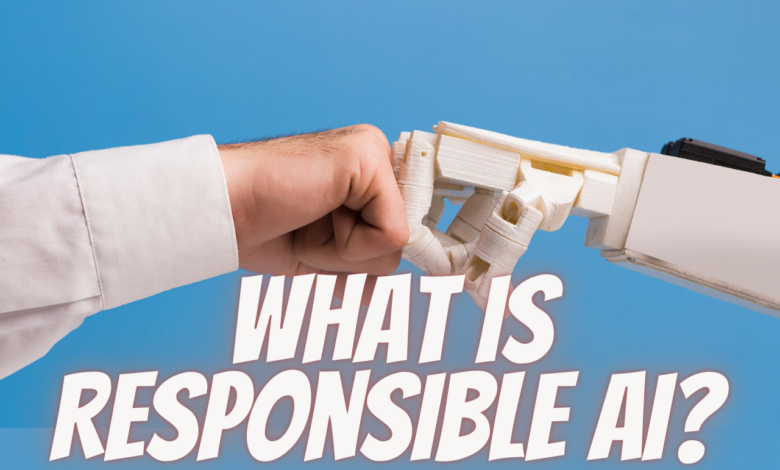
XAI Bringing Transparency and Ethics to AI
Xai bringing transparency and ethics to artificial intelligence – XAI: Bringing transparency and ethics to artificial intelligence sets the stage for a fascinating exploration of how we can build AI systems that are not only powerful but also understandable and responsible. We’re diving deep into the world of Explainable AI, examining its core principles, and exploring how it’s transforming various sectors. Get ready to uncover the ethical considerations, practical applications, and future potential of this groundbreaking field!
This post will unpack the complexities of XAI, from defining its core principles and comparing different techniques, to examining its role in promoting transparency and mitigating bias in AI systems. We’ll delve into real-world examples, showcasing how XAI is being used to improve decision-making across diverse industries, and consider the challenges and opportunities that lie ahead. Prepare to gain a clearer understanding of how XAI is shaping the future of artificial intelligence and its impact on our lives.
Defining XAI and its Core Principles
Explainable AI (XAI) is a rapidly developing field aiming to make the decision-making processes of artificial intelligence systems more transparent and understandable to humans. This is crucial for building trust, ensuring accountability, and identifying potential biases within AI systems. Unlike traditional AI, which often operates as a “black box,” XAI prioritizes the ability to inspect and interpret the reasoning behind AI’s outputs.XAI’s core principles revolve around providing insights into how an AI model arrives at its conclusions.
This involves not only understanding the model’s internal workings but also explaining its predictions in a way that is meaningful and accessible to both technical and non-technical audiences. Key characteristics that differentiate XAI from traditional AI include its focus on interpretability, transparency, and the ability to provide justifications for its decisions. This contrasts sharply with traditional AI models, many of which are highly complex and opaque, making it difficult to understand why they produce specific outputs.
XAI Techniques: A Comparative Analysis
Several techniques are used to achieve explainability in AI. These techniques vary in their approach, complexity, and the level of insight they provide. Broadly, they can be categorized into methods that explain the model’s overall behavior and those that focus on explaining individual predictions.
Comparison of XAI Methods
The following table compares different XAI methods based on their transparency and interpretability levels. Transparency refers to the ease with which the model’s internal workings can be understood, while interpretability refers to the clarity and ease of understanding the explanations provided by the model.
Method | Transparency Level | Interpretability Level | Application Examples |
---|---|---|---|
Linear Regression | High | High | Predicting house prices based on features like size and location. The coefficients directly show the impact of each feature. |
Decision Trees | High | High | Classifying customers into different risk categories based on their credit history. The decision tree visually represents the decision-making process. |
Rule-Based Systems | High | High | Expert systems for medical diagnosis, where the rules are explicitly defined and easily understood. |
LIME (Local Interpretable Model-agnostic Explanations) | Medium | Medium | Explaining the predictions of a complex image classifier by highlighting the relevant image regions contributing to the classification. |
SHAP (SHapley Additive exPlanations) | Medium | Medium | Understanding the feature importance in a black-box model like a neural network for fraud detection. It assigns contribution scores to each feature. |
Deep Neural Networks (with specific architectures for explainability) | Low | Low | While inherently complex, techniques like attention mechanisms can provide some insights into the network’s focus during processing. However, full transparency remains a challenge. |
XAI’s Role in Promoting Transparency in AI Systems
XAI, or Explainable AI, is crucial for bridging the gap between the complex inner workings of artificial intelligence and the need for human understanding. Without transparency, AI systems risk becoming “black boxes,” making it impossible to understand how they arrive at their decisions. This lack of insight undermines trust and hinders the responsible development and deployment of AI.
XAI tackles this challenge head-on by providing methods and techniques to make AI decision-making processes more understandable and interpretable.XAI enhances the understanding of AI decision-making processes by offering various techniques to dissect the internal logic of AI models. These techniques range from simpler methods like visualizing decision trees to more sophisticated approaches involving feature importance analysis or generating natural language explanations of predictions.
Instead of simply receiving a prediction, users gain insights into the factors that contributed to that outcome, allowing for better evaluation and validation of the AI’s performance. This granular level of understanding allows for improved model debugging, identifying biases, and ensuring fairness and accuracy.
Transparency’s Impact on Trust and Accountability
Transparency in AI fosters trust among users and stakeholders. When individuals understand how an AI system arrives at its conclusions, they are more likely to accept its decisions and trust its recommendations. This is particularly important in high-stakes applications such as healthcare, finance, and criminal justice, where trust is paramount. Moreover, transparency enhances accountability. By making the decision-making process visible, it becomes easier to identify and address errors, biases, or unintended consequences.
This accountability is essential for ensuring the responsible and ethical use of AI.
Examples of Negative Consequences Due to Lack of Transparency, Xai bringing transparency and ethics to artificial intelligence
Several instances demonstrate the risks associated with opaque AI systems. For example, in loan applications, an AI system that lacks transparency might unfairly deny loans to certain demographic groups without providing any explanation. This lack of transparency not only leads to potential discrimination but also makes it difficult to address the underlying bias within the AI model. Similarly, in the healthcare sector, an AI system diagnosing a disease without providing a clear rationale could lead to mistrust and reluctance to use the technology, even if the diagnosis is accurate.
The lack of transparency hinders the ability to verify the system’s performance and address any potential errors or biases.
Visual Representation of XAI’s Impact on Transparency
Imagine a diagram showing two AI systems side-by-side. The first system, representing a non-explainable AI, is depicted as a closed, black box with an input and output. Arrows represent data entering the box and a final decision exiting, but the internal processes remain hidden and opaque. The second system, incorporating XAI, is represented as a transparent box.
The internal workings, including the data processing steps, algorithms used, and key factors influencing the decision, are clearly visible through the transparent casing. Colored pathways highlight the flow of data and the influence of specific features on the final output. This visual clearly illustrates how XAI transforms a mysterious “black box” into a readily understandable and transparent system, enhancing trust and accountability.
Ethical Implications of XAI and AI Development
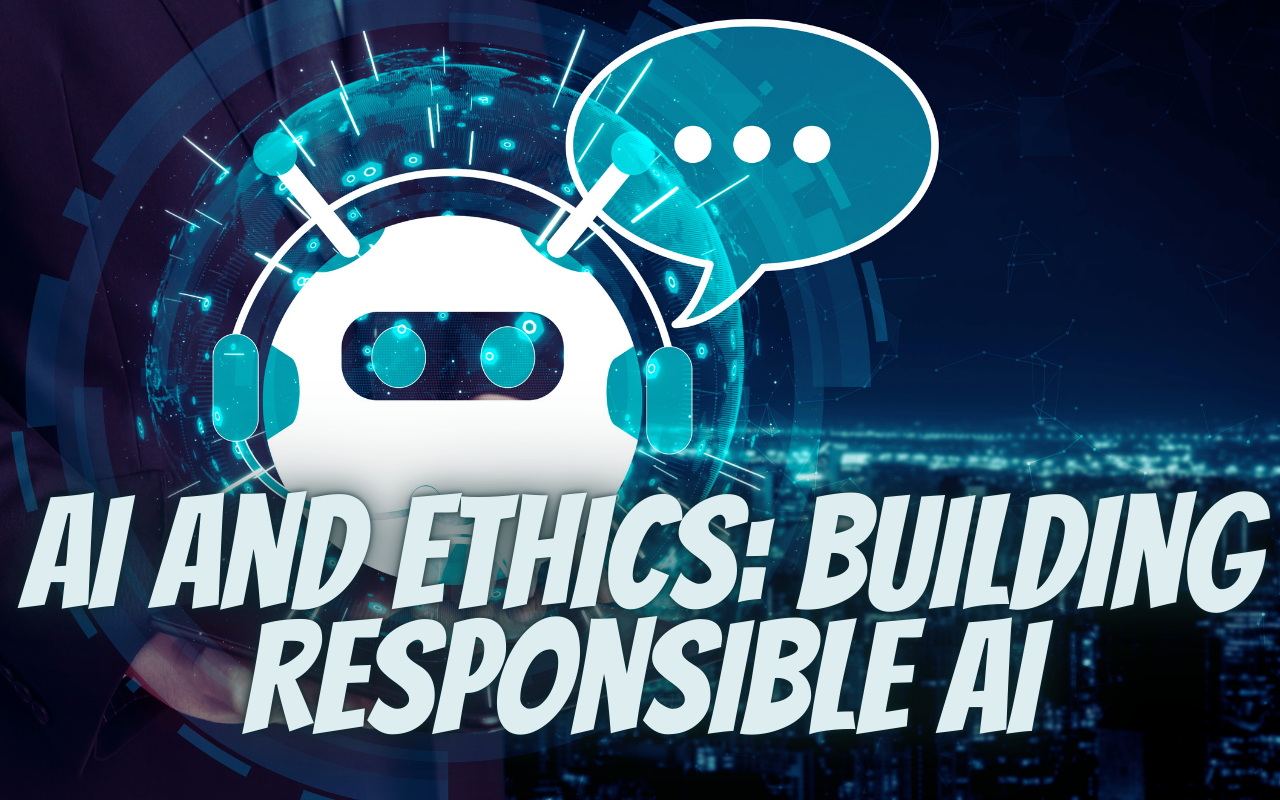
The development and deployment of Explainable AI (XAI) presents a complex tapestry of ethical considerations. While XAI aims to increase transparency and accountability in AI systems, its very existence introduces new challenges and necessitates careful consideration of its potential impact on individuals and society. The promise of understanding how AI arrives at its decisions is powerful, but we must also grapple with the ethical implications that arise from both the use and misuse of this enhanced understanding.
Potential Ethical Challenges Associated with XAI Deployment
The pursuit of explainability doesn’t automatically guarantee ethical outcomes. For example, the explanations generated by XAI might be overly simplistic, misleading, or even deliberately manipulated to obscure biases or errors. Furthermore, the very act of providing explanations can inadvertently reveal sensitive information about individuals or groups, leading to privacy violations. The availability of explanations might also be unevenly distributed, creating further disparities between those who can understand and those who cannot.
Consider a medical diagnosis system: while XAI might provide an explanation for a diagnosis, a lack of medical expertise could prevent patients from fully understanding or challenging the system’s reasoning. This unequal access to understanding can exacerbate existing inequalities.
XAI’s Role in Mitigating Biases and Discrimination in AI Algorithms
XAI offers a crucial tool for identifying and mitigating biases embedded within AI algorithms. By examining the factors influencing an AI’s decision-making process, developers can pinpoint sources of bias stemming from biased training data, flawed algorithms, or human intervention. For instance, facial recognition systems have been shown to exhibit significant bias against certain ethnic groups. XAI can help dissect the underlying reasons for these biases, allowing developers to adjust algorithms and training data to create more equitable outcomes.
However, it’s crucial to remember that XAI is not a silver bullet. Simply identifying biases is not enough; concrete steps must be taken to rectify them, and this requires ongoing monitoring and evaluation.
Ethical Considerations of XAI in High-Stakes Decision-Making
The ethical implications of XAI are amplified in high-stakes domains such as healthcare and finance. In healthcare, an XAI-powered diagnostic tool might provide an explanation for a diagnosis, but the responsibility for the final decision still rests with the medical professional. The trustworthiness of the explanation and the potential for misinterpretation must be carefully considered. Similarly, in finance, XAI systems used for loan applications or risk assessment must be scrutinized for fairness and transparency.
The potential for discriminatory outcomes, even with explanations provided, necessitates rigorous ethical oversight and validation. Consider a loan application denied by an AI system. While XAI might provide reasons for the denial, it’s crucial to ensure these reasons are not discriminatory and that the system is not perpetuating existing societal biases.
Ethical Guidelines for Developing and Deploying XAI Systems
The development and deployment of XAI systems demand a robust ethical framework. A set of guiding principles is essential to ensure responsible innovation.
Before listing the guidelines, it’s important to note that these guidelines should be viewed as a starting point and should be adapted and expanded upon as the field of XAI evolves and new challenges emerge. Furthermore, constant evaluation and auditing are crucial to ensure ongoing ethical compliance.
- Transparency and Explainability: Ensure that the explanations provided by XAI systems are accurate, understandable, and relevant to the users.
- Fairness and Non-discrimination: Actively mitigate biases and ensure that XAI systems do not perpetuate or exacerbate existing societal inequalities.
- Privacy and Data Security: Protect the privacy and security of sensitive data used to train and operate XAI systems.
- Accountability and Responsibility: Establish clear lines of accountability for the decisions made by XAI systems and their impact on individuals and society.
- Human Oversight and Control: Maintain appropriate levels of human oversight and control over XAI systems to prevent unintended consequences.
- Continuous Monitoring and Evaluation: Regularly monitor and evaluate the performance and ethical implications of XAI systems to identify and address any emerging issues.
Practical Applications of XAI Across Different Sectors
Explainable AI (XAI) is no longer a futuristic concept; it’s rapidly becoming a crucial component in various industries, driving both innovation and trust in AI-powered systems. By providing insights into the decision-making processes of AI, XAI empowers users to understand, validate, and ultimately, improve the performance and reliability of these systems. This section explores the practical applications of XAI across diverse sectors, highlighting its impact on decision-making and user understanding.
XAI in Healthcare
XAI is revolutionizing healthcare by enhancing the accuracy and transparency of diagnostic tools and treatment recommendations. For instance, XAI can be integrated into medical imaging analysis systems, providing detailed explanations for the AI’s identification of tumors or other anomalies. This allows medical professionals to validate the AI’s findings and make more informed decisions, potentially leading to earlier and more effective interventions.
Moreover, XAI can help personalize treatment plans by explaining the factors considered by the AI in recommending a specific course of action, promoting patient trust and understanding. Consider a scenario where an AI system recommends a specific drug dosage based on a patient’s genetic profile and medical history. XAI can break down this complex decision-making process, explaining the weight given to each factor and thus improving the doctor’s confidence in the recommendation.
- Benefits: Improved diagnostic accuracy, personalized treatment plans, enhanced patient trust, reduced medical errors.
- Limitations: Data privacy concerns, need for extensive training data, potential for bias in algorithms.
XAI in Finance
The financial sector relies heavily on AI for tasks such as fraud detection, credit scoring, and algorithmic trading. XAI plays a vital role in making these processes more transparent and accountable. For example, an XAI-powered fraud detection system could not only identify suspicious transactions but also explain the reasons behind its flagging, allowing investigators to focus their efforts efficiently.
Similarly, XAI can provide insights into credit scoring models, explaining why a loan application was approved or denied, fostering fairness and reducing bias. Imagine a scenario where a loan application is rejected. With XAI, the applicant could understand the factors that led to the rejection, such as low credit score or insufficient income, allowing them to address those issues and improve their chances in the future.
- Benefits: Increased transparency in financial decisions, reduced bias in lending and investment, improved fraud detection, enhanced regulatory compliance.
- Limitations: Complexity of financial models, need for specialized expertise to interpret XAI explanations, potential for manipulation of explanations.
XAI in Autonomous Driving
Autonomous vehicles rely on complex AI systems to navigate roads and make driving decisions. XAI is crucial for ensuring the safety and reliability of these systems. By providing explanations for the AI’s actions, such as braking or lane changes, XAI can help build trust among drivers and regulators. For example, if an autonomous vehicle unexpectedly brakes, an XAI system could explain the reason, such as detecting a pedestrian or an obstacle, enhancing transparency and accountability.
This is particularly important in accident investigations, where understanding the AI’s decision-making process is crucial for determining liability.
- Benefits: Increased safety and reliability of autonomous vehicles, improved trust among drivers, enhanced regulatory compliance, faster accident investigation.
- Limitations: Complexity of sensor data and decision-making processes, need for robust and reliable XAI systems, potential for adversarial attacks targeting explanations.
Future Directions and Challenges in XAI Research
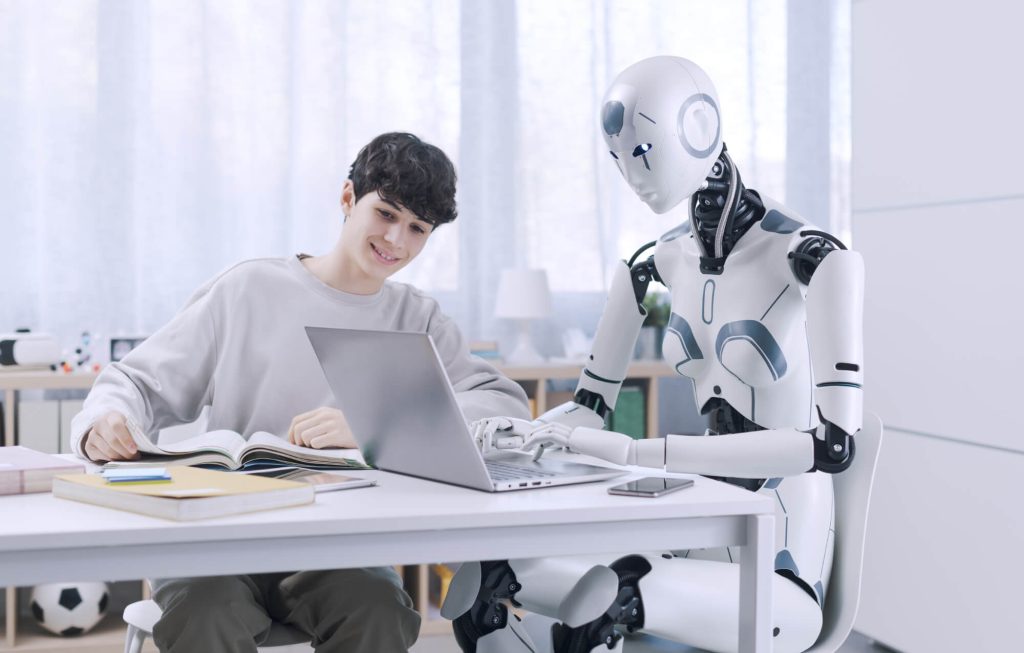
The field of Explainable AI (XAI) is rapidly evolving, driven by the increasing need for transparency and accountability in AI systems. While significant progress has been made, numerous challenges remain, and ongoing research is crucial to unlock XAI’s full potential and address its limitations. Future advancements will significantly impact various sectors, from healthcare and finance to autonomous driving and environmental science.Ongoing research focuses on refining existing XAI techniques and developing novel approaches to explain complex AI models more effectively.
This includes exploring new methods for generating explanations that are not only accurate but also understandable and trustworthy for both technical and non-technical users. The ultimate goal is to create AI systems that are not just powerful but also inherently transparent and ethically sound.
Improving XAI Explanations
Current XAI methods often struggle to provide explanations that are both faithful to the model’s internal workings and easily interpretable by humans. Research is concentrating on developing more robust and nuanced explanation methods. This includes exploring techniques like counterfactual explanations, which illustrate how changing input features would alter the model’s output, and attention mechanisms, which highlight the parts of the input data most influential in the model’s decision.
For instance, in medical diagnosis, counterfactual explanations could show a patient what changes in their health metrics would have led to a different diagnosis.
Addressing the Trade-off Between Accuracy and Explainability
A significant challenge in XAI is the inherent trade-off between model accuracy and the explainability of its decisions. Simpler, more interpretable models often sacrifice accuracy, while highly accurate, complex models are difficult to explain. Researchers are actively investigating methods to mitigate this trade-off, exploring model architectures that prioritize both accuracy and explainability, and developing techniques to extract meaningful explanations from complex models without significantly compromising their performance.
One example is the development of hybrid models combining interpretable and non-interpretable components.
Ensuring the Fairness and Robustness of XAI Systems
Bias in AI systems is a major concern, and XAI must address this issue effectively. Research focuses on developing techniques to detect and mitigate bias in both the data used to train AI models and the explanations generated by XAI systems. Robustness is another critical aspect, ensuring that explanations remain reliable even when faced with noisy or adversarial inputs.
For example, in loan applications, XAI can help identify and rectify biases in the algorithm that might unfairly discriminate against certain demographic groups.
Scaling XAI to Handle Complex Systems
Many real-world AI systems are incredibly complex, involving multiple interconnected components. Scaling XAI techniques to handle such systems presents a significant challenge. Research is exploring methods for decomposing complex systems into smaller, more manageable parts, and developing techniques for aggregating explanations from individual components into a coherent overall explanation. This is particularly important in applications such as autonomous driving, where understanding the reasoning behind a self-driving car’s actions is crucial for safety and trust.
Timeline of Key Milestones in XAI Research and Development
A concise timeline highlighting key advancements is difficult to create due to the multifaceted nature of XAI research, which often involves contributions from various fields. However, we can identify some pivotal moments:
- Early 2000s: Initial research on interpretable machine learning models and rule-based systems.
- Mid-2010s: Growing interest in XAI spurred by the increasing complexity and impact of deep learning models.
- Late 2010s – Present: Development of various XAI techniques (e.g., LIME, SHAP), increased focus on ethical implications, and establishment of XAI as a distinct research area.
- Future (Next 5-10 years): Expected advancements in techniques for explaining complex models, improved methods for handling bias and robustness, and wider adoption of XAI across various sectors.
The Human-AI Collaboration Enabled by XAI
XAI, or Explainable Artificial Intelligence, is revolutionizing the way humans and AI systems interact. By making the decision-making processes of AI transparent and understandable, XAI fosters a collaborative environment where humans and AI can work together more effectively, leveraging the strengths of both. This collaboration is crucial for building trust, ensuring ethical use, and maximizing the potential of AI across various fields.XAI facilitates better collaboration by bridging the communication gap between humans and AI.
Traditional “black box” AI systems often leave users unsure of how a system arrived at a particular outcome. XAI addresses this by providing insights into the reasoning behind AI decisions, allowing human users to understand, validate, and even correct the AI’s output. This transparency allows for a more nuanced and productive partnership, rather than a passive reliance on opaque algorithms.
Benefits of Human-in-the-Loop Systems Enhanced by XAI
Human-in-the-loop systems, where humans actively participate in the AI’s decision-making process, are significantly improved by XAI. The ability to understand the AI’s reasoning allows human experts to identify biases, errors, or limitations in the AI’s approach. This allows for course correction, improving the accuracy and reliability of the AI system while simultaneously enhancing human understanding of the problem domain.
Furthermore, human oversight ensures that the AI remains aligned with ethical guidelines and societal values. This collaborative approach leads to more robust, reliable, and responsible AI systems.
XAI is crucial for building ethical AI, ensuring we understand how these powerful systems make decisions. But developing these transparent AI solutions requires accessible development tools, which is where the advancements in domino app dev the low code and pro code future come into play. This ease of development helps democratize AI creation, paving the way for more responsible and ethical applications of XAI in the future.
Examples of Successful Human-AI Collaborations Enabled by XAI
In medical diagnosis, XAI-powered systems can provide doctors with explanations for their AI-assisted diagnoses. Imagine a system that not only identifies a potential tumor but also highlights the specific image features that led to this conclusion, allowing the doctor to quickly verify the findings and make an informed decision. This collaboration improves diagnostic accuracy and patient care. Similarly, in financial risk assessment, XAI can provide transparent explanations for credit scoring decisions, enabling human analysts to identify and mitigate potential biases within the algorithm, leading to fairer and more equitable lending practices.
These are just two examples demonstrating the powerful synergy between human expertise and AI augmented by XAI.
XAI’s Empowerment of Human Understanding and Control of AI Systems
XAI empowers human users by providing the tools to understand and control AI systems. It allows for a shift from passive reliance on AI to active participation in its operation and improvement. This control is not merely about understanding the output but also about shaping the input and parameters of the AI. By understanding the factors influencing AI decisions, users can refine datasets, adjust algorithms, and ultimately steer the AI toward more desirable outcomes.
This active engagement fosters trust and allows humans to maintain a crucial level of oversight, preventing unintended consequences and ensuring responsible AI development and deployment.
Last Word: Xai Bringing Transparency And Ethics To Artificial Intelligence
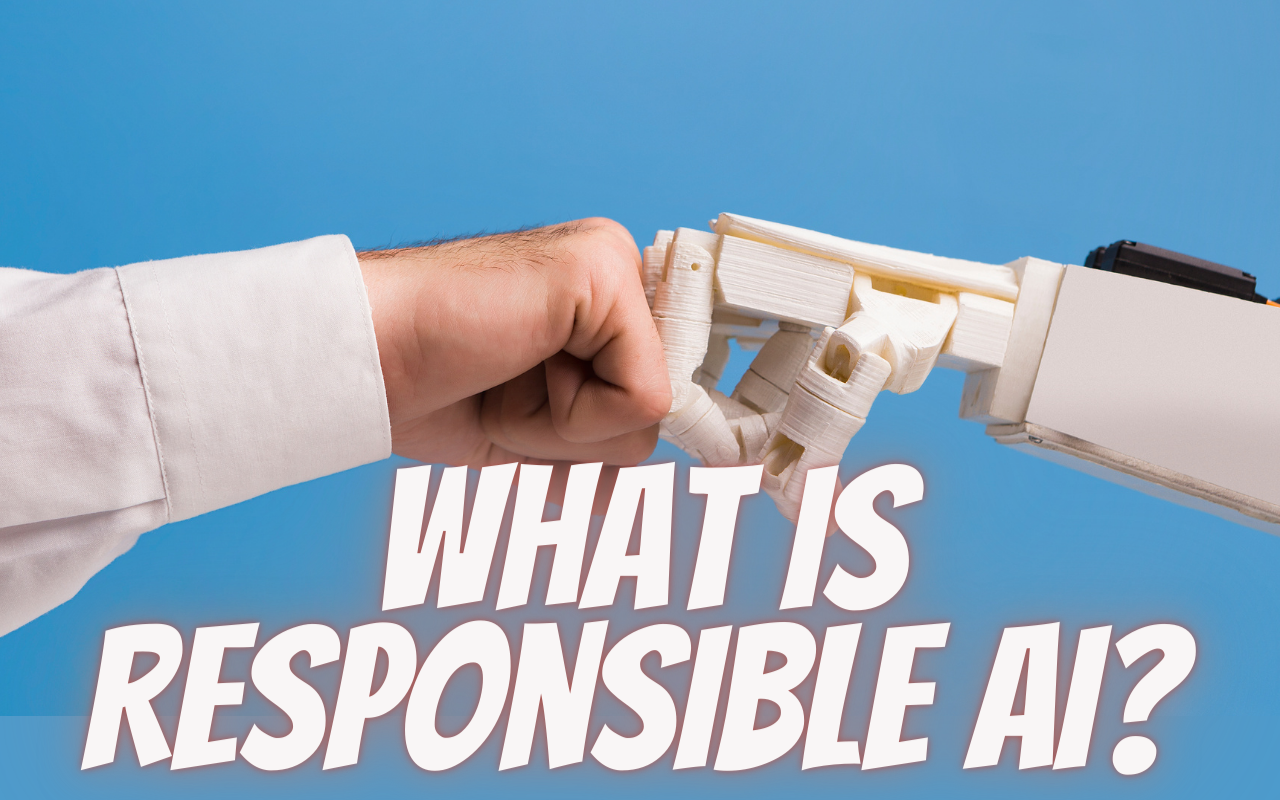
Ultimately, XAI isn’t just about making AI more transparent; it’s about building trust, fostering accountability, and ensuring that these powerful technologies are used ethically and responsibly. By embracing the principles of explainability and interpretability, we can unlock the full potential of AI while mitigating its inherent risks. The journey toward a more ethical and transparent AI future is an ongoing one, but with continued research and collaboration, we can create AI systems that benefit all of humanity.
FAQ Corner
What are the limitations of XAI?
While XAI offers significant advantages, it’s not a silver bullet. Current XAI methods can be computationally expensive, may not always provide complete explanations, and their effectiveness can vary depending on the complexity of the AI system.
How can I learn more about XAI?
There are many resources available! Start with online courses, research papers from leading universities and institutions, and industry publications focusing on AI and machine learning. Many conferences and workshops also focus on XAI.
Is XAI only relevant for large tech companies?
No! While large companies are heavily involved, XAI principles are applicable to organizations of all sizes. Even smaller businesses can benefit from increased transparency and understanding of their AI-driven processes.
What are some examples of XAI failures?
While XAI aims to prevent failures, instances where XAI explanations are misleading or incomplete can still occur. These often highlight the need for ongoing refinement and improvement of XAI techniques.