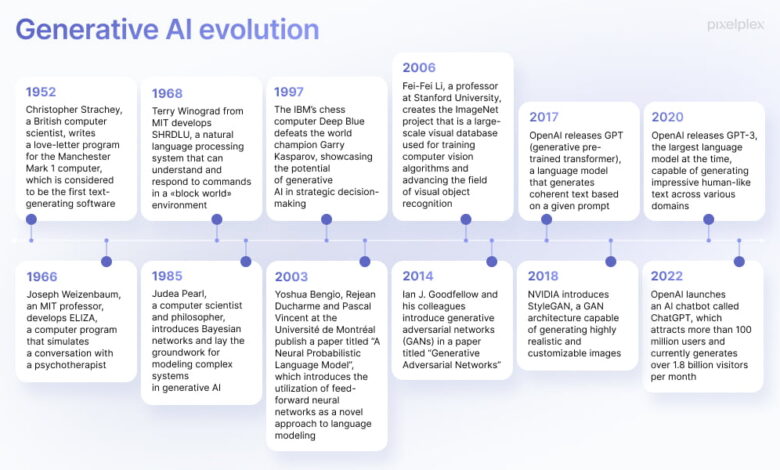
Enterprises Get Ready The Generative AI Revolution Is Coming
Enterprises get ready the generative ai revolution is coming – Enterprises get ready: the generative AI revolution is coming, and it’s poised to transform how we work, innovate, and compete. This isn’t just hype; we’re on the cusp of a technological shift that will fundamentally alter business operations across every sector. From automating tedious tasks to creating entirely new products and services, generative AI promises unprecedented efficiency and growth opportunities.
But with this immense potential comes the need for careful planning, ethical considerations, and a proactive approach to adoption.
This post delves into the practical implications of generative AI for businesses, exploring how various departments can leverage its power, the necessary steps for successful integration, and the crucial ethical considerations that must be addressed. We’ll examine the competitive advantages early adopters will gain, future trends, and the crucial aspects of investment and return on investment (ROI).
The Impact of Generative AI on Enterprise Operations
Generative AI is poised to revolutionize how businesses operate, impacting nearly every department and significantly altering workflows. Its ability to create new content, automate tasks, and analyze data at unprecedented speeds offers a transformative potential for increased efficiency, innovation, and profitability. However, successful implementation requires careful planning and consideration of potential challenges.
Generative AI’s Influence on Enterprise Departments
Generative AI’s impact spans various enterprise departments. In marketing, it can automate content creation for social media, email campaigns, and website copy, leading to increased output and potentially improved engagement. Sales teams can benefit from AI-powered tools that generate personalized sales pitches, predict customer behavior, and streamline lead qualification processes. Customer service can leverage generative AI to provide instant, accurate responses to customer inquiries through chatbots and automated email systems, improving response times and customer satisfaction.
Finally, in R&D, generative AI can accelerate the design and development process by generating novel designs, optimizing existing products, and automating simulations. Specific tasks such as writing marketing materials, generating sales leads, responding to customer inquiries, and designing new products can all be significantly enhanced or automated.
Examples of Generative AI Across Sectors
The transformative power of generative AI is evident across various sectors. The following table illustrates specific applications, benefits, and challenges in different industries:
Sector | Specific Application | Benefits | Potential Challenges |
---|---|---|---|
Finance | Automated report generation, fraud detection, personalized financial advice | Increased efficiency, reduced errors, improved risk management, enhanced customer experience | Data privacy concerns, regulatory compliance, potential for bias in algorithms |
Healthcare | Drug discovery, personalized medicine, medical image analysis, generating patient summaries | Accelerated drug development, improved diagnostics, enhanced treatment plans, reduced administrative burden | Data security and privacy, ethical considerations, algorithm explainability, validation of AI-generated results |
Manufacturing | Predictive maintenance, supply chain optimization, design optimization, generating instructions for robots | Reduced downtime, improved efficiency, optimized resource allocation, enhanced product quality | Integration with existing systems, workforce retraining, potential for job displacement, ensuring AI reliability |
Preparing Enterprises for Generative AI Adoption
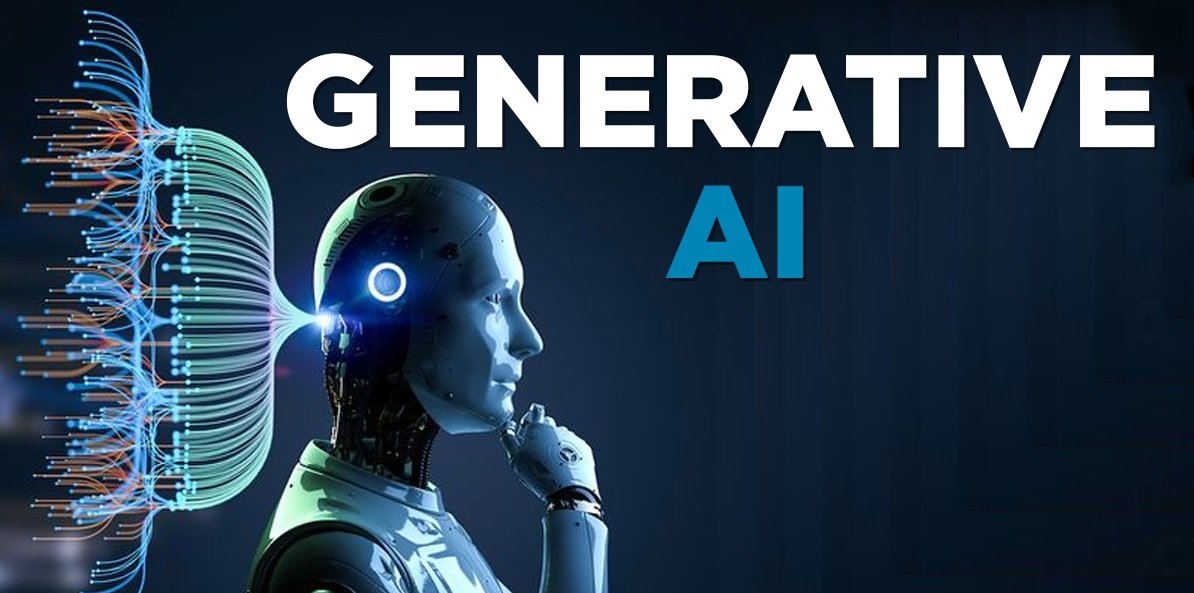
The generative AI revolution isn’t just around the corner; it’s knocking on the door. For enterprises to thrive, not just survive, in this new landscape, proactive and strategic adoption is crucial. This means moving beyond simply understanding the technology’s potential and developing a concrete, actionable plan for integration. This plan needs to encompass technological infrastructure, employee training, and a clear understanding of the potential risks and rewards.Successful integration of generative AI requires a multifaceted approach, moving beyond a simple technological implementation to a holistic transformation of workflows and employee skills.
This isn’t just about adding a new tool; it’s about fundamentally changing how work gets done.
A Step-by-Step Plan for Generative AI Integration
A successful generative AI integration requires a structured approach. This phased implementation minimizes disruption and maximizes the chances of a positive return on investment. The following steps provide a roadmap:
- Assessment and Strategy: Begin with a thorough assessment of current operations, identifying areas where generative AI could provide the greatest value. This might involve analyzing data processing bottlenecks, improving customer service responsiveness, or accelerating product development cycles. A clear strategy outlining goals, metrics for success, and a phased implementation timeline is essential.
- Pilot Projects: Before a full-scale deployment, initiate small-scale pilot projects to test the feasibility and effectiveness of generative AI in specific workflows. This allows for iterative refinement and minimizes the risk of large-scale failures. For example, a marketing team might use a generative AI tool to create variations of ad copy for A/B testing, assessing the impact on click-through rates before expanding its use.
- Integration and Refinement: Based on pilot project results, integrate generative AI into larger workflows. This phase requires careful monitoring and continuous refinement to ensure the technology aligns with evolving business needs and delivers the expected benefits. Regular feedback loops with employees using the tools are critical.
- Scaling and Optimization: Once the technology proves successful, scale its deployment across the enterprise. This may involve integrating generative AI with existing systems and optimizing workflows to fully leverage its capabilities. This phase focuses on maximizing efficiency and return on investment.
Key Technological Infrastructure Requirements
Implementing generative AI solutions demands significant investment in robust technological infrastructure. This goes beyond simply purchasing the necessary software; it requires careful planning and consideration of several key factors.
- High-Performance Computing (HPC): Generative AI models are computationally intensive, requiring powerful hardware capable of handling large datasets and complex computations. This often involves investing in specialized GPUs or cloud-based HPC resources.
- Data Storage and Management: Generative AI models require vast amounts of data for training and operation. Enterprises need robust data storage solutions, along with effective data management and governance strategies to ensure data quality, security, and compliance.
- Network Infrastructure: The ability to quickly and efficiently transfer large datasets is crucial. This requires a high-bandwidth, low-latency network infrastructure capable of supporting the demands of generative AI applications.
- Security and Privacy Measures: Generative AI models often handle sensitive data, requiring robust security measures to protect against unauthorized access and data breaches. This includes implementing encryption, access controls, and regular security audits.
Necessary Skills and Training Programs
The successful adoption of generative AI depends heavily on the skills and knowledge of the workforce. Therefore, comprehensive training programs are crucial.The training should cover both technical aspects and the ethical implications of using the technology. This includes data handling, model interpretation, and the potential biases embedded within the models. Moreover, it’s essential to equip employees with the skills to effectively leverage generative AI tools within their specific roles, ensuring seamless integration into existing workflows.
This might involve workshops, online courses, or mentorship programs tailored to specific job functions and skill levels. For example, marketing professionals might learn how to effectively use generative AI for content creation, while data scientists would focus on model training and optimization. Investing in upskilling and reskilling initiatives is vital to maximize the benefits of generative AI adoption.
Addressing Ethical and Practical Concerns of Generative AI
The rapid advancement of generative AI presents enterprises with incredible opportunities, but also significant ethical and practical challenges. Successfully integrating this technology requires a proactive approach to mitigating risks and ensuring responsible implementation. Ignoring these concerns could lead to reputational damage, legal issues, and ultimately, hinder the potential benefits of this transformative technology.The potential pitfalls of generative AI are multifaceted and require careful consideration.
From biases embedded in training data to concerns around data privacy and intellectual property, navigating this landscape demands a robust ethical framework and practical strategies for risk management.
Bias and Fairness in Generative AI Outputs
Generative AI models are trained on vast datasets, and if these datasets reflect existing societal biases, the AI will likely perpetuate and even amplify those biases in its outputs. For example, a recruitment tool trained on historical data might inadvertently discriminate against certain demographic groups if that data reflects past discriminatory hiring practices. Mitigating this requires careful curation of training data, employing techniques like data augmentation to address imbalances, and implementing ongoing monitoring and auditing of AI outputs for bias.
Regular audits, coupled with diverse teams involved in the AI development process, are crucial for ensuring fairness and equity.
Data Privacy and Security in Generative AI
Generative AI models often require access to large amounts of sensitive data during training and operation. This raises significant concerns about data privacy and security. Breaches could expose confidential information, leading to reputational damage and legal repercussions. Strategies for mitigating these risks include implementing robust data encryption and access control measures, adhering to relevant data privacy regulations (such as GDPR and CCPA), and employing differential privacy techniques to protect individual data while still allowing for model training.
Regular security audits and penetration testing are also essential to identify and address vulnerabilities.
Intellectual Property Rights and Generative AI
The use of generative AI raises complex questions around intellectual property rights. Who owns the copyright to content generated by an AI? What happens if the AI generates content that infringes on existing copyrights? These are critical legal and ethical questions that need careful consideration. Clear guidelines and policies are needed to address ownership, licensing, and potential infringement issues.
Transparency in the AI’s training data and processes can also help to establish accountability and reduce the risk of disputes. For example, clearly defining the terms of use for AI-generated content and establishing clear processes for handling potential copyright infringement claims are crucial steps.
Managing Job Displacement from AI Automation
The automation potential of generative AI inevitably raises concerns about job displacement. While some jobs may be lost to automation, others will be created, requiring a proactive approach to workforce transition. This includes investing in reskilling and upskilling programs to equip employees with the skills needed for new roles in the AI-driven economy. Furthermore, fostering a culture of continuous learning and adaptability within the organization is crucial to navigating this shift successfully.
Partnerships with educational institutions and industry organizations can help create pathways for employees to acquire new skills and adapt to changing job demands. For example, companies like Google and Microsoft are investing heavily in training programs to help employees transition to new roles in the AI field. Another approach is to focus on augmenting human capabilities with AI, rather than simply replacing human workers.
This collaborative approach allows employees to focus on higher-level tasks that require human creativity and critical thinking.
The Competitive Advantage of Generative AI for Enterprises
The rapid advancement of generative AI is creating a paradigm shift in business, offering enterprises a potent tool to gain a significant competitive edge. Early adoption isn’t just about staying ahead of the curve; it’s about fundamentally reshaping operational efficiency, product development, and customer engagement in ways previously unimaginable. Those who embrace this technology strategically will reap substantial rewards, while those who hesitate risk being left behind in a rapidly evolving market.Generative AI offers a multitude of ways to enhance competitive advantage.
By automating complex tasks, improving decision-making processes, and creating personalized experiences, enterprises can significantly boost productivity, reduce costs, and increase revenue. This competitive edge stems from the ability to innovate faster, personalize offerings more effectively, and operate with greater efficiency than competitors lacking comparable AI capabilities. The ability to analyze vast datasets and identify previously unseen patterns also allows for proactive adaptation to market changes and the development of innovative products and services.
Enterprises, buckle up! The generative AI revolution is poised to reshape everything. To stay ahead, you’ll need adaptable development strategies, and that’s where exploring options like domino app dev, the low-code and pro-code future , becomes crucial. Mastering these tools will be key to building the next generation of AI-powered applications, ensuring your business thrives in this rapidly evolving landscape.
Get ready for the AI tsunami!
Innovative Applications of Generative AI Disrupting Traditional Business Models, Enterprises get ready the generative ai revolution is coming
Generative AI is already disrupting numerous industries. In marketing, AI-powered tools are creating hyper-personalized ad campaigns and generating compelling marketing copy at scale, leading to increased engagement and conversion rates. In the design sector, AI is assisting in the creation of innovative product designs and architectural blueprints, shortening development cycles and reducing costs. In customer service, AI-powered chatbots are providing 24/7 support, resolving issues efficiently, and freeing up human agents to focus on more complex tasks.
Furthermore, in drug discovery, generative AI is accelerating the identification of potential drug candidates, significantly reducing the time and cost associated with traditional methods. The applications are vast and constantly expanding, leading to new business models and opportunities.
Case Study: Synergistic Solutions and Generative AI
Synergistic Solutions, a hypothetical mid-sized manufacturing company, faced challenges with slow product development cycles and high design costs. They implemented a generative AI system to assist in the design and prototyping of new components. The AI, trained on a vast dataset of existing designs and material properties, significantly reduced design time by automating repetitive tasks and suggesting innovative solutions.
Furthermore, the AI’s ability to predict component failure rates allowed Synergistic Solutions to optimize designs for durability and reduce material waste. The result was a 30% reduction in product development time, a 20% decrease in manufacturing costs, and a 15% increase in overall revenue within two years of implementation. This success story exemplifies how the strategic adoption of generative AI can drive substantial growth and efficiency gains.
Future Trends and Predictions in Enterprise Generative AI
Generative AI is rapidly evolving, promising to reshape enterprise operations in profound ways. We’re moving beyond the initial hype cycle, and concrete applications are emerging across various sectors. This section explores key technological advancements and emerging applications, while also considering the transformative impact on the future of work.
Key Technological Advancements in Generative AI
Several technological advancements will significantly influence the future of enterprise generative AI. These improvements will lead to more efficient, accurate, and versatile AI systems. We can expect to see greater integration with other technologies, enhancing capabilities and broadening applications.
One crucial area is the development of more efficient and scalable models. Current large language models (LLMs) are computationally expensive to train and deploy. Future advancements in model architecture, training techniques (such as reinforcement learning from human feedback – RLHF), and hardware (specialized AI accelerators) will dramatically reduce these costs, making generative AI accessible to a wider range of enterprises.
For example, research into smaller, more efficient models that retain high performance is actively underway, aiming to overcome the limitations of current large-scale models. Another key advancement will be improved multimodal capabilities. Current models often excel in a single modality (text, image, audio), but future systems will seamlessly integrate multiple modalities, allowing for more complex and nuanced interactions.
Imagine AI systems that can understand and generate content across text, images, and video simultaneously, enabling entirely new applications in areas like product design, marketing, and customer service.
Emerging Applications of Generative AI Across Sectors
Generative AI’s potential is vast, extending across numerous enterprise sectors. The following list highlights some potential applications:
The following applications demonstrate how generative AI is poised to revolutionize various industries. The ability to automate complex tasks, personalize experiences, and generate novel solutions is transforming the way businesses operate.
- Finance: Automated fraud detection, personalized financial advice, and generation of financial reports.
- Healthcare: Drug discovery and development, personalized medicine, and generation of medical reports and summaries.
- Manufacturing: Design optimization, predictive maintenance, and automated quality control.
- Marketing and Sales: Personalized marketing campaigns, content generation, and chatbots for customer service.
- Retail: Personalized product recommendations, virtual try-on experiences, and inventory management.
Transformation of Work and Required Skills
Generative AI will undoubtedly transform the nature of work. While some jobs may be automated, many new roles will emerge requiring different skill sets.
The integration of generative AI will necessitate a shift in the workforce’s skillset. While certain routine tasks will be automated, new opportunities will arise in areas requiring human oversight, creativity, and critical thinking. This transition will require reskilling and upskilling initiatives to ensure a smooth integration of AI into the workplace.
The demand for professionals skilled in prompt engineering, AI ethics, and data interpretation will increase significantly. Additionally, roles focused on AI model training, fine-tuning, and maintenance will become increasingly important. Furthermore, the ability to critically evaluate and interpret AI-generated outputs will be a crucial skill for all employees, ensuring responsible and effective use of this technology. For example, marketing professionals will need to understand how to effectively utilize generative AI for content creation while maintaining brand consistency and ethical standards.
Similarly, data scientists will need to develop expertise in fine-tuning and managing these large models to ensure they perform optimally and ethically within their specific enterprise context.
Investment and Return on Investment (ROI) in Generative AI
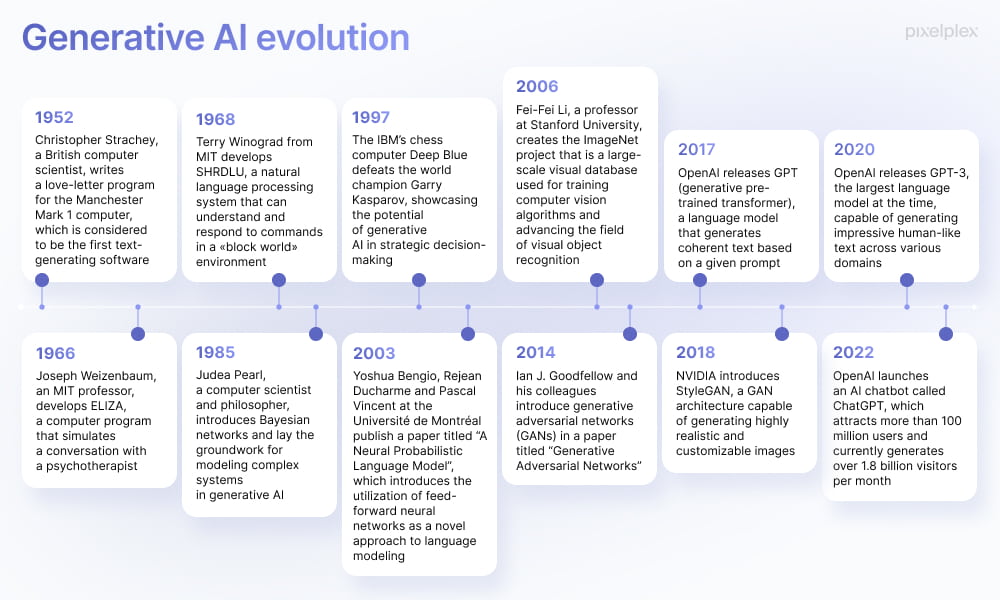
Generative AI offers transformative potential, but its implementation requires careful consideration of costs and potential returns. Understanding the financial implications is crucial for enterprises to make informed decisions and secure the necessary funding. This section explores the various cost factors, provides a hypothetical ROI analysis, and examines different funding models for generative AI initiatives.
Cost Factors Associated with Generative AI Implementation
Implementing generative AI solutions involves a multifaceted cost structure. These costs extend beyond the initial software acquisition and encompass ongoing operational expenses and potential risks. Key cost components include:
- Software Licensing and Acquisition Costs: The cost of purchasing or licensing generative AI software varies significantly depending on the chosen platform, features, and scale of deployment. This can range from subscription fees for cloud-based services to substantial upfront investments for on-premise solutions.
- Hardware Infrastructure Costs: Generative AI models are computationally intensive, requiring robust hardware infrastructure. This includes high-performance computing (HPC) servers, specialized GPUs, and substantial storage capacity. The cost can be substantial, especially for large-scale deployments.
- Data Acquisition and Preparation Costs: High-quality data is the lifeblood of generative AI. Acquiring, cleaning, and preparing this data for model training is a significant undertaking, requiring substantial time and resources. This includes costs associated with data acquisition, data cleaning, labeling, and validation.
- Personnel Costs: Implementing and managing generative AI requires skilled personnel, including data scientists, machine learning engineers, and AI ethicists. Salaries and benefits for these specialized roles can significantly contribute to the overall cost.
- Integration and Maintenance Costs: Integrating generative AI into existing enterprise systems and maintaining the software and infrastructure require ongoing investment. This includes costs associated with system integration, software updates, and ongoing maintenance.
- Training and Development Costs: Employees need training to effectively utilize and understand generative AI tools and their implications. This can include workshops, online courses, and on-the-job training.
- Risk Mitigation Costs: Addressing potential risks associated with generative AI, such as bias, security vulnerabilities, and ethical concerns, requires dedicated resources and investment in mitigation strategies.
Hypothetical Cost-Benefit Analysis of a Generative AI Project
Let’s consider a hypothetical scenario: a large e-commerce company uses generative AI to personalize customer recommendations and automate marketing copy generation.
Cost Category | Estimated Cost (USD) |
---|---|
Software Licensing | $50,000/year |
Hardware Infrastructure | $100,000 (one-time investment) |
Data Preparation | $20,000 |
Personnel (1 data scientist, 1 engineer) | $200,000/year |
Integration & Maintenance | $30,000/year |
Training | $10,000 |
Total Estimated Cost (Year 1) | $360,000 |
Total Estimated Cost (Year 2 onwards) | $260,000 |
Benefit Category | Estimated Benefit (USD) |
---|---|
Increased Sales (10% increase) | $500,000/year |
Reduced Marketing Costs (15% reduction) | $75,000/year |
Improved Customer Satisfaction | (Difficult to quantify directly, but contributes to increased loyalty and reduced churn) |
In this hypothetical scenario, the ROI in year 1 is approximately 28% (($500,000 + $75,000) / $360,000 -1) and increases significantly in subsequent years. This calculation doesn’t include the long-term benefits of improved customer satisfaction and brand loyalty.
Funding Models for Generative AI Initiatives
Enterprises can explore several funding models to finance their generative AI initiatives:
- Capital Expenditure (CapEx): This involves upfront investment in hardware and software, often financed through internal budgets or loans.
- Operational Expenditure (OpEx): This model focuses on recurring subscription fees for cloud-based services, reducing upfront investment but leading to ongoing expenses.
- Venture Capital and Private Equity: For ambitious projects, securing external funding from venture capitalists or private equity firms can provide significant capital.
- Government Grants and Subsidies: Several governments offer grants and subsidies to support AI research and development, potentially reducing the financial burden on enterprises.
- Internal Funding Reallocation: Companies may reallocate funds from other projects or departments to support generative AI initiatives.
Final Conclusion: Enterprises Get Ready The Generative Ai Revolution Is Coming
The generative AI revolution isn’t just around the corner; it’s already knocking on the door. Businesses that proactively embrace this technology, strategically planning for integration and addressing ethical concerns, will be best positioned to thrive in this new era. Ignoring this technological leap is not an option; it’s a strategic risk. By understanding the potential benefits, mitigating the challenges, and investing wisely, enterprises can unlock the transformative power of generative AI and secure a competitive advantage in the years to come.
The future of business is intelligent, and that future is now.
Question Bank
What are the biggest risks associated with implementing generative AI?
The biggest risks include data breaches, bias in AI outputs leading to unfair or discriminatory outcomes, and the potential for misuse of generated content (e.g., deepfakes).
How can my company ensure ethical use of generative AI?
Establish clear ethical guidelines, implement robust data privacy measures, regularly audit AI systems for bias, and prioritize transparency in how AI is used.
What kind of training will my employees need?
Training will vary depending on the specific AI tools used, but generally includes data literacy, understanding AI limitations, and responsible AI practices.
What is the typical ROI for generative AI projects?
ROI varies significantly depending on the specific application and implementation. While some projects show immediate returns, others require longer-term investment to see significant benefits.