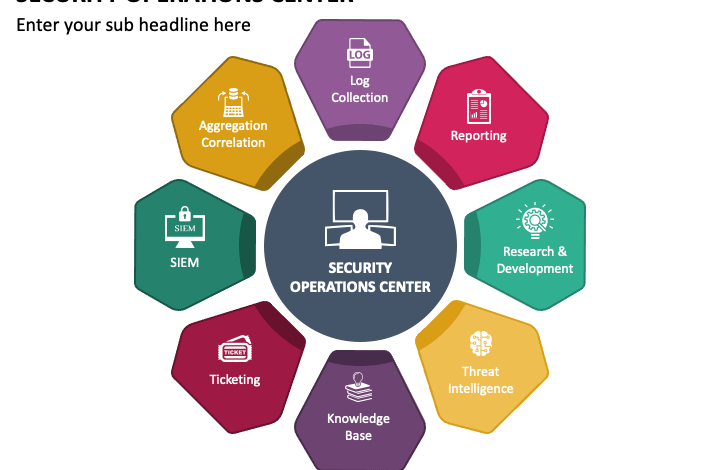
Understanding Intelligent Operations A Comprehensive Introduction
Understanding intelligent operations a comprehensive introduction – Understanding intelligent operations: a comprehensive introduction – that’s what this blog post is all about! We’re diving deep into the fascinating world of how AI and advanced analytics are transforming business operations. From the history and core principles to the practical implementation and future trends, we’ll explore everything you need to know to get up to speed on this game-changing field.
Get ready to discover how intelligent operations are streamlining processes, improving decision-making, and ultimately driving success in today’s competitive landscape.
This post will cover the key technologies enabling intelligent operations, such as AI and machine learning, and how they are applied to optimize various processes. We’ll also delve into the practical aspects of implementation, including planning, integration, data management, and risk mitigation. We’ll look at how to measure the effectiveness of intelligent operations using key performance indicators (KPIs) and explore the future trends shaping this rapidly evolving field.
Defining Intelligent Operations
Intelligent Operations (IOps) represent a significant evolution in how businesses manage and optimize their operational processes. It’s a paradigm shift driven by the convergence of advanced technologies, aiming for greater efficiency, agility, and proactive problem-solving. Instead of reacting to issues, IOps anticipates and addresses them before they impact business performance.
Core Principles of Intelligent Operations
The core principles of IOps revolve around leveraging data-driven insights and automation to enhance operational efficiency. This includes real-time monitoring and analysis of operational data, predictive modeling to anticipate potential problems, and automated responses to optimize performance. Central to this is the use of artificial intelligence (AI), machine learning (ML), and other advanced analytics techniques to gain a deeper understanding of operational processes and identify areas for improvement.
A key aspect is the integration of these technologies across various operational silos to provide a holistic view and enable coordinated actions.
Historical Overview of Intelligent Operations
The evolution of IOps is intrinsically linked to the advancements in computing power and data analytics. Early forms of operational optimization relied on manual processes and rudimentary reporting. The advent of Business Intelligence (BI) tools in the late 20th century marked a significant step towards data-driven decision-making. However, true IOps emerged with the rise of big data, cloud computing, and AI/ML technologies in the 21st century.
These technologies enabled the processing and analysis of vast amounts of data in real-time, paving the way for predictive maintenance, automated anomaly detection, and intelligent process automation.
Examples of Successful Intelligent Operations Implementations
Several industries have successfully implemented IOps, reaping significant benefits. In manufacturing, predictive maintenance using sensor data and ML algorithms minimizes downtime by anticipating equipment failures. Logistics companies use AI-powered route optimization to reduce delivery times and fuel consumption. Financial institutions leverage AI for fraud detection and risk management, improving security and regulatory compliance. Healthcare providers utilize IOps to improve patient care through predictive analytics for disease management and personalized treatment plans.
Key Differences Between Traditional Operations and Intelligent Operations
Traditional operations often rely on reactive measures, responding to problems after they occur. This approach is often inefficient and costly. IOps, conversely, focuses on proactive measures, leveraging data analytics and automation to anticipate and mitigate potential issues before they arise. Traditional operations typically involve manual processes and limited data analysis, whereas IOps utilizes advanced technologies like AI and ML for real-time monitoring, analysis, and automation.
The result is a significant improvement in efficiency, agility, and overall operational performance. Furthermore, traditional operations struggle with complex, interconnected systems, while IOps excels at managing and optimizing such systems through integrated data analysis and automated responses.
Framework for Classifying Different Types of Intelligent Operations
A framework for classifying IOps could be based on the primary technology employed and the operational area targeted. For example, one category could focus on AI-driven predictive maintenance in manufacturing, another on ML-based fraud detection in finance, and a third on robotic process automation (RPA) in customer service. Another classification could be based on the level of automation: reactive automation (responding to events), proactive automation (anticipating events), and self-optimizing automation (continuously adapting to changing conditions).
A comprehensive framework would consider both the technology used and the level of automation achieved, providing a nuanced understanding of the diverse applications of IOps.
Key Technologies Enabling Intelligent Operations
Intelligent operations rely heavily on a convergence of cutting-edge technologies. These technologies work synergistically to automate processes, analyze vast amounts of data, and ultimately, improve decision-making across all operational aspects. This section will delve into the specific roles of Artificial Intelligence, Machine Learning, and Big Data analytics in achieving this transformation.
The Role of Artificial Intelligence in Intelligent Operations
AI is the backbone of intelligent operations, providing the cognitive capabilities necessary to automate complex tasks and learn from operational data. AI systems, through techniques like natural language processing (NLP) and computer vision, can interpret unstructured data, identify patterns, and predict future outcomes with a level of accuracy previously unattainable. For instance, AI-powered chatbots can handle customer service inquiries, freeing up human agents to focus on more complex issues.
Predictive maintenance systems, using AI to analyze sensor data from machinery, can anticipate equipment failures and schedule maintenance proactively, minimizing downtime and maximizing efficiency. The ability of AI to handle complex, nuanced situations and learn continuously makes it an invaluable asset in optimizing operational performance.
Machine Learning Applications in Optimizing Operational Processes
Machine learning (ML), a subset of AI, focuses on enabling systems to learn from data without explicit programming. This is crucial for optimizing operational processes because it allows for the identification of patterns and trends that might be missed by human analysts. ML algorithms can be trained on historical operational data to predict future demand, optimize inventory levels, and improve supply chain efficiency.
For example, a retailer might use ML to predict customer demand for specific products during peak seasons, enabling them to optimize their inventory levels and avoid stockouts or overstocking. Similarly, ML can be used to optimize logistics routes, reducing transportation costs and delivery times. The continuous learning capability of ML ensures that operational processes are constantly refined and improved over time.
Benefits of Big Data Analytics for Improved Decision-Making in Operations
Big data analytics provides the fuel for AI and ML algorithms. By analyzing massive datasets from various sources, organizations gain valuable insights into their operations. This data-driven approach enables more informed decision-making, leading to improved efficiency, reduced costs, and increased profitability. For example, analyzing customer data can reveal purchasing patterns and preferences, allowing businesses to personalize their marketing efforts and improve customer satisfaction.
Analyzing operational data can identify bottlenecks and inefficiencies in production processes, enabling organizations to optimize their workflows and reduce waste. The ability to process and analyze vast amounts of data in real-time provides a significant competitive advantage.
Comparison of AI/ML Algorithms Used in Intelligent Operations
Various AI/ML algorithms are employed in intelligent operations, each with its strengths and weaknesses. The choice of algorithm depends on the specific task and the nature of the data. For example, supervised learning algorithms, such as linear regression and support vector machines (SVMs), are suitable for tasks where labeled data is available, while unsupervised learning algorithms, such as clustering and dimensionality reduction, are used when dealing with unlabeled data.
Reinforcement learning, on the other hand, is used to train agents to make decisions in dynamic environments. Each algorithm offers a unique approach to problem-solving, allowing for tailored solutions to specific operational challenges.
Technology Suitability for Different Operational Tasks
Technology | Application | Benefits | Limitations |
---|---|---|---|
Supervised Learning (e.g., Linear Regression) | Predictive maintenance, demand forecasting | High accuracy with labeled data, relatively easy to interpret | Requires large amounts of labeled data, can be less effective with complex relationships |
Unsupervised Learning (e.g., K-means Clustering) | Customer segmentation, anomaly detection | Can identify patterns in unlabeled data, useful for exploratory analysis | Results can be difficult to interpret, sensitive to initial conditions |
Deep Learning (e.g., Convolutional Neural Networks) | Image recognition (quality control), natural language processing (chatbots) | Excellent performance on complex tasks, ability to learn from large datasets | Requires significant computational resources, can be difficult to train and interpret |
Reinforcement Learning | Robotics, autonomous systems, resource allocation | Ability to learn optimal strategies in dynamic environments | Can be computationally expensive, requires careful design of reward functions |
Implementing Intelligent Operations: Understanding Intelligent Operations A Comprehensive Introduction
Implementing intelligent operations is a transformative journey, requiring careful planning, execution, and ongoing adaptation. It’s not a simple switch-flip but a phased approach involving people, processes, and technology. Success hinges on a clear understanding of your organization’s specific needs and a commitment to continuous improvement. This process demands a strategic mindset, recognizing that intelligent operations are an ongoing evolution, not a one-time project.
Planning for Intelligent Operations Implementation
A successful implementation begins with a well-defined plan. This involves identifying key operational areas ripe for improvement through AI/ML, assessing existing infrastructure and data capabilities, and establishing clear goals and metrics for success. A thorough needs assessment should pinpoint specific pain points and opportunities for optimization. This stage also involves securing buy-in from key stakeholders across the organization, ensuring alignment on objectives and resource allocation.
Finally, a phased rollout approach minimizes disruption and allows for iterative learning and adjustment.
Integrating AI/ML Tools into Existing Workflows
Integrating AI/ML tools requires a methodical approach. First, select the right tools based on your specific needs and existing infrastructure. This often involves a proof-of-concept phase to test different solutions and evaluate their effectiveness. Next, focus on data preparation and integration. This involves cleaning, transforming, and structuring data to ensure compatibility with AI/ML algorithms.
Subsequently, develop and deploy AI/ML models, integrating them seamlessly into existing workflows. Continuous monitoring and evaluation are crucial, allowing for model retraining and adjustments as needed. Finally, ensure adequate training for employees to effectively use and manage the new tools.
Data Management and Governance in Intelligent Operations, Understanding intelligent operations a comprehensive introduction
Effective data management and governance are paramount for successful intelligent operations. This involves establishing clear data ownership, access control, and security protocols. Data quality is critical; implement robust data validation and cleansing processes to ensure accuracy and reliability. Develop a comprehensive data governance framework that aligns with relevant regulations and industry best practices. This framework should address data privacy, security, and compliance issues.
Regular data audits and reviews should be conducted to ensure ongoing compliance and identify areas for improvement.
Challenges in Implementing Intelligent Operations and Strategies for Overcoming Them
Implementing intelligent operations presents several challenges. One significant hurdle is the lack of skilled personnel with expertise in AI/ML. Addressing this requires investing in training and development programs, and potentially recruiting specialized talent. Another challenge is data silos and inconsistencies across different departments. This can be overcome by implementing data integration strategies and establishing a centralized data management system.
Resistance to change from employees accustomed to traditional methods is also common. Addressing this requires clear communication, demonstrating the value proposition of AI/ML, and providing adequate training and support. Finally, the high initial investment costs associated with AI/ML tools and infrastructure can be a deterrent. This can be mitigated through phased implementation, exploring cloud-based solutions, and securing appropriate funding.
Potential Risks and Mitigation Strategies
Implementing intelligent operations carries inherent risks that need careful consideration and proactive mitigation strategies.
- Risk: Biased AI/ML models leading to unfair or discriminatory outcomes. Mitigation: Employ diverse datasets, regularly audit models for bias, and implement fairness-aware algorithms.
- Risk: Data breaches and security vulnerabilities. Mitigation: Implement robust cybersecurity measures, including encryption, access controls, and regular security audits.
- Risk: Lack of transparency and explainability in AI/ML models. Mitigation: Utilize explainable AI (XAI) techniques to understand model decisions and ensure accountability.
- Risk: Over-reliance on AI/ML, neglecting human oversight. Mitigation: Maintain human-in-the-loop systems, allowing human intervention when necessary.
- Risk: High implementation costs and unexpected delays. Mitigation: Conduct thorough cost-benefit analysis, adopt a phased implementation approach, and establish realistic timelines.
Measuring the Effectiveness of Intelligent Operations
Implementing intelligent operations is only half the battle; understanding its impact is crucial for continuous improvement and justifying further investment. Effective measurement requires a focused approach, tracking key performance indicators (KPIs) and analyzing data to reveal the true value delivered. This allows for data-driven decisions, optimizing processes, and maximizing the return on investment (ROI) of your intelligent operations initiatives.
Key Performance Indicators (KPIs) for Intelligent Operations
Choosing the right KPIs depends heavily on your specific goals and the areas where intelligent operations are deployed. However, some common metrics provide a strong foundation for assessment. These metrics should be aligned with the overall business objectives and track the impact of intelligent operations across different aspects of the operation. Focusing on a few key indicators initially, rather than trying to track everything at once, will provide more meaningful insights.
Metric | Data Source | Target | Current Value |
---|---|---|---|
Reduced Operational Costs | Financial systems, operational databases | 15% reduction within 6 months | 10% reduction (after 3 months) |
Improved Efficiency (e.g., reduced cycle time) | Process monitoring tools, workflow management systems | 20% reduction in average processing time | 12% reduction |
Increased Productivity | Employee time tracking, output metrics | 10% increase in output per employee | 8% increase |
Enhanced Customer Satisfaction | Customer surveys, feedback forms, support tickets | 90% customer satisfaction rating | 85% customer satisfaction rating |
Tracking and Analyzing Data for Impact Assessment
Data collection is paramount. This involves integrating data sources from various systems – ERP, CRM, operational databases, and specialized AI/ML platforms – to create a holistic view of performance. Data should be regularly collected, cleaned, and analyzed using appropriate techniques, ranging from simple descriptive statistics to more advanced machine learning algorithms depending on the complexity of the data and the questions being asked.
For example, analyzing the correlation between AI-driven predictions and actual outcomes can reveal the accuracy and value of the implemented intelligent operations solutions.
Dashboard Design for Visualizing Key Metrics
A well-designed dashboard provides a clear and concise overview of performance. The table above serves as a basic example. A more sophisticated dashboard might use interactive charts and graphs to visualize trends and patterns over time. For instance, a line graph could show the trend of operational costs over the past year, highlighting the impact of intelligent operations initiatives.
Similarly, a bar chart could compare the efficiency gains across different departments or processes. Color-coding could be used to highlight areas performing above or below target. The responsiveness of the dashboard ensures easy access and readability across different devices.
Interpreting Results and Informing Future Improvements
Analyzing the data from the dashboard allows for a data-driven assessment of the success of intelligent operations. Significant deviations from targets should be investigated to identify root causes. This may involve reviewing process workflows, analyzing the performance of AI models, or evaluating the effectiveness of implemented technologies. The insights gained inform future improvements, such as refining AI models, optimizing processes, or adjusting KPIs to better reflect business priorities.
For instance, if customer satisfaction remains below target despite efficiency gains, this suggests a need to focus on improving the customer experience aspects of the intelligent operations implementation.
Continuous Monitoring and Improvement
Intelligent operations is not a one-time implementation; it’s an ongoing process. Continuous monitoring is essential to ensure that the implemented solutions remain effective and aligned with evolving business needs. Regular review of KPIs, data analysis, and adjustments to strategies are critical for maximizing the long-term benefits of intelligent operations. This iterative approach ensures that the system learns and adapts to changing conditions, maintaining its effectiveness and value over time.
For example, regularly updating AI models with new data ensures their continued accuracy and relevance.
Future Trends in Intelligent Operations
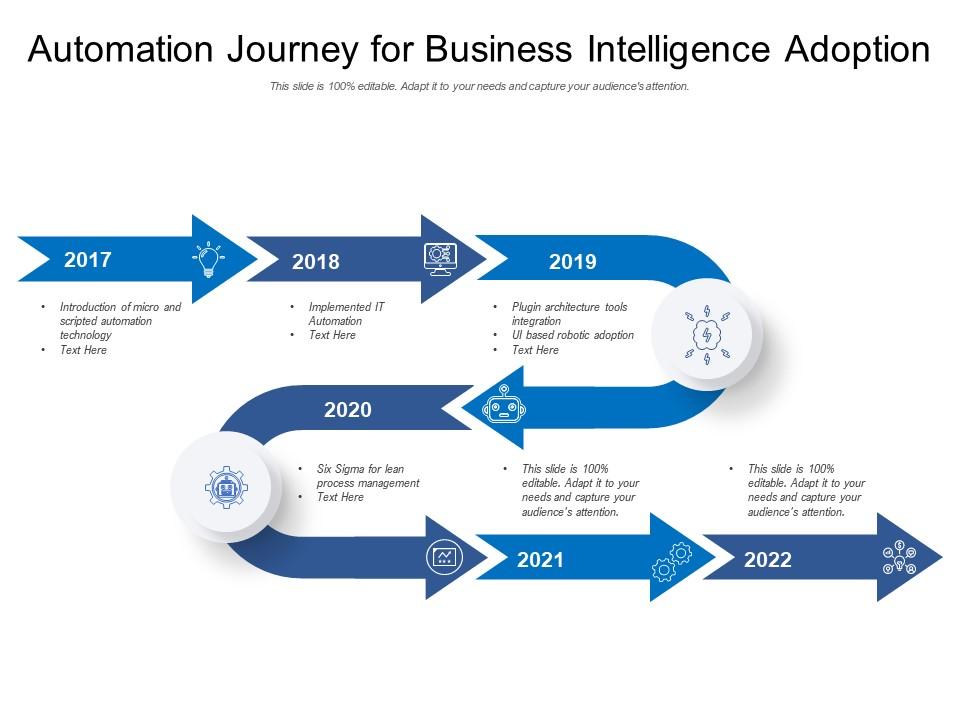
Intelligent Operations (IO) is rapidly evolving, driven by breakthroughs in artificial intelligence, machine learning, and data analytics. The next decade will witness a dramatic transformation in how businesses operate, fueled by increasingly sophisticated IO systems. This section explores the key trends shaping the future of IO, its applications in emerging industries, and its impact on the workforce and global economy.
Emerging Technologies Shaping Intelligent Operations
Several emerging technologies will significantly influence the future of IO. These include advancements in generative AI, capable of creating new content and solutions, the expansion of edge computing, bringing processing power closer to data sources for faster responses, and the development of more robust and explainable AI (XAI) models, increasing transparency and trust in AI-driven decisions. Quantum computing, while still in its nascent stages, holds the potential to revolutionize complex optimization problems crucial to IO, ultimately enabling faster and more accurate predictions and decision-making.
The convergence of these technologies will create more powerful and adaptable IO systems.
Intelligent Operations in Emerging Industries
The application of IO is expanding beyond traditional sectors. In the burgeoning field of personalized medicine, IO systems can analyze vast amounts of patient data to predict disease risk, personalize treatments, and optimize healthcare resource allocation. Similarly, in sustainable energy, IO can enhance the efficiency of renewable energy sources, optimize smart grids, and improve energy consumption forecasting. The agricultural sector is also poised for significant transformation, with IO enabling precision farming, optimized resource management, and improved crop yields through real-time data analysis and predictive modeling.
For example, a system could analyze soil conditions, weather patterns, and crop health data to automatically adjust irrigation and fertilization, minimizing waste and maximizing productivity.
Impact of Intelligent Operations on the Workforce and Economy
The widespread adoption of IO will undoubtedly reshape the workforce and the global economy. While some jobs may be automated, new roles requiring skills in AI management, data analysis, and system integration will emerge. This necessitates a shift in educational and training programs to equip the workforce with the skills needed to thrive in this evolving landscape. The overall economic impact is expected to be positive, with increased productivity, efficiency, and innovation driving economic growth.
However, managing the transition and ensuring equitable access to opportunities will be crucial to mitigating potential negative consequences. Reskilling and upskilling initiatives will be essential to prepare the workforce for the changing job market.
Projected Evolution of Intelligent Operations (2024-2034)
Year | Key Development | Example |
---|---|---|
2024-2026 | Widespread adoption of AI-powered automation in routine tasks. | Increased use of robotic process automation (RPA) in customer service and data entry. |
2027-2029 | Emergence of advanced predictive analytics and real-time optimization. | Proactive maintenance in manufacturing using sensor data and predictive models. |
2030-2034 | Integration of quantum computing for complex optimization problems. Increased use of digital twins for real-time system monitoring and simulation. | Optimizing supply chains using quantum algorithms for route planning and inventory management. Real-time simulation of complex systems (e.g., power grids) for improved resilience and efficiency. |
A Futuristic Intelligent Operations Center
Imagine a futuristic IO center: a sleek, minimalist space dominated by large interactive holographic displays showcasing real-time data visualizations. AI-powered assistants provide immediate insights and recommendations, anticipating potential issues before they arise. The center uses advanced sensor networks and data analytics to monitor and manage global operations across multiple industries, seamlessly integrating data from various sources. Sophisticated AI algorithms continuously learn and adapt, optimizing processes and predicting future trends with unprecedented accuracy.
The environment fosters collaboration, with human experts working alongside AI systems to make informed decisions, leveraging the strengths of both human intuition and machine intelligence. This futuristic center represents the culmination of decades of innovation in IO, demonstrating the transformative power of intelligent systems to enhance efficiency, optimize resource allocation, and drive innovation across all sectors.
Wrap-Up
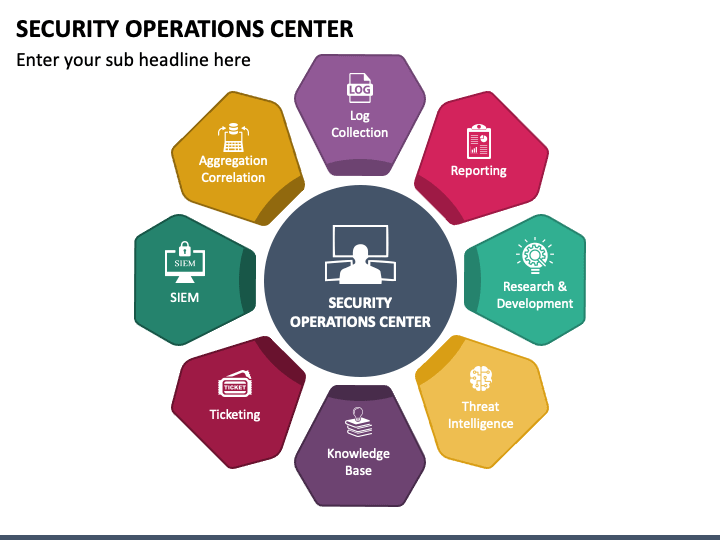
So, there you have it – a comprehensive overview of intelligent operations! From its foundational principles to its future potential, we’ve covered a lot of ground. The journey into intelligent operations might seem daunting at first, but with a structured approach, the right tools, and a focus on continuous improvement, organizations can unlock significant benefits. Remember, the key is to start small, focus on clear goals, and iterate based on data-driven insights.
The future of operations is intelligent, and it’s an exciting time to be a part of it.
FAQs
What are the ethical considerations of using AI in intelligent operations?
Ethical considerations are paramount. Bias in data can lead to unfair or discriminatory outcomes. Transparency and explainability of AI decisions are crucial for accountability. Data privacy and security must be rigorously protected.
How much does implementing intelligent operations cost?
Costs vary widely depending on the scale and complexity of the implementation. Factors include software licenses, hardware infrastructure, data integration, consulting fees, and employee training.
What skills are needed for a career in intelligent operations?
Skills in data analysis, programming (Python, R), machine learning, cloud computing, and project management are highly valued. Domain expertise in the relevant industry is also beneficial.