ratings, and search queries, to predict which shows and movies individual users are most likely to enjoy. This personalized experience drastically reduces user churn and increases engagement.
- Strategy: Leveraging machine learning algorithms, specifically collaborative filtering and content-based filtering, to analyze user data and generate personalized recommendations.
- Results: A significant increase in user engagement and viewing time, leading to higher subscription retention rates and increased revenue. Estimates suggest that the recommendation system accounts for a substantial portion of Netflix’s overall revenue.
- Lessons Learned: The importance of high-quality data, continuous model improvement through A/B testing, and the ability to adapt the system to evolving user preferences are crucial for long-term success.
Amazon’s AI-Driven Supply Chain Optimization
Amazon’s vast and complex supply chain relies heavily on AI to optimize logistics, predict demand, and manage inventory. Their AI systems analyze sales data, weather patterns, and various other factors to forecast demand accurately, enabling them to efficiently manage inventory levels and reduce waste.
- Strategy: Utilizing machine learning models to predict demand, optimize warehouse operations, and route shipments efficiently. This includes using robotics and automation in their fulfillment centers.
- Results: Significant reductions in inventory costs, faster delivery times, and improved overall supply chain efficiency. These optimizations contribute directly to Amazon’s profitability and competitive advantage.
- Lessons Learned: The integration of AI requires significant investment in infrastructure and data management. Successful implementation necessitates a collaborative approach across different departments and a commitment to continuous improvement.
Siemens’ AI for Predictive Maintenance
Siemens uses AI to predict equipment failures in industrial settings, enabling proactive maintenance and preventing costly downtime. Their system analyzes sensor data from various machines to identify patterns that indicate potential malfunctions before they occur.
- Strategy: Employing machine learning algorithms to analyze sensor data from industrial equipment, identifying patterns indicative of potential failures and predicting their likelihood.
- Results: Significant reduction in unplanned downtime, improved equipment lifespan, and lower maintenance costs. The predictive maintenance system allows Siemens to optimize maintenance schedules and allocate resources more effectively.
- Lessons Learned: Data quality is paramount. Accurate and reliable sensor data is crucial for the effectiveness of the predictive maintenance system. Furthermore, successful implementation requires close collaboration between data scientists and domain experts.
Last Recap
The AI revolution isn’t just a trend; it’s a paradigm shift. Embracing AI isn’t optional for modern enterprises seeking sustainable growth and a competitive edge. By strategically integrating AI, businesses can unlock unprecedented opportunities, optimize operations, and ultimately, transform their futures. The journey might present challenges, but the rewards – increased efficiency, data-driven insights, and accelerated growth – far outweigh the risks.
The future belongs to those who dare to embrace the power of AI.
Questions and Answers
What are the biggest barriers to AI adoption in enterprises?
High implementation costs, lack of skilled personnel, data integration challenges, and concerns about data security and ethical implications are common barriers.
How can I measure the ROI of AI investments?
Track key performance indicators (KPIs) relevant to your business goals, such as improved efficiency, reduced costs, increased sales, and enhanced customer satisfaction. Compare these metrics before and after AI implementation.
Is AI replacing human jobs?
While AI automates some tasks, it also creates new roles and opportunities. The focus should be on reskilling and upskilling the workforce to work alongside AI, leveraging its capabilities to enhance human potential.
What are some ethical considerations when implementing AI?
Ensure fairness and avoid bias in algorithms, protect user privacy and data security, and be transparent about how AI systems are used to make decisions.