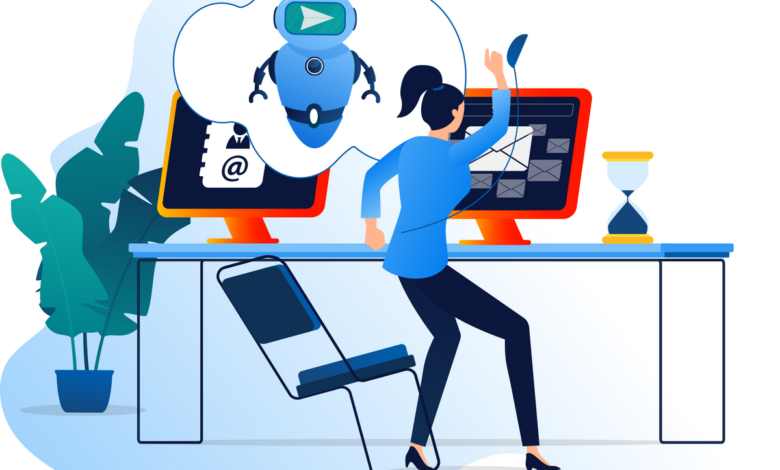
Using AI and RPA to Accelerate Digital Transformation
Using AI and RPA to accelerate digital transformation is no longer a futuristic concept; it’s the present reality for businesses aiming to thrive. This powerful combination automates tasks, improves decision-making, and ultimately, helps companies leapfrog their competition. We’ll explore how AI’s predictive power and RPA’s tireless efficiency converge to reshape industries, from streamlining customer service to optimizing complex financial processes.
Get ready to dive into the exciting world of intelligent automation!
This post will unpack the synergistic relationship between AI and RPA, exploring real-world examples and addressing common challenges. We’ll cover everything from defining digital transformation in this context to outlining the steps for successful implementation, including the crucial considerations for data security and ethical implications. Whether you’re a seasoned tech professional or just starting to explore the potential of AI and RPA, this guide will provide valuable insights and practical advice.
Defining Digital Transformation with AI and RPA
Digital transformation is more than just adopting new technologies; it’s a fundamental shift in how businesses operate, driven by the need to improve efficiency, agility, and customer experience in an increasingly digital world. It involves rethinking processes, adopting new technologies, and changing the culture of an organization to embrace innovation. This transformation isn’t a one-time event but an ongoing journey of continuous improvement.AI and RPA are powerful catalysts accelerating this journey.
They automate tasks, analyze data to reveal insights, and improve decision-making, allowing businesses to achieve their digital transformation goals much faster than traditional methods. The synergy between these two technologies is particularly potent.
Core Components of Digital Transformation
Digital transformation encompasses several key areas. These include process optimization, data management and analytics, customer experience enhancement, workforce enablement, and the adoption of emerging technologies like AI and RPA. Successful transformation requires a holistic approach, addressing all these components simultaneously to achieve maximum impact. Ignoring any one area can limit the overall success of the initiative. For example, optimizing processes without addressing data management can lead to inefficient use of information, hindering the full potential of the transformation.
AI and RPA’s Contribution to Faster Digital Transformation
AI, with its capabilities in machine learning and deep learning, empowers businesses to analyze vast datasets, predict future trends, and automate complex decision-making processes. RPA, on the other hand, excels at automating repetitive, rule-based tasks, freeing up human employees to focus on more strategic and creative work. The combination of AI’s analytical power and RPA’s automation capabilities allows for a significantly faster and more efficient transformation.
AI can identify areas ripe for automation, while RPA executes the automation, creating a virtuous cycle of continuous improvement.
Benefits of Integrating AI and RPA
Integrating AI and RPA offers numerous benefits. Cost reduction is a significant advantage, as automation reduces labor costs and improves operational efficiency. Improved accuracy and reduced error rates are also key benefits, as AI-powered systems make fewer mistakes than humans performing repetitive tasks. Enhanced customer experience is another significant outcome, as faster and more efficient processes lead to better service and increased customer satisfaction.
Finally, increased agility and adaptability allow businesses to respond more quickly to changing market conditions and customer demands.
Examples of AI and RPA in Digital Transformation
The successful integration of AI and RPA is evident across numerous industries. The following table illustrates some examples:
Industry | AI Application | RPA Application | Transformation Outcome |
---|---|---|---|
Finance | Fraud detection, risk assessment, algorithmic trading | Account reconciliation, loan processing, KYC/AML compliance | Reduced costs, improved accuracy, faster processing times |
Healthcare | Medical image analysis, disease prediction, personalized medicine | Patient data entry, appointment scheduling, claims processing | Improved patient care, reduced administrative burden, faster diagnosis |
Manufacturing | Predictive maintenance, quality control, supply chain optimization | Inventory management, order processing, production scheduling | Increased efficiency, reduced downtime, improved product quality |
Retail | Personalized recommendations, customer segmentation, demand forecasting | Order fulfillment, inventory management, customer service | Improved customer experience, increased sales, optimized inventory |
AI’s Role in Accelerating Digital Transformation
AI is no longer a futuristic concept; it’s a powerful engine driving today’s digital transformation. Its ability to automate tasks, analyze vast datasets, and learn from experience allows businesses to operate more efficiently, make smarter decisions, and ultimately, gain a competitive edge. This section will explore the multifaceted ways AI accelerates digital transformation, focusing on key applications and their impact.
AI-Driven Automation of Decision-Making Processes
AI significantly streamlines decision-making by automating processes that previously relied on human judgment. Machine learning algorithms, trained on historical data and real-time inputs, can analyze complex situations, identify patterns, and recommend optimal courses of action. This automation reduces human error, speeds up decision cycles, and frees up human resources for more strategic tasks. For instance, in fraud detection, AI algorithms can analyze transaction data in real-time to identify suspicious patterns and automatically flag them for review, significantly reducing losses and improving response times.
This surpasses human capability in speed and scale of analysis.
AI’s Capabilities in Predictive Analytics for Business Improvement
Predictive analytics, powered by AI, provides businesses with the ability to anticipate future trends and outcomes. By analyzing historical data, market trends, and other relevant information, AI algorithms can generate accurate forecasts for sales, customer churn, equipment failure, and more. This foresight allows businesses to proactively adjust their strategies, optimize resource allocation, and mitigate potential risks. For example, a retail company might use AI to predict seasonal demand fluctuations, allowing them to optimize inventory levels and avoid stockouts or overstocking, leading to significant cost savings.
AI-Powered Chatbots for Enhanced Customer Service
AI-powered chatbots are revolutionizing customer service by providing instant, 24/7 support. These chatbots can handle a wide range of inquiries, from simple questions about product information to complex troubleshooting issues. They learn and improve over time, becoming increasingly adept at understanding and responding to customer needs. This leads to improved customer satisfaction, reduced wait times, and increased efficiency for customer service teams.
Companies like Amazon and Sephora utilize AI-powered chatbots extensively, handling a large volume of customer queries and freeing up human agents to deal with more complex issues.
AI Streamlining a Business Process: Scenario – Supply Chain Optimization
Consider a large manufacturing company facing challenges in supply chain management. Inefficient processes lead to delays, increased costs, and stockouts. By implementing an AI-powered system, the company can analyze real-time data from various sources – including supplier performance, inventory levels, transportation logistics, and demand forecasts – to optimize the entire supply chain. The AI system can predict potential disruptions, suggest alternative routes, optimize inventory levels, and even automate ordering processes.
This results in reduced lead times, lower inventory costs, improved on-time delivery rates, and increased customer satisfaction. The AI system continuously learns and adapts to changing conditions, making the supply chain more resilient and efficient. This scenario illustrates how AI can transform a complex business process, creating significant value and competitive advantage.
RPA’s Contribution to Digital Transformation Speed
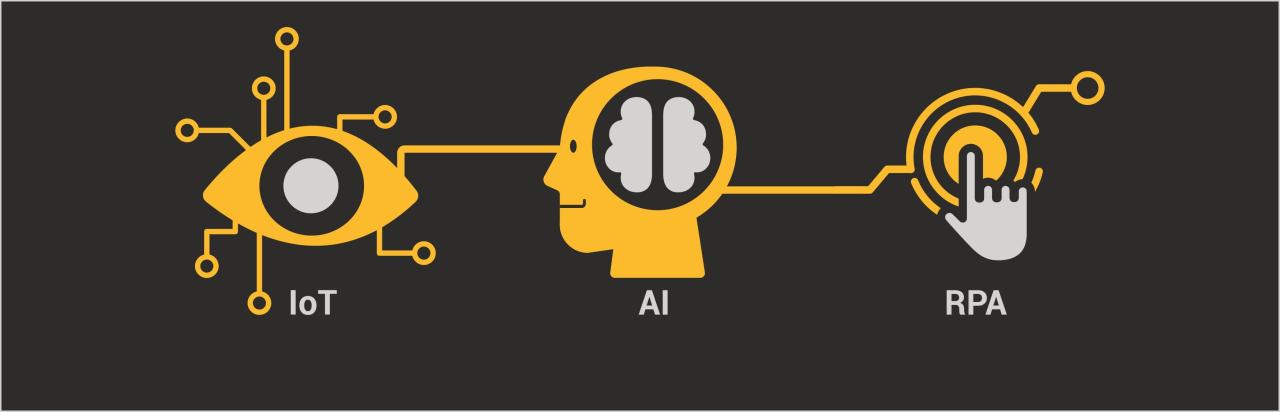
Robotic Process Automation (RPA) is a game-changer in the pursuit of faster digital transformation. It allows businesses to significantly accelerate their progress by automating repetitive, rule-based tasks, freeing up valuable human resources for more strategic and creative endeavors. This ultimately leads to increased efficiency, reduced costs, and improved accuracy across the organization.RPA handles repetitive tasks by mimicking human actions interacting with digital systems.
It can automate data entry, process invoices, extract information from documents, and much more, all without human intervention. This frees up employees from tedious, time-consuming work, allowing them to focus on higher-value activities that require human judgment, critical thinking, and creativity. The result is a more engaged and productive workforce.
RPA Applications Across Departments
The versatility of RPA makes it applicable across numerous departments. Consider the following examples:
- Finance: RPA can automate tasks like invoice processing, reconciliation, and financial reporting, significantly reducing processing time and minimizing errors. Imagine a scenario where an RPA bot automatically extracts invoice data from emails, verifies it against purchase orders, and then updates the accounting system – a task that previously might have taken hours for a human employee can now be completed in minutes.
Automating processes with AI and RPA is key to speeding up digital transformation, but building the right apps is crucial. To efficiently create these applications, exploring platforms like Domino’s low-code/pro-code approach, as detailed in this insightful article domino app dev the low code and pro code future , is a game-changer. Ultimately, leveraging both powerful AI/RPA tools and efficient development methods ensures a faster, smoother digital transformation journey.
- Human Resources (HR): RPA can streamline onboarding processes by automatically collecting and verifying employee data, generating offer letters, and managing employee records. This reduces administrative burden on HR staff, allowing them to focus on strategic initiatives such as talent acquisition and employee engagement. For example, an RPA bot can automatically populate new hire information into various HR systems, eliminating manual data entry and ensuring consistency.
- Customer Service: RPA can automate responses to frequently asked questions, track customer interactions, and escalate complex issues to human agents. This improves customer satisfaction by providing faster response times and consistent service levels. A chatbot integrated with an RPA system could handle simple inquiries, freeing up human agents to address more challenging customer needs.
Challenges in RPA Implementation and Solutions
While RPA offers substantial benefits, implementation can present challenges.
- Integration complexities: Integrating RPA with existing systems can be complex, requiring careful planning and potentially custom development. Solution: Thorough system analysis and a phased implementation approach can mitigate these complexities.
- Legacy system limitations: RPA may struggle with outdated or poorly documented systems. Solution: Prioritize system modernization efforts alongside RPA implementation to ensure smooth integration.
- Change management: Employees may resist RPA adoption due to concerns about job security. Solution: Open communication, training, and reskilling initiatives are crucial to address these concerns and foster buy-in.
Comparison of RPA and Traditional Automation Methods
RPA offers distinct advantages over traditional automation methods.
- Traditional Automation (e.g., scripting, macros): Often requires significant coding expertise, is inflexible and difficult to adapt to changing processes, and is typically limited to specific applications.
- RPA: Utilizes a visual, low-code/no-code approach, making it easier to implement and maintain. It’s more adaptable to process changes and can integrate with multiple applications, offering greater flexibility and scalability.
“RPA provides a faster, more cost-effective, and less disruptive approach to automation than traditional methods, particularly for businesses with legacy systems or complex processes.”
Synergistic Effects of AI and RPA: Using Ai And Rpa To Accelerate Digital Transformation
AI and Robotic Process Automation (RPA) are powerful technologies individually, but their combined strength truly revolutionizes digital transformation. When integrated, they create a synergistic effect, exceeding the sum of their individual capabilities and driving unprecedented efficiency gains. This powerful combination allows businesses to automate complex processes that were previously impossible to automate with either technology alone.AI significantly enhances RPA by providing the intelligence needed to handle exceptions, learn from data, and adapt to changing circumstances.
RPA, in turn, provides the tireless execution engine to carry out the tasks directed by AI, handling large volumes of data with speed and accuracy. This partnership is essential for achieving true intelligent automation.
AI-Enhanced RPA Efficiency
The core strength of the AI-RPA synergy lies in the ability to handle complex, unstructured data. RPA excels at structured, repetitive tasks, but struggles with variations or exceptions. AI, through techniques like machine learning and natural language processing (NLP), can analyze and interpret unstructured data, making decisions and guiding RPA in handling exceptions and unpredictable situations. For example, an AI-powered RPA system processing invoices could identify and flag unusual amounts or discrepancies, routing them for human review only when necessary.
This dramatically reduces human intervention, freeing up valuable human resources for more strategic tasks.
Case Study: Fraud Detection in Financial Services
A large financial institution implemented an AI-powered RPA solution to detect fraudulent transactions. The RPA component processed vast volumes of transaction data, identifying potential anomalies based on pre-defined rules. However, the real power came from the integrated AI. The AI component, using machine learning algorithms, learned from historical fraud patterns and identified subtle anomalies that the rule-based RPA system might have missed.
This proactive approach significantly improved fraud detection rates, minimizing financial losses and enhancing the security of the financial institution. The system not only detected fraud more effectively but also reduced the time spent investigating potential fraudulent activity, saving considerable resources and improving overall operational efficiency.
Improving RPA Processes Through Intelligent Automation
AI can improve RPA processes in several key ways. First, it allows for more intelligent decision-making within the automation process, reducing the need for human intervention. Second, AI enables RPA to handle unstructured data, expanding the range of automatable tasks. Third, AI facilitates self-learning and adaptation within RPA, enabling the system to continuously improve its performance over time. For instance, an RPA system using AI-powered OCR can extract data from invoices of varying formats with greater accuracy than a traditional RPA system, improving data entry speed and reducing errors.
Moreover, AI can help RPA systems prioritize tasks based on urgency and importance, optimizing workflow and resource allocation.
Implementing an AI-Powered RPA Solution: A Step-by-Step Guide
Implementing an AI-powered RPA solution requires a structured approach.
- Needs Assessment and Process Selection: Identify processes suitable for automation, focusing on those involving high volumes of repetitive tasks and unstructured data. Prioritize processes with the greatest potential for efficiency gains.
- Technology Selection: Choose appropriate AI and RPA tools based on specific needs and budget constraints. Consider factors such as scalability, integration capabilities, and ease of use.
- Data Preparation and Training: Prepare and clean the data required for AI training. The quality of the training data directly impacts the accuracy and effectiveness of the AI model.
- AI Model Development and Testing: Develop and rigorously test the AI model to ensure accuracy and reliability. Iterative refinement is crucial for optimal performance.
- RPA Workflow Design and Integration: Design the RPA workflow, integrating the AI model to handle exceptions and unstructured data. Thorough testing is critical to ensure seamless integration.
- Deployment and Monitoring: Deploy the AI-powered RPA solution and continuously monitor its performance. Regular adjustments and updates are necessary to maintain optimal efficiency and accuracy.
Challenges and Considerations in AI/RPA Implementation
Embarking on the journey of integrating AI and RPA to accelerate digital transformation is exciting, but it’s crucial to acknowledge the potential hurdles. A successful implementation requires careful planning, resource allocation, and a realistic understanding of the challenges involved. Ignoring these aspects can lead to project delays, cost overruns, and ultimately, failure to achieve the desired benefits.
Potential Risks Associated with AI and RPA Adoption, Using ai and rpa to accelerate digital transformation
Implementing AI and RPA involves inherent risks that need careful mitigation. These risks range from technical challenges to organizational and ethical considerations. For example, the initial investment in software, hardware, and training can be substantial. Furthermore, the integration process itself can be complex, requiring significant time and effort to ensure seamless interaction between systems. Poorly integrated systems can lead to data inconsistencies and operational inefficiencies, negating the intended benefits.
Another key risk is the potential for unforeseen errors in AI algorithms, which could lead to incorrect decisions or actions. Finally, the dependence on technology introduces vulnerability to cyberattacks and data breaches, necessitating robust security measures.
Data Security and Privacy Concerns in AI/RPA Deployment
The use of AI and RPA often involves processing large volumes of sensitive data. Protecting this data is paramount. Robust security measures, including data encryption, access control, and regular security audits, are essential. Compliance with relevant data privacy regulations, such as GDPR and CCPA, is also crucial. Failure to adequately address data security and privacy can result in significant legal and reputational damage.
Consider, for instance, a healthcare provider using AI/RPA to process patient records. A data breach could expose sensitive patient information, leading to legal action and loss of patient trust.
The Need for Skilled Professionals in AI and RPA
Successful AI/RPA implementation relies heavily on skilled professionals. These individuals need expertise in areas such as AI algorithm development, RPA software configuration, data analysis, and cybersecurity. A shortage of skilled professionals can hinder project timelines and increase implementation costs. Furthermore, effective training programs are needed to upskill existing employees and attract new talent. Companies often struggle to find individuals with the necessary combination of technical and business skills to effectively manage and maintain AI/RPA systems.
This skills gap necessitates proactive investment in training and development initiatives.
Decision-Making Process for AI/RPA Integration
The decision to integrate AI and RPA should be a carefully considered process. A structured approach, such as the one illustrated below, can help organizations make informed decisions.
[Start] | V Is there a clearly defined business problem?| Yes --------|--------> Proceed to feasibility assessment | No --------|--------> Re-evaluate business needs | V Feasibility Assessment (Cost-benefit analysis, resource availability, risk assessment) | V Is the project feasible?
| Yes --------|--------> Develop a detailed implementation plan | No --------|--------> Re-evaluate or abandon the project | V Implementation (Development, testing, deployment, monitoring) | V Post-implementation review and optimization | V [End]
This flowchart provides a simplified overview; each stage would typically involve more detailed sub-steps.
The feasibility assessment, for example, might include a detailed cost-benefit analysis, risk assessment, and evaluation of the available resources and expertise.
Future Trends in AI and RPA for Digital Transformation
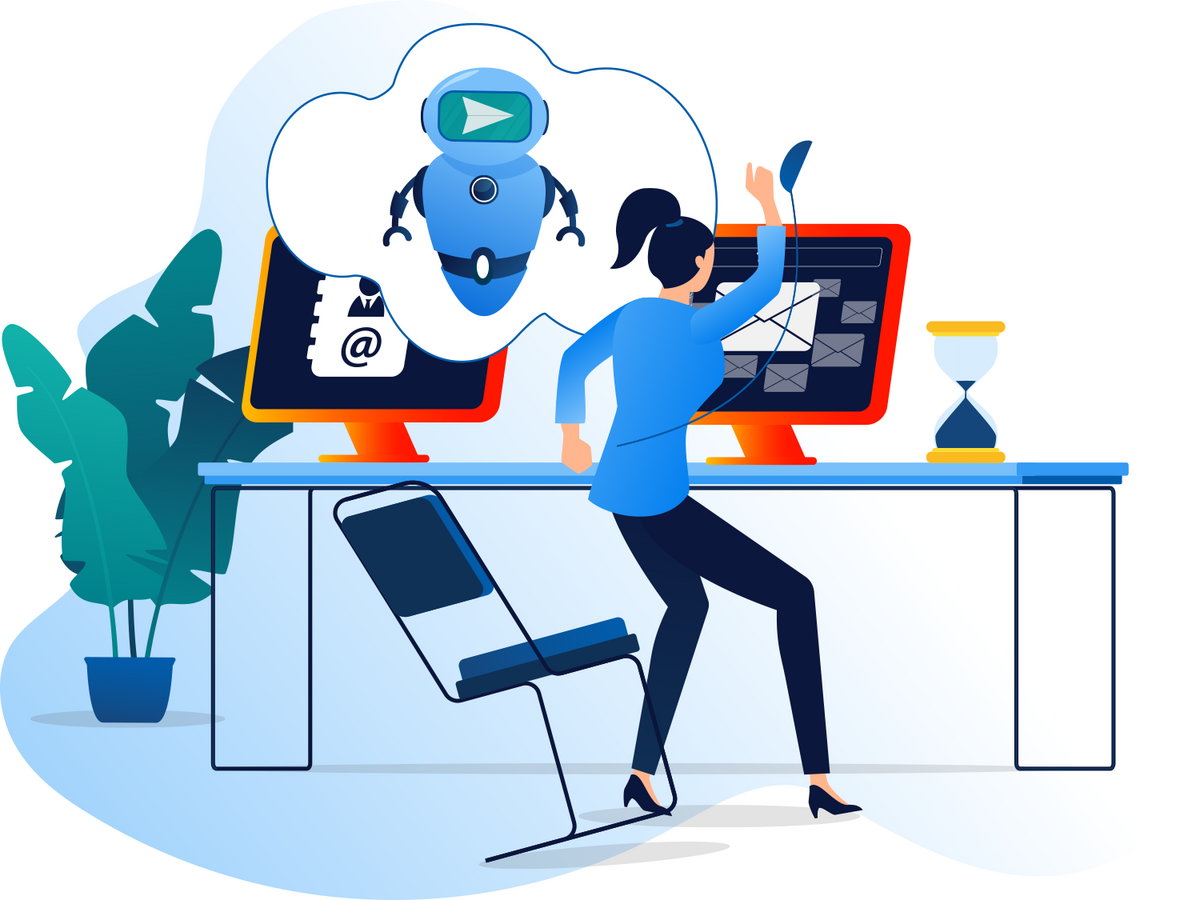
The convergence of Artificial Intelligence (AI) and Robotic Process Automation (RPA) is rapidly reshaping the landscape of digital transformation. Looking ahead, we can expect even more profound impacts on business operations, driven by technological advancements and a deeper understanding of the ethical considerations involved. This section explores some key future trends and their implications.
Hyperautomation and Intelligent Automation
The future of AI and RPA lies in their seamless integration, creating what’s known as hyperautomation. This goes beyond simply automating individual tasks; it involves orchestrating complex workflows that combine AI’s cognitive abilities with RPA’s execution capabilities. Intelligent automation will enable businesses to automate end-to-end processes, significantly increasing efficiency and reducing human error. For instance, a hyperautomated system could handle everything from receiving an order, verifying customer information, processing payments, to scheduling shipping, all with minimal human intervention.
This level of automation requires sophisticated AI models capable of decision-making and problem-solving within the automated workflow.
Emerging Technologies Impacting AI and RPA Adoption
Several emerging technologies are poised to significantly impact the adoption and capabilities of AI and RPA. These include advancements in natural language processing (NLP), allowing for more sophisticated interactions with humans and data; computer vision, enabling RPA to interact with more complex visual interfaces; and blockchain technology, offering increased security and transparency in automated processes. The rise of edge computing will also allow for faster processing and reduced latency, improving the responsiveness of AI-powered RPA systems.
For example, imagine a manufacturing plant using edge computing to deploy AI-powered quality control checks in real-time, minimizing production delays and improving product quality.
Ethical Considerations in AI/RPA Implementation
The increasing reliance on AI and RPA raises important ethical considerations. Bias in AI algorithms, leading to unfair or discriminatory outcomes, is a major concern. Ensuring data privacy and security within automated systems is also crucial. Transparency and explainability in AI decision-making are paramount, allowing humans to understand and audit the automated processes. Job displacement due to automation needs careful consideration and proactive measures, such as reskilling and upskilling initiatives, must be implemented.
Regulations and guidelines are being developed to address these ethical concerns, but continuous monitoring and adaptation are necessary. For instance, the EU’s General Data Protection Regulation (GDPR) already sets strict standards for data handling, impacting the implementation of AI and RPA systems that process personal data.
Innovative Applications of AI and RPA in Digital Transformation
The combined power of AI and RPA is unlocking innovative applications across various industries.
- Intelligent Customer Service: AI-powered chatbots and virtual assistants handle routine customer inquiries, freeing up human agents to focus on complex issues. RPA can automate back-office tasks related to customer service, such as order processing and account updates.
- Predictive Maintenance in Manufacturing: AI analyzes sensor data from machinery to predict potential failures, allowing for proactive maintenance and reducing downtime. RPA automates the scheduling and execution of maintenance tasks.
- Fraud Detection in Finance: AI algorithms analyze transaction data to identify suspicious patterns and flag potential fraud. RPA automates the investigation and reporting processes.
- Personalized Healthcare: AI analyzes patient data to personalize treatment plans and predict potential health risks. RPA automates administrative tasks, such as scheduling appointments and managing medical records.
Wrap-Up

In short, the fusion of AI and RPA offers a transformative path to accelerate digital transformation. By intelligently automating tasks and enhancing decision-making, businesses can unlock unprecedented levels of efficiency, improve customer experiences, and gain a significant competitive edge. While challenges exist, the potential rewards far outweigh the risks, making it a strategic imperative for organizations across all sectors. Embracing this technological synergy is not just about keeping up; it’s about leading the charge into a future defined by intelligent automation.
Q&A
What are the initial costs associated with implementing AI and RPA?
Initial costs vary greatly depending on the complexity of the implementation, the scale of the project, and the specific software and infrastructure required. Expect costs associated with software licenses, consulting fees, integration with existing systems, and employee training.
How long does it typically take to see a return on investment (ROI) from AI and RPA?
The ROI timeline depends on factors such as project scope and complexity. While some projects show quick returns, others may take longer to realize the full benefits. Careful planning and a phased approach can help accelerate ROI.
What are the key metrics for measuring the success of AI and RPA implementation?
Key metrics include increased efficiency (e.g., reduced processing time), improved accuracy, cost savings, enhanced customer satisfaction, and improved employee productivity. Regular monitoring and analysis of these metrics are crucial.
What skills are needed for a successful AI and RPA implementation team?
A successful team requires a blend of technical expertise (AI/ML, RPA development, data science) and business acumen. Strong project management skills and a collaborative approach are also essential.