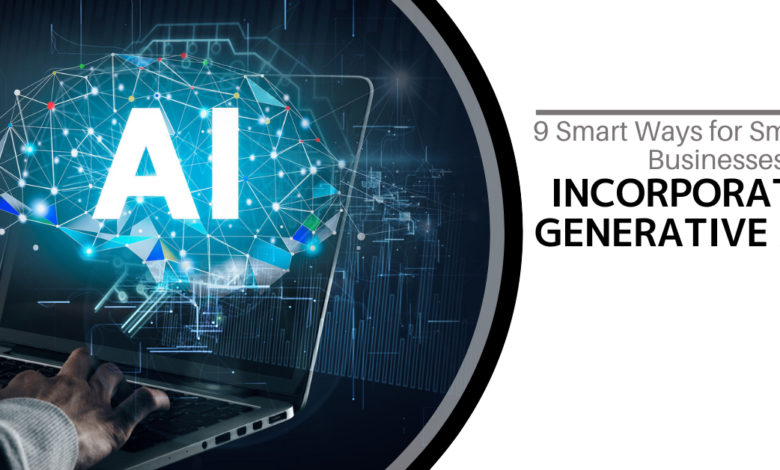
Transforming Enterprises with Generative AI
Transforming enterprises with generative ai overcoming limitations and unlocking potential – Transforming enterprises with generative AI: overcoming limitations and unlocking potential. It sounds like science fiction, right? But the reality is, generative AI is rapidly changing the business landscape. We’re not just talking about incremental improvements; we’re talking about a fundamental shift in how companies operate, innovate, and compete. This post dives deep into how businesses can harness the power of generative AI, navigate its challenges, and ultimately, unlock a future brimming with unprecedented opportunities.
Get ready to explore the exciting possibilities and the practical steps needed to make this transformation a reality.
From automating tedious tasks and personalizing customer experiences to fueling groundbreaking innovation, generative AI offers a plethora of benefits. But it’s not all smooth sailing. We’ll also tackle the hurdles – the ethical considerations, the security concerns, and the potential for bias – and explore strategies for mitigating these risks. By the end, you’ll have a clear understanding of how to strategically integrate generative AI into your enterprise, maximizing its potential while minimizing its inherent challenges.
Defining Generative AI’s Role in Enterprise Transformation
Generative AI is rapidly reshaping the business landscape, offering unprecedented opportunities for increased efficiency, innovation, and competitive advantage. Its ability to create new content – text, images, audio, and even code – is transforming how enterprises operate and interact with their customers. This shift represents a significant leap beyond traditional AI systems, which primarily focus on analysis and prediction.Generative AI’s Core Functionalities and Differentiation from Traditional AIGenerative AI models leverage sophisticated algorithms, often based on deep learning techniques like transformers, to generate new data instances that resemble the training data.
Unlike traditional AI, which primarily focuses on analyzing existing data to make predictions or classifications, generative AI actively creates new content. This ability opens doors to applications that were previously impossible. For example, traditional AI might analyze customer data to predict churn, while generative AI could create personalized marketing copy to proactively reduce churn. The core functionalities relevant to business operations include text generation (for marketing, reports, and customer service), image generation (for product design and marketing materials), code generation (for software development), and data augmentation (for improving the performance of other AI models).Generative AI’s Transformative Potential Across Business FunctionsGenerative AI’s transformative potential is most significant in areas requiring content creation, automation, and personalization.
Marketing and sales teams can leverage it to create highly targeted advertising campaigns and personalized customer communications. Product development teams can use it to accelerate design processes and explore new product concepts. Customer service teams can utilize it to automate responses to common inquiries and provide 24/7 support. In operations, generative AI can optimize processes, predict potential failures, and automate repetitive tasks.
Furthermore, it can significantly enhance research and development by accelerating the discovery of new materials, drugs, and solutions.Examples of Successful Generative AI ImplementationsThe following table showcases successful generative AI implementations across diverse industries:
Industry | Company | Application | Results |
---|---|---|---|
Pharmaceuticals | Atomwise | Drug discovery using generative AI to design novel molecules | Faster identification of potential drug candidates, reduced research and development costs. |
Marketing & Advertising | Persado | Generating personalized marketing copy and subject lines | Improved click-through rates and conversion rates in marketing campaigns. |
Software Development | GitHub Copilot | AI-powered code completion and suggestion tool | Increased developer productivity and reduced coding errors. |
E-commerce | Shopify | AI-powered image generation for product marketing | Enhanced product presentations, leading to increased sales. |
Overcoming Limitations of Generative AI in Enterprise Settings: Transforming Enterprises With Generative Ai Overcoming Limitations And Unlocking Potential
Generative AI holds immense promise for enterprise transformation, but its deployment isn’t without significant hurdles. Successfully integrating this technology requires a clear understanding of its limitations and the development of robust mitigation strategies. Ignoring these challenges could lead to inaccurate outputs, ethical breaches, and significant security risks. This section dives into the key limitations and proposes practical solutions.
Technical Challenges in Generative AI Deployment
Deploying generative AI within an enterprise environment presents several technical challenges. These range from computational resource requirements to the complexity of integrating these models into existing workflows. High-performance computing infrastructure is often necessary to handle the demanding computational needs of large language models and image generation algorithms. Furthermore, integrating these models with existing enterprise systems and data sources can be complex and require significant software engineering expertise.
Model fine-tuning and adaptation to specific enterprise needs also add to the complexity, demanding specialized skills and resources. Finally, ensuring the reliability and robustness of generative AI models in real-world enterprise applications, particularly in high-stakes decision-making processes, is crucial and often challenging to achieve.
Ethical Considerations and Potential Biases
Generative AI models are trained on vast datasets, and these datasets can reflect existing societal biases. This can lead to the AI generating outputs that perpetuate or even amplify harmful stereotypes and discrimination. For instance, a model trained on biased data might produce job descriptions that unintentionally favor certain demographics. Furthermore, the use of generative AI in decision-making processes raises ethical concerns about transparency and accountability.
If a crucial decision is based on an AI’s output, it’s important to understand the reasoning behind the decision and to have mechanisms for human oversight and intervention. The lack of transparency in some generative AI models makes it difficult to identify and correct biased outputs, posing a significant ethical challenge.
Data Security and Privacy Concerns
The implementation of generative AI raises significant data security and privacy concerns. These models often require access to large amounts of sensitive enterprise data during training and operation. This data may include confidential customer information, proprietary business data, and intellectual property. Ensuring the confidentiality, integrity, and availability of this data is paramount. Data breaches resulting from vulnerabilities in generative AI systems could have severe consequences, including financial losses, reputational damage, and legal liabilities.
Moreover, the use of generative AI to process personal data raises privacy concerns, particularly in compliance with regulations like GDPR and CCPA. Careful consideration of data anonymization and access control mechanisms is crucial to mitigate these risks.
Strategies for Mitigating Risks and Addressing Limitations
Addressing the limitations of generative AI in enterprise settings requires a multi-pronged approach. The following strategies can help mitigate the risks and unlock the full potential of this transformative technology:
- Invest in robust infrastructure: Deploy high-performance computing resources capable of handling the computational demands of generative AI models.
- Develop comprehensive data governance policies: Establish clear guidelines for data access, usage, and security to protect sensitive information.
- Implement rigorous bias detection and mitigation techniques: Regularly audit models for biases and develop strategies to correct them.
- Prioritize model explainability and transparency: Use techniques that allow for understanding the reasoning behind the AI’s outputs.
- Ensure human oversight and control: Implement mechanisms for human review and intervention in critical decision-making processes.
- Adopt a phased implementation approach: Start with pilot projects to test and refine the technology before widespread deployment.
- Invest in skilled personnel: Hire and train individuals with expertise in AI, data science, and cybersecurity.
- Stay updated on regulatory developments: Keep abreast of evolving data privacy and security regulations.
Unlocking the Potential of Generative AI for Enhanced Productivity
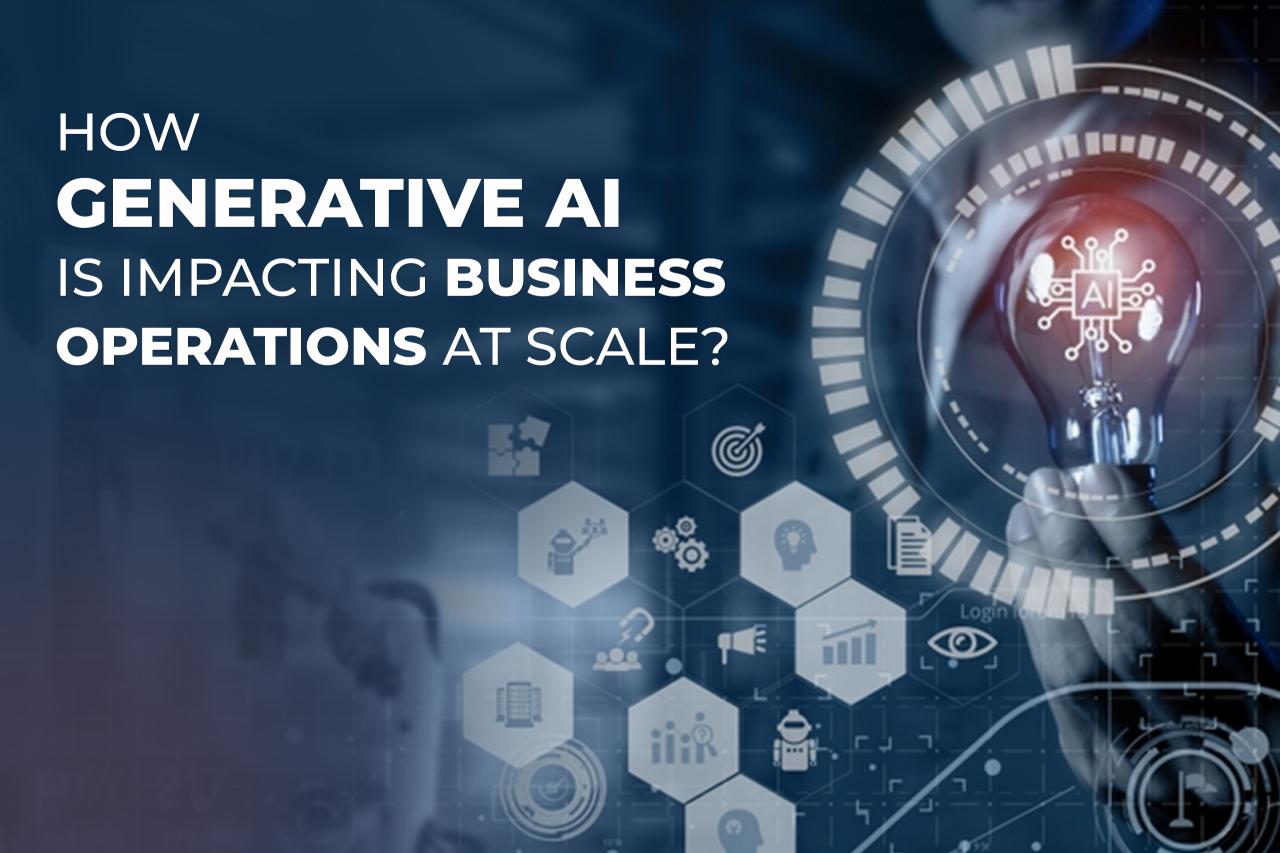
Generative AI is no longer a futuristic concept; it’s a powerful tool reshaping how businesses operate and achieve their goals. By automating tedious tasks, personalizing customer experiences, and fostering innovation, generative AI is unlocking unprecedented levels of productivity and efficiency across various industries. This transformative technology is not just about incremental improvements; it’s about fundamentally altering the way work gets done, freeing up human capital for more strategic and creative endeavors.Generative AI’s ability to automate repetitive tasks and improve operational efficiency is particularly impactful.
This translates to significant cost savings and allows employees to focus on higher-value activities.
Automating Repetitive Tasks and Improving Operational Efficiency
Generative AI can significantly streamline operations by automating various repetitive tasks. For instance, in customer service, AI-powered chatbots can handle routine inquiries, freeing up human agents to deal with more complex issues. In finance, generative AI can automate report generation, data entry, and even aspects of fraud detection. In manufacturing, it can optimize production schedules and predict equipment maintenance needs.
The result is a leaner, more efficient operation with reduced operational costs and increased employee satisfaction. For example, a large bank reported a 30% reduction in customer service call handling time after implementing a generative AI-powered chatbot. This freed up human agents to focus on more complex and high-value interactions, leading to improved customer satisfaction scores and reduced operational expenses.
Enhancing Customer Service and Engagement Through Personalized Interactions, Transforming enterprises with generative ai overcoming limitations and unlocking potential
Beyond automation, generative AI excels at personalizing customer interactions. By analyzing customer data, generative AI can tailor product recommendations, create personalized marketing campaigns, and generate customized responses to customer inquiries. This level of personalization fosters stronger customer relationships, increases brand loyalty, and drives sales. Imagine a clothing retailer using generative AI to create personalized outfit recommendations based on a customer’s browsing history and preferences.
This level of tailored service enhances the shopping experience and increases the likelihood of a purchase. Similarly, a travel agency can use generative AI to craft unique itineraries based on individual customer needs and travel styles.
Facilitating Innovation by Generating New Ideas and Solutions
Generative AI’s ability to analyze vast datasets and identify patterns allows it to generate novel ideas and solutions that might otherwise be overlooked. This capability is invaluable for fostering innovation across various departments. For example, in research and development, generative AI can assist in designing new products or processes, while in marketing, it can help generate creative ad copy or campaign concepts.
A pharmaceutical company could use generative AI to design new drug molecules, accelerating the drug discovery process. A software company could leverage it to generate new code snippets, speeding up software development.
Workflow Improvement Visualization
Imagine a flowchart. The top section depicts a traditional workflow, cluttered with numerous manual tasks represented by small, grey boxes connected by thick, dark arrows, indicating a slow and complex process. Human figures are depicted within several of these boxes, visibly stressed and overwhelmed. Below this, a second flowchart shows the same process after generative AI integration. The grey boxes are significantly reduced in number, replaced by larger, bright green boxes representing automated tasks.
The arrows are thinner and more streamlined, illustrating a smoother, more efficient flow. Human figures are still present, but they appear relaxed and focused on higher-level tasks within brightly lit, spacious boxes. The overall visual impression is one of significantly improved efficiency and reduced workload, highlighting the transformative impact of generative AI.
Generative AI and the Future of Work
The integration of generative AI into the workplace is rapidly reshaping the landscape of employment, presenting both exciting opportunities and significant challenges. While concerns about job displacement are valid, a more nuanced perspective reveals a complex interplay of job creation, transformation, and the evolution of required skills. Understanding this dynamic is crucial for navigating the future of work effectively.
Generative AI’s impact on job roles and workforce skills is multifaceted. Some tasks currently performed by humans will undoubtedly be automated, leading to potential job displacement in certain sectors. However, simultaneously, new roles and opportunities will emerge, requiring individuals to adapt and acquire new skills. This necessitates a proactive approach to workforce development and upskilling initiatives.
Job Displacement Versus Job Creation
The debate surrounding job displacement versus job creation fueled by generative AI is ongoing. While repetitive, data-entry-heavy tasks are indeed susceptible to automation, the overall impact is likely to be more complex than simple job losses. For example, the legal profession might see a reduction in paralegals focused on document review, but a simultaneous increase in demand for legal professionals skilled in utilizing and managing AI-driven legal research tools.
Similarly, marketing departments might see a decrease in need for certain types of content creators, but an increase in demand for individuals who can effectively manage and guide AI-driven content generation. The net effect is a shift in job requirements, rather than a simple reduction in overall employment. We can anticipate that certain jobs will become obsolete, while others will be created, requiring a workforce capable of adapting and learning new skills.
Essential Skills for a Generative AI-Powered Workplace
To thrive in this evolving environment, employees will need a diverse skillset extending beyond technical proficiency. Crucially, individuals will need to be adept at critical thinking, problem-solving, and creativity. These skills will be essential to guide, interpret, and refine the outputs of generative AI tools. Furthermore, skills in data analysis, ethical considerations related to AI, and prompt engineering will become increasingly valuable.
Effective communication and collaboration remain vital, as humans will continue to play a crucial role in overseeing and directing AI-driven processes.
A Training Program for Upskilling the Workforce
A comprehensive upskilling program is vital to equip the workforce with the necessary competencies to navigate the age of generative AI. This program should be modular and adaptable to different roles and skill levels.
The following modules are crucial components of such a program:
- Introduction to Generative AI: This module will provide a foundational understanding of generative AI, its capabilities, and limitations.
- Ethical Considerations in AI: This module will cover responsible AI use, bias detection, and the ethical implications of AI-driven decisions.
- Prompt Engineering and Optimization: This module will focus on crafting effective prompts to maximize the quality and relevance of AI-generated outputs.
- Data Analysis and Interpretation: This module will teach employees how to analyze and interpret data generated by or used by AI systems.
- AI Tool Proficiency: This module will provide hands-on training with specific generative AI tools relevant to individual roles and departments.
- Human-AI Collaboration: This module will focus on strategies for effective collaboration between humans and AI systems, emphasizing the strengths of each.
- Future of Work and Career Development: This module will help employees understand the evolving job market and develop strategies for career advancement in an AI-driven world.
Strategic Implementation of Generative AI
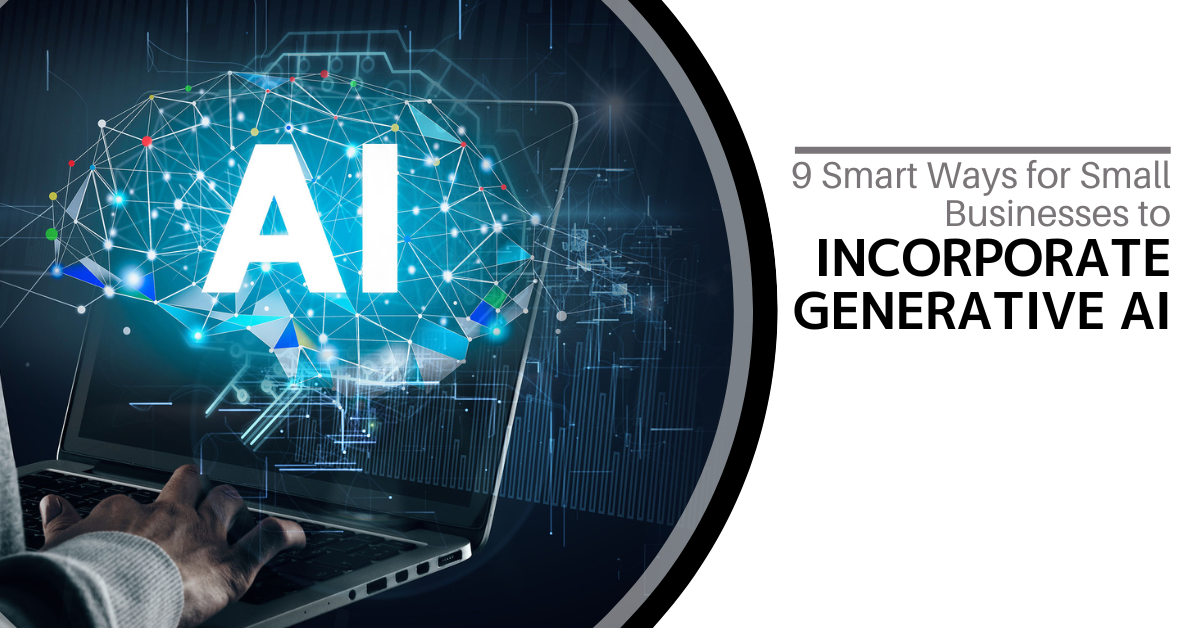
Integrating generative AI successfully requires a well-defined strategy that goes beyond simply acquiring the technology. It necessitates a phased approach, careful consideration of existing infrastructure, and a commitment to ongoing monitoring and refinement. This process ensures a smooth transition, maximizes ROI, and minimizes potential disruptions.Successfully integrating generative AI into an enterprise requires a structured, multi-stage process. This involves careful planning, resource allocation, and ongoing monitoring to ensure the technology delivers its promised benefits.
Ignoring these steps can lead to wasted resources and a failure to realize the full potential of generative AI.
A Step-by-Step Process for Generative AI Integration
Implementing generative AI effectively involves a series of well-defined steps. Each stage builds upon the previous one, ensuring a robust and scalable deployment. Failing to follow a structured approach can lead to integration challenges and suboptimal performance.
Stage | Action | Metric | Responsibility |
---|---|---|---|
Assessment & Planning | Identify use cases, assess existing infrastructure, define project scope and objectives, and assemble a cross-functional team. | Number of viable use cases identified, feasibility assessment score, project scope document completion. | IT Department, Business Units, Project Management Office |
Proof of Concept (POC) | Develop and test a small-scale implementation of generative AI for a prioritized use case. | Success rate of POC, time taken for implementation, cost of POC, user feedback. | Data Science Team, IT Department |
Pilot Deployment | Deploy the generative AI solution to a limited user group within a specific department. | User adoption rate, improvement in key performance indicators (KPIs), issue resolution time. | IT Department, Business Unit, Project Management Office |
Full-Scale Deployment | Roll out the generative AI solution across the enterprise, integrating it with existing systems and workflows. | System uptime, data accuracy, user satisfaction, return on investment (ROI). | IT Department, Business Units, Operations Team |
Monitoring & Optimization | Continuously monitor system performance, gather user feedback, and make necessary adjustments to optimize the solution. | System performance metrics, user feedback scores, cost savings, efficiency gains. | IT Department, Data Science Team, Business Units |
Evaluating the ROI of Generative AI Initiatives
Measuring the return on investment (ROI) of generative AI is crucial for justifying its implementation and securing continued funding. A comprehensive approach considers both tangible and intangible benefits. Without clear ROI metrics, it’s difficult to demonstrate the value proposition of generative AI to stakeholders.A robust ROI evaluation should include quantifiable metrics such as cost savings from automation, increased efficiency, improved customer satisfaction, and enhanced product quality.
For example, a company might measure the reduction in customer service call times or the increase in sales leads generated by a generative AI-powered chatbot. Qualitative benefits, such as improved employee morale or enhanced decision-making, should also be considered, though they are harder to quantify.
Establishing Clear Metrics for Measuring Success
Defining clear, measurable, achievable, relevant, and time-bound (SMART) metrics is essential for tracking the success of generative AI implementations. These metrics should align with the overall business objectives and provide a clear picture of the technology’s impact. Without well-defined metrics, it’s difficult to assess the effectiveness of the implementation and make data-driven improvements.Examples of SMART metrics include: a 20% reduction in processing time for a specific task within three months; a 15% increase in customer satisfaction scores within six months; or a 10% reduction in operational costs within one year.
Regularly tracking and reporting on these metrics allows for proactive adjustments and ensures the generative AI initiative remains aligned with business goals.
Best Practices for Managing and Monitoring Generative AI Systems
Effective management and monitoring are critical for ensuring the optimal performance and security of generative AI systems. This includes establishing robust security protocols, implementing regular system updates, and providing ongoing training for users. Neglecting these aspects can lead to security vulnerabilities, system downtime, and inaccurate outputs.Best practices include establishing clear roles and responsibilities for managing the system, implementing regular security audits, and utilizing monitoring tools to track system performance and identify potential issues.
Regular user training is also crucial to ensure users understand how to effectively utilize the system and avoid common pitfalls. Proactive maintenance and updates are essential to address security vulnerabilities and enhance system performance.
Last Recap
The journey to transforming your enterprise with generative AI is undoubtedly ambitious, but the rewards are equally immense. By thoughtfully addressing the limitations, focusing on ethical implementation, and investing in upskilling your workforce, you can unlock a new era of productivity, innovation, and competitive advantage. Remember, it’s not just about adopting the technology; it’s about strategically integrating it into your existing infrastructure and processes to achieve tangible, measurable results.
The future of business is here, and it’s powered by generative AI. Are you ready to embrace it?
Answers to Common Questions
What are the biggest risks associated with implementing generative AI?
The biggest risks include data breaches, biased outputs leading to unfair decisions, and the potential for job displacement if not managed properly. Robust security measures, careful data selection, and a focus on reskilling employees are crucial to mitigating these risks.
How much does it cost to implement generative AI?
The cost varies drastically depending on the scale of implementation, the chosen tools, and the level of customization required. Factors like infrastructure needs, data preparation, and employee training all contribute to the overall expense. A thorough cost-benefit analysis is essential before embarking on any implementation.
What kind of data is best suited for generative AI?
Generative AI thrives on large, high-quality datasets. The data needs to be relevant to the intended application, clean (free of errors and inconsistencies), and representative of the problem you’re trying to solve. Poor quality data will lead to poor results.
How can I measure the success of my generative AI implementation?
Success should be measured using specific, measurable, achievable, relevant, and time-bound (SMART) goals. These might include improvements in efficiency, customer satisfaction, innovation rates, or cost reduction. Regular monitoring and adjustments are key to optimizing performance.