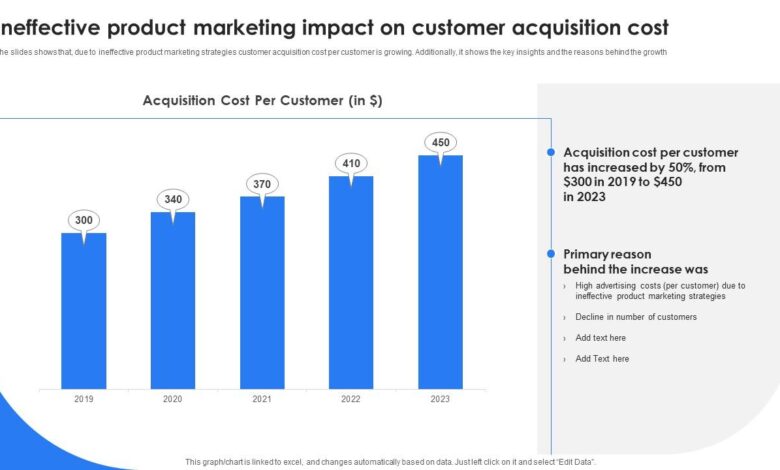
Why Your Product Recommendations Arent Working
Why your product recommendations arent working – Why your product recommendations aren’t working? It’s a question plaguing many businesses, and the answer isn’t always straightforward. It’s a complex puzzle with pieces ranging from flawed algorithms and inaccurate data to poor user experience and ineffective product presentation. This post dives deep into the common culprits, offering actionable insights to boost your recommendation engine’s performance and drive more sales.
From understanding the crucial role of data accuracy – think complete purchase histories and detailed browsing behavior – to mastering the nuances of different recommendation algorithms (collaborative filtering, anyone?), we’ll unpack the key factors that influence the success (or failure) of your recommendations. We’ll even explore how to use A/B testing to continuously optimize your approach, ensuring your recommendations are always on point.
Data Accuracy and Completeness
Getting accurate product recommendations relies heavily on having accurate and complete user data. Inaccurate or incomplete data leads to irrelevant suggestions, frustrating users and ultimately harming your business. Think of it like trying to navigate with a faulty GPS – you’ll likely end up in the wrong place. The more complete and accurate the data, the better the recommendations will be.
Inaccurate user data can manifest in many ways, from incorrect age and location information to misrepresented purchase history or browsing preferences. Even small inaccuracies can have a significant snowball effect, leading to a cascade of irrelevant recommendations. For example, recommending winter coats to someone who lives in a tropical climate based on inaccurate location data is a clear sign of a problem.
Similarly, suggesting products a user has already purchased points to an issue with data synchronization or record-keeping.
Impact of Inaccurate User Data on Recommendation Relevance
Inaccurate data directly impacts the relevance of product recommendations. Recommendation engines rely on algorithms that analyze user data to identify patterns and predict preferences. If the input data is flawed, the algorithms will produce flawed outputs. This results in irrelevant suggestions, a diminished user experience, and potentially lost sales. For example, recommending hiking boots to a user who has only ever purchased dress shoes and formal wear is a clear indicator of inaccurate data interpretation.
The more inaccurate the data, the more likely the recommendations are to miss the mark, leading to user dissatisfaction and decreased engagement.
Crucial Data Points for Effective Product Recommendations, Why your product recommendations arent working
Several data points are crucial for generating effective product recommendations. Their absence significantly impacts the accuracy and relevance of the suggestions. Let’s look at some key examples.
Data Type | Impact on Recommendation Accuracy | Example of Absence Impact | Example of Accurate Data Impact |
---|---|---|---|
Purchase History | High | Without purchase history, recommendations are purely speculative, potentially offering irrelevant items. | Analyzing past purchases allows for recommending similar items or complementary products, increasing the likelihood of a successful recommendation. |
Browsing Behavior | Medium-High | Lack of browsing data limits the ability to understand user interests and preferences, leading to generic and less targeted recommendations. | Tracking browsing behavior reveals specific product categories or individual items a user is interested in, enabling highly personalized recommendations. |
Demographics | Medium | Without demographic data, recommendations may lack personalization and fail to consider factors like age, gender, or location, resulting in less relevant suggestions. | Using demographic data allows for targeted advertising and product recommendations tailored to specific demographics, improving the chances of a successful recommendation. For example, recommending children’s toys to users identified as parents. |
Reviews and Ratings | Medium | Absence of this data prevents the system from understanding user preferences regarding specific product attributes, hindering accurate recommendations. | Analyzing reviews and ratings helps identify popular products and those with specific features users appreciate, leading to more tailored and reliable recommendations. |
Recommendation Algorithm Effectiveness
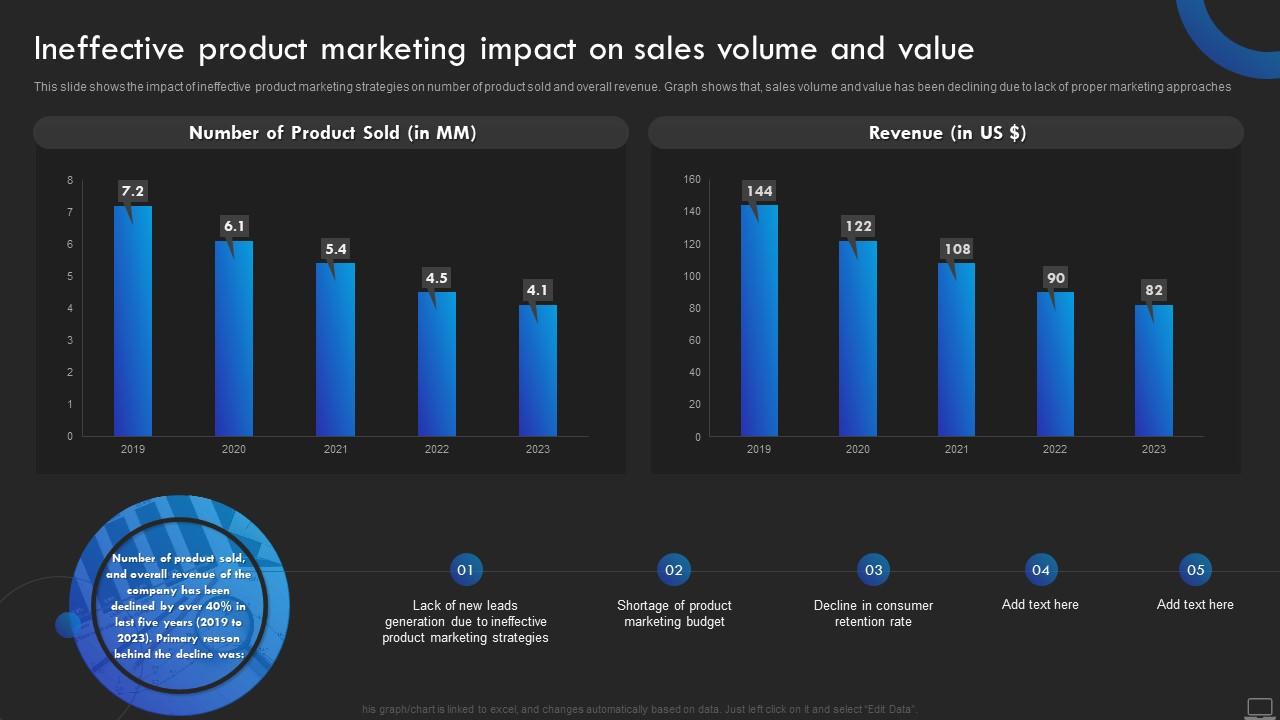
So, we’ve tackled data issues, but our recommendations are still off. The problem might lie not in the data itself, but in how we’re using it. The effectiveness of our recommendation engine hinges heavily on the algorithm powering it. Let’s dive into the heart of the matter: algorithm choice and optimization.
Choosing the right recommendation algorithm is crucial. There are several approaches, each with its strengths and weaknesses, and the best choice often depends on the specific data and user base. Poor performance can often be traced back to an algorithm mismatch or suboptimal tuning.
Comparison of Recommendation Algorithms
Three major types of recommendation algorithms are commonly used: collaborative filtering, content-based filtering, and hybrid approaches. Collaborative filtering relies on user-item interaction data to predict what a user might like based on what similar users have liked. Content-based filtering, on the other hand, focuses on the characteristics of items themselves, recommending items similar to those a user has previously enjoyed.
Hybrid approaches combine elements of both, leveraging the strengths of each to provide more robust recommendations.
Examples of Algorithm Flaws Leading to Poor Recommendations
Let’s look at some concrete examples. A collaborative filtering system might struggle with new users or niche items, as there isn’t enough data to establish meaningful similarities. Imagine a new online bookstore. A collaborative filter would struggle to recommend books to new users since there is no history of their preferences. The system might simply recommend popular books, missing opportunities to connect users with more relevant titles.
Similarly, content-based filtering can lead to a “filter bubble,” recommending only items similar to what a user has already seen, limiting exposure to new genres or perspectives. A user who only reads science fiction might only receive science fiction recommendations, missing out on potentially interesting books in other genres. A poorly designed hybrid system might simply combine the weaknesses of both approaches, leading to even worse results.
Importance of Algorithm Tuning and Retraining
Algorithm tuning and retraining are vital for maintaining optimal performance. Algorithms are not static; they need to adapt to changing user preferences and item catalogs. Regular retraining with updated data ensures the algorithm stays relevant and accurate. For example, seasonal trends greatly impact product popularity. A fashion recommendation engine trained only on past data might recommend winter coats in the summer.
Retraining the model periodically with fresh data accounts for such shifts and ensures recommendations remain relevant and timely. Tuning involves adjusting parameters within the algorithm to optimize its performance on specific metrics, such as precision and recall. Without regular tuning and retraining, the algorithm can become stale and provide increasingly irrelevant recommendations.
User Preferences and Personalization
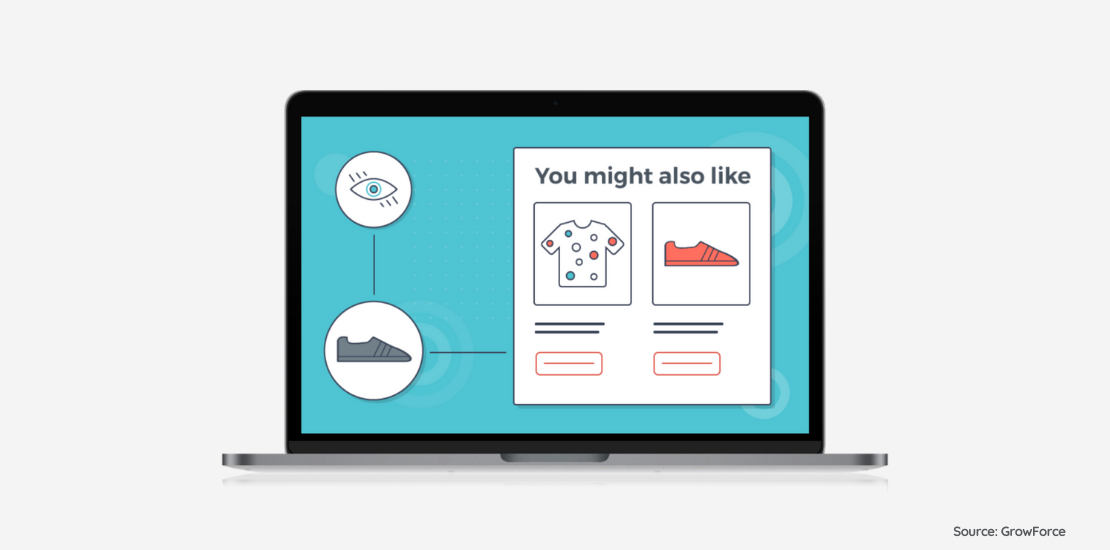
So, we’ve tackled data issues and algorithm tweaks, but our recommendation engine still isn’t hitting the mark. A big reason why could be that we’re not truly understanding and leveraging our users’ preferences. It’s not enough to justhave* data; we need to actively and effectively use it to personalize the experience. This means moving beyond basic demographic information and diving deeper into individual tastes and behaviors.Understanding and accurately reflecting user preferences is crucial for effective product recommendations.
Failing to do so leads to irrelevant suggestions, user frustration, and ultimately, a decline in engagement and conversions. Imagine a user consistently browsing high-end cookware only to receive recommendations for budget-friendly plastic utensils. This disconnect erodes trust and discourages further interaction with the platform. The lack of personalization directly impacts customer satisfaction and, consequently, business success.
Methods for Better Capturing and Utilizing User Preferences
Effective personalization hinges on comprehensive data collection and intelligent analysis. We need to go beyond simple purchase history and explore more nuanced methods to understand our users. This involves actively soliciting feedback, tracking browsing behavior, and utilizing advanced analytics to identify patterns and preferences. For example, analyzing the time spent on product pages can indicate interest level, while comparing browsing patterns across users can reveal hidden affinities.
We should also consider incorporating sentiment analysis from user reviews and social media interactions to gain a richer understanding of user preferences.
Implications of Inadequate Personalization
Inadequate personalization translates directly into missed opportunities. Users bombarded with irrelevant recommendations are more likely to abandon the platform and seek alternatives offering a more tailored experience. This results in decreased engagement, lower conversion rates, and a diminished return on investment in the recommendation system. Moreover, poor personalization can damage brand reputation, as users perceive a lack of understanding and attention to their needs.
Amazon, for example, heavily relies on personalized recommendations, and their success is directly tied to the effectiveness of this system. A failure to personalize recommendations would severely impact their customer engagement and sales.
Step-by-Step Procedure for Improving Personalization
To improve the personalization of product recommendations, a systematic approach is required.
- Enrich User Profiles: Implement mechanisms to collect detailed user information beyond basic demographics. This includes explicit preferences (e.g., through questionnaires or preference settings), implicit preferences (e.g., browsing history, purchase history, time spent on product pages), and contextual data (e.g., location, device, time of day).
- Implement Advanced Analytics: Utilize machine learning algorithms, such as collaborative filtering and content-based filtering, to analyze the collected user data and identify patterns and preferences. This allows for more accurate and nuanced recommendations.
- A/B Test Different Personalization Strategies: Experiment with different approaches to personalization, such as recommending similar products, complementary products, products viewed by other users with similar profiles, or products based on user-provided preferences. A/B testing helps identify the most effective strategies.
- Iterate and Refine: Continuously monitor and evaluate the performance of the recommendation system. Use key metrics such as click-through rates, conversion rates, and user engagement to identify areas for improvement and refine the personalization strategies accordingly. Regular updates based on user feedback and performance data are crucial.
- Provide Transparency and Control: Allow users to control their preferences and see how the recommendations are generated. This builds trust and enhances user experience. For example, allowing users to explicitly state preferences or opt-out of certain recommendation types enhances transparency and control.
Product Catalog and Presentation
Our recommendation engine relies heavily on the accuracy and completeness of the product data it uses. If the product catalog is flawed, our recommendations will inevitably suffer, leading to irrelevant suggestions and frustrated users. In essence, garbage in, garbage out. Let’s explore how improving our product catalog can dramatically improve recommendation accuracy.Incomplete or poorly categorized product information directly impacts the recommendation engine’s ability to understand and match products with user needs.
For example, if a product description lacks key features or uses vague terminology, the system may fail to connect it to relevant searches or user profiles. Similarly, inconsistent or missing categorization can prevent the system from effectively grouping similar products, resulting in a diluted pool of recommendations. A product tagged inconsistently as both “outdoor gear” and “home decor” will likely appear in irrelevant recommendations.
Effective Product Descriptions and Tagging Strategies
Well-structured product descriptions are crucial for accurate recommendations. Each description should be detailed, comprehensive, and utilize relevant s. Consider the following example: Instead of simply stating “Running Shoes,” a more effective description would be: “Lightweight, breathable running shoes designed for neutral runners. Featuring responsive cushioning, a durable outsole, and a comfortable, supportive fit. Ideal for daily training and races up to marathon distance.
Available in sizes 6-13.” Notice the inclusion of specifics like runner type, cushioning, and use case.To enhance relevance, implement a robust tagging strategy. Use a hierarchical system with broad categories and increasingly specific subcategories. For instance, a “Running Shoes” category could be further broken down into “Neutral,” “Supination,” and “Pronation,” allowing for more precise targeting. Employ both manual tagging and automated tagging techniques, leveraging natural language processing to extract relevant s from product descriptions.
Using Images to Improve Product Understanding
High-quality product images are invaluable for enhancing user understanding and, consequently, improving recommendation accuracy. A single image is often insufficient. Consider using a series of images to highlight different aspects of a product.For example, imagine a product page for a new backpack. Instead of one generic image, we could use:
- A main image showcasing the backpack’s overall design and color, in a lifestyle setting (e.g., a hiker wearing it on a trail).
- Close-up images highlighting specific features like the adjustable straps, breathable back panel, and durable zippers. These images should be clearly labeled to avoid ambiguity.
- Images showing the backpack’s various compartments and their capacity, possibly with items inside to illustrate their size and utility.
- An image showing the backpack’s dimensions compared to a common object (e.g., a water bottle) for scale and better size perception.
These detailed visuals allow users to better grasp the product’s features and functionality, improving their understanding and thus leading to more accurate recommendations based on their perceived needs. The system can even analyze image features to further enhance recommendation accuracy.
User Interface and Experience (UI/UX)
Let’s face it: even the most brilliant recommendation engine is useless if users can’t find or understand it. A poorly designed user interface can completely sabotage the effectiveness of your product recommendations, no matter how accurate your data or sophisticated your algorithm. The key is to seamlessly integrate recommendations into the user journey, making them both discoverable and appealing.Poor UI/UX design can significantly hinder the effectiveness of product recommendations in several ways.
A cluttered or confusing interface can overwhelm users, leading them to ignore recommendations altogether. Poorly placed recommendations, for example, buried deep within a website or app, are effectively invisible. Similarly, recommendations that are not visually distinct from other content can easily get lost in the shuffle. Finally, a lack of clear calls to action can prevent users from engaging with the recommendations, even if they’re interested.
The user experience needs to be intuitive and effortless; otherwise, your carefully crafted recommendations will be wasted.
Examples of User Interface Elements that Enhance Recommendation Visibility and Usability
Effective UI/UX design is crucial for making product recommendations visible and easy to use. Here are some examples of interface elements that can significantly improve the user experience:
- Clear and Concise Headings: Instead of vague titles like “Recommended for You,” use more specific and engaging headings such as “Customers Who Bought This Also Bought…” or “Based on Your Recent Activity…”. This provides context and encourages users to explore the suggestions.
- Visually Appealing Presentation: Use high-quality images and compelling product descriptions to showcase recommended items. A carousel or grid layout, rather than a simple list, can make recommendations more visually appealing and easier to browse.
- Strategic Placement: Recommendations should be placed in high-traffic areas of the website or app, such as the homepage, product detail pages, and checkout pages. Consider A/B testing different placements to find what works best.
- Interactive Elements: Incorporating interactive elements like “See More” buttons or expandable sections allows users to easily explore more recommendations without feeling overwhelmed. This caters to users who want more choices but also those who want a quick and easy overview.
- Personalized Messages: Tailoring the message accompanying the recommendations based on user behavior or preferences adds a personal touch and increases engagement. For example, “Based on your love for cozy sweaters…” or “We think you’ll love these new arrivals based on your recent purchases…”
Redesigned Recommendation Section Mock-up
Imagine an e-commerce website currently displaying recommendations as a small, unassuming list at the very bottom of the product page. This is ineffective. Let’s redesign it.Our redesigned recommendation section would be prominently featured on the product page, just below the main product description. It would be visually distinct, using a clean and modern design.The design would include:
- Headline: “Customers Who Bought This Also Bought…”
- Layout: A visually appealing carousel showcasing 4-6 products at a time, with clear, high-quality product images and concise descriptions. Arrows would allow users to navigate through additional recommendations.
- Visual Hierarchy: The recommended products would be visually distinct from other content on the page, using a contrasting background color or border. This makes them stand out and draws the user’s attention.
- Call to Action: Each product would have a clear “Add to Cart” button, making it easy for users to purchase recommended items.
- Dynamic Content: The recommendations would update in real-time based on the user’s browsing history and purchase behavior, ensuring relevance and personalization.
Justifications for Design Choices:* Prominent Placement: Increases visibility and encourages engagement.
Carousel Layout
Allows users to easily browse multiple recommendations without overwhelming them.
High-Quality Images
Enhance the visual appeal and make products more desirable.
Concise Descriptions
Provide essential information without overwhelming users.
Clear Call to Action
Makes it easy for users to purchase recommended items.
Dynamic Content
Ensures the recommendations remain relevant and personalized.
A/B Testing and Iteration
So, we’ve tackled data issues, algorithm tweaks, personalization problems, and even UI/UX snags. Our recommendation engine still isn’t performing as hoped? Time to get scientific. A/B testing is our next weapon in this optimization arsenal. It’s the key to understanding what truly resonates with our users and refining our recommendations accordingly.A/B testing allows us to compare different versions of our recommendation system simultaneously.
By systematically varying one aspect at a time – a different algorithm, a new ranking system, a changed presentation style – and tracking user engagement, we can pinpoint what drives the best results. This iterative approach, based on concrete data, allows us to make informed decisions and avoid costly guesswork.
A/B Testing Strategies for Recommendation Systems
Before diving into specific tests, we need a clear methodology. We’ll define key performance indicators (KPIs) to measure success. These KPIs could include click-through rates (CTR), conversion rates, average order value (AOV), and overall user engagement. Choosing the right KPIs depends on our business objectives. For example, if our goal is to increase sales, AOV and conversion rate will be crucial.
If we’re focusing on user engagement, CTR and time spent on the recommendation page will be more relevant. We also need to define our sample size – a sufficiently large group of users to ensure statistically significant results. Finally, we’ll need to establish a clear duration for the test to gather enough data.
Interpreting A/B Test Results and Iterating
Once the A/B test concludes, we analyze the collected data. Statistical significance is paramount; we need to be confident that observed differences aren’t due to random chance. Let’s say we’re comparing two recommendation algorithms: Algorithm A and Algorithm B. If Algorithm B shows a statistically significant 15% increase in CTR compared to Algorithm A, we have strong evidence that Algorithm B is superior.
This doesn’t mean we’re done; we might want to conduct further tests to see how Algorithm B performs under different conditions, or against yet another algorithm. The iterative process continues; we learn, adapt, and improve based on data-driven insights. For example, a test might reveal that personalized recommendations based on past purchase history significantly outperform generic recommendations, leading us to refine our personalization algorithms.
Ongoing Monitoring and Improvement Plan
A/B testing isn’t a one-off event; it’s an ongoing process. We need a plan for continuous monitoring and improvement. This involves regularly running A/B tests to evaluate new features, algorithm updates, and UI/UX changes. We should also track key metrics over time to identify trends and potential areas for improvement. Regularly reviewing our data will help us proactively identify and address any performance dips.
Implementing a robust monitoring system, perhaps using dashboards that visually represent key KPIs, will ensure we stay informed and can react quickly to any changes in user behavior or system performance. This continuous improvement cycle is crucial for maintaining a high-performing recommendation system. For example, we might notice a seasonal shift in user preferences, requiring adjustments to our algorithms and product presentations.
This could involve highlighting summer clothing during warmer months and focusing on winter wear during the colder season.
End of Discussion: Why Your Product Recommendations Arent Working
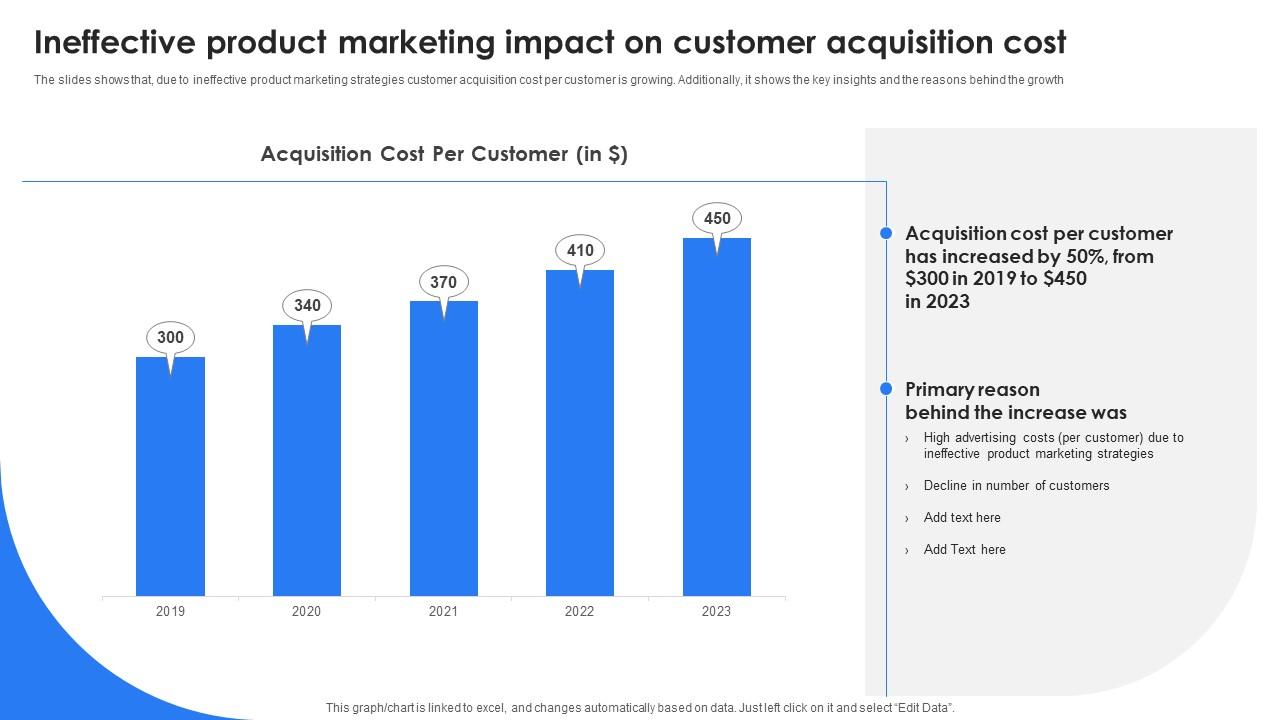
Ultimately, improving your product recommendations is an iterative process. It requires a holistic approach, addressing data quality, algorithm effectiveness, user personalization, product presentation, and user experience. By diligently addressing these areas, implementing A/B testing, and continuously monitoring results, you can transform your recommendations from a source of frustration into a powerful driver of engagement and sales. Don’t let those underperforming recommendations hold your business back – start optimizing today!
Quick FAQs
What if my product catalog is incomplete?
Incomplete product information directly impacts recommendation accuracy. Ensure your catalog is up-to-date, comprehensive, and includes high-quality images and detailed descriptions.
How can I better understand user preferences?
Utilize user data (purchase history, browsing behavior, ratings, reviews) and incorporate feedback mechanisms like surveys and polls. Consider offering personalized quizzes or preference selectors.
My recommendations seem random – what’s wrong?
Your algorithm might need tuning or retraining. Consider exploring different algorithms (collaborative filtering, content-based filtering, hybrid approaches) and evaluating their performance with A/B testing.
How often should I A/B test my recommendations?
Regular A/B testing is crucial. Aim for continuous testing, experimenting with different recommendation strategies, and analyzing the results to identify what works best for your audience.