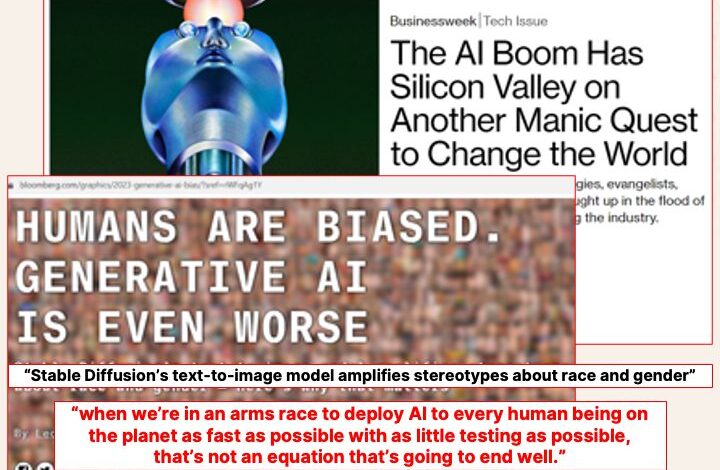
The Risks of Sharing Business Data with AI
The risks of sharing business data with AI are far-reaching and often underestimated. While AI offers incredible potential for streamlining operations and gaining insights, blindly handing over sensitive information can lead to devastating consequences. From data breaches that expose customer details and intellectual property to the insidious creep of algorithmic bias and the complexities of regulatory compliance, the journey into AI-powered business intelligence is paved with potential pitfalls.
Understanding these risks is crucial for navigating this exciting, yet treacherous, technological landscape.
This post delves into the key concerns surrounding data sharing with AI, exploring the potential vulnerabilities, outlining mitigation strategies, and offering practical advice to help businesses harness the power of AI while safeguarding their most valuable assets. We’ll examine everything from data breaches and intellectual property theft to the ethical dilemmas posed by algorithmic bias and the legal minefield of data privacy regulations.
Data Breaches and Leaks
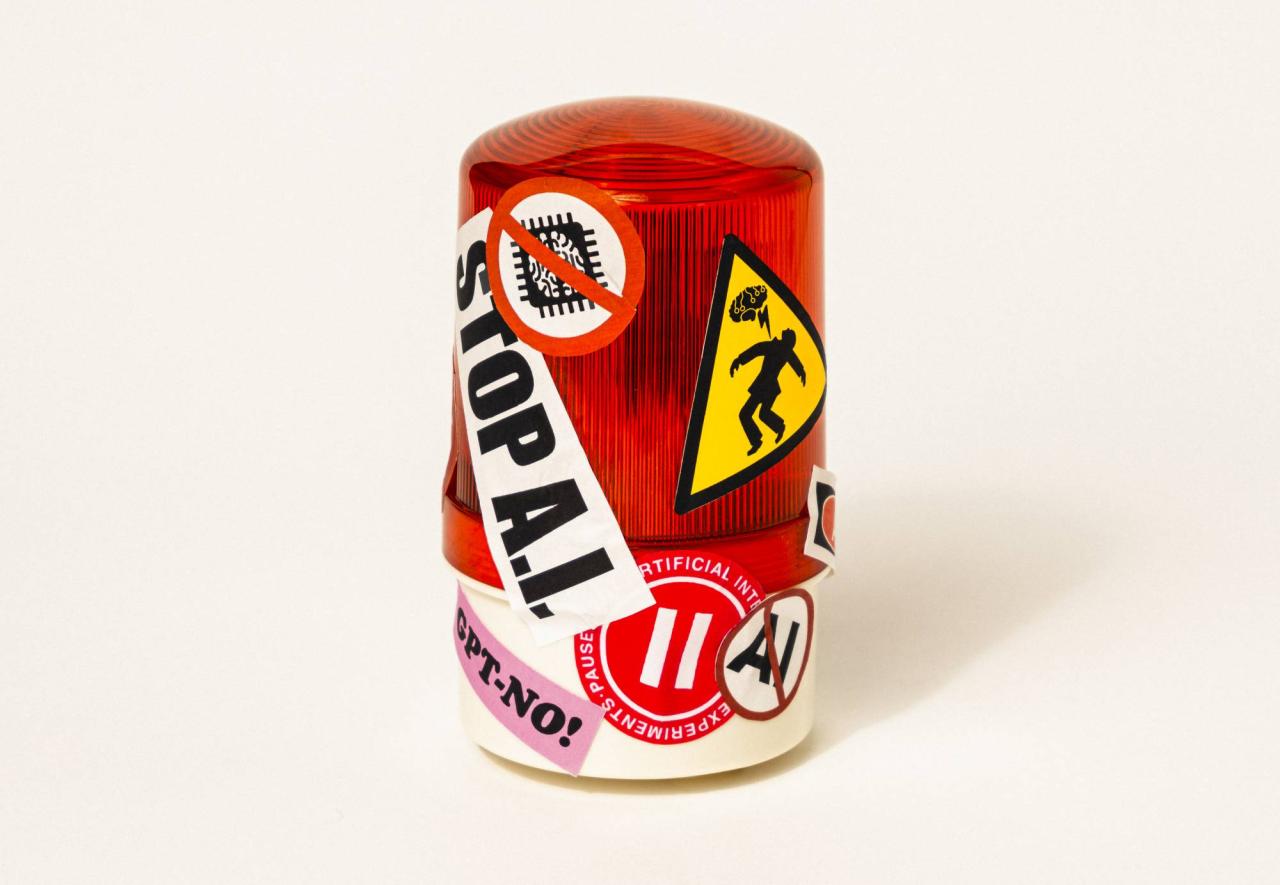
Sharing your business data with AI systems, while offering incredible potential, introduces significant risks. One of the most critical concerns is the increased vulnerability to data breaches and leaks. The interconnected nature of AI systems and the vast amounts of data they process create numerous potential pathways for malicious actors to gain unauthorized access.The potential pathways for data breaches when sharing business data with AI systems are multifaceted.
Data breaches can occur at any point in the data lifecycle – from the initial upload to the AI system, during processing and analysis, or even during the storage of results. Vulnerabilities in the AI system itself, insecure APIs, insufficient data encryption, and human error in managing access controls are all contributing factors. Furthermore, the use of cloud-based AI solutions introduces additional security challenges related to third-party access and potential vulnerabilities within the cloud provider’s infrastructure.
Weak security protocols within the AI platform, inadequate employee training, and phishing attacks targeting employees with access to the system are other significant risks.
Consequences of Data Breaches
A data breach resulting from AI system use can have devastating consequences for a business. Financially, the costs can be astronomical, encompassing expenses related to incident response, legal fees, regulatory fines, credit monitoring for affected customers, and potential loss of business. Reputational damage can be equally severe, leading to loss of customer trust, damage to brand image, and difficulty attracting investors.
The legal repercussions can be substantial, including potential lawsuits from affected customers and regulatory investigations resulting in hefty fines and penalties under laws like GDPR or CCPA.
Examples of Real-World Data Breaches Involving AI Systems
While specific details of data breaches directly attributable to AI system vulnerabilities are often kept confidential for competitive and legal reasons, numerous examples highlight the risks. Consider the case of a hypothetical large retailer using an AI-powered system to analyze customer purchase data. A successful cyberattack targeting the retailer’s cloud storage, where AI processing occurred, could expose sensitive customer information like names, addresses, payment details, and purchase history.
The impact would include significant financial losses from compensating customers, legal action, and regulatory fines. Furthermore, the retailer’s reputation would suffer, potentially leading to long-term loss of market share. Another example might involve a healthcare provider using AI for diagnostics. A breach here could expose sensitive patient medical records, leading to significant reputational damage, legal repercussions, and even endangerment of patients.
So, you’re thinking about using AI, but worried about the security implications of sharing your business data? It’s a valid concern! Building secure, custom applications is key, and that’s where exploring options like domino app dev the low code and pro code future becomes really important. Ultimately, controlling your data and how it’s handled, even within AI systems, is paramount to mitigating those risks.
Hypothetical Data Breach Scenario and Mitigation Strategies
Imagine a financial institution using an AI-powered system to detect fraudulent transactions. A sophisticated attacker gains access to the AI system’s training data, which includes sensitive financial information. The attacker modifies the training data, subtly altering the AI’s fraud detection algorithms. The result is that the AI now fails to detect fraudulent transactions initiated by the attacker, leading to significant financial losses for the institution.To mitigate this risk, the institution should have implemented robust security measures, including:
- Data encryption both in transit and at rest.
- Regular security audits and penetration testing of the AI system.
- Strict access control measures, including multi-factor authentication.
- Employee training on security best practices and awareness of phishing attempts.
- A comprehensive incident response plan to effectively handle data breaches.
- Regular monitoring of the AI system’s performance and logs for suspicious activity.
- Data loss prevention (DLP) tools to prevent sensitive data from leaving the organization’s control.
Intellectual Property Theft
The integration of AI into business operations, while offering immense potential, introduces significant vulnerabilities concerning intellectual property (IP). The very nature of AI, relying on vast datasets for training and processing, creates opportunities for malicious actors to access and exploit sensitive business information, potentially leading to the theft of trade secrets and proprietary algorithms. Understanding these vulnerabilities and implementing robust protective measures is crucial for maintaining a competitive edge.AI systems, particularly those utilizing machine learning, learn patterns and relationships from the data they are trained on.
If this data includes sensitive IP, such as source code, design specifications, or customer data, there’s a risk that the AI model itself could inadvertently reveal or leak this information. Furthermore, the very act of uploading sensitive data to a cloud-based AI platform introduces vulnerabilities to external attacks and data breaches.
Vulnerabilities of Intellectual Property in AI Data Processing
The vulnerabilities stem from several interconnected factors. Firstly, the reliance on external cloud-based AI services means data is often transmitted and stored outside of a company’s direct control. This introduces risks associated with data security breaches and unauthorized access. Secondly, the “black box” nature of some AI algorithms makes it difficult to audit and verify that the model isn’t inadvertently revealing sensitive information.
Finally, the potential for adversarial attacks, where malicious actors manipulate the input data to extract sensitive information or cause the AI to behave in unintended ways, presents a significant threat. For example, a competitor might subtly alter training data to subtly bias an AI model’s output, revealing valuable information about a company’s strategies.
Examples of AI-Enabled Intellectual Property Theft
Imagine a scenario where a company uses an AI-powered system to optimize its manufacturing processes. The AI is trained on data that includes proprietary algorithms and design specifications. If a security breach occurs, a competitor could gain access to this data and reverse-engineer the company’s processes, significantly undermining its competitive advantage. Similarly, a company using AI for natural language processing might inadvertently leak sensitive information about its product development plans if the training data includes internal communications or documents.
A sophisticated attacker could even craft malicious prompts to elicit sensitive information from the AI model.
Methods for Protecting Intellectual Property in AI Systems
Protecting IP in the context of AI requires a multi-layered approach. This includes implementing robust access controls, data encryption, and regular security audits. Differential privacy techniques can be used to add noise to the training data, making it more difficult to extract sensitive information. Homomorphic encryption allows computations to be performed on encrypted data without decryption, further enhancing security.
Furthermore, carefully selecting and vetting AI service providers is crucial. Companies should choose providers with strong security track records and transparent data handling practices. Finally, employing robust watermarking techniques can help identify the source of leaked intellectual property.
Comparison of Data Encryption Techniques
Several encryption techniques can be used to protect sensitive data within AI systems. Symmetric encryption, like AES, uses the same key for encryption and decryption, offering high speed but requiring secure key exchange. Asymmetric encryption, such as RSA, uses separate keys for encryption and decryption, providing better security for key management but being slower. Homomorphic encryption, as mentioned earlier, allows computation on encrypted data without decryption, offering a unique level of security.
The choice of encryption technique depends on the specific needs and security requirements of the application. For instance, AES might be suitable for encrypting large datasets used for training, while RSA could be used for securing communication channels.
Vendor Lock-in and Dependence: The Risks Of Sharing Business Data With Ai
Choosing an AI vendor for data processing can feel like a leap of faith. The promise of streamlined operations and insightful analytics is enticing, but the potential for vendor lock-in is a significant risk that many businesses overlook. Becoming overly reliant on a single provider can severely restrict your flexibility and potentially inflate your costs in the long run.
This section explores the dangers of vendor lock-in and offers strategies for mitigating this risk.The risks associated with becoming overly reliant on a specific AI vendor for data processing are substantial. Once your business processes are deeply integrated with a particular platform, switching vendors becomes a complex, costly, and time-consuming undertaking. This dependence can leave you vulnerable to price hikes, reduced service quality, or even vendor bankruptcy, leaving your critical data stranded.
Furthermore, your ability to innovate and adapt to changing market demands might be stifled by the limitations of a single vendor’s technology. This inflexibility can be a major competitive disadvantage.
Vendor Lock-in’s Impact on Flexibility and Costs
Vendor lock-in significantly impacts a business’s flexibility and budget. Imagine your entire data pipeline is built around a specific AI vendor’s software. If that vendor raises prices or changes its service offerings, you’re essentially stuck. Switching to a different provider requires migrating your data, retraining your staff, and potentially rewriting significant portions of your internal processes. This transition not only consumes valuable time and resources but also carries a high risk of disrupting operations and potentially leading to data loss.
The cost implications can be staggering, far exceeding the initial investment in the chosen AI solution. For example, a small business might find itself locked into a contract with escalating fees, hindering its growth and profitability.
Strategies for Mitigating Vendor Lock-in, The risks of sharing business data with ai
Several strategies can help businesses mitigate the risks of vendor lock-in. First, choose vendors that offer open APIs and data formats. This ensures that your data is not trapped within a proprietary system and can be easily transferred to other platforms if needed. Second, invest in building internal expertise in data management and AI technologies. This allows you to better understand the intricacies of your chosen solution and empowers you to make informed decisions regarding vendor selection and future migrations.
Third, regularly evaluate alternative solutions and maintain a clear understanding of the market landscape. This proactive approach allows for timely adjustments and prevents becoming overly reliant on a single vendor. Finally, negotiate contracts carefully, paying close attention to exit clauses and data ownership provisions. These clauses can significantly reduce the cost and complexity of switching vendors if necessary.
Comparison of AI Data Processing Solutions
The following table compares three hypothetical AI data processing solutions, highlighting their potential for vendor lock-in:
Solution | Openness/API Access | Data Portability | Contract Flexibility | Vendor Lock-in Risk |
---|---|---|---|---|
Solution A | Proprietary, limited API access | Low | Long-term, inflexible contract | High |
Solution B | Open APIs, standard data formats | High | Flexible contract terms, easy cancellation | Low |
Solution C | Partially open APIs, some proprietary elements | Medium | Moderate contract terms | Medium |
Algorithmic Bias and Discrimination
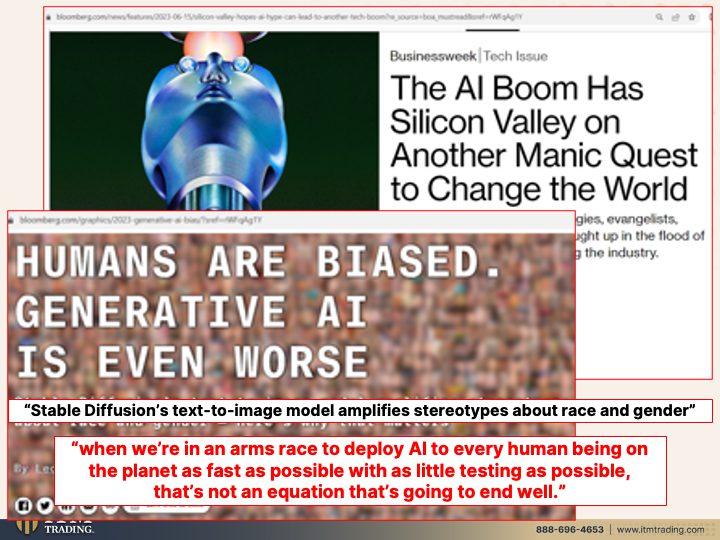
Feeding your business data to AI algorithms offers incredible potential, but it also carries significant risks. One of the most pressing concerns is the inherent possibility of algorithmic bias, leading to discriminatory outcomes that can severely damage your brand reputation and even lead to legal repercussions. Algorithmic bias isn’t intentional malice; it’s a reflection of the biases present in the data used to train the AI.The implications of biased algorithms for business decisions are far-reaching.
For example, a biased recruitment AI might unfairly screen out qualified candidates from underrepresented groups, resulting in a less diverse and potentially less innovative workforce. Similarly, a loan application algorithm trained on biased data could deny credit to individuals based on factors unrelated to their creditworthiness, leading to financial exclusion. These biases not only affect individuals but also negatively impact the overall performance and ethical standing of the business.
Sources of Algorithmic Bias
Biased algorithms stem from biased data. This data can reflect existing societal biases related to gender, race, age, socioeconomic status, and other factors. For instance, if a historical dataset used to train a loan approval algorithm contains a disproportionate number of loan defaults from a particular demographic, the algorithm might unfairly penalize applicants from that group even if their individual risk profile is low.
Furthermore, the very design of the algorithm itself, the features selected for analysis, and the choices made during the development process can introduce bias. A poorly designed algorithm might inadvertently amplify existing biases in the data or even create new ones.
Implications of Biased Algorithms for Business Decisions
The consequences of deploying biased AI systems are severe. Beyond reputational damage and potential legal challenges, biased algorithms can lead to inaccurate predictions, flawed business strategies, and ultimately, financial losses. For instance, a biased marketing algorithm might target advertisements inefficiently, leading to wasted marketing spend. A biased pricing algorithm might charge different prices to different customer segments based on discriminatory factors, leading to ethical concerns and potential legal repercussions.
The erosion of trust among customers and stakeholders is another critical consequence.
Detecting and Mitigating Algorithmic Bias
Detecting and mitigating algorithmic bias requires a multi-faceted approach. Regular audits of the data used to train AI systems are crucial. This involves carefully examining the data for imbalances and biases across different demographic groups. Techniques like fairness-aware machine learning can be employed to modify algorithms and ensure fairer outcomes. This involves incorporating fairness constraints into the algorithm’s training process or using techniques like re-weighting data samples to address imbalances.
Transparency in the development and deployment of AI systems is also vital, allowing for scrutiny and identification of potential biases. Moreover, diverse teams working on AI development can help identify and address biases that might otherwise go unnoticed.
Auditing AI Algorithms for Fairness
A comprehensive audit of AI algorithms to ensure fairness and prevent discriminatory outcomes should include several key steps. First, a thorough assessment of the data used to train the algorithm should be conducted, looking for biases in representation and potential discriminatory features. Second, the algorithm’s performance should be evaluated across different demographic groups to identify disparities in outcomes.
Third, the algorithm’s decision-making process should be analyzed to understand how it arrives at its conclusions and identify any potential sources of bias. Finally, appropriate mitigation strategies should be implemented, and the algorithm’s performance should be continuously monitored and re-evaluated to ensure ongoing fairness. This process should be documented thoroughly, providing transparency and accountability.
Lack of Transparency and Explainability
The increasing reliance on AI in business decision-making presents a significant challenge: understanding how these complex systems arrive at their conclusions. While AI can process vast amounts of data with incredible speed, the internal workings of many algorithms remain opaque, making it difficult to trace the reasoning behind their outputs. This lack of transparency creates significant risks, impacting both the reliability of business decisions and the trust placed in AI systems.Many AI systems, particularly deep learning models, operate as “black boxes.” Their intricate networks of interconnected nodes and weights process data in ways that are often impossible to fully decipher, even by their creators.
This lack of insight hinders our ability to identify and correct errors, biases, or unexpected behavior. Imagine an AI system used for loan applications inexplicably rejecting qualified applicants – without understanding its internal logic, identifying and rectifying the issue becomes extremely difficult.
Challenges in Understanding AI Processing
The complexity of AI algorithms, especially deep learning models with millions or even billions of parameters, makes it incredibly challenging to trace the decision-making process. Traditional debugging techniques are often insufficient. Furthermore, the sheer volume of data processed by these systems can overwhelm human comprehension. Even if we could understand the individual steps, the cumulative effect on the final output remains difficult to grasp fully.
For example, a fraud detection system might flag a transaction based on subtle interactions between various data points, making it hard to pinpoint the exact reasons for the alert.
Implications for Accountability and Trust
A lack of transparency directly impacts accountability. When an AI system makes a flawed decision with significant consequences, determining responsibility becomes problematic. Is it the developers who designed the algorithm, the data scientists who trained it, or the business leaders who deployed it? The inability to explain the AI’s reasoning makes it challenging to assign blame and implement corrective measures.
This lack of accountability also erodes trust, both internally within the organization and externally with customers and stakeholders. Without understanding how an AI system works, users are less likely to accept its recommendations or rely on its outputs.
Methods for Improving Transparency and Explainability
Several approaches can enhance the transparency and explainability of AI systems. One method involves designing AI models that are inherently more interpretable, such as linear models or decision trees. These simpler models allow for a clearer understanding of how input data influences the output. Another approach focuses on developing techniques to explain the predictions of complex “black box” models.
These techniques, such as LIME (Local Interpretable Model-agnostic Explanations) and SHAP (SHapley Additive exPlanations), provide insights into the relative importance of different input features in shaping the AI’s decision. Finally, rigorous testing and validation are crucial. By systematically evaluating the AI system’s performance across various scenarios and data sets, we can gain a better understanding of its strengths and limitations.
Best Practices for Ensuring Transparency and Accountability
To foster trust and accountability in AI-driven business decisions, several best practices should be implemented.
First, document the entire AI lifecycle, from data collection and preprocessing to model training, deployment, and monitoring. This detailed record helps trace the origins of any issues and facilitates troubleshooting.
Second, establish clear lines of responsibility for AI-related decisions. This clarifies who is accountable for the system’s performance and its impact on the business.
Third, implement robust data governance procedures to ensure data quality, accuracy, and fairness. Biased or inaccurate data can lead to unfair or discriminatory outcomes, further exacerbating the lack of transparency.
Fourth, incorporate explainability techniques into the AI system’s design and development process. This allows for a deeper understanding of the AI’s decision-making process and helps identify potential biases or errors.
Finally, regularly audit and evaluate the AI system’s performance. This ongoing monitoring helps identify potential problems and ensures the system continues to meet its intended purpose.
Regulatory Compliance and Privacy Concerns
Using AI in your business opens a Pandora’s Box of regulatory compliance issues, particularly concerning data privacy. The increasing reliance on AI for data processing and analysis means businesses must navigate a complex web of laws and regulations to avoid hefty fines and reputational damage. Understanding these regulations and implementing robust compliance measures is crucial for responsible AI adoption.The landscape of data privacy regulations is constantly evolving, but some key players consistently impact how businesses handle data, especially when AI is involved.
These regulations often dictate how personal data can be collected, processed, stored, and shared, placing significant restrictions on how AI systems can operate. Non-compliance can lead to severe consequences, including substantial financial penalties, legal battles, and irreversible damage to brand reputation.
Relevant Data Privacy Regulations and Compliance Requirements
Several significant regulations govern data privacy, with variations across jurisdictions. The General Data Protection Regulation (GDPR) in Europe, the California Consumer Privacy Act (CCPA) in California, and the various state privacy laws emerging in the US are prime examples. These regulations establish stringent requirements for data processing, including consent, data minimization, and the right to be forgotten. Businesses must understand the specific requirements of the regions where they operate and the data they process.
For example, GDPR requires explicit consent for processing personal data, while CCPA grants consumers rights to access, delete, and opt-out of the sale of their data. Failure to adhere to these stipulations can result in significant penalties.
Examples of AI Systems Violating Data Privacy Regulations
AI systems, by their very nature, often process vast amounts of data. This presents several opportunities for privacy violations. For instance, an AI system trained on sensitive medical data without proper anonymization could inadvertently reveal patients’ identities, directly violating HIPAA regulations. Similarly, facial recognition technology deployed without appropriate consent and safeguards could breach GDPR’s requirements for data processing.
Another example involves AI-powered profiling tools that categorize individuals based on sensitive attributes, leading to discriminatory outcomes and potentially violating anti-discrimination laws. The use of AI for surveillance without transparency or accountability also raises serious privacy concerns and might violate existing legal frameworks.
Potential Penalties and Legal Consequences of Non-Compliance
The consequences of non-compliance with data privacy regulations when using AI can be severe. GDPR, for example, imposes fines of up to €20 million or 4% of annual global turnover, whichever is higher. CCPA also includes significant penalties for violations. Beyond financial penalties, businesses face reputational damage, loss of customer trust, and potential legal action from individuals whose data has been mishandled.
In some cases, non-compliance can lead to criminal charges. The legal complexities and potential costs associated with data breaches and privacy violations significantly outweigh the risks of proactive compliance.
Checklist for Ensuring Compliance with Data Privacy Regulations When Using AI
Before implementing any AI system that handles personal data, businesses should undertake a thorough risk assessment. This should include:
- Identifying all personal data processed by the AI system.
- Determining the legal basis for processing that data (e.g., consent, contract, legal obligation).
- Implementing appropriate technical and organizational measures to ensure data security and privacy (e.g., data encryption, access controls).
- Developing a data breach response plan.
- Regularly auditing the AI system’s compliance with data privacy regulations.
- Ensuring transparency with data subjects about how their data is being used.
- Providing individuals with their data privacy rights (e.g., access, rectification, erasure).
- Appointing a Data Protection Officer (DPO) where required by law.
By proactively addressing these concerns, businesses can mitigate the risks associated with using AI and ensure compliance with data privacy regulations. This proactive approach not only avoids legal pitfalls but also fosters trust with customers and stakeholders.
Security Risks of Cloud-Based AI Solutions
The increasing reliance on cloud-based AI solutions presents significant security challenges for businesses. Storing and processing sensitive business data in a third-party environment introduces vulnerabilities that must be carefully addressed to prevent data breaches, financial losses, and reputational damage. The inherent complexity of AI systems, combined with the distributed nature of cloud infrastructure, expands the attack surface and necessitates a proactive and multi-layered security approach.The security risks associated with cloud-based AI are multifaceted, stemming from both the inherent vulnerabilities of cloud environments and the unique characteristics of AI technologies themselves.
Data breaches, unauthorized access, and malicious attacks can have devastating consequences for businesses, impacting not only their operational efficiency but also their customer trust and regulatory compliance. Understanding these risks and implementing robust security measures is crucial for mitigating potential threats.
Data Breaches and Unauthorized Access in Cloud AI
Cloud-based AI systems, like any cloud-based service, are susceptible to data breaches and unauthorized access. Vulnerabilities in the cloud provider’s infrastructure, misconfigurations in the AI system’s settings, or weak access controls can allow malicious actors to gain access to sensitive business data. For instance, a poorly secured API endpoint could allow unauthorized access to training data or model parameters, potentially revealing confidential business information or intellectual property.
A sophisticated attack might involve exploiting vulnerabilities in the underlying cloud infrastructure to gain access to multiple AI systems and their associated data. This could lead to a significant data breach with far-reaching consequences.
Vulnerabilities in AI Model and Data Security
AI models themselves can be vulnerable to attacks. Adversarial attacks, for example, involve subtly manipulating input data to cause the AI model to produce incorrect or malicious outputs. This could have serious implications in applications like fraud detection or medical diagnosis. Furthermore, the data used to train AI models can contain sensitive information. If this data is not properly secured, it could be exposed, leading to privacy violations or intellectual property theft.
For example, a model trained on customer data might inadvertently reveal sensitive personal information if the training data is not properly anonymized or secured.
Securing Cloud-Based AI Systems
Effective security strategies for cloud-based AI systems require a multi-layered approach combining robust security measures at various levels. This includes implementing strong access controls, encryption both in transit and at rest, regular security audits and penetration testing, and the use of advanced security technologies such as intrusion detection and prevention systems. Data loss prevention (DLP) tools can monitor and prevent sensitive data from leaving the cloud environment unauthorized.
Employing a zero-trust security model, which assumes no implicit trust, and implementing strong authentication and authorization mechanisms are also critical. Regular updates and patching of the AI system and underlying infrastructure are essential to address known vulnerabilities.
Implementing Robust Security Measures
Robust security measures for cloud-based AI involve several key steps. Firstly, a comprehensive risk assessment should identify potential vulnerabilities and prioritize mitigation strategies. This assessment should consider the specific risks associated with the type of data being processed, the AI model being used, and the cloud environment being utilized. Next, implement encryption for all data at rest and in transit.
This protects data from unauthorized access even if a breach occurs. Regular security audits and penetration testing help identify vulnerabilities before they can be exploited. Implementing strong access controls, such as multi-factor authentication and role-based access control, limits access to sensitive data and AI systems. Finally, ongoing monitoring and threat detection are critical to identify and respond to potential security incidents promptly.
Regularly reviewing and updating security policies and procedures is crucial to maintain a strong security posture.
Epilogue
Embracing AI for business growth is undeniably enticing, but it’s a double-edged sword. The benefits are immense, but the risks are real and substantial. By understanding the potential pitfalls – from data breaches and intellectual property theft to algorithmic bias and regulatory compliance challenges – businesses can proactively mitigate these risks and build a robust, secure, and ethical foundation for their AI initiatives.
It’s about finding the balance between innovation and responsible data management, ensuring that the power of AI serves to enhance, not endanger, your business.
Frequently Asked Questions
What happens if my AI vendor goes bankrupt?
Losing access to your data or facing significant disruption in your operations are key risks. Consider data portability and diverse vendor strategies to avoid this.
How can I ensure my AI system is truly unbiased?
Regular audits, diverse datasets, and transparency in algorithmic design are crucial. There’s no single solution, but a multi-faceted approach is essential.
Can I use AI without any risk?
No technology is entirely risk-free. However, implementing robust security measures, thorough due diligence on vendors, and a strong understanding of relevant regulations can significantly reduce risks.
What are the potential legal ramifications of an AI-related data breach?
Depending on the jurisdiction and the severity of the breach, penalties can range from significant fines to legal action from affected individuals and regulatory bodies. It’s crucial to have robust legal counsel.