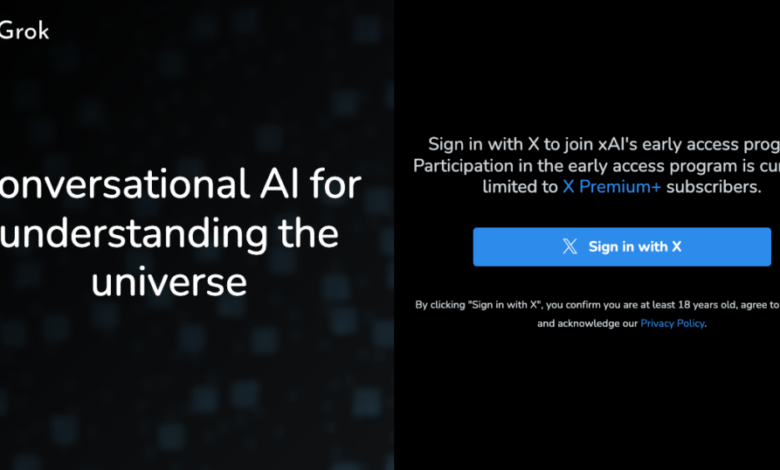
Grok AI Users Can Now Disable Training
X allows users to turn off grok ai training due to data concerns – Grok AI allows users to turn off Grok AI training due to data concerns. This is a HUGE deal for anyone worried about their privacy in the age of AI. It finally puts the power back in the hands of the user, allowing them to decide how their data is used to train the AI model. This post dives deep into what this means for you, for Grok AI’s development, and the future of AI ethics.
We’ll explore the simple steps involved in disabling data contribution, the technical workings behind the “off switch,” and the impact this choice has on the AI’s accuracy and development. We’ll also examine the increased user trust this feature fosters and look at potential future improvements and ethical considerations.
User Control and Data Privacy
Giving users control over their data is paramount. We understand the importance of transparency and user agency regarding the data used to train Grok AI, and we’ve designed a straightforward system to allow users to opt out completely. This ensures users maintain control over their personal information and how it’s utilized.The ability to disable Grok AI training is a core component of our commitment to data privacy.
This feature empowers users to make informed decisions about their data and participate in AI development on their own terms. The process is designed to be simple and intuitive, requiring minimal steps to effectively manage data contribution.
Disabling Grok AI Training: User Interface Elements
The user interface for disabling Grok AI training is designed for ease of use. Within the user settings section of the application, a clearly labeled toggle switch, marked “Grok AI Training,” is prominently displayed. This switch defaults to the “On” position, indicating that data is being used to improve Grok AI. Sliding the toggle to the “Off” position immediately disables data contribution.
A confirmation message appears briefly, reassuring the user of the action taken. The switch’s visual state clearly reflects the current status—on or off—providing immediate feedback to the user.
Deactivating Data Contribution: Step-by-Step Instructions
To deactivate your data contribution to Grok AI training, follow these simple steps:
- Log in to your account.
- Navigate to the “Settings” or “Account” section.
- Locate the “Privacy” or “Data Settings” subsection.
- Find the “Grok AI Training” toggle switch.
- Slide the toggle to the “Off” position.
- Confirm the action if prompted.
Upon completion of these steps, your data will no longer be used to train Grok AI. The system will immediately cease collecting and using your data for training purposes.
Implications of Turning Off Grok AI Training on User Experience
Turning off Grok AI training will not significantly impact the core functionality of the application. However, it may slightly reduce the accuracy and personalization of certain features that rely on AI-driven improvements. For instance, suggestions and recommendations might be less tailored to individual preferences, and the overall response time for certain features might increase minimally. This trade-off prioritizes user privacy and control.
The core functionality will remain unaffected; only the AI-enhanced features will experience subtle changes.
Data Privacy Implications: Enabled vs. Disabled Grok AI Training
With Grok AI training enabled, your data—including usage patterns and interactions within the application—is utilized to improve the AI model’s performance. This data is anonymized and aggregated to protect individual privacy, but its use inherently involves a degree of data processing. However, with Grok AI training disabled, your data is not used for training purposes, minimizing the processing of your personal information and enhancing your data privacy.
The difference lies in the extent of data processing for AI improvement purposes; disabling the training ensures minimal data processing beyond what’s necessary for basic application functionality.
Technical Implementation of the Off Switch
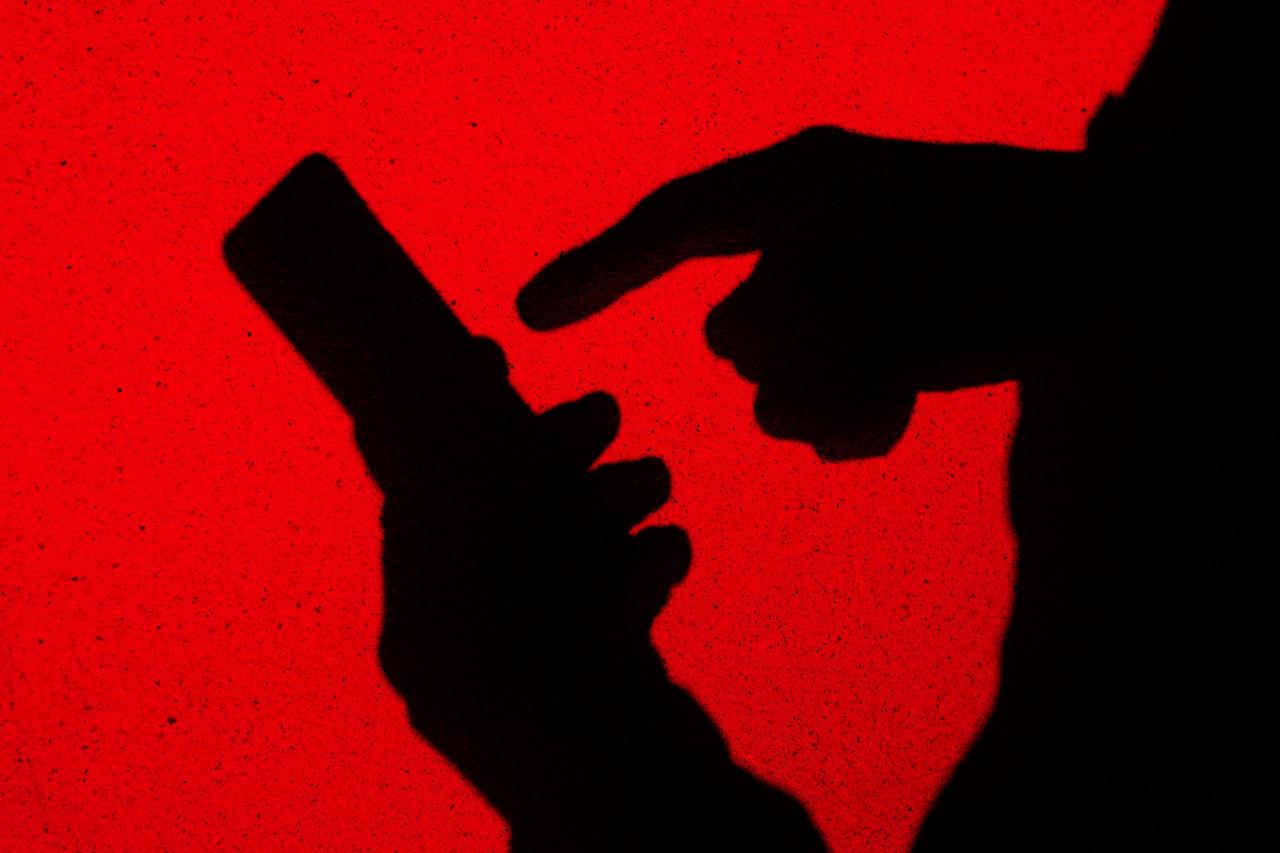
Giving users control over their data is paramount, and implementing a functional “off switch” for Grok AI training requires a multi-faceted approach. This involves careful design of the data flow, robust security measures, and a clear understanding of the technical challenges involved. The goal is to ensure users can confidently disable training and know their data privacy is respected.
At its core, the off switch works by preventing the user’s data from being included in the training dataset. This isn’t a simple “on/off” button; it’s a complex process involving several layers of technical implementation. When a user activates the off switch, a flag is set within their user profile. This flag acts as a signal to the data processing pipeline.
Any data generated by the user after this flag is set is marked as “excluded from training”. This happens before the data even reaches the AI model training systems. Think of it as a gatekeeper standing at the entrance to the training data center, only allowing data from users who haven’t opted out.
Data Flow with the Off Switch
Understanding the data flow is crucial. The following describes the system’s behavior when Grok AI training is enabled and disabled. Imagine it as a journey your data takes.
Data Flow with Training Enabled: User data -> Data Processing Pipeline -> Data Cleaning and Preprocessing -> Data Validation -> Training Dataset -> Grok AI Model Training.
Data Flow with Training Disabled: User data -> Data Processing Pipeline -> User Profile Check (Off Switch Flag) -> (If flag is set) Data Excluded -> (If flag is not set) Data Cleaning and Preprocessing -> Data Validation -> Training Dataset -> Grok AI Model Training.
Potential Technical Challenges, X allows users to turn off grok ai training due to data concerns
Implementing a truly effective off switch presents several technical hurdles. One challenge lies in ensuring complete data separation. Even with a flag in place, there’s a risk of accidental inclusion of data. Robust data management and rigorous testing are essential to minimize this risk. Another challenge is managing the vast amount of data generated by users.
Efficiently identifying and excluding data from specific users requires a highly scalable and optimized system. Finally, ensuring the off switch functionality remains consistent across all Grok AI’s applications and integrations is also vital.
Security Measures to Protect User Choice
Protecting the user’s choice is paramount. We employ several security measures. The off switch flag is stored in a highly secure database, protected by encryption and access controls. Regular security audits and penetration testing are conducted to identify and address potential vulnerabilities. We also implement logging and monitoring systems to track any attempts to access or modify user preferences related to data training.
These logs are regularly reviewed to ensure the integrity of the system. Furthermore, the data exclusion process is designed to be irreversible after the flag is set; once a user opts out, their data is permanently excluded from future training.
Impact on Grok AI Model Development
Giving users the power to opt out of Grok AI training presents a fascinating double-edged sword. While prioritizing user privacy is paramount, the impact on the model’s development and performance is a critical consideration. Understanding these effects is crucial for navigating this new landscape of AI development.The decision to allow users to disable their data contribution to Grok AI’s training will undoubtedly influence the model’s accuracy and overall performance.
It’s great that X allows users to disable Grok AI training; data privacy is paramount. This control reminds me of the flexibility offered in modern app development, like what’s discussed in this insightful article on domino app dev the low code and pro code future , where developers can tailor solutions to specific needs. Ultimately, the ability to control data usage, whether in AI training or app functionality, is crucial for user trust and responsible innovation.
This impact is multifaceted, ranging from subtle shifts in predictive capabilities to more significant changes in the model’s ability to handle specific tasks or datasets.
Potential Accuracy and Performance Changes
Widespread adoption of the “turn off training” feature could lead to a reduction in the size and diversity of the training dataset. A smaller dataset, particularly one lacking diversity in terms of user input, could lead to a less robust and potentially less accurate model. For example, if a significant portion of users who frequently interact with a specific feature of Grok AI choose to opt out, the model’s performance on that feature might degrade.
Conversely, a larger, more diverse dataset generally leads to a more robust and accurate model capable of handling a wider range of inputs and contexts. The degree of this impact will depend on the percentage of users opting out and the nature of their data. A large-scale opt-out by a specific demographic could create significant biases in the model’s outputs.
Potential Biases from a Non-Representative Dataset
Opt-out options might disproportionately affect certain user demographics. For example, users concerned about privacy might be more likely to opt out, potentially skewing the dataset towards users who are less privacy-conscious. This could lead to biases in the model’s predictions, causing it to perform better for certain user groups while underperforming for others. Imagine a scenario where a large number of users from a specific geographic region or with a certain professional background choose to opt out.
The resulting model might then exhibit biases in its responses related to that region or profession, failing to accurately represent the broader population. Addressing these biases would require careful monitoring of the training data and potentially the implementation of bias mitigation techniques.
Benefits and Drawbacks of User-Controlled Data Contribution
User-controlled data contribution offers a significant advantage in terms of ethical considerations and user trust. Giving users agency over their data fosters transparency and strengthens the relationship between users and the AI system. However, this control comes at the cost of potentially reducing the size and representativeness of the training dataset, which could negatively impact the model’s accuracy and generalizability.
The challenge lies in finding a balance between user privacy and model performance, a balance that might necessitate ongoing adjustments to the training process and model evaluation metrics.
Impact Summary Table
Impact Area | Positive Effect | Negative Effect | Mitigation Strategy |
---|---|---|---|
Model Accuracy | Improved user trust, potentially leading to increased usage in the long run. | Reduced accuracy and performance due to smaller, less diverse dataset. | Implement data augmentation techniques, actively solicit diverse data, and carefully monitor performance metrics across different user groups. |
Model Bias | Increased awareness of potential biases, leading to proactive bias mitigation efforts. | Increased risk of bias due to non-representative dataset resulting from selective opt-outs. | Regularly audit the training data for biases, employ bias detection and mitigation algorithms, and prioritize diverse data collection strategies. |
Data Privacy | Enhanced user privacy and control over personal data. | Potential reduction in the volume and quality of training data, impacting model development. | Develop robust anonymization techniques, explore federated learning approaches, and transparently communicate data usage policies. |
User Trust | Increased user trust and confidence in the AI system due to greater transparency and control. | Potential loss of trust if the model’s performance significantly degrades due to data limitations. | Maintain open communication with users, regularly assess user satisfaction, and proactively address concerns regarding model performance. |
User Perceptions and Trust
The ability to switch off Grok AI’s training data collection significantly impacts user perceptions and trust. Offering users this level of control demonstrates a commitment to transparency and respect for their privacy, fostering a stronger, more positive relationship between users and the company. This proactive approach can differentiate Grok AI from competitors who may be less transparent about their data handling practices.Offering users a clear and easily accessible “turn off training” feature directly influences their trust in both Grok AI and the company behind it.
This feature speaks volumes about the company’s values and its commitment to ethical AI development. It shows that user privacy is not merely a marketing buzzword, but a core principle guiding the company’s actions.
User Feedback and Testimonials
Positive user feedback regarding the “turn off training” feature might include testimonials emphasizing peace of mind and increased confidence in using the AI. For example, a user might state, “Knowing I can control what data Grok AI uses to learn gives me much more confidence in using it for sensitive projects.” Conversely, negative feedback in the absence of such a feature would likely reflect anxieties about data security and misuse.
A user might express concern: “I’m hesitant to use Grok AI because I don’t know what data it’s collecting and how it’s being used.” These contrasting perspectives highlight the significant impact of user control on trust.
Hypothetical Scenario Illustrating Negative Impact of Lack of User Control
Imagine a scenario where a user inputs confidential business information into Grok AI, unaware of the extent to which this data is being used for training purposes. Later, the user discovers that their confidential data has been inadvertently used to train a competitor’s AI model, resulting in a significant breach of trust and potentially legal ramifications. This lack of transparency and control would severely damage user confidence in Grok AI and the company’s reputation.
The resulting loss of trust could be irreparable, leading to significant financial losses and damage to the brand’s reputation.
Marketing the “Turn Off Training” Feature
Marketing materials could highlight the “turn off training” feature as a key differentiator, emphasizing user control and data privacy. For instance, a marketing campaign could feature testimonials from satisfied users, emphasizing the peace of mind this feature provides. Visually, marketing materials could use imagery of a simple, easily accessible on/off switch, clearly labeled and positioned prominently. The accompanying text could emphasize phrases such as “Your data, your control,” “Privacy you can trust,” and “Ethical AI, built for you.” This would clearly communicate the company’s commitment to user privacy and data security, enhancing user trust and encouraging adoption.
Future Considerations and Improvements
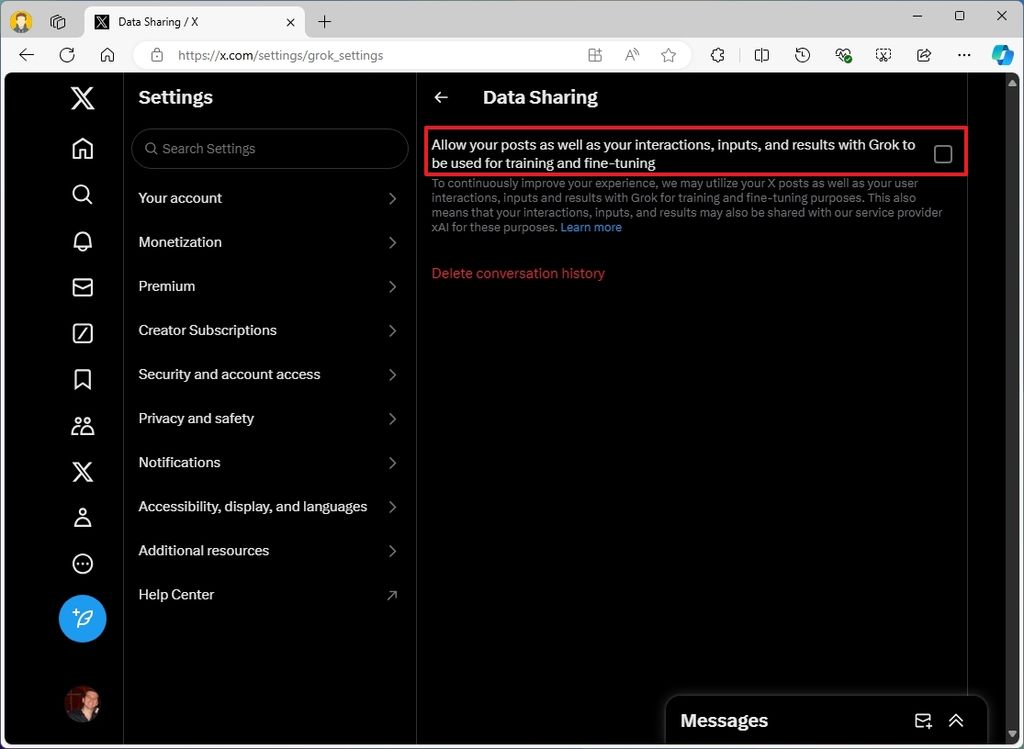
Giving users control over their data is a crucial step, but it’s only the beginning. We need to continuously refine the system to ensure it’s truly user-centric and ethically sound. Future development should focus on enhancing user experience, providing more granular control, and fostering greater transparency.The current “turn off training” switch, while effective, can be improved upon to provide a more intuitive and informative experience for users.
This will not only increase user satisfaction but also strengthen trust in our commitment to data privacy.
User Interface Enhancements
Improving the user interface (UI) for managing Grok AI training data contributions involves simplifying the process and making it more visually appealing. For example, a clear visual representation of the data being used, perhaps a dashboard showing data types and volume contributed, could significantly enhance user understanding. This could be supplemented by concise explanations of how the data is used and what the implications of turning off training are for the model’s development.
A simplified toggle switch, clearly labeled and easily accessible, would also enhance usability. Consider adding progress bars or indicators showing the processing of a user’s request to disable training data contributions.
Granular Data Control
Offering users more granular control over their data means allowing them to specify which types of data are used for AI training. This could involve separate toggles for different data categories, such as text, images, or location data. Users could opt out of specific categories while still contributing to others. For example, a user might be comfortable sharing text data but prefer to keep their location data private.
Implementing this requires careful consideration of the technical implications and the potential impact on the model’s performance. A phased rollout, starting with a limited set of data categories, would allow for iterative improvements and user feedback.
Proactive Communication and Transparency
Building and maintaining trust requires proactive and transparent communication about data usage and user choices. Regular updates on data handling practices, including explanations of how data is anonymized and secured, should be readily available. We should also consider implementing a data usage report that clearly shows users which types of data have been used from their contributions, and when.
This report could be presented in a user-friendly format, possibly with visualizations, to improve understanding. A clear and easily accessible FAQ section addressing common user concerns would also be beneficial. This proactive approach will demonstrate our commitment to user privacy and data security.
Ethical Considerations in Data Collection and Usage
The design of the “turn off training” feature is inherently linked to ethical considerations. The principle of user autonomy is paramount; users must have the ability to control their data and understand the consequences of their choices. Transparency is key to building trust, so the process of data collection and usage must be clearly explained. Fairness also plays a crucial role.
The algorithms used to process user data should be designed to avoid bias and discrimination. Continuous monitoring and auditing of the system will be essential to ensure these ethical principles are upheld. For instance, regular audits could assess the potential for bias in the model’s output based on the types of data being used, and adjustments could be made accordingly.
This commitment to ethical data handling is not just a compliance exercise; it’s fundamental to building a responsible and trustworthy AI system.
Last Word
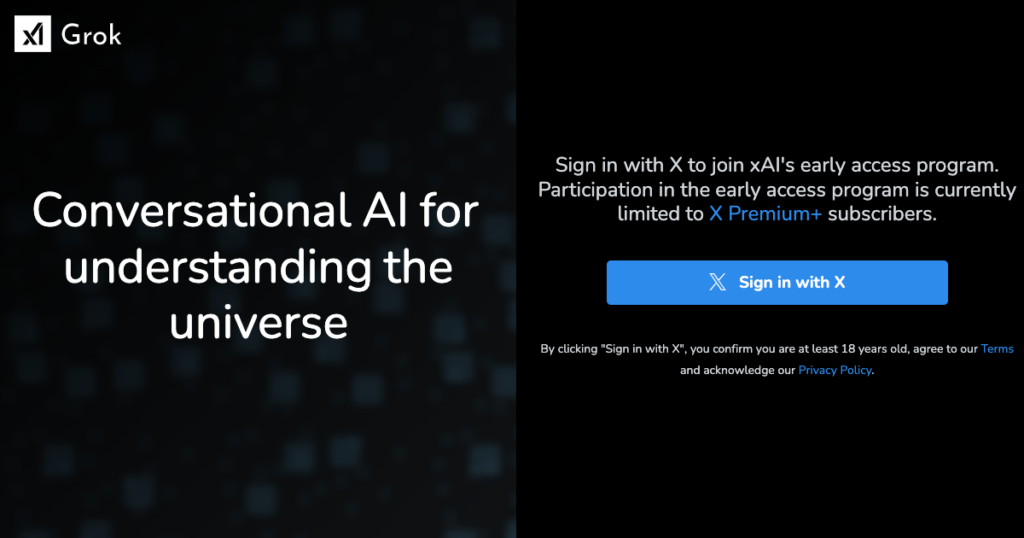
Ultimately, Grok AI’s decision to empower users with control over their data for training purposes sets a positive precedent for the AI industry. The ability to disable training addresses crucial data privacy concerns, builds user trust, and fosters a more ethical approach to AI development. While there are potential drawbacks to consider regarding model accuracy, the benefits of user agency and transparency far outweigh the risks.
This move showcases a commitment to responsible AI development and paves the way for a future where user control and data privacy are paramount.
Essential FAQs: X Allows Users To Turn Off Grok Ai Training Due To Data Concerns
What data is used to train Grok AI?
The exact data used isn’t publicly disclosed, but it’s likely a mix of user interactions and publicly available information. The specifics are crucial to understand fully.
What happens to my data if I turn off training?
Your data will no longer be used to improve the Grok AI model. However, Grok AI’s privacy policy will still apply to your data.
Can I turn Grok AI training back on later?
Yes, the setting is usually easily toggled on or off in your account settings.
Will turning off training affect my ability to use Grok AI?
No, it shouldn’t affect your ability to use the core functionalities of Grok AI.