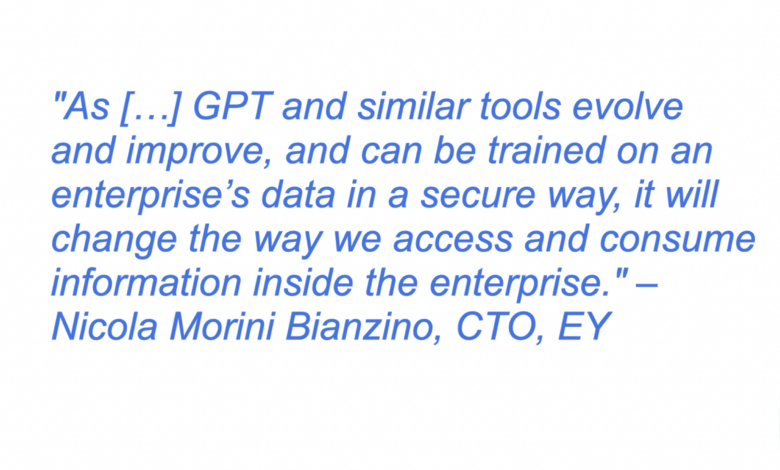
Reflecting on Generative AI One Year Later
Reflecting on generative ai one year post chatgpt launch – Reflecting on generative AI one year post-launch reveals a landscape dramatically reshaped. What began as a novelty has rapidly evolved into a powerful force across numerous industries, sparking both excitement and apprehension. This post delves into the key advancements, societal impacts, ethical considerations, and future predictions surrounding this transformative technology.
From initial models with limited capabilities to the sophisticated systems we see today, the progress has been astounding. We’ll explore this evolution, examining the impact on creative fields, business practices, and the very fabric of society. We’ll also tackle the crucial ethical questions that arise with such powerful tools, looking at bias, intellectual property, and responsible development.
The Evolution of Generative AI
One year after Kami’s launch, the field of generative AI has undergone a dramatic transformation. The initial excitement has solidified into a period of rapid development, marked by significant advancements in model architecture, training methodologies, and overall capabilities. This evolution has not only expanded the potential applications of generative AI but also highlighted its limitations and ethical considerations.
Key Advancements in Generative AI Technology
Since the initial release of Kami, we’ve seen a flurry of improvements across the board. Larger models with increased parameter counts have become commonplace, leading to demonstrably improved performance in various tasks. This increase in size, however, isn’t the only factor. Researchers have also focused on refining model architectures, exploring alternatives to the transformer model and experimenting with hybrid approaches that combine different strengths.
Furthermore, advancements in training techniques, such as the incorporation of reinforcement learning from human feedback (RLHF), have significantly enhanced the quality and coherence of generated outputs. The move towards more efficient training methods, addressing the substantial computational cost associated with large language models, has also been a key area of progress.
Comparison of Early and Current State-of-the-Art Models
Early models, like the initial versions of GPT-3, often struggled with factual accuracy, logical consistency, and the generation of truly creative text. They were prone to producing outputs that were nonsensical or exhibited biases present in their training data. Current state-of-the-art models, however, demonstrate a significant improvement in these areas. They exhibit a better understanding of context, can generate more nuanced and coherent text, and are less susceptible to producing hallucinations or biased outputs (though biases remain a significant concern).
This improvement is a direct result of larger datasets, more sophisticated training techniques, and refined model architectures. For example, the ability to reason and follow complex instructions has improved dramatically, moving beyond simple text generation to more complex tasks like code generation and problem-solving.
Impact of Improved Training Data and Algorithms
The advancements in generative AI are intrinsically linked to improvements in both training data and algorithms. The sheer volume of data used to train these models has increased exponentially. Access to high-quality, diverse datasets, including text, code, and images, has been crucial. Furthermore, the careful curation and cleaning of this data have minimized the impact of biases and inaccuracies.
Simultaneously, algorithmic improvements have focused on enhancing the efficiency and effectiveness of the training process. This includes innovations in attention mechanisms, optimization algorithms, and techniques for mitigating overfitting. The combined effect of better data and algorithms has resulted in models that are not only larger but also significantly more capable and robust.
Comparison of Three Key Generative AI Models
Model | Strengths | Weaknesses | Notable Features |
---|---|---|---|
GPT-4 | Improved reasoning, nuanced text generation, multilingual capabilities | Computational cost, potential for bias, still susceptible to hallucinations | Advanced reasoning abilities, multimodal capabilities (in some versions) |
LaMDA | Strong conversational abilities, engaging dialogue generation | Limited access, potential for generating inappropriate responses | Focus on conversational AI, emphasis on safety and ethical considerations |
Stable Diffusion | High-quality image generation, diverse artistic styles, relatively low computational cost | Potential for misuse, ethical concerns regarding copyright and intellectual property | Open-source nature, allows for community contributions and customization |
Impact on Various Industries
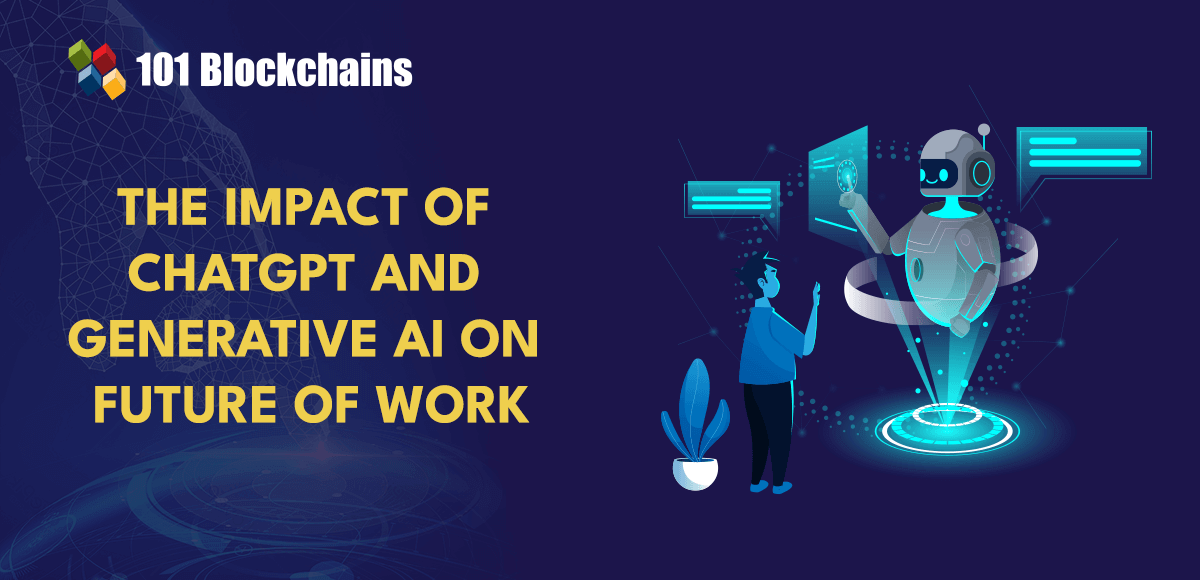
Generative AI’s rapid advancement in the past year has sent ripples across numerous sectors, fundamentally altering workflows and creating both exciting opportunities and significant challenges. Its impact is far-reaching, impacting not only how we create content but also how businesses operate and compete. This section will explore the transformative effects of generative AI across various industries, highlighting both its successes and its potential disruptions.
Generative AI in Creative Industries, Reflecting on generative ai one year post chatgpt launch
The creative industries – art, music, writing – have experienced a dramatic shift with the advent of generative AI. Tools capable of generating images, composing music, and crafting written content have democratized creative processes, allowing individuals with limited technical skills to produce sophisticated outputs. For example, Midjourney and Dall-E 2 have empowered artists to explore new visual styles and generate unique artwork based on text prompts, while platforms like Amper Music and Jukebox offer composers tools for rapid prototyping and experimentation.
In writing, AI tools assist with everything from generating initial drafts to refining existing text, improving efficiency and potentially increasing productivity. However, this has also sparked debates about authorship, copyright, and the potential displacement of human creatives. The long-term impact on the value and nature of creative work remains a subject of ongoing discussion and analysis.
Generative AI in Business and Marketing
Businesses are rapidly adopting generative AI to streamline operations and enhance marketing efforts. In marketing, AI is used to create personalized ad copy, generate engaging social media content, and even develop entirely new marketing campaigns. For example, companies are using AI to personalize email marketing campaigns, improving open and click-through rates. In business operations, generative AI can automate tasks such as report generation, data analysis, and customer service interactions, freeing up human employees to focus on more strategic initiatives.
Companies like Jasper and Copy.ai have become popular choices for businesses seeking to leverage the power of AI for content creation and marketing automation. The successful application of generative AI in these areas hinges on careful integration and a focus on using the technology to augment, not replace, human expertise.
Disruptions and Challenges in Specific Sectors
The adoption of generative AI is not without its challenges. Some sectors face significant disruptions as the technology matures. For example, the customer service industry is undergoing a transformation, with AI-powered chatbots handling a growing proportion of customer inquiries. This can lead to job displacement for human customer service representatives if not managed carefully. Similarly, the legal profession is grappling with the implications of AI-powered legal research and document drafting tools, raising concerns about accuracy, ethical considerations, and the potential for bias in algorithms.
The media industry also faces challenges, as generative AI can produce realistic fake news and deepfakes, potentially undermining public trust and the integrity of information.
Case Study: The Publishing Industry
Let’s consider the publishing industry. Generative AI offers significant potential benefits: authors can use AI tools to overcome writer’s block, editors can leverage AI for faster proofreading and editing, and publishers can use AI to personalize marketing campaigns and identify potential bestsellers. This could lead to increased efficiency and potentially lower production costs. However, the potential for plagiarism, the ethical implications of AI-generated content, and the potential displacement of human editors and writers pose significant challenges.
A scenario where AI generates large volumes of low-quality content could flood the market, devaluing the work of human authors and potentially harming the overall quality of published materials. Conversely, successful integration could lead to a more efficient and innovative publishing ecosystem, with authors and publishers working collaboratively with AI to produce high-quality content and reach wider audiences.
Ethical and Societal Considerations
The rapid advancement of generative AI, while offering incredible potential, necessitates a careful examination of its ethical and societal implications. The power to create realistic text, images, and even videos raises serious concerns about misuse, bias, and the very fabric of intellectual property. Addressing these challenges proactively is crucial to harnessing the benefits of this technology responsibly.
Bias in Generative AI Models and Potential for Misuse
Generative AI models are trained on massive datasets, which often reflect existing societal biases. This can lead to AI systems perpetuating and even amplifying harmful stereotypes related to gender, race, religion, and other sensitive attributes. For example, a language model trained on a dataset with predominantly male voices might generate text that underrepresents or misrepresents women. Furthermore, the potential for misuse is significant.
Reflecting on generative AI a year after ChatGPT’s launch, it’s amazing how quickly things have changed. The speed of innovation is mind-boggling, and it makes me wonder about the future of development. This is particularly relevant when considering how platforms like Domino are evolving, as you can read more about in this insightful article on domino app dev, the low-code and pro-code future , which explores the impact of these tools.
Ultimately, the intersection of AI and rapid app development will redefine how we build software in the coming years.
Deepfakes, generated by AI, can be used to create convincing but false videos, potentially damaging reputations or influencing elections. The ease of generating realistic but fabricated content poses a threat to trust and the integrity of information. Malicious actors could leverage this technology to spread disinformation or engage in other forms of harmful activity.
Implications of Generative AI for Intellectual Property Rights and Copyright
The ability of generative AI to create original content raises complex questions about intellectual property rights and copyright. Who owns the copyright to an image or a piece of text generated by an AI? Is it the user who prompted the AI, the developers who created the AI model, or perhaps no one? Existing copyright laws are struggling to keep pace with this rapidly evolving technology.
Consider the case of an artist using an AI tool to generate artwork. While the artist may have creative input, the AI also plays a significant role in the creation process. Determining the appropriate ownership and licensing rights in such scenarios is a significant challenge requiring legal and ethical frameworks to be updated. Furthermore, the ease of generating derivative works raises concerns about copyright infringement.
Societal Impacts of Widespread Generative AI Adoption
The widespread adoption of generative AI will undoubtedly have profound societal impacts, both positive and negative. On the positive side, generative AI has the potential to revolutionize various industries, increasing productivity and efficiency. For example, AI-powered tools can automate tedious tasks, freeing up human workers to focus on more creative and strategic endeavors. In healthcare, AI could assist in drug discovery and personalized medicine.
However, there are also potential negative impacts. Job displacement due to automation is a significant concern. As AI systems become more capable, they may replace human workers in certain roles, leading to unemployment and economic inequality. The spread of misinformation and deepfakes also poses a serious threat to social cohesion and trust. The potential for algorithmic bias to further marginalize already disadvantaged groups is another significant concern.
Potential Solutions to Mitigate the Ethical Challenges Posed by Generative AI
Addressing the ethical challenges posed by generative AI requires a multi-faceted approach. Developing more robust and transparent AI models that are less prone to bias is crucial. This involves carefully curating training datasets and incorporating techniques to detect and mitigate bias. Establishing clear legal and ethical guidelines for the use of generative AI is also necessary. This could involve updating copyright laws to address the unique challenges posed by AI-generated content and developing regulations to prevent the misuse of generative AI for malicious purposes.
Furthermore, promoting AI literacy and critical thinking skills among the public is essential to enable individuals to discern between genuine and AI-generated content. Encouraging responsible innovation and collaboration between researchers, policymakers, and industry stakeholders is vital to ensuring that generative AI is developed and deployed in a way that benefits society as a whole. Open-source initiatives and public discourse around the ethical implications of AI can play a significant role in achieving this.
Future Trends and Predictions: Reflecting On Generative Ai One Year Post Chatgpt Launch
Generative AI has exploded onto the scene in the past year, and its trajectory over the next five years promises to be nothing short of transformative. We’re moving beyond the initial wow factor and into a phase of refinement, integration, and increasingly sophisticated applications across diverse sectors. This section will explore the likely developmental paths, potential collaborations with other technologies, and anticipate both the exciting breakthroughs and the inevitable challenges that lie ahead.
Generative AI Model Development Trajectories
The next five years will likely witness a significant shift in the focus of generative AI development. We’ll see a move away from simply increasing model size towards a greater emphasis on efficiency, controllability, and specialized models tailored for specific tasks. This includes advancements in areas like prompt engineering, allowing for more nuanced and precise control over the generated output.
We can expect to see more models trained on diverse and specialized datasets, leading to more accurate and less biased results in specific domains, such as medical diagnosis or legal research. Furthermore, research into more efficient training methods, such as improved optimization algorithms and hardware acceleration, will be crucial for making generative AI more accessible and sustainable. For example, the development of more efficient transformer architectures, potentially moving beyond the current limitations of scaling, will be key.
Integration with Other Emerging Technologies
Generative AI’s potential is greatly amplified when combined with other emerging technologies. The synergy between generative AI and the metaverse, for instance, could revolutionize virtual world creation, providing dynamic and responsive environments tailored to individual user experiences. Imagine AI generating personalized avatars, interactive narratives, and even entire virtual worlds on demand. Similarly, the integration with robotics could lead to more autonomous and adaptable robots capable of creative problem-solving and complex interactions with humans.
Consider a robot capable of generating unique solutions to assembly line challenges, adapting to unforeseen circumstances based on its generative AI capabilities. The combination of generative AI with quantum computing could lead to exponential leaps in model capabilities, enabling the creation of significantly more complex and nuanced models. This could lead to breakthroughs in areas like drug discovery and materials science.
Potential Breakthroughs and Challenges
Several potential breakthroughs are on the horizon. One is the development of truly multimodal models capable of seamlessly integrating text, images, audio, and video. Imagine an AI system that can understand and generate content across all these modalities, allowing for the creation of incredibly rich and immersive experiences. However, significant challenges remain. The ethical implications of increasingly powerful generative AI models need careful consideration.
Concerns around bias, misinformation, and the potential for malicious use must be addressed proactively. Furthermore, ensuring the responsible development and deployment of these technologies requires a collaborative effort between researchers, policymakers, and the public. For example, developing robust methods for detecting AI-generated content (deepfakes, etc.) will be critical to mitigate potential harm. Another major challenge will be managing the vast computational resources required to train and run these advanced models, necessitating innovations in both hardware and software.
Anticipated Evolution of Generative AI Capabilities
Imagine a graph charting the evolution of generative AI capabilities over the next five years. The X-axis represents time, and the Y-axis represents capabilities, measured by a composite score incorporating factors like accuracy, creativity, efficiency, and the range of modalities handled. Initially, the curve shows a relatively steep incline, reflecting the rapid advancements seen in the past year.
However, the curve gradually begins to flatten as the low-hanging fruit are picked. Specific breakthroughs, such as the development of truly multimodal models or significant advancements in efficiency, would be represented by sharp increases in the curve’s slope. Challenges like ethical concerns and resource limitations would manifest as periods of slower growth or even temporary plateaus. The overall trend, however, would show a consistent upward trajectory, indicating a steady improvement in generative AI capabilities, albeit with fluctuations reflecting the challenges and breakthroughs along the way.
This is not a linear progression; we expect bursts of innovation interspersed with periods of consolidation and refinement.
Accessibility and Democratization of Generative AI
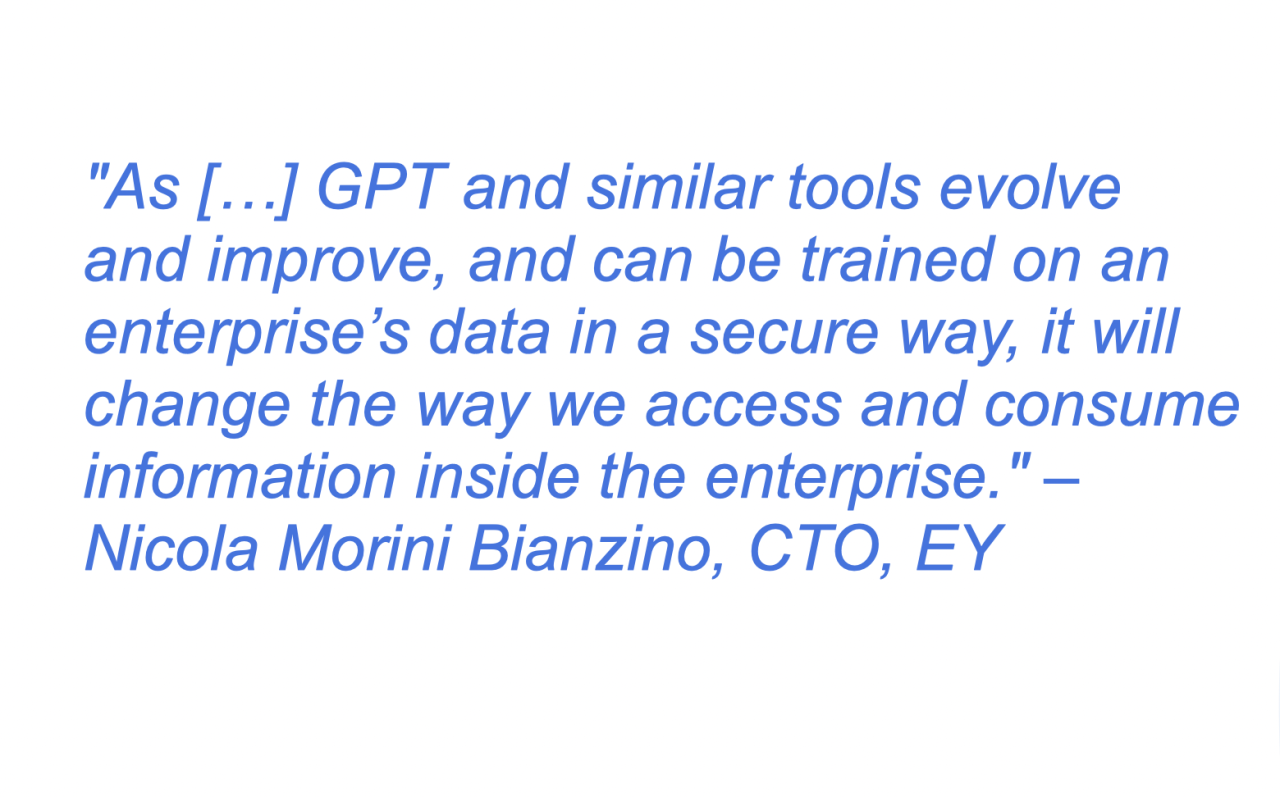
The launch of Kami marked a pivotal moment, bringing the power of generative AI to a wider audience than ever before. However, a year on, the question of true accessibility and democratization remains complex. While tools are increasingly available, significant hurdles still prevent equitable access for all. This section explores the current state of accessibility, identifies key barriers, and proposes strategies for broader and responsible adoption.Generative AI tools have become significantly more accessible to the general public in the past year.
The release of user-friendly interfaces like Kami’s conversational format lowered the technical barrier to entry for many. Open-source models and APIs have also empowered developers and researchers to build upon existing technology, leading to a proliferation of new applications and services. This increased availability has fostered experimentation and innovation across diverse fields, from creative writing and art to software development and scientific research.
However, this progress is unevenly distributed.
Barriers to Broader Adoption of Generative AI
Several factors limit broader adoption and use of generative AI technologies. Cost remains a significant barrier, with access to powerful models and sufficient computing resources often prohibitive for individuals and smaller organizations. Digital literacy is another crucial factor; understanding the capabilities and limitations of generative AI, as well as responsible usage practices, requires a certain level of technical proficiency.
Furthermore, language barriers prevent equitable access for those who don’t speak the dominant languages supported by most current models. Finally, concerns about data privacy and security, particularly regarding the input and output of these systems, can deter potential users. For instance, smaller businesses might hesitate to use AI tools due to worries about data breaches or the potential for misuse of sensitive information.
Strategies for Promoting Wider Accessibility and Responsible Use
Promoting wider accessibility necessitates a multi-pronged approach. Firstly, initiatives to reduce the cost of access are vital. This could involve the development of more efficient and less resource-intensive models, as well as the creation of community-supported computing resources. Secondly, improving digital literacy through educational programs and resources is essential. This includes providing accessible tutorials, workshops, and online courses that explain the technology’s capabilities, limitations, and ethical implications in a clear and engaging manner.
Thirdly, developing multilingual models and interfaces is crucial for global accessibility. Finally, establishing clear guidelines and best practices for responsible AI use is essential to address privacy and security concerns and build trust. For example, clear guidelines on data usage and security protocols could be developed and promoted through public awareness campaigns.
Improving User Interfaces and Educational Resources
Improved user interfaces are key to democratizing access. Intuitive and user-friendly interfaces, such as drag-and-drop interfaces for image generation or simple natural language prompts for text generation, can significantly lower the barrier to entry for non-technical users. Similarly, educational resources should be tailored to different levels of technical expertise, ranging from introductory guides for beginners to advanced tutorials for experienced users.
Interactive tutorials, gamified learning experiences, and readily available online resources can help demystify the technology and foster a better understanding of its potential and limitations. For example, a simple drag-and-drop interface for creating marketing materials using AI could make the technology accessible to small business owners with limited technical skills. Similarly, interactive tutorials that guide users through the process of generating different types of creative content could help beginners learn and experiment with the technology safely and effectively.
Concluding Remarks
One year on, the generative AI revolution is far from over. The technology continues to advance at a breathtaking pace, promising both incredible opportunities and significant challenges. Navigating this new landscape requires careful consideration of the ethical implications, proactive mitigation of potential risks, and a commitment to responsible innovation. The future is unwritten, but one thing is certain: generative AI will continue to shape our world in profound ways.
Key Questions Answered
What are the biggest limitations of current generative AI models?
Current limitations include biases in training data leading to unfair or inaccurate outputs, a lack of true understanding or reasoning, and potential for misuse in creating deepfakes or spreading misinformation.
How can I learn more about generative AI?
Numerous online courses, tutorials, and research papers are available. Start with introductory materials and then delve into more specialized areas based on your interests.
What jobs are most at risk from generative AI?
Jobs involving repetitive tasks, data entry, and content creation are most vulnerable. However, new roles focused on AI development, maintenance, and ethical oversight are also emerging.
Is generative AI truly creative?
That’s a complex question. While generative AI can produce impressive outputs, it doesn’t possess genuine human creativity, originality, or emotional intelligence. It leverages patterns and data to create novel combinations, but the underlying “creative spark” remains a human attribute.