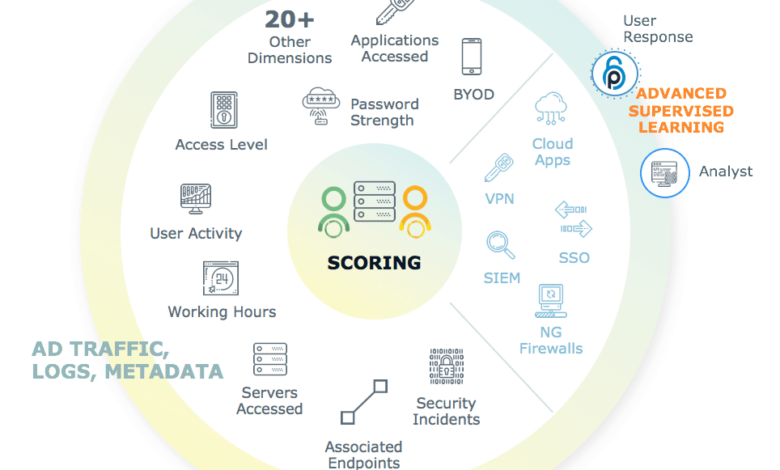
Unleashing the power of behavior analytics to improve marketing efforts
Unleashing the power of behavior analytics to improve marketing efforts is no longer a futuristic concept; it’s a present-day necessity. In today’s data-driven world, understanding how your customers behave online and offline is crucial for crafting effective marketing strategies. By analyzing user interactions, preferences, and purchasing patterns, businesses can personalize their marketing messages, optimize their websites, and ultimately, boost their ROI.
This exploration delves into the practical applications of behavior analytics, from identifying customer segments to predicting future trends and measuring campaign success.
We’ll cover the various methods for collecting and interpreting behavioral data, including website analytics, social media monitoring, and CRM data. We’ll also discuss the ethical considerations involved in using this data and how to ensure data privacy. Get ready to discover how understanding your customers’ behavior can unlock significant improvements in your marketing results.
Understanding Customer Behavior
Unlocking the true potential of behavior analytics hinges on a deep understanding of how your customers actually interact with your brand and its offerings. By analyzing their actions, we can move beyond simple demographics and delve into the motivations, preferences, and pain points that drive their decisions. This allows for more targeted, effective, and ultimately, more profitable marketing strategies.
Common Behavioral Patterns in Successful Marketing Campaigns
Successful marketing campaigns often reveal consistent behavioral patterns. For instance, customers who engage with a specific type of content (e.g., video tutorials) are more likely to convert than those who only read blog posts. Similarly, customers who add items to their cart but don’t complete the purchase often respond well to targeted email reminders or discount offers. Another key pattern is the frequency and timing of website visits; repeat visitors, particularly those visiting at specific times of day, often indicate higher levels of engagement and interest.
Analyzing these patterns allows marketers to optimize content, timing, and offers for maximum impact.
Data Sources for Gathering Customer Behavioral Information
A wealth of data informs our understanding of customer behavior. Website analytics platforms like Google Analytics provide detailed information on user traffic, page views, bounce rates, and conversion paths. CRM systems track customer interactions, purchase history, and communication preferences. Social media platforms offer insights into user engagement with brand content, sentiment analysis, and demographic information. Email marketing platforms track open rates, click-through rates, and unsubscribe rates, revealing user engagement and preferences.
Finally, transactional data from point-of-sale systems and e-commerce platforms provides direct insights into purchasing behavior. Combining data from these various sources paints a comprehensive picture of the customer journey.
Unique Behavioral Traits Across Customer Segments
Different customer segments exhibit unique behavioral traits. For example, a segment of “high-value customers” might show a higher average order value, frequent purchases, and a greater propensity to engage with premium content. Conversely, a “price-sensitive” segment might be more responsive to discounts and promotions, exhibiting shorter purchase cycles and a preference for lower-priced products. Understanding these differences is crucial for tailoring marketing messages and offers to resonate with each segment effectively.
Examples of Audience Segmentation Based on Observed Behaviors
Imagine an e-commerce store selling clothing. They could segment customers based on their browsing history: customers frequently viewing “activewear” could be targeted with ads for new running shoes or yoga pants. Customers who frequently abandon their carts could receive targeted email reminders with free shipping offers. Those who consistently engage with social media posts featuring sustainable materials could be targeted with ads highlighting eco-friendly products.
Segmentation based on behavior allows for highly personalized and effective marketing.
Comparison of Behavioral Tracking Methods
Method | Data Collected | Advantages | Disadvantages |
---|---|---|---|
Website Analytics (e.g., Google Analytics) | Website traffic, page views, bounce rate, conversion paths | Comprehensive website usage data, readily available, relatively inexpensive | Limited to online behavior, requires technical expertise to interpret fully |
CRM Systems | Customer interactions, purchase history, communication preferences | Detailed customer profiles, enables personalized communication | Data silos can exist if not integrated with other systems, requires ongoing data maintenance |
Social Media Analytics | User engagement, sentiment, demographics | Insights into brand perception and customer sentiment | Data privacy concerns, algorithm changes can impact data accuracy |
Email Marketing Platforms | Open rates, click-through rates, unsubscribe rates | Measures email campaign effectiveness, allows for segmentation based on email engagement | Limited scope, relies on email opt-in, can be impacted by spam filters |
Leveraging Behavioral Data for Improved Targeting
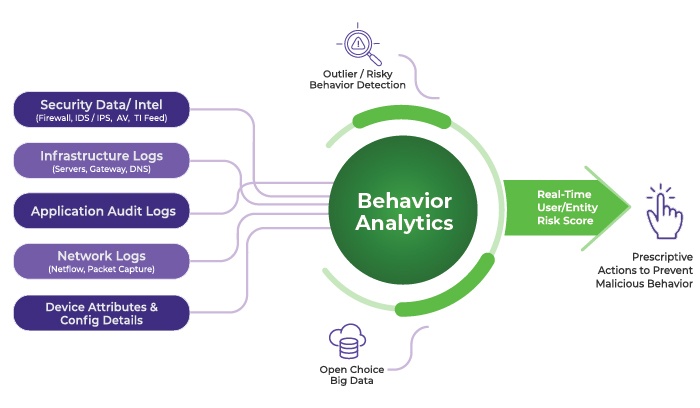
Understanding customer behavior is only half the battle; the real power lies in leveraging that understanding to refine your marketing strategies. Behavioral data, when properly analyzed and applied, allows for incredibly precise targeting, leading to higher conversion rates and a more efficient use of marketing resources. This means reaching the right people, with the right message, at the right time.
Personalizing Marketing Messages with Behavioral Data
Behavioral data offers a wealth of information for crafting personalized marketing messages. For example, if a user frequently visits your website’s product pages related to running shoes, but hasn’t made a purchase, you can send them a targeted email showcasing new arrivals in that category, perhaps including a limited-time discount or free shipping offer. Similarly, if a user abandons their shopping cart, a timely reminder email can gently nudge them towards completing their purchase.
This level of personalization significantly improves engagement and increases the likelihood of conversion. Analyzing browsing history, purchase history, and engagement with previous marketing campaigns provides a rich tapestry of insights for highly customized messaging.
Creating Targeted Ad Campaigns Based on User Behavior
The process of creating targeted ad campaigns based on user behavior begins with segmenting your audience. Instead of a broad, generic approach, you can divide your audience into smaller groups based on their demonstrated interests and behaviors. For instance, users who frequently view content related to organic skincare could be targeted with ads promoting organic skincare products. Users who regularly engage with your social media posts about sustainable practices might be receptive to ads for eco-friendly products.
This segmentation allows for the delivery of highly relevant ads, improving click-through rates and ultimately, return on investment (ROI). Each segment receives tailored messaging and creative assets optimized for their specific interests. Sophisticated platforms allow for real-time bidding and ad optimization based on ongoing user behavior.
A/B Test: Generic vs. Behaviorally Targeted Ad Campaign
Let’s imagine an A/B test comparing a generic ad campaign for a new line of headphones with a behaviorally targeted campaign. The generic campaign uses broad targeting parameters and a general message highlighting the headphones’ features. The behaviorally targeted campaign, on the other hand, targets users who have previously shown interest in audio equipment, music streaming services, or related products.
The creative assets are also tailored to reflect these interests. We might expect the behaviorally targeted campaign to achieve a significantly higher click-through rate (CTR) and conversion rate compared to the generic campaign. For instance, a CTR of 2% for the generic campaign might be compared to a 5% CTR for the behaviorally targeted campaign, demonstrating the effectiveness of personalized targeting.
Ethical Considerations of Using Behavioral Data for Marketing
The use of behavioral data for marketing presents ethical considerations that must be carefully addressed. Transparency is paramount. Users should be clearly informed about how their data is being collected and used. Data privacy must be a top priority, employing robust security measures to protect sensitive information. Avoid manipulative tactics and ensure the use of data is fair and respectful.
Compliance with relevant data privacy regulations, such as GDPR and CCPA, is crucial. Respecting user preferences and providing options for opting out of personalized advertising are essential for maintaining trust and ethical practices.
Best Practices for Ensuring Data Privacy When Utilizing Behavioral Analytics
Maintaining user privacy while leveraging behavioral data requires a proactive approach. This includes implementing strong data encryption, anonymizing data wherever possible, and regularly auditing data security practices. Obtain explicit consent before collecting and using sensitive data. Be transparent about your data collection practices and provide users with clear and accessible privacy policies. Regularly update security protocols to address emerging threats.
Unleashing the power of behavior analytics means understanding your audience deeply, leading to laser-focused campaigns. To effectively manage and analyze this data, consider exploring the efficient development options offered by domino app dev the low code and pro code future , which can streamline your workflow. This allows you to focus more on interpreting the insights from your behavior analytics and less on data management, ultimately boosting the effectiveness of your marketing efforts.
Consider employing differential privacy techniques to protect individual user data while still enabling meaningful aggregate analysis. Establish clear data retention policies and procedures for securely deleting data when it’s no longer needed. These measures build trust and demonstrate a commitment to responsible data handling.
Analyzing Website Behavior for Optimization
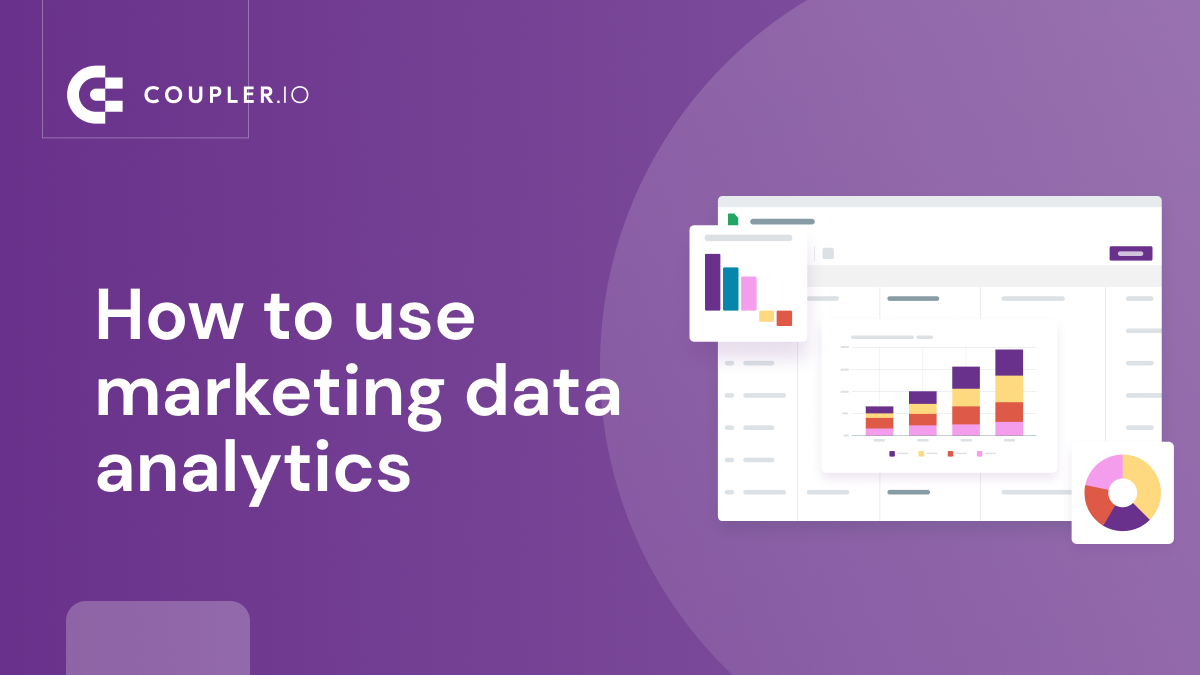
Understanding how users interact with your website is crucial for boosting conversions and improving the overall user experience. By analyzing website behavior, you gain invaluable insights into what’s working and what needs improvement, allowing you to make data-driven decisions that directly impact your bottom line. This involves identifying key metrics, leveraging visualization tools, and interpreting data to optimize content and enhance user journeys.
Key Website Metrics Indicating Successful User Engagement
Successful user engagement on a website isn’t solely about attracting visitors; it’s about keeping them engaged and guiding them towards desired actions, such as making a purchase or filling out a form. Several key metrics provide a clear picture of user engagement levels. These metrics, when analyzed together, paint a comprehensive picture of user behavior and website effectiveness.
- Average Session Duration: A longer average session duration suggests users are finding your content valuable and engaging. A significant increase in this metric over time indicates positive changes to your website.
- Pages per Visit: This metric reveals how deeply users are navigating your site. Higher numbers indicate a more thorough exploration of your content, suggesting improved site architecture or more compelling content.
- Bounce Rate: A low bounce rate suggests users are finding what they’re looking for and staying on your site. A high bounce rate, conversely, often points to issues with content relevance or website usability.
- Conversion Rate: This is the ultimate metric. It measures the percentage of visitors who complete a desired action (e.g., purchase, signup). Improvements in this metric directly translate to business success.
Utilizing Heatmaps and Session Recordings to Reveal User Behavior Patterns
Heatmaps and session recordings offer a powerful visual representation of user behavior on your website. These tools go beyond simple numerical data, providing a richer understanding of how users interact with your pages.Heatmaps visually represent user activity by showing areas of high and low interaction on a webpage. For example, a heatmap might highlight areas of a landing page that receive the most clicks or where users spend the most time.
This information can be invaluable in optimizing page layout and content placement.Session recordings capture a video replay of a user’s browsing session, showing exactly how they navigate your site, what elements they interact with, and where they encounter friction. These recordings provide invaluable qualitative data to complement quantitative metrics. For instance, a session recording might reveal a user struggling to find a specific piece of information, highlighting a potential usability problem.
Using Website Analytics to Improve User Experience and Conversion Rates
Website analytics data provides the foundation for iterative improvements. By analyzing data points such as those mentioned previously, coupled with heatmaps and session recordings, you can identify areas for optimization. This might involve:
- Improving Website Navigation: Simplifying navigation menus, improving internal linking, and creating clearer calls to action can significantly reduce bounce rates and increase engagement.
- Optimizing Content: Analyzing which content resonates best with users (based on time spent on page, scroll depth, etc.) helps in tailoring future content to meet user needs and preferences.
- Enhancing Website Design: Heatmaps can pinpoint areas of low engagement, suggesting design changes to improve user flow and draw attention to key elements.
- A/B Testing: Conducting A/B tests on different design elements or calls to action helps to identify what works best in converting visitors into customers.
Interpreting Website Bounce Rates and Exit Pages to Optimize Content
High bounce rates and specific exit pages often indicate underlying issues. A high bounce rate on a specific landing page might suggest the page isn’t effectively communicating its value proposition or is poorly optimized for search engines. Similarly, a high exit rate on a specific page within a conversion funnel might indicate a problem with that particular step in the process.
By identifying these problematic areas, you can refine content, improve design, or simplify the user journey to reduce bounce rates and improve conversions.
Step-by-Step Guide on Using Website Analytics to Identify Areas for Improvement
Optimizing your website based on analytics is an iterative process. Here’s a step-by-step guide:
- Set Clear Goals: Define what you want to achieve with your website (e.g., increase sales, generate leads, improve brand awareness).
- Choose the Right Analytics Tools: Select a web analytics platform (like Google Analytics) that aligns with your needs and goals.
- Identify Key Metrics: Focus on metrics relevant to your goals (e.g., conversion rate, bounce rate, average session duration).
- Analyze Data: Regularly review your website analytics data to identify trends and patterns.
- Use Heatmaps and Session Recordings: Leverage these tools to gain a deeper understanding of user behavior.
- Identify Areas for Improvement: Based on your analysis, pinpoint specific areas on your website that need optimization.
- Implement Changes: Make necessary changes to your website design, content, or user flow.
- Monitor Results: Track your key metrics after implementing changes to measure their effectiveness.
- Iterate: Continuously analyze data, make improvements, and repeat the process to continuously refine your website.
Predictive Modeling and Future Behavior: Unleashing The Power Of Behavior Analytics To Improve Marketing Efforts
Predictive modeling is the key to unlocking the true potential of behavioral analytics in marketing. By analyzing past customer behavior and other relevant data, we can build models that anticipate future actions, allowing for proactive and personalized marketing strategies. This moves us beyond simply reacting to customer behavior and into a realm of preemptive engagement and optimization.Predictive modeling allows marketers to move from reactive to proactive strategies.
Instead of simply analyzing past performance, we can use data to forecast future trends and tailor our marketing efforts accordingly. This leads to more efficient resource allocation and a higher return on investment.
Anticipating Customer Needs with Predictive Modeling
Predictive models leverage historical data, such as past purchases, website interactions, and demographic information, to identify patterns and predict future customer needs. For example, a model might predict that customers who recently purchased gardening supplies are likely to be interested in related products like fertilizers or gardening tools in the near future. This allows marketers to proactively offer relevant product recommendations or targeted promotions.
A retailer could use a model trained on purchase history and browsing behavior to anticipate the demand for specific items during peak seasons, allowing for efficient inventory management and preventing stockouts.
Enhancing Marketing Campaign Effectiveness with Predictive Modeling
Predictive modeling significantly improves the effectiveness of marketing campaigns by identifying the most responsive customer segments. Instead of broadcasting a message to a large, generic audience, marketers can use predictive models to segment customers based on their likelihood to engage with specific offers. For instance, a model might identify a group of customers with a high probability of responding positively to a discount offer, allowing for targeted email campaigns with a much higher conversion rate than a mass email blast.
Similarly, a model could predict which customers are most likely to click on a specific ad creative, optimizing ad spend and improving click-through rates. Consider a financial institution using a model to predict which customers are most likely to take out a loan; this allows them to focus their loan advertising on those most likely to convert.
Identifying Potential Churn Risks with Predictive Analytics
Customer churn is a significant concern for businesses. Predictive models can identify customers at high risk of churning based on their behavior. For example, a model might flag customers who have significantly reduced their purchase frequency, haven’t engaged with marketing emails recently, or have expressed negative feedback. Early identification of at-risk customers allows businesses to implement retention strategies, such as personalized offers, targeted communication, or proactive customer service interventions.
A SaaS company might use a model to identify customers who haven’t logged in for a certain period or haven’t used key features, prompting them with helpful tutorials or personalized onboarding to encourage continued use.
Personalizing the Customer Journey with Predictive Models
Predictive models enable highly personalized customer experiences. By analyzing individual customer data, models can predict preferences and tailor interactions accordingly. This could involve recommending relevant products, providing customized content, or adjusting the timing and frequency of marketing communications. For example, an e-commerce platform could use a model to recommend products based on a customer’s browsing history and past purchases, leading to increased sales and customer satisfaction.
A streaming service could use a model to predict which shows a customer will enjoy based on their viewing history, resulting in increased engagement and retention.
Building and Deploying a Predictive Model for Marketing
The following flowchart illustrates the process:“`[Start] –> [Data Collection & Preparation] –> [Feature Engineering] –> [Model Selection] –> [Model Training & Evaluation] –> [Model Deployment] –> [Monitoring & Refinement] –> [End]“`Data Collection & Preparation involves gathering relevant data (purchase history, website activity, demographics, etc.) and cleaning it to ensure accuracy. Feature Engineering transforms raw data into features that the model can use (e.g., creating a “days since last purchase” feature).
Model Selection involves choosing an appropriate algorithm (e.g., logistic regression, decision tree, random forest). Model Training & Evaluation involves training the model on historical data and evaluating its performance. Model Deployment involves integrating the model into marketing systems. Monitoring & Refinement involves continuously monitoring the model’s performance and retraining it as needed.
Measuring the Impact of Behavioral Analytics
So, you’ve implemented behavioral analytics into your marketing strategy. That’s fantastic! But now comes the crucial part: proving its value. Measuring the impact of your behavioral analytics initiatives isn’t just about justifying the investment; it’s about refining your strategies and maximizing your return. This means understanding which metrics matter most and how to link them directly to your marketing successes.
Effectively measuring the impact of behavioral analytics requires a multi-faceted approach. It’s not enough to simply track website visits; you need to connect those visits to tangible business outcomes. This involves carefully selecting relevant KPIs, employing robust attribution models, and regularly analyzing your data to identify trends and areas for improvement. By doing so, you can demonstrate the clear and quantifiable benefits of your behavioral-driven marketing efforts.
Key Performance Indicators (KPIs) for Behavioral-Driven Marketing, Unleashing the power of behavior analytics to improve marketing efforts
Understanding which KPIs to track is paramount. Focusing on the wrong metrics can lead to misinterpretations and wasted resources. The most relevant KPIs will depend on your specific marketing objectives, but some common and effective ones include:
- Conversion Rate: This measures the percentage of website visitors who complete a desired action, such as making a purchase, signing up for a newsletter, or filling out a contact form. A rise in conversion rate directly indicates the effectiveness of behavioral targeting in guiding users towards desired actions.
- Customer Lifetime Value (CLTV): This metric represents the total revenue a customer is expected to generate throughout their relationship with your business. Behavioral analytics can help identify high-value customers and tailor strategies to retain them, ultimately boosting CLTV.
- Customer Acquisition Cost (CAC): This measures the cost of acquiring a new customer. By optimizing marketing campaigns based on behavioral insights, you can reduce CAC and improve efficiency.
- Website Engagement Metrics: These include metrics like bounce rate, time on site, pages per visit, and scroll depth. Improvements in these metrics suggest that your content and website design are resonating better with your target audience, leading to improved engagement and ultimately, conversions.
- Return on Ad Spend (ROAS): This crucial metric measures the revenue generated for every dollar spent on advertising. Behavioral targeting allows for more precise ad delivery, leading to higher ROAS.
Attribution Models for Measuring Marketing ROI
Attributing marketing ROI to behavioral analytics initiatives requires a well-defined attribution model. Several approaches exist, each with its strengths and weaknesses:
- Last-Click Attribution: This simple model attributes the entire conversion to the last marketing touchpoint. While easy to understand, it overlooks the influence of earlier interactions.
- First-Click Attribution: This model attributes the conversion to the first interaction a customer had with your brand. Like last-click, it oversimplifies the customer journey.
- Multi-Touch Attribution (MTA): This more sophisticated model distributes credit for a conversion across multiple touchpoints, acknowledging the influence of various marketing activities. MTA models, such as linear, time decay, and position-based, provide a more holistic view of the customer journey and allow for a more accurate assessment of the impact of behavioral analytics.
Choosing the right attribution model is crucial for accurate ROI measurement. MTA models, while more complex, offer a more realistic representation of the customer journey and the impact of behavioral analytics.
Comparing Approaches to Measuring Impact
Different approaches to measuring the impact of behavioral analytics exist, ranging from simple A/B testing to more advanced statistical modeling. A/B testing allows for direct comparison of different versions of marketing materials or website designs, while statistical modeling can uncover complex relationships between behavioral data and marketing outcomes. The choice of approach depends on the complexity of the marketing campaign and the available resources.
For instance, a simple email campaign might benefit from A/B testing, while a large-scale omnichannel campaign might require more sophisticated statistical modeling.
Successful Case Studies
Many companies have successfully leveraged behavioral analytics to improve marketing outcomes. For example, Amazon uses behavioral data to personalize product recommendations, significantly increasing sales. Netflix uses viewing history to suggest shows and movies, leading to higher user engagement and retention. These examples demonstrate the power of behavioral analytics in driving tangible business results.
Actionable Steps for Implementing and Measuring Behaviorally-Driven Marketing
To effectively implement and measure behaviorally-driven marketing strategies, consider these steps:
- Define Clear Objectives: Establish specific, measurable, achievable, relevant, and time-bound (SMART) goals for your behavioral marketing initiatives.
- Choose the Right Tools: Select analytics platforms and tools that align with your needs and budget.
- Collect and Analyze Data: Gather relevant behavioral data from various sources and analyze it to identify patterns and insights.
- Develop Targeted Strategies: Create marketing campaigns and website experiences tailored to specific customer segments based on their behavior.
- Implement A/B Testing: Continuously test and optimize your strategies to improve their effectiveness.
- Track and Measure KPIs: Monitor key performance indicators to assess the impact of your initiatives.
- Refine and Iterate: Regularly review your results, identify areas for improvement, and adjust your strategies accordingly.
Final Thoughts
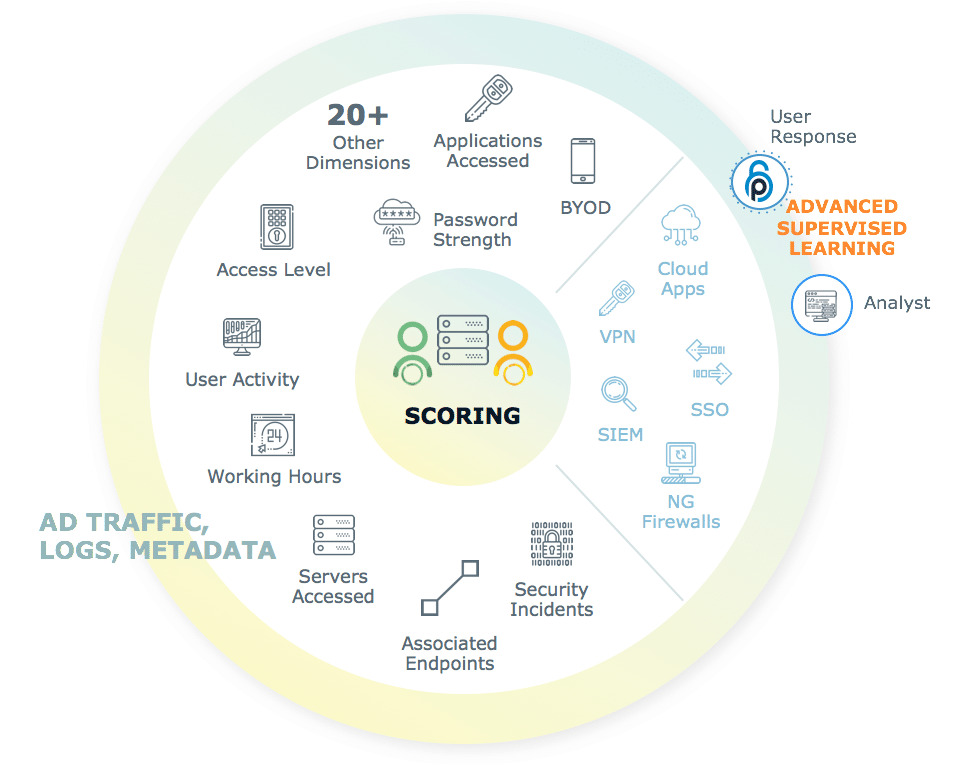
Mastering the art of behavior analytics isn’t just about collecting data; it’s about using that data to build stronger relationships with your customers and drive meaningful business outcomes. By understanding the nuances of customer behavior, you can personalize your marketing efforts, optimize your website experience, and predict future trends. This allows for more effective resource allocation, reduced marketing waste, and a more targeted approach to reaching your ideal customers.
The journey to unlocking the true power of behavior analytics requires a commitment to data-driven decision-making and a willingness to adapt your strategies based on real-time insights. So, start analyzing, start personalizing, and start seeing the incredible results behavior analytics can deliver.
FAQ Compilation
What are some common mistakes businesses make when using behavior analytics?
Common mistakes include failing to define clear objectives, ignoring ethical considerations, misinterpreting data, and not integrating analytics into the overall marketing strategy.
How much does behavior analytics software cost?
The cost varies greatly depending on the features, scale, and vendor. Options range from free tools with limited functionality to enterprise-level solutions with substantial pricing.
What if I don’t have a lot of data to analyze?
Even with limited data, you can still gain valuable insights. Focus on the most crucial metrics and use available data to identify initial trends and areas for improvement. As your data volume increases, your analysis will become more sophisticated.
How can I ensure the ethical use of customer data?
Prioritize transparency with your customers, obtain explicit consent for data collection, ensure data security, and comply with all relevant privacy regulations (like GDPR and CCPA).